diff --git a/Axon.Activations.html b/Axon.Activations.html index 3e1d7aa0..29afd628 100644 --- a/Axon.Activations.html +++ b/Axon.Activations.html @@ -115,19 +115,19 @@
Activation functions.
Activation functions are element-wise, (typically) non-linear functions called on the output of another layer, such as a dense layer:
x
-|> dense(weight, bias)
-|> relu()
Activation functions output the "activation" or how active +|> dense(weight, bias) +|> relu()
Activation functions output the "activation" or how active a given layer's neurons are in learning a representation of the data-generating distribution.
Some activations are commonly used as output activations. For
example softmax
is often used as the output in multiclass
classification problems because it returns a categorical
-probability distribution:
iex> Axon.Activations.softmax(Nx.tensor([[1, 2, 3]], type: {:f, 32}))
-#Nx.Tensor<
- f32[1][3]
- [
- [0.09003057330846786, 0.2447284758090973, 0.6652409434318542]
- ]
->
Other activations such as tanh
or sigmoid
are used because
+probability distribution:
iex> Axon.Activations.softmax(Nx.tensor([[1, 2, 3]], type: {:f, 32}))
+#Nx.Tensor<
+ f32[1][3]
+ [
+ [0.09003057330846786, 0.2447284758090973, 0.6652409434318542]
+ ]
+>
Other activations such as tanh
or sigmoid
are used because
they have desirable properties, such as keeping the output
tensor constrained within a certain range.
Generally, the choice of activation function is arbitrary; although some activations work better than others in certain @@ -421,26 +421,26 @@
iex> Axon.Activations.celu(Nx.tensor([-3.0, -2.0, -1.0, 0.0, 1.0, 2.0, 3.0]))
-#Nx.Tensor<
- f32[7]
- [-0.9502129554748535, -0.8646647334098816, -0.6321205496788025, 0.0, 1.0, 2.0, 3.0]
->
-
-iex> Axon.Activations.celu(Nx.tensor([[-1.0, -2.0, -3.0], [1.0, 2.0, 3.0]], type: {:bf, 16}))
-#Nx.Tensor<
- bf16[2][3]
- [
- [-0.62890625, -0.86328125, -0.94921875],
- [1.0, 2.0, 3.0]
- ]
->
iex> Axon.Activations.celu(Nx.tensor([-3.0, -2.0, -1.0, 0.0, 1.0, 2.0, 3.0]))
+#Nx.Tensor<
+ f32[7]
+ [-0.9502129554748535, -0.8646647334098816, -0.6321205496788025, 0.0, 1.0, 2.0, 3.0]
+>
+
+iex> Axon.Activations.celu(Nx.tensor([[-1.0, -2.0, -3.0], [1.0, 2.0, 3.0]], type: {:bf, 16}))
+#Nx.Tensor<
+ bf16[2][3]
+ [
+ [-0.62890625, -0.86328125, -0.94921875],
+ [1.0, 2.0, 3.0]
+ ]
+>
error-cases
Error casesiex> Axon.Activations.celu(Nx.tensor([0.0, 1.0, 2.0], type: {:f, 32}), alpha: 0.0)
+iex> Axon.Activations.celu(Nx.tensor([0.0, 1.0, 2.0], type: {:f, 32}), alpha: 0.0)
** (ArgumentError) :alpha must be non-zero in CELU activation
references
@@ -483,20 +483,20 @@ elu(x, opts \\ [])
Examples
-iex> Axon.Activations.elu(Nx.tensor([-3.0, -2.0, -1.0, 0.0, 1.0, 2.0, 3.0]))
-#Nx.Tensor<
- f32[7]
- [-0.9502129554748535, -0.8646647334098816, -0.6321205496788025, 0.0, 1.0, 2.0, 3.0]
->
-
-iex> Axon.Activations.elu(Nx.tensor([[-1.0, -2.0, -3.0], [1.0, 2.0, 3.0]], type: {:bf, 16}))
-#Nx.Tensor<
- bf16[2][3]
- [
- [-0.62890625, -0.86328125, -0.94921875],
- [1.0, 2.0, 3.0]
- ]
->
+iex> Axon.Activations.elu(Nx.tensor([-3.0, -2.0, -1.0, 0.0, 1.0, 2.0, 3.0]))
+#Nx.Tensor<
+ f32[7]
+ [-0.9502129554748535, -0.8646647334098816, -0.6321205496788025, 0.0, 1.0, 2.0, 3.0]
+>
+
+iex> Axon.Activations.elu(Nx.tensor([[-1.0, -2.0, -3.0], [1.0, 2.0, 3.0]], type: {:bf, 16}))
+#Nx.Tensor<
+ bf16[2][3]
+ [
+ [-0.62890625, -0.86328125, -0.94921875],
+ [1.0, 2.0, 3.0]
+ ]
+>
references
@@ -530,20 +530,20 @@ exp(x)
Examples
-iex> Axon.Activations.exp(Nx.tensor([-3.0, -2.0, -1.0, 0.0, 1.0, 2.0, 3.0], names: [:data]))
-#Nx.Tensor<
- f32[data: 7]
- [0.049787066876888275, 0.1353352814912796, 0.3678794503211975, 1.0, 2.7182817459106445, 7.389056205749512, 20.08553695678711]
->
-
-iex> Axon.Activations.exp(Nx.tensor([[-1.0, -2.0, -3.0], [1.0, 2.0, 3.0]], type: {:bf, 16}, names: [:batch, :data]))
-#Nx.Tensor<
- bf16[batch: 2][data: 3]
- [
- [0.3671875, 0.134765625, 0.049560546875],
- [2.703125, 7.375, 20.0]
- ]
->
+iex> Axon.Activations.exp(Nx.tensor([-3.0, -2.0, -1.0, 0.0, 1.0, 2.0, 3.0], names: [:data]))
+#Nx.Tensor<
+ f32[data: 7]
+ [0.049787066876888275, 0.1353352814912796, 0.3678794503211975, 1.0, 2.7182817459106445, 7.389056205749512, 20.08553695678711]
+>
+
+iex> Axon.Activations.exp(Nx.tensor([[-1.0, -2.0, -3.0], [1.0, 2.0, 3.0]], type: {:bf, 16}, names: [:batch, :data]))
+#Nx.Tensor<
+ bf16[batch: 2][data: 3]
+ [
+ [0.3671875, 0.134765625, 0.049560546875],
+ [2.703125, 7.375, 20.0]
+ ]
+>
@@ -571,20 +571,20 @@ gelu(x)
Examples
-iex> Axon.Activations.gelu(Nx.tensor([-3.0, -2.0, -1.0, 0.0, 1.0, 2.0, 3.0], names: [:data]))
-#Nx.Tensor<
- f32[data: 7]
- [-0.0040496885776519775, -0.04550027847290039, -0.15865525603294373, 0.0, 0.8413447141647339, 1.9544997215270996, 2.995950222015381]
->
-
-iex> Axon.Activations.gelu(Nx.tensor([[-1.0, -2.0, -3.0], [1.0, 2.0, 3.0]], type: {:bf, 16}, names: [:batch, :data]))
-#Nx.Tensor<
- bf16[batch: 2][data: 3]
- [
- [-0.16015625, -0.046875, -0.005859375],
- [0.83984375, 1.953125, 2.984375]
- ]
->
+iex> Axon.Activations.gelu(Nx.tensor([-3.0, -2.0, -1.0, 0.0, 1.0, 2.0, 3.0], names: [:data]))
+#Nx.Tensor<
+ f32[data: 7]
+ [-0.0040496885776519775, -0.04550027847290039, -0.15865525603294373, 0.0, 0.8413447141647339, 1.9544997215270996, 2.995950222015381]
+>
+
+iex> Axon.Activations.gelu(Nx.tensor([[-1.0, -2.0, -3.0], [1.0, 2.0, 3.0]], type: {:bf, 16}, names: [:batch, :data]))
+#Nx.Tensor<
+ bf16[batch: 2][data: 3]
+ [
+ [-0.16015625, -0.046875, -0.005859375],
+ [0.83984375, 1.953125, 2.984375]
+ ]
+>
references
@@ -620,20 +620,20 @@ hard_sigmoid(x, opts \\ [])
Examples
-iex> Axon.Activations.hard_sigmoid(Nx.tensor([-3.0, -2.0, -1.0, 0.0, 1.0, 2.0, 3.0], names: [:data]))
-#Nx.Tensor<
- f32[data: 7]
- [0.0, 0.0, 0.0, 0.20000000298023224, 0.4000000059604645, 0.6000000238418579, 0.800000011920929]
->
-
-iex> Axon.Activations.hard_sigmoid(Nx.tensor([[-1.0, -2.0, -3.0], [1.0, 2.0, 3.0]], type: {:bf, 16}, names: [:batch, :data]))
-#Nx.Tensor<
- bf16[batch: 2][data: 3]
- [
- [7.781982421875e-4, 0.0, 0.0],
- [0.3984375, 0.59765625, 0.796875]
- ]
->
+iex> Axon.Activations.hard_sigmoid(Nx.tensor([-3.0, -2.0, -1.0, 0.0, 1.0, 2.0, 3.0], names: [:data]))
+#Nx.Tensor<
+ f32[data: 7]
+ [0.0, 0.0, 0.0, 0.20000000298023224, 0.4000000059604645, 0.6000000238418579, 0.800000011920929]
+>
+
+iex> Axon.Activations.hard_sigmoid(Nx.tensor([[-1.0, -2.0, -3.0], [1.0, 2.0, 3.0]], type: {:bf, 16}, names: [:batch, :data]))
+#Nx.Tensor<
+ bf16[batch: 2][data: 3]
+ [
+ [7.781982421875e-4, 0.0, 0.0],
+ [0.3984375, 0.59765625, 0.796875]
+ ]
+>
@@ -665,20 +665,20 @@ hard_silu(x, opts \\ [])
Examples
-iex> Axon.Activations.hard_silu(Nx.tensor([-3.0, -2.0, -1.0, 0.0, 1.0, 2.0, 3.0], names: [:data]))
-#Nx.Tensor<
- f32[data: 7]
- [-0.0, -0.0, -0.0, 0.0, 0.4000000059604645, 1.2000000476837158, 2.4000000953674316]
->
-
-iex> Axon.Activations.hard_silu(Nx.tensor([[-1.0, -2.0, -3.0], [1.0, 2.0, 3.0]], type: {:bf, 16}, names: [:batch, :data]))
-#Nx.Tensor<
- bf16[batch: 2][data: 3]
- [
- [-7.781982421875e-4, -0.0, -0.0],
- [0.3984375, 1.1953125, 2.390625]
- ]
->
+iex> Axon.Activations.hard_silu(Nx.tensor([-3.0, -2.0, -1.0, 0.0, 1.0, 2.0, 3.0], names: [:data]))
+#Nx.Tensor<
+ f32[data: 7]
+ [-0.0, -0.0, -0.0, 0.0, 0.4000000059604645, 1.2000000476837158, 2.4000000953674316]
+>
+
+iex> Axon.Activations.hard_silu(Nx.tensor([[-1.0, -2.0, -3.0], [1.0, 2.0, 3.0]], type: {:bf, 16}, names: [:batch, :data]))
+#Nx.Tensor<
+ bf16[batch: 2][data: 3]
+ [
+ [-7.781982421875e-4, -0.0, -0.0],
+ [0.3984375, 1.1953125, 2.390625]
+ ]
+>
@@ -706,20 +706,20 @@ hard_tanh(x)
Examples
-iex> Axon.Activations.hard_tanh(Nx.tensor([-3.0, -2.0, -1.0, 0.0, 1.0, 2.0, 3.0], names: [:data]))
-#Nx.Tensor<
- f32[data: 7]
- [-1.0, -1.0, -1.0, 0.0, 1.0, 1.0, 1.0]
->
-
-iex> Axon.Activations.hard_tanh(Nx.tensor([[-1.0, -2.0, -3.0], [1.0, 2.0, 3.0]], type: {:bf, 16}, names: [:batch, :data]))
-#Nx.Tensor<
- bf16[batch: 2][data: 3]
- [
- [-1.0, -1.0, -1.0],
- [1.0, 1.0, 1.0]
- ]
->
+iex> Axon.Activations.hard_tanh(Nx.tensor([-3.0, -2.0, -1.0, 0.0, 1.0, 2.0, 3.0], names: [:data]))
+#Nx.Tensor<
+ f32[data: 7]
+ [-1.0, -1.0, -1.0, 0.0, 1.0, 1.0, 1.0]
+>
+
+iex> Axon.Activations.hard_tanh(Nx.tensor([[-1.0, -2.0, -3.0], [1.0, 2.0, 3.0]], type: {:bf, 16}, names: [:batch, :data]))
+#Nx.Tensor<
+ bf16[batch: 2][data: 3]
+ [
+ [-1.0, -1.0, -1.0],
+ [1.0, 1.0, 1.0]
+ ]
+>
@@ -755,20 +755,20 @@ leaky_relu(x, opts \\ [])
Examples
-iex> Axon.Activations.leaky_relu(Nx.tensor([-3.0, -2.0, -1.0, 0.0, 1.0, 2.0, 3.0], names: [:data]), alpha: 0.5)
-#Nx.Tensor<
- f32[data: 7]
- [-1.5, -1.0, -0.5, 0.0, 1.0, 2.0, 3.0]
->
-
-iex> Axon.Activations.leaky_relu(Nx.tensor([[-1.0, -2.0, -3.0], [1.0, 2.0, 3.0]], names: [:batch, :data]), alpha: 0.5)
-#Nx.Tensor<
- f32[batch: 2][data: 3]
- [
- [-0.5, -1.0, -1.5],
- [1.0, 2.0, 3.0]
- ]
->
+iex> Axon.Activations.leaky_relu(Nx.tensor([-3.0, -2.0, -1.0, 0.0, 1.0, 2.0, 3.0], names: [:data]), alpha: 0.5)
+#Nx.Tensor<
+ f32[data: 7]
+ [-1.5, -1.0, -0.5, 0.0, 1.0, 2.0, 3.0]
+>
+
+iex> Axon.Activations.leaky_relu(Nx.tensor([[-1.0, -2.0, -3.0], [1.0, 2.0, 3.0]], names: [:batch, :data]), alpha: 0.5)
+#Nx.Tensor<
+ f32[batch: 2][data: 3]
+ [
+ [-0.5, -1.0, -1.5],
+ [1.0, 2.0, 3.0]
+ ]
+>
@@ -796,20 +796,20 @@ linear(x)
Examples
-iex> Axon.Activations.linear(Nx.tensor([-3.0, -2.0, -1.0, 0.0, 1.0, 2.0, 3.0], names: [:data]))
-#Nx.Tensor<
- f32[data: 7]
- [-3.0, -2.0, -1.0, 0.0, 1.0, 2.0, 3.0]
->
-
-iex> Axon.Activations.linear(Nx.tensor([[-1.0, -2.0, -3.0], [1.0, 2.0, 3.0]], type: {:bf, 16}, names: [:batch, :data]))
-#Nx.Tensor<
- bf16[batch: 2][data: 3]
- [
- [-1.0, -2.0, -3.0],
- [1.0, 2.0, 3.0]
- ]
->
+iex> Axon.Activations.linear(Nx.tensor([-3.0, -2.0, -1.0, 0.0, 1.0, 2.0, 3.0], names: [:data]))
+#Nx.Tensor<
+ f32[data: 7]
+ [-3.0, -2.0, -1.0, 0.0, 1.0, 2.0, 3.0]
+>
+
+iex> Axon.Activations.linear(Nx.tensor([[-1.0, -2.0, -3.0], [1.0, 2.0, 3.0]], type: {:bf, 16}, names: [:batch, :data]))
+#Nx.Tensor<
+ bf16[batch: 2][data: 3]
+ [
+ [-1.0, -2.0, -3.0],
+ [1.0, 2.0, 3.0]
+ ]
+>
@@ -837,20 +837,20 @@ log_sigmoid(x)
Examples
-iex> Axon.Activations.log_sigmoid(Nx.tensor([-3.0, -2.0, -1.0, 0.0, 1.0, 2.0, 3.0], type: {:f, 32}, names: [:data]))
-#Nx.Tensor<
- f32[data: 7]
- [-3.0485873222351074, -2.1269280910491943, -1.3132617473602295, -0.6931471824645996, -0.3132616877555847, -0.12692801654338837, -0.04858734831213951]
->
-
-iex> Axon.Activations.log_sigmoid(Nx.tensor([[-1.0, -2.0, -3.0], [1.0, 2.0, 3.0]], type: {:bf, 16}, names: [:batch, :data]))
-#Nx.Tensor<
- bf16[batch: 2][data: 3]
- [
- [-1.3125, -2.125, -3.046875],
- [-0.3125, -0.1259765625, -0.04833984375]
- ]
->
+iex> Axon.Activations.log_sigmoid(Nx.tensor([-3.0, -2.0, -1.0, 0.0, 1.0, 2.0, 3.0], type: {:f, 32}, names: [:data]))
+#Nx.Tensor<
+ f32[data: 7]
+ [-3.0485873222351074, -2.1269280910491943, -1.3132617473602295, -0.6931471824645996, -0.3132616877555847, -0.12692801654338837, -0.04858734831213951]
+>
+
+iex> Axon.Activations.log_sigmoid(Nx.tensor([[-1.0, -2.0, -3.0], [1.0, 2.0, 3.0]], type: {:bf, 16}, names: [:batch, :data]))
+#Nx.Tensor<
+ bf16[batch: 2][data: 3]
+ [
+ [-1.3125, -2.125, -3.046875],
+ [-0.3125, -0.1259765625, -0.04833984375]
+ ]
+>
@@ -880,20 +880,20 @@ log_softmax(x, opts \\ [])
Examples
-iex> Axon.Activations.log_softmax(Nx.tensor([-3.0, -2.0, -1.0, 0.0, 1.0, 2.0, 3.0], type: {:f, 32}, names: [:data]))
-#Nx.Tensor<
- f32[data: 7]
- [-6.457762718200684, -5.457762718200684, -4.457762718200684, -3.4577627182006836, -2.4577627182006836, -1.4577628374099731, -0.45776283740997314]
->
-
-iex> Axon.Activations.log_softmax(Nx.tensor([[-1.0, -2.0, -3.0], [1.0, 2.0, 3.0]], type: {:bf, 16}, names: [:batch, :data]))
-#Nx.Tensor<
- bf16[batch: 2][data: 3]
- [
- [-0.404296875, -1.3984375, -2.390625],
- [-2.390625, -1.3984375, -0.404296875]
- ]
->
+iex> Axon.Activations.log_softmax(Nx.tensor([-3.0, -2.0, -1.0, 0.0, 1.0, 2.0, 3.0], type: {:f, 32}, names: [:data]))
+#Nx.Tensor<
+ f32[data: 7]
+ [-6.457762718200684, -5.457762718200684, -4.457762718200684, -3.4577627182006836, -2.4577627182006836, -1.4577628374099731, -0.45776283740997314]
+>
+
+iex> Axon.Activations.log_softmax(Nx.tensor([[-1.0, -2.0, -3.0], [1.0, 2.0, 3.0]], type: {:bf, 16}, names: [:batch, :data]))
+#Nx.Tensor<
+ bf16[batch: 2][data: 3]
+ [
+ [-0.404296875, -1.3984375, -2.390625],
+ [-2.390625, -1.3984375, -0.404296875]
+ ]
+>
@@ -923,20 +923,20 @@ log_sumexp(x, opts \\ [])
Examples
-iex> Axon.Activations.log_sumexp(Nx.tensor([-3.0, -2.0, -1.0, 0.0, 1.0, 2.0, 3.0], names: [:data]))
-#Nx.Tensor<
- f32[data: 1]
- [0.45776283740997314]
->
-
-iex> Axon.Activations.log_sumexp(Nx.tensor([[-1.0, -2.0, -3.0], [1.0, 2.0, 3.0]], type: {:bf, 16}, names: [:batch, :data]))
-#Nx.Tensor<
- bf16[batch: 2][data: 1]
- [
- [0.404296875],
- [0.404296875]
- ]
->
+iex> Axon.Activations.log_sumexp(Nx.tensor([-3.0, -2.0, -1.0, 0.0, 1.0, 2.0, 3.0], names: [:data]))
+#Nx.Tensor<
+ f32[data: 1]
+ [0.45776283740997314]
+>
+
+iex> Axon.Activations.log_sumexp(Nx.tensor([[-1.0, -2.0, -3.0], [1.0, 2.0, 3.0]], type: {:bf, 16}, names: [:batch, :data]))
+#Nx.Tensor<
+ bf16[batch: 2][data: 1]
+ [
+ [0.404296875],
+ [0.404296875]
+ ]
+>
@@ -964,20 +964,20 @@ mish(x)
Examples
-iex> Axon.Activations.mish(Nx.tensor([-3.0, -2.0, -1.0, 0.0, 1.0, 2.0, 3.0], type: {:f, 32}, names: [:data]))
-#Nx.Tensor<
- f32[data: 7]
- [-0.14564745128154755, -0.2525014877319336, -0.30340147018432617, 0.0, 0.8650984168052673, 1.9439589977264404, 2.98653507232666]
->
-
-iex> Axon.Activations.mish(Nx.tensor([[-1.0, -2.0, -3.0], [1.0, 2.0, 3.0]], type: {:bf, 16}, names: [:batch, :data]))
-#Nx.Tensor<
- bf16[batch: 2][data: 3]
- [
- [-0.30078125, -0.25, -0.1435546875],
- [0.86328125, 1.9375, 2.96875]
- ]
->
+iex> Axon.Activations.mish(Nx.tensor([-3.0, -2.0, -1.0, 0.0, 1.0, 2.0, 3.0], type: {:f, 32}, names: [:data]))
+#Nx.Tensor<
+ f32[data: 7]
+ [-0.14564745128154755, -0.2525014877319336, -0.30340147018432617, 0.0, 0.8650984168052673, 1.9439589977264404, 2.98653507232666]
+>
+
+iex> Axon.Activations.mish(Nx.tensor([[-1.0, -2.0, -3.0], [1.0, 2.0, 3.0]], type: {:bf, 16}, names: [:batch, :data]))
+#Nx.Tensor<
+ bf16[batch: 2][data: 3]
+ [
+ [-0.30078125, -0.25, -0.1435546875],
+ [0.86328125, 1.9375, 2.96875]
+ ]
+>
@@ -1005,20 +1005,20 @@ relu6(x)
Examples
-iex> Axon.Activations.relu6(Nx.tensor([-3.0, -2.0, -1.0, 0.0, 1.0, 2.0, 3.0]))
-#Nx.Tensor<
- f32[7]
- [0.0, 0.0, 0.0, 0.0, 1.0, 2.0, 3.0]
->
-
-iex> Axon.Activations.relu6(Nx.tensor([[-1.0, -2.0, -3.0], [1.0, 2.0, 3.0]], type: {:bf, 16}, names: [:batch, :data]))
-#Nx.Tensor<
- bf16[batch: 2][data: 3]
- [
- [0.0, 0.0, 0.0],
- [1.0, 2.0, 3.0]
- ]
->
+iex> Axon.Activations.relu6(Nx.tensor([-3.0, -2.0, -1.0, 0.0, 1.0, 2.0, 3.0]))
+#Nx.Tensor<
+ f32[7]
+ [0.0, 0.0, 0.0, 0.0, 1.0, 2.0, 3.0]
+>
+
+iex> Axon.Activations.relu6(Nx.tensor([[-1.0, -2.0, -3.0], [1.0, 2.0, 3.0]], type: {:bf, 16}, names: [:batch, :data]))
+#Nx.Tensor<
+ bf16[batch: 2][data: 3]
+ [
+ [0.0, 0.0, 0.0],
+ [1.0, 2.0, 3.0]
+ ]
+>
references
@@ -1052,20 +1052,20 @@ relu(x)
Examples
-iex> Axon.Activations.relu(Nx.tensor([-3.0, -2.0, -1.0, 0.0, 1.0, 2.0, 3.0], names: [:data]))
-#Nx.Tensor<
- f32[data: 7]
- [0.0, 0.0, 0.0, 0.0, 1.0, 2.0, 3.0]
->
-
-iex> Axon.Activations.relu(Nx.tensor([[-1.0, -2.0, -3.0], [1.0, 2.0, 3.0]], type: {:bf, 16}, names: [:batch, :data]))
-#Nx.Tensor<
- bf16[batch: 2][data: 3]
- [
- [0.0, 0.0, 0.0],
- [1.0, 2.0, 3.0]
- ]
->
+iex> Axon.Activations.relu(Nx.tensor([-3.0, -2.0, -1.0, 0.0, 1.0, 2.0, 3.0], names: [:data]))
+#Nx.Tensor<
+ f32[data: 7]
+ [0.0, 0.0, 0.0, 0.0, 1.0, 2.0, 3.0]
+>
+
+iex> Axon.Activations.relu(Nx.tensor([[-1.0, -2.0, -3.0], [1.0, 2.0, 3.0]], type: {:bf, 16}, names: [:batch, :data]))
+#Nx.Tensor<
+ bf16[batch: 2][data: 3]
+ [
+ [0.0, 0.0, 0.0],
+ [1.0, 2.0, 3.0]
+ ]
+>
@@ -1097,20 +1097,20 @@ selu(x, opts \\ [])
Examples
-iex> Axon.Activations.selu(Nx.tensor([-3.0, -2.0, -1.0, 0.0, 1.0, 2.0, 3.0], names: [:data]))
-#Nx.Tensor<
- f32[data: 7]
- [-1.670568823814392, -1.5201665163040161, -1.1113307476043701, 0.0, 1.0507010221481323, 2.1014020442962646, 3.1521029472351074]
->
-
-iex> Axon.Activations.selu(Nx.tensor([[-1.0, -2.0, -3.0], [1.0, 2.0, 3.0]], type: {:bf, 16}, names: [:batch, :data]))
-#Nx.Tensor<
- bf16[batch: 2][data: 3]
- [
- [-1.09375, -1.5078125, -1.6640625],
- [1.046875, 2.09375, 3.140625]
- ]
->
+iex> Axon.Activations.selu(Nx.tensor([-3.0, -2.0, -1.0, 0.0, 1.0, 2.0, 3.0], names: [:data]))
+#Nx.Tensor<
+ f32[data: 7]
+ [-1.670568823814392, -1.5201665163040161, -1.1113307476043701, 0.0, 1.0507010221481323, 2.1014020442962646, 3.1521029472351074]
+>
+
+iex> Axon.Activations.selu(Nx.tensor([[-1.0, -2.0, -3.0], [1.0, 2.0, 3.0]], type: {:bf, 16}, names: [:batch, :data]))
+#Nx.Tensor<
+ bf16[batch: 2][data: 3]
+ [
+ [-1.09375, -1.5078125, -1.6640625],
+ [1.046875, 2.09375, 3.140625]
+ ]
+>
references
@@ -1147,20 +1147,20 @@ sigmoid(x)
Examples
-iex> Axon.Activations.sigmoid(Nx.tensor([-3.0, -2.0, -1.0, 0.0, 1.0, 2.0, 3.0], names: [:data]))
-#Nx.Tensor<
- f32[data: 7]
- [0.04742587357759476, 0.11920291930437088, 0.2689414322376251, 0.5, 0.7310585975646973, 0.8807970881462097, 0.9525741338729858]
->
-
-iex> Axon.Activations.sigmoid(Nx.tensor([[-1.0, -2.0, -3.0], [1.0, 2.0, 3.0]], type: {:bf, 16}, names: [:batch, :data]))
-#Nx.Tensor<
- bf16[batch: 2][data: 3]
- [
- [0.267578125, 0.119140625, 0.04736328125],
- [0.73046875, 0.87890625, 0.94921875]
- ]
->
+iex> Axon.Activations.sigmoid(Nx.tensor([-3.0, -2.0, -1.0, 0.0, 1.0, 2.0, 3.0], names: [:data]))
+#Nx.Tensor<
+ f32[data: 7]
+ [0.04742587357759476, 0.11920291930437088, 0.2689414322376251, 0.5, 0.7310585975646973, 0.8807970881462097, 0.9525741338729858]
+>
+
+iex> Axon.Activations.sigmoid(Nx.tensor([[-1.0, -2.0, -3.0], [1.0, 2.0, 3.0]], type: {:bf, 16}, names: [:batch, :data]))
+#Nx.Tensor<
+ bf16[batch: 2][data: 3]
+ [
+ [0.267578125, 0.119140625, 0.04736328125],
+ [0.73046875, 0.87890625, 0.94921875]
+ ]
+>
@@ -1188,20 +1188,20 @@ silu(x)
Examples
-iex> Axon.Activations.silu(Nx.tensor([-3.0, -2.0, -1.0, 0.0, 1.0, 2.0, 3.0], names: [:data]))
-#Nx.Tensor<
- f32[data: 7]
- [-0.14227762818336487, -0.23840583860874176, -0.2689414322376251, 0.0, 0.7310585975646973, 1.7615941762924194, 2.857722282409668]
->
-
-iex> Axon.Activations.silu(Nx.tensor([[-1.0, -2.0, -3.0], [1.0, 2.0, 3.0]], type: {:bf, 16}, names: [:batch, :data]))
-#Nx.Tensor<
- bf16[batch: 2][data: 3]
- [
- [-0.267578125, -0.23828125, -0.1416015625],
- [0.73046875, 1.7578125, 2.84375]
- ]
->
+iex> Axon.Activations.silu(Nx.tensor([-3.0, -2.0, -1.0, 0.0, 1.0, 2.0, 3.0], names: [:data]))
+#Nx.Tensor<
+ f32[data: 7]
+ [-0.14227762818336487, -0.23840583860874176, -0.2689414322376251, 0.0, 0.7310585975646973, 1.7615941762924194, 2.857722282409668]
+>
+
+iex> Axon.Activations.silu(Nx.tensor([[-1.0, -2.0, -3.0], [1.0, 2.0, 3.0]], type: {:bf, 16}, names: [:batch, :data]))
+#Nx.Tensor<
+ bf16[batch: 2][data: 3]
+ [
+ [-0.267578125, -0.23828125, -0.1416015625],
+ [0.73046875, 1.7578125, 2.84375]
+ ]
+>
references
@@ -1247,22 +1247,22 @@ softmax(x, opts \\ [])
Examples
-iex> Axon.Activations.softmax(Nx.tensor([[-3.0, -2.0, -1.0, 0.0, 1.0, 2.0, 3.0]], names: [:batch, :data]))
-#Nx.Tensor<
- f32[batch: 1][data: 7]
- [
- [0.0015683004166930914, 0.004263082519173622, 0.011588259600102901, 0.03150015324354172, 0.08562629669904709, 0.23275642096996307, 0.6326975226402283]
- ]
->
-
-iex> Axon.Activations.softmax(Nx.tensor([[-1.0, -2.0, -3.0], [1.0, 2.0, 3.0]], type: {:bf, 16}, names: [:batch, :data]))
-#Nx.Tensor<
- bf16[batch: 2][data: 3]
- [
- [0.6640625, 0.2431640625, 0.08935546875],
- [0.08935546875, 0.2431640625, 0.6640625]
- ]
->
+iex> Axon.Activations.softmax(Nx.tensor([[-3.0, -2.0, -1.0, 0.0, 1.0, 2.0, 3.0]], names: [:batch, :data]))
+#Nx.Tensor<
+ f32[batch: 1][data: 7]
+ [
+ [0.0015683004166930914, 0.004263082519173622, 0.011588259600102901, 0.03150015324354172, 0.08562629669904709, 0.23275642096996307, 0.6326975226402283]
+ ]
+>
+
+iex> Axon.Activations.softmax(Nx.tensor([[-1.0, -2.0, -3.0], [1.0, 2.0, 3.0]], type: {:bf, 16}, names: [:batch, :data]))
+#Nx.Tensor<
+ bf16[batch: 2][data: 3]
+ [
+ [0.6640625, 0.2431640625, 0.08935546875],
+ [0.08935546875, 0.2431640625, 0.6640625]
+ ]
+>
@@ -1290,20 +1290,20 @@ softplus(x)
Examples
-iex> Axon.Activations.softplus(Nx.tensor([-3.0, -2.0, -1.0, 0.0, 1.0, 2.0, 3.0], names: [:data]))
-#Nx.Tensor<
- f32[data: 7]
- [0.04858734831213951, 0.12692801654338837, 0.3132616877555847, 0.6931471824645996, 1.3132617473602295, 2.1269280910491943, 3.0485873222351074]
->
-
-iex> Axon.Activations.softplus(Nx.tensor([[-1.0, -2.0, -3.0], [1.0, 2.0, 3.0]], type: {:bf, 16}, names: [:batch, :data]))
-#Nx.Tensor<
- bf16[batch: 2][data: 3]
- [
- [0.3125, 0.1259765625, 0.04833984375],
- [1.3125, 2.125, 3.046875]
- ]
->
+iex> Axon.Activations.softplus(Nx.tensor([-3.0, -2.0, -1.0, 0.0, 1.0, 2.0, 3.0], names: [:data]))
+#Nx.Tensor<
+ f32[data: 7]
+ [0.04858734831213951, 0.12692801654338837, 0.3132616877555847, 0.6931471824645996, 1.3132617473602295, 2.1269280910491943, 3.0485873222351074]
+>
+
+iex> Axon.Activations.softplus(Nx.tensor([[-1.0, -2.0, -3.0], [1.0, 2.0, 3.0]], type: {:bf, 16}, names: [:batch, :data]))
+#Nx.Tensor<
+ bf16[batch: 2][data: 3]
+ [
+ [0.3125, 0.1259765625, 0.04833984375],
+ [1.3125, 2.125, 3.046875]
+ ]
+>
@@ -1331,20 +1331,20 @@ softsign(x)
Examples
-iex> Axon.Activations.softsign(Nx.tensor([-3.0, -2.0, -1.0, 0.0, 1.0, 2.0, 3.0], names: [:data]))
-#Nx.Tensor<
- f32[data: 7]
- [-0.75, -0.6666666865348816, -0.5, 0.0, 0.5, 0.6666666865348816, 0.75]
->
-
-iex> Axon.Activations.softsign(Nx.tensor([[-1.0, -2.0, -3.0], [1.0, 2.0, 3.0]], type: {:bf, 16}, names: [:batch, :data]))
-#Nx.Tensor<
- bf16[batch: 2][data: 3]
- [
- [-0.5, -0.6640625, -0.75],
- [0.5, 0.6640625, 0.75]
- ]
->
+iex> Axon.Activations.softsign(Nx.tensor([-3.0, -2.0, -1.0, 0.0, 1.0, 2.0, 3.0], names: [:data]))
+#Nx.Tensor<
+ f32[data: 7]
+ [-0.75, -0.6666666865348816, -0.5, 0.0, 0.5, 0.6666666865348816, 0.75]
+>
+
+iex> Axon.Activations.softsign(Nx.tensor([[-1.0, -2.0, -3.0], [1.0, 2.0, 3.0]], type: {:bf, 16}, names: [:batch, :data]))
+#Nx.Tensor<
+ bf16[batch: 2][data: 3]
+ [
+ [-0.5, -0.6640625, -0.75],
+ [0.5, 0.6640625, 0.75]
+ ]
+>
@@ -1372,20 +1372,20 @@ tanh(x)
Examples
-iex> Axon.Activations.tanh(Nx.tensor([-3.0, -2.0, -1.0, 0.0, 1.0, 2.0, 3.0], names: [:data]))
-#Nx.Tensor<
- f32[data: 7]
- [-0.9950547814369202, -0.9640275835990906, -0.7615941762924194, 0.0, 0.7615941762924194, 0.9640275835990906, 0.9950547814369202]
->
-
-iex> Axon.Activations.tanh(Nx.tensor([[-1.0, -2.0, -3.0], [1.0, 2.0, 3.0]], type: {:bf, 16}, names: [:batch, :data]))
-#Nx.Tensor<
- bf16[batch: 2][data: 3]
- [
- [-0.7578125, -0.9609375, -0.9921875],
- [0.7578125, 0.9609375, 0.9921875]
- ]
->
+iex> Axon.Activations.tanh(Nx.tensor([-3.0, -2.0, -1.0, 0.0, 1.0, 2.0, 3.0], names: [:data]))
+#Nx.Tensor<
+ f32[data: 7]
+ [-0.9950547814369202, -0.9640275835990906, -0.7615941762924194, 0.0, 0.7615941762924194, 0.9640275835990906, 0.9950547814369202]
+>
+
+iex> Axon.Activations.tanh(Nx.tensor([[-1.0, -2.0, -3.0], [1.0, 2.0, 3.0]], type: {:bf, 16}, names: [:batch, :data]))
+#Nx.Tensor<
+ bf16[batch: 2][data: 3]
+ [
+ [-0.7578125, -0.9609375, -0.9921875],
+ [0.7578125, 0.9609375, 0.9921875]
+ ]
+>
diff --git a/Axon.Display.html b/Axon.Display.html
index 7e2c930c..072a7c11 100644
--- a/Axon.Display.html
+++ b/Axon.Display.html
@@ -201,7 +201,7 @@ as_graph(axon, input_templates, opts \\ [])
Examples
-
Given an Axon model:
model = Axon.input("input") |> Axon.dense(32)
You can define input templates for each input:
input = Nx.template({1, 16}, :f32)
And then display the execution flow of the model:
Axon.Display.as_graph(model, input, direction: :top_down)
+Given an Axon model:
model = Axon.input("input") |> Axon.dense(32)
You can define input templates for each input:
input = Nx.template({1, 16}, :f32)
And then display the execution flow of the model:
Axon.Display.as_graph(model, input, direction: :top_down)
@@ -231,7 +231,7 @@ as_table(axon, input_templates)
Examples
-Given an Axon model:
model = Axon.input("input") |> Axon.dense(32)
You can define input templates for each input:
input = Nx.template({1, 16}, :f32)
And then display the execution flow of the model:
Axon.Display.as_table(model, input)
+Given an Axon model:
model = Axon.input("input") |> Axon.dense(32)
You can define input templates for each input:
input = Nx.template({1, 16}, :f32)
And then display the execution flow of the model:
Axon.Display.as_table(model, input)
diff --git a/Axon.Initializers.html b/Axon.Initializers.html
index 6f1760c6..2f1a6204 100644
--- a/Axon.Initializers.html
+++ b/Axon.Initializers.html
@@ -132,8 +132,8 @@
small enough to avoid exploding values. The initializers in
this module have a default scale known to work well with
the initialization strategy.
The functions in this module return initialization functions which
-take shapes and types and return tensors:
init_fn = Axon.Initializers.zeros()
-init_fn.({1, 2}, {:f, 32})
You may use these functions from within defn
or outside.
+take shapes and types and return tensors:init_fn = Axon.Initializers.zeros()
+init_fn.({1, 2}, {:f, 32})
You may use these functions from within defn
or outside.
@@ -330,16 +330,16 @@ full(value)
Examples
-iex> init_fn = Axon.Initializers.full(1.00)
-iex> out = init_fn.({2, 2}, {:f, 32})
+iex> init_fn = Axon.Initializers.full(1.00)
+iex> out = init_fn.({2, 2}, {:f, 32})
iex> out
-#Nx.Tensor<
- f32[2][2]
- [
- [1.0, 1.0],
- [1.0, 1.0]
- ]
->
+#Nx.Tensor<
+ f32[2][2]
+ [
+ [1.0, 1.0],
+ [1.0, 1.0]
+ ]
+>
@@ -378,19 +378,19 @@ glorot_normal(opts \\ [])
Examples
-iex> init_fn = Axon.Initializers.glorot_normal()
-iex> t = init_fn.({2, 2}, {:f, 32}, Nx.Random.key(1))
-iex> Nx.shape(t)
-{2, 2}
-iex> Nx.type(t)
-{:f, 32}
-
-iex> init_fn = Axon.Initializers.glorot_normal(scale: 1.0e-3)
-iex> t = init_fn.({2, 2}, {:bf, 16}, Nx.Random.key(1))
-iex> Nx.shape(t)
-{2, 2}
-iex> Nx.type(t)
-{:bf, 16}
+iex> init_fn = Axon.Initializers.glorot_normal()
+iex> t = init_fn.({2, 2}, {:f, 32}, Nx.Random.key(1))
+iex> Nx.shape(t)
+{2, 2}
+iex> Nx.type(t)
+{:f, 32}
+
+iex> init_fn = Axon.Initializers.glorot_normal(scale: 1.0e-3)
+iex> t = init_fn.({2, 2}, {:bf, 16}, Nx.Random.key(1))
+iex> Nx.shape(t)
+{2, 2}
+iex> Nx.type(t)
+{:bf, 16}
references
@@ -435,19 +435,19 @@ glorot_uniform(opts \\ [])
Examples
-iex> init_fn = Axon.Initializers.glorot_uniform()
-iex> t = init_fn.({2, 2}, {:f, 32}, Nx.Random.key(1))
-iex> Nx.shape(t)
-{2, 2}
-iex> Nx.type(t)
-{:f, 32}
-
-iex> init_fn = Axon.Initializers.glorot_uniform(scale: 1.0e-3)
-iex> t = init_fn.({2, 2}, {:bf, 16}, Nx.Random.key(1))
-iex> Nx.shape(t)
-{2, 2}
-iex> Nx.type(t)
-{:bf, 16}
+iex> init_fn = Axon.Initializers.glorot_uniform()
+iex> t = init_fn.({2, 2}, {:f, 32}, Nx.Random.key(1))
+iex> Nx.shape(t)
+{2, 2}
+iex> Nx.type(t)
+{:f, 32}
+
+iex> init_fn = Axon.Initializers.glorot_uniform(scale: 1.0e-3)
+iex> t = init_fn.({2, 2}, {:bf, 16}, Nx.Random.key(1))
+iex> Nx.shape(t)
+{2, 2}
+iex> Nx.type(t)
+{:bf, 16}
references
@@ -491,19 +491,19 @@ he_normal(opts \\ [])
Examples
-iex> init_fn = Axon.Initializers.he_normal()
-iex> t = init_fn.({2, 2}, {:f, 32}, Nx.Random.key(1))
-iex> Nx.shape(t)
-{2, 2}
-iex> Nx.type(t)
-{:f, 32}
-
-iex> init_fn = Axon.Initializers.he_normal(scale: 1.0e-3)
-iex> t = init_fn.({2, 2}, {:bf, 16}, Nx.Random.key(1))
-iex> Nx.shape(t)
-{2, 2}
-iex> Nx.type(t)
-{:bf, 16}
+iex> init_fn = Axon.Initializers.he_normal()
+iex> t = init_fn.({2, 2}, {:f, 32}, Nx.Random.key(1))
+iex> Nx.shape(t)
+{2, 2}
+iex> Nx.type(t)
+{:f, 32}
+
+iex> init_fn = Axon.Initializers.he_normal(scale: 1.0e-3)
+iex> t = init_fn.({2, 2}, {:bf, 16}, Nx.Random.key(1))
+iex> Nx.shape(t)
+{2, 2}
+iex> Nx.type(t)
+{:bf, 16}
references
@@ -547,19 +547,19 @@ he_uniform(opts \\ [])
Examples
-iex> init_fn = Axon.Initializers.he_uniform()
-iex> t = init_fn.({2, 2}, {:f, 32}, Nx.Random.key(1))
-iex> Nx.shape(t)
-{2, 2}
-iex> Nx.type(t)
-{:f, 32}
-
-iex> init_fn = Axon.Initializers.he_uniform(scale: 1.0e-3)
-iex> t = init_fn.({2, 2}, {:bf, 16}, Nx.Random.key(1))
-iex> Nx.shape(t)
-{2, 2}
-iex> Nx.type(t)
-{:bf, 16}
+iex> init_fn = Axon.Initializers.he_uniform()
+iex> t = init_fn.({2, 2}, {:f, 32}, Nx.Random.key(1))
+iex> Nx.shape(t)
+{2, 2}
+iex> Nx.type(t)
+{:f, 32}
+
+iex> init_fn = Axon.Initializers.he_uniform(scale: 1.0e-3)
+iex> t = init_fn.({2, 2}, {:bf, 16}, Nx.Random.key(1))
+iex> Nx.shape(t)
+{2, 2}
+iex> Nx.type(t)
+{:bf, 16}
references
@@ -593,16 +593,16 @@ identity()
Examples
-iex> init_fn = Axon.Initializers.identity()
-iex> out = init_fn.({2, 2}, {:f, 32})
+iex> init_fn = Axon.Initializers.identity()
+iex> out = init_fn.({2, 2}, {:f, 32})
iex> out
-#Nx.Tensor<
- f32[2][2]
- [
- [1.0, 0.0],
- [0.0, 1.0]
- ]
->
+#Nx.Tensor<
+ f32[2][2]
+ [
+ [1.0, 0.0],
+ [0.0, 1.0]
+ ]
+>
@@ -640,19 +640,19 @@ lecun_normal(opts \\ [])
Examples
-iex> init_fn = Axon.Initializers.lecun_normal()
-iex> t = init_fn.({2, 2}, {:f, 32}, Nx.Random.key(1))
-iex> Nx.shape(t)
-{2, 2}
-iex> Nx.type(t)
-{:f, 32}
-
-iex> init_fn = Axon.Initializers.lecun_normal(scale: 1.0e-3)
-iex> t = init_fn.({2, 2}, {:bf, 16}, Nx.Random.key(1))
-iex> Nx.shape(t)
-{2, 2}
-iex> Nx.type(t)
-{:bf, 16}
+iex> init_fn = Axon.Initializers.lecun_normal()
+iex> t = init_fn.({2, 2}, {:f, 32}, Nx.Random.key(1))
+iex> Nx.shape(t)
+{2, 2}
+iex> Nx.type(t)
+{:f, 32}
+
+iex> init_fn = Axon.Initializers.lecun_normal(scale: 1.0e-3)
+iex> t = init_fn.({2, 2}, {:bf, 16}, Nx.Random.key(1))
+iex> Nx.shape(t)
+{2, 2}
+iex> Nx.type(t)
+{:bf, 16}
references
@@ -696,19 +696,19 @@ lecun_uniform(opts \\ [])
Examples
-iex> init_fn = Axon.Initializers.lecun_uniform()
-iex> t = init_fn.({2, 2}, {:f, 32}, Nx.Random.key(1))
-iex> Nx.shape(t)
-{2, 2}
-iex> Nx.type(t)
-{:f, 32}
-
-iex> init_fn = Axon.Initializers.lecun_uniform(scale: 1.0e-3)
-iex> t = init_fn.({2, 2}, {:bf, 16}, Nx.Random.key(1))
-iex> Nx.shape(t)
-{2, 2}
-iex> Nx.type(t)
-{:bf, 16}
+iex> init_fn = Axon.Initializers.lecun_uniform()
+iex> t = init_fn.({2, 2}, {:f, 32}, Nx.Random.key(1))
+iex> Nx.shape(t)
+{2, 2}
+iex> Nx.type(t)
+{:f, 32}
+
+iex> init_fn = Axon.Initializers.lecun_uniform(scale: 1.0e-3)
+iex> t = init_fn.({2, 2}, {:bf, 16}, Nx.Random.key(1))
+iex> Nx.shape(t)
+{2, 2}
+iex> Nx.type(t)
+{:bf, 16}
references
@@ -750,19 +750,19 @@ normal(opts \\ [])
Examples
-iex> init_fn = Axon.Initializers.normal()
-iex> t = init_fn.({2, 2}, {:f, 32}, Nx.Random.key(1))
-iex> Nx.shape(t)
-{2, 2}
-iex> Nx.type(t)
-{:f, 32}
-
-iex> init_fn = Axon.Initializers.normal(mean: 1.0, scale: 1.0)
-iex> t = init_fn.({2, 2}, {:bf, 16}, Nx.Random.key(1))
-iex> Nx.shape(t)
-{2, 2}
-iex> Nx.type(t)
-{:bf, 16}
+iex> init_fn = Axon.Initializers.normal()
+iex> t = init_fn.({2, 2}, {:f, 32}, Nx.Random.key(1))
+iex> Nx.shape(t)
+{2, 2}
+iex> Nx.type(t)
+{:f, 32}
+
+iex> init_fn = Axon.Initializers.normal(mean: 1.0, scale: 1.0)
+iex> t = init_fn.({2, 2}, {:bf, 16}, Nx.Random.key(1))
+iex> Nx.shape(t)
+{2, 2}
+iex> Nx.type(t)
+{:bf, 16}
@@ -790,16 +790,16 @@ ones()
Examples
-iex> init_fn = Axon.Initializers.ones()
-iex> out = init_fn.({2, 2}, {:f, 32})
+iex> init_fn = Axon.Initializers.ones()
+iex> out = init_fn.({2, 2}, {:f, 32})
iex> out
-#Nx.Tensor<
- f32[2][2]
- [
- [1.0, 1.0],
- [1.0, 1.0]
- ]
->
+#Nx.Tensor<
+ f32[2][2]
+ [
+ [1.0, 1.0],
+ [1.0, 1.0]
+ ]
+>
@@ -838,19 +838,19 @@ orthogonal(opts \\ [])
Examples
-iex> init_fn = Axon.Initializers.orthogonal()
-iex> t = init_fn.({3, 3}, {:f, 32}, Nx.Random.key(1))
-iex> Nx.type(t)
-{:f, 32}
-iex> Nx.shape(t)
-{3, 3}
-
-iex> init_fn = Axon.Initializers.orthogonal()
-iex> t = init_fn.({1, 2, 3, 4}, {:f, 64}, Nx.Random.key(1))
-iex> Nx.type(t)
-{:f, 64}
-iex> Nx.shape(t)
-{1, 2, 3, 4}
+iex> init_fn = Axon.Initializers.orthogonal()
+iex> t = init_fn.({3, 3}, {:f, 32}, Nx.Random.key(1))
+iex> Nx.type(t)
+{:f, 32}
+iex> Nx.shape(t)
+{3, 3}
+
+iex> init_fn = Axon.Initializers.orthogonal()
+iex> t = init_fn.({1, 2, 3, 4}, {:f, 64}, Nx.Random.key(1))
+iex> Nx.type(t)
+{:f, 64}
+iex> Nx.shape(t)
+{1, 2, 3, 4}
@@ -886,19 +886,19 @@ uniform(opts \\ [])
Examples
-iex> init_fn = Axon.Initializers.uniform()
-iex> t = init_fn.({2, 2}, {:f, 32}, Nx.Random.key(1))
-iex> Nx.shape(t)
-{2, 2}
-iex> Nx.type(t)
-{:f, 32}
-
-iex> init_fn = Axon.Initializers.uniform(scale: 1.0e-3)
-iex> t = init_fn.({2, 2}, {:bf, 16}, Nx.Random.key(1))
-iex> Nx.shape(t)
-{2, 2}
-iex> Nx.type(t)
-{:bf, 16}
+iex> init_fn = Axon.Initializers.uniform()
+iex> t = init_fn.({2, 2}, {:f, 32}, Nx.Random.key(1))
+iex> Nx.shape(t)
+{2, 2}
+iex> Nx.type(t)
+{:f, 32}
+
+iex> init_fn = Axon.Initializers.uniform(scale: 1.0e-3)
+iex> t = init_fn.({2, 2}, {:bf, 16}, Nx.Random.key(1))
+iex> Nx.shape(t)
+{2, 2}
+iex> Nx.type(t)
+{:bf, 16}
@@ -938,26 +938,26 @@ variance_scaling(opts \\ [])
Examples
-iex> init_fn = Axon.Initializers.variance_scaling()
-iex> t = init_fn.({2, 2}, {:f, 32}, Nx.Random.key(1))
-iex> Nx.shape(t)
-{2, 2}
-iex> Nx.type(t)
-{:f, 32}
-
-iex> init_fn = Axon.Initializers.variance_scaling(mode: :fan_out, distribution: :truncated_normal)
-iex> t = init_fn.({2, 2}, {:bf, 16}, Nx.Random.key(1))
-iex> Nx.shape(t)
-{2, 2}
-iex> Nx.type(t)
-{:bf, 16}
-
-iex> init_fn = Axon.Initializers.variance_scaling(mode: :fan_out, distribution: :normal)
-iex> t = init_fn.({64, 3, 32, 32}, {:f, 32}, Nx.Random.key(1))
-iex> Nx.shape(t)
-{64, 3, 32, 32}
-iex> Nx.type(t)
-{:f, 32}
+iex> init_fn = Axon.Initializers.variance_scaling()
+iex> t = init_fn.({2, 2}, {:f, 32}, Nx.Random.key(1))
+iex> Nx.shape(t)
+{2, 2}
+iex> Nx.type(t)
+{:f, 32}
+
+iex> init_fn = Axon.Initializers.variance_scaling(mode: :fan_out, distribution: :truncated_normal)
+iex> t = init_fn.({2, 2}, {:bf, 16}, Nx.Random.key(1))
+iex> Nx.shape(t)
+{2, 2}
+iex> Nx.type(t)
+{:bf, 16}
+
+iex> init_fn = Axon.Initializers.variance_scaling(mode: :fan_out, distribution: :normal)
+iex> t = init_fn.({64, 3, 32, 32}, {:f, 32}, Nx.Random.key(1))
+iex> Nx.shape(t)
+{64, 3, 32, 32}
+iex> Nx.type(t)
+{:f, 32}
@@ -985,16 +985,16 @@ zeros()
Examples
-iex> init_fn = Axon.Initializers.zeros()
-iex> out = init_fn.({2, 2}, {:f, 32})
+iex> init_fn = Axon.Initializers.zeros()
+iex> out = init_fn.({2, 2}, {:f, 32})
iex> out
-#Nx.Tensor<
- f32[2][2]
- [
- [0.0, 0.0],
- [0.0, 0.0]
- ]
->
+#Nx.Tensor<
+ f32[2][2]
+ [
+ [0.0, 0.0],
+ [0.0, 0.0]
+ ]
+>
diff --git a/Axon.Layers.html b/Axon.Layers.html
index db33840d..fd1c45a0 100644
--- a/Axon.Layers.html
+++ b/Axon.Layers.html
@@ -120,20 +120,20 @@
These implementations do not assume the responsibility of
managing state - instead opting to delegate this responsibility
to the caller.
Basic neural networks can be seen as a composition of functions:
input
-|> dense(w1, b1)
-|> relu()
-|> dense(w2, b2)
-|> softmax()
These kinds of models are often referred to as deep feedforward networks
+
|> dense(w1, b1)
+|> relu()
+|> dense(w2, b2)
+|> softmax()
These kinds of models are often referred to as deep feedforward networks or multilayer perceptrons (MLPs) because information flows forward through the network with no feedback connections. Mathematically, a feedforward network can be represented as:
$$f(x) = f^{(3)}(f^{(2)}(f^{(1)}(x)))$$
You can see a similar pattern emerge if we condense the call stack -in the previous example:
softmax(dense(relu(dense(input, w1, b1)), w2, b2))
The chain structure shown here is the most common structure used +in the previous example:
softmax(dense(relu(dense(input, w1, b1)), w2, b2))
The chain structure shown here is the most common structure used in neural networks. You can consider each function $f^{(n)}$ as a layer in the neural network - for example $f^{(2)} is the 2nd layer in the network. The number of function calls in the structure is the depth of the network. This is where the term deep learning comes from.
Neural networks are often written as the mapping:
$$y = f(x; \theta)$$
Where $x$ is the input to the neural network and $\theta$ are the -set of learned parameters. In Elixir, you would write this:
y = model(input, params)
From the previous example, params
would represent the collection:
{w1, b1, w2, b2}
where w1
and w2
are layer kernels, and b1
and b2
are layer
+set of learned parameters. In Elixir, you would write this:
y = model(input, params)
From the previous example, params
would represent the collection:
{w1, b1, w2, b2}
where w1
and w2
are layer kernels, and b1
and b2
are layer
biases.
iex> inp1 = Nx.iota({3, 2}, type: {:f, 32})
-iex> inp2 = Nx.iota({3, 4}, type: {:f, 32})
-iex> kernel = Nx.iota({1, 2, 4}, type: {:f, 32})
-iex> bias = Nx.tensor(1.0)
-iex> Axon.Layers.bilinear(inp1, inp2, kernel, bias)
-#Nx.Tensor<
- f32[3][1]
- [
- [39.0],
- [455.0],
- [1319.0]
- ]
->
+iex> inp1 = Nx.iota({3, 2}, type: {:f, 32})
+iex> inp2 = Nx.iota({3, 4}, type: {:f, 32})
+iex> kernel = Nx.iota({1, 2, 4}, type: {:f, 32})
+iex> bias = Nx.tensor(1.0)
+iex> Axon.Layers.bilinear(inp1, inp2, kernel, bias)
+#Nx.Tensor<
+ f32[3][1]
+ [
+ [39.0],
+ [455.0],
+ [1319.0]
+ ]
+>
Functional implementation of a dense layer.
Linear transformation of the input such that:
$$y = xW^T + b$$
A dense layer or fully connected layer transforms the input using the given kernel matrix and bias -to compute:
Nx.dot(input, kernel) + bias
Typically, both kernel
and bias
are learnable
+to compute:
Nx.dot(input, kernel) + bias
Typically, both kernel
and bias
are learnable
parameters trained using gradient-based optimization.
parameter-shapes
@@ -769,17 +769,17 @@iex> input = Nx.tensor([[1.0, 0.5, 1.0, 0.5], [0.0, 0.0, 0.0, 0.0]], type: {:f, 32})
-iex> kernel = Nx.tensor([[0.2], [0.3], [0.5], [0.8]], type: {:f, 32})
-iex> bias = Nx.tensor([1.0], type: {:f, 32})
-iex> Axon.Layers.dense(input, kernel, bias)
-#Nx.Tensor<
- f32[2][1]
- [
- [2.25],
- [1.0]
- ]
->
+iex> input = Nx.tensor([[1.0, 0.5, 1.0, 0.5], [0.0, 0.0, 0.0, 0.0]], type: {:f, 32})
+iex> kernel = Nx.tensor([[0.2], [0.3], [0.5], [0.8]], type: {:f, 32})
+iex> bias = Nx.tensor([1.0], type: {:f, 32})
+iex> Axon.Layers.dense(input, kernel, bias)
+#Nx.Tensor<
+ f32[2][1]
+ [
+ [2.25],
+ [1.0]
+ ]
+>
iex> input = Nx.tensor([[1, 2, 4, 5], [4, 3, 2, 9]])
-iex> kernels = Nx.tensor([
-...> [0.46299999952316284, 0.5562999844551086, 0.18170000612735748],
-...> [0.9801999926567078, 0.09780000150203705, 0.5333999991416931],
-...> [0.6980000138282776, 0.9240999817848206, 0.23479999601840973],
-...> [0.31929999589920044, 0.42250001430511475, 0.7865999937057495],
-...> [0.5519000291824341, 0.5662999749183655, 0.20559999346733093],
-...> [0.1898999959230423, 0.9311000108718872, 0.8356000185012817],
-...> [0.6383000016212463, 0.8794000148773193, 0.5282999873161316],
-...> [0.9523000121116638, 0.7597000002861023, 0.08250000327825546],
-...> [0.6622999906539917, 0.02329999953508377, 0.8205999732017517],
-...> [0.9855999946594238, 0.36419999599456787, 0.5372999906539917]
-...> ])
-iex> Axon.Layers.embedding(input, kernels)
-#Nx.Tensor<
- f32[2][4][3]
- [
- [
- [0.9801999926567078, 0.09780000150203705, 0.5333999991416931],
- [0.6980000138282776, 0.9240999817848206, 0.23479999601840973],
- [0.5519000291824341, 0.5662999749183655, 0.20559999346733093],
- [0.1898999959230423, 0.9311000108718872, 0.8356000185012817]
- ],
- [
- [0.5519000291824341, 0.5662999749183655, 0.20559999346733093],
- [0.31929999589920044, 0.42250001430511475, 0.7865999937057495],
- [0.6980000138282776, 0.9240999817848206, 0.23479999601840973],
- [0.9855999946594238, 0.36419999599456787, 0.5372999906539917]
- ]
- ]
->
+iex> input = Nx.tensor([[1, 2, 4, 5], [4, 3, 2, 9]])
+iex> kernels = Nx.tensor([
+...> [0.46299999952316284, 0.5562999844551086, 0.18170000612735748],
+...> [0.9801999926567078, 0.09780000150203705, 0.5333999991416931],
+...> [0.6980000138282776, 0.9240999817848206, 0.23479999601840973],
+...> [0.31929999589920044, 0.42250001430511475, 0.7865999937057495],
+...> [0.5519000291824341, 0.5662999749183655, 0.20559999346733093],
+...> [0.1898999959230423, 0.9311000108718872, 0.8356000185012817],
+...> [0.6383000016212463, 0.8794000148773193, 0.5282999873161316],
+...> [0.9523000121116638, 0.7597000002861023, 0.08250000327825546],
+...> [0.6622999906539917, 0.02329999953508377, 0.8205999732017517],
+...> [0.9855999946594238, 0.36419999599456787, 0.5372999906539917]
+...> ])
+iex> Axon.Layers.embedding(input, kernels)
+#Nx.Tensor<
+ f32[2][4][3]
+ [
+ [
+ [0.9801999926567078, 0.09780000150203705, 0.5333999991416931],
+ [0.6980000138282776, 0.9240999817848206, 0.23479999601840973],
+ [0.5519000291824341, 0.5662999749183655, 0.20559999346733093],
+ [0.1898999959230423, 0.9311000108718872, 0.8356000185012817]
+ ],
+ [
+ [0.5519000291824341, 0.5662999749183655, 0.20559999346733093],
+ [0.31929999589920044, 0.42250001430511475, 0.7865999937057495],
+ [0.6980000138282776, 0.9240999817848206, 0.23479999601840973],
+ [0.9855999946594238, 0.36419999599456787, 0.5372999906539917]
+ ]
+ ]
+>
iex> Axon.Layers.global_avg_pool(Nx.iota({3, 2, 3}, type: {:f, 32}), channels: :first)
-#Nx.Tensor<
- f32[3][2]
- [
- [1.0, 4.0],
- [7.0, 10.0],
- [13.0, 16.0]
- ]
->
-
-iex> Axon.Layers.global_avg_pool(Nx.iota({1, 3, 2, 2}, type: {:f, 32}), channels: :first, keep_axes: true)
-#Nx.Tensor<
- f32[1][3][1][1]
- [
- [
- [
- [1.5]
- ],
- [
- [5.5]
- ],
- [
- [9.5]
- ]
- ]
- ]
->
+iex> Axon.Layers.global_avg_pool(Nx.iota({3, 2, 3}, type: {:f, 32}), channels: :first)
+#Nx.Tensor<
+ f32[3][2]
+ [
+ [1.0, 4.0],
+ [7.0, 10.0],
+ [13.0, 16.0]
+ ]
+>
+
+iex> Axon.Layers.global_avg_pool(Nx.iota({1, 3, 2, 2}, type: {:f, 32}), channels: :first, keep_axes: true)
+#Nx.Tensor<
+ f32[1][3][1][1]
+ [
+ [
+ [
+ [1.5]
+ ],
+ [
+ [5.5]
+ ],
+ [
+ [9.5]
+ ]
+ ]
+ ]
+>
iex> Axon.Layers.global_lp_pool(Nx.iota({3, 2, 3}, type: {:f, 32}), norm: 1, channels: :first)
-#Nx.Tensor<
- f32[3][2]
- [
- [3.0, 12.0],
- [21.0, 30.0],
- [39.0, 48.0]
- ]
->
-
-iex> Axon.Layers.global_lp_pool(Nx.iota({1, 3, 2, 2}, type: {:f, 16}), keep_axes: true, channels: :first)
-#Nx.Tensor<
- f16[1][3][1][1]
- [
- [
- [
- [3.7421875]
- ],
- [
- [11.2265625]
- ],
- [
- [19.125]
- ]
- ]
- ]
->
+iex> Axon.Layers.global_lp_pool(Nx.iota({3, 2, 3}, type: {:f, 32}), norm: 1, channels: :first)
+#Nx.Tensor<
+ f32[3][2]
+ [
+ [3.0, 12.0],
+ [21.0, 30.0],
+ [39.0, 48.0]
+ ]
+>
+
+iex> Axon.Layers.global_lp_pool(Nx.iota({1, 3, 2, 2}, type: {:f, 16}), keep_axes: true, channels: :first)
+#Nx.Tensor<
+ f16[1][3][1][1]
+ [
+ [
+ [
+ [3.7421875]
+ ],
+ [
+ [11.2265625]
+ ],
+ [
+ [19.125]
+ ]
+ ]
+ ]
+>
iex> Axon.Layers.global_max_pool(Nx.iota({3, 2, 3}, type: {:f, 32}), channels: :first)
-#Nx.Tensor<
- f32[3][2]
- [
- [2.0, 5.0],
- [8.0, 11.0],
- [14.0, 17.0]
- ]
->
-
-iex> Axon.Layers.global_max_pool(Nx.iota({1, 3, 2, 2}, type: {:f, 32}), keep_axes: true, channels: :first)
-#Nx.Tensor<
- f32[1][3][1][1]
- [
- [
- [
- [3.0]
- ],
- [
- [7.0]
- ],
- [
- [11.0]
- ]
- ]
- ]
->
+iex> Axon.Layers.global_max_pool(Nx.iota({3, 2, 3}, type: {:f, 32}), channels: :first)
+#Nx.Tensor<
+ f32[3][2]
+ [
+ [2.0, 5.0],
+ [8.0, 11.0],
+ [14.0, 17.0]
+ ]
+>
+
+iex> Axon.Layers.global_max_pool(Nx.iota({1, 3, 2, 2}, type: {:f, 32}), keep_axes: true, channels: :first)
+#Nx.Tensor<
+ f32[1][3][1][1]
+ [
+ [
+ [
+ [3.0]
+ ],
+ [
+ [7.0]
+ ],
+ [
+ [11.0]
+ ]
+ ]
+ ]
+>
iex> t = Nx.tensor([[[0.9450, 0.4684, 1.8146], [1.2663, 0.4354, -0.0781], [-0.4759, 0.3251, 0.8742]]], type: {:f, 32})
-iex> Axon.Layers.lp_pool(t, kernel_size: 2, norm: 2, channels: :first)
-#Nx.Tensor<
- f32[1][3][1]
- [
- [
- [1.0547149181365967],
- [1.3390626907348633],
- [0.5763426423072815]
- ]
- ]
->
+iex> t = Nx.tensor([[[0.9450, 0.4684, 1.8146], [1.2663, 0.4354, -0.0781], [-0.4759, 0.3251, 0.8742]]], type: {:f, 32})
+iex> Axon.Layers.lp_pool(t, kernel_size: 2, norm: 2, channels: :first)
+#Nx.Tensor<
+ f32[1][3][1]
+ [
+ [
+ [1.0547149181365967],
+ [1.3390626907348633],
+ [0.5763426423072815]
+ ]
+ ]
+>
iex> t = Nx.tensor([[
-...> [0.051500000059604645, -0.7042999863624573, -0.32899999618530273],
-...> [-0.37130001187324524, 1.6191999912261963, -0.11829999834299088],
-...> [0.7099999785423279, 0.7282999753952026, -0.18639999628067017]]], type: {:f, 32})
-iex> Axon.Layers.max_pool(t, kernel_size: 2, channels: :first)
-#Nx.Tensor<
- f32[1][3][1]
- [
- [
- [0.051500000059604645],
- [1.6191999912261963],
- [0.7282999753952026]
- ]
- ]
->
+iex> t = Nx.tensor([[
+...> [0.051500000059604645, -0.7042999863624573, -0.32899999618530273],
+...> [-0.37130001187324524, 1.6191999912261963, -0.11829999834299088],
+...> [0.7099999785423279, 0.7282999753952026, -0.18639999628067017]]], type: {:f, 32})
+iex> Axon.Layers.max_pool(t, kernel_size: 2, channels: :first)
+#Nx.Tensor<
+ f32[1][3][1]
+ [
+ [
+ [0.051500000059604645],
+ [1.6191999912261963],
+ [0.7282999753952026]
+ ]
+ ]
+>
iex> Axon.Layers.flatten(Nx.iota({1, 2, 2}, type: {:f, 32}))
-#Nx.Tensor<
- f32[1][4]
- [
- [0.0, 1.0, 2.0, 3.0]
- ]
->
+iex> Axon.Layers.flatten(Nx.iota({1, 2, 2}, type: {:f, 32}))
+#Nx.Tensor<
+ f32[1][4]
+ [
+ [0.0, 1.0, 2.0, 3.0]
+ ]
+>
iex> img = Nx.iota({1, 1, 3, 3}, type: {:f, 32})
-iex> Axon.Layers.resize(img, size: {4, 4}, channels: :first)
-#Nx.Tensor<
- f32[1][1][4][4]
- [
- [
- [
- [0.0, 1.0, 1.0, 2.0],
- [3.0, 4.0, 4.0, 5.0],
- [3.0, 4.0, 4.0, 5.0],
- [6.0, 7.0, 7.0, 8.0]
- ]
- ]
- ]
->
iex> img = Nx.iota({1, 1, 3, 3}, type: {:f, 32})
+iex> Axon.Layers.resize(img, size: {4, 4}, channels: :first)
+#Nx.Tensor<
+ f32[1][1][4][4]
+ [
+ [
+ [
+ [0.0, 1.0, 1.0, 2.0],
+ [3.0, 4.0, 4.0, 5.0],
+ [3.0, 4.0, 4.0, 5.0],
+ [6.0, 7.0, 7.0, 8.0]
+ ]
+ ]
+ ]
+>
error-cases
Error casesiex> img = Nx.iota({1, 1, 3, 3}, type: {:f, 32})
-iex> Axon.Layers.resize(img, size: {4, 4}, method: :foo)
+iex> img = Nx.iota({1, 1, 3, 3}, type: {:f, 32})
+iex> Axon.Layers.resize(img, size: {4, 4}, method: :foo)
** (ArgumentError) expected :method to be either of :nearest, :bilinear, :bicubic, :lanczos3, :lanczos5, got: :foo
iex> input = Nx.tensor([[[0.1294, -0.6638, 1.0251]], [[ 0.9182, 1.1512, -1.6149]]], type: {:f, 32})
-iex> kernel = Nx.tensor([[[-1.5475, 1.2425]], [[0.1871, 0.5458]], [[-0.4488, 0.8879]]], type: {:f, 32})
-iex> bias = Nx.tensor([0.7791, 0.1676, 1.5971], type: {:f, 32})
-iex> Axon.Layers.conv(input, kernel, bias, channels: :first)
-#Nx.Tensor<
- f32[2][3][2]
- [
- [
- [-0.24591797590255737, 3.08001708984375],
- [-0.1704912781715393, 0.6029025316238403],
- [0.9496372938156128, 2.80519962310791]
- ],
- [
- [0.7885514497756958, -3.0088953971862793],
- [0.9677201509475708, -0.4984228312969208],
- [2.207162380218506, -0.3534282445907593]
- ]
- ]
->
iex> input = Nx.tensor([[[0.1294, -0.6638, 1.0251]], [[ 0.9182, 1.1512, -1.6149]]], type: {:f, 32})
+iex> kernel = Nx.tensor([[[-1.5475, 1.2425]], [[0.1871, 0.5458]], [[-0.4488, 0.8879]]], type: {:f, 32})
+iex> bias = Nx.tensor([0.7791, 0.1676, 1.5971], type: {:f, 32})
+iex> Axon.Layers.conv(input, kernel, bias, channels: :first)
+#Nx.Tensor<
+ f32[2][3][2]
+ [
+ [
+ [-0.24591797590255737, 3.08001708984375],
+ [-0.1704912781715393, 0.6029025316238403],
+ [0.9496372938156128, 2.80519962310791]
+ ],
+ [
+ [0.7885514497756958, -3.0088953971862793],
+ [0.9677201509475708, -0.4984228312969208],
+ [2.207162380218506, -0.3534282445907593]
+ ]
+ ]
+>
two-dimensional-convolution
Two-dimensional convolutioniex> input = Nx.tensor([[[[-1.0476, -0.5041], [-0.9336, 1.5907]]]], type: {:f, 32})
-iex> kernel = Nx.tensor([
-...> [[[0.7514, 0.7356], [1.3909, 0.6800]]],
-...> [[[-0.3450, 0.4551], [-0.6275, -0.9875]]],
-...> [[[1.8587, 0.4722], [0.6058, -1.0301]]]
-...> ], type: {:f, 32})
-iex> bias = Nx.tensor([1.9564, 0.2822, -0.5385], type: {:f, 32})
-iex> Axon.Layers.conv(input, kernel, bias, channels: :first)
-#Nx.Tensor<
- f32[1][3][1][1]
- [
- [
- [
- [0.5815491676330566]
- ],
- [
- [-0.5707762241363525]
- ],
- [
- [-4.927865028381348]
- ]
- ]
- ]
->
iex> input = Nx.tensor([[[[-1.0476, -0.5041], [-0.9336, 1.5907]]]], type: {:f, 32})
+iex> kernel = Nx.tensor([
+...> [[[0.7514, 0.7356], [1.3909, 0.6800]]],
+...> [[[-0.3450, 0.4551], [-0.6275, -0.9875]]],
+...> [[[1.8587, 0.4722], [0.6058, -1.0301]]]
+...> ], type: {:f, 32})
+iex> bias = Nx.tensor([1.9564, 0.2822, -0.5385], type: {:f, 32})
+iex> Axon.Layers.conv(input, kernel, bias, channels: :first)
+#Nx.Tensor<
+ f32[1][3][1][1]
+ [
+ [
+ [
+ [0.5815491676330566]
+ ],
+ [
+ [-0.5707762241363525]
+ ],
+ [
+ [-4.927865028381348]
+ ]
+ ]
+ ]
+>
three-dimensional-convolution
Three-dimensional convolutioniex> input = Nx.tensor([[[[[-0.6497], [1.0939]], [[-2.5465], [0.7801]]]]], type: {:f, 32})
-iex> kernel = Nx.tensor([
-...> [[[[ 0.7390], [-0.0927]], [[-0.8675], [-0.9209]]]],
-...> [[[[-0.6638], [0.4341]], [[0.6368], [1.1846]]]]
-...> ], type: {:f, 32})
-iex> bias = Nx.tensor([-0.4101, 0.1776], type: {:f, 32})
-iex> Axon.Layers.conv(input, kernel, bias, channels: :first)
-#Nx.Tensor<
- f32[1][2][1][1][1]
- [
- [
- [
- [
- [0.49906185269355774]
- ]
- ],
- [
- [
- [0.38622811436653137]
- ]
- ]
- ]
- ]
->
+iex> input = Nx.tensor([[[[[-0.6497], [1.0939]], [[-2.5465], [0.7801]]]]], type: {:f, 32})
+iex> kernel = Nx.tensor([
+...> [[[[ 0.7390], [-0.0927]], [[-0.8675], [-0.9209]]]],
+...> [[[[-0.6638], [0.4341]], [[0.6368], [1.1846]]]]
+...> ], type: {:f, 32})
+iex> bias = Nx.tensor([-0.4101, 0.1776], type: {:f, 32})
+iex> Axon.Layers.conv(input, kernel, bias, channels: :first)
+#Nx.Tensor<
+ f32[1][2][1][1][1]
+ [
+ [
+ [
+ [
+ [0.49906185269355774]
+ ]
+ ],
+ [
+ [
+ [0.38622811436653137]
+ ]
+ ]
+ ]
+ ]
+>
iex> input = Nx.iota({1, 3, 3}, type: {:f, 32})
-iex> kernel = Nx.iota({6, 3, 2}, type: {:f, 32})
-iex> bias = Nx.tensor(1.0, type: {:f, 32})
-iex> Axon.Layers.conv_transpose(input, kernel, bias, channels: :first)
-#Nx.Tensor<
- f32[1][6][4]
- [
- [
- [40.0, 79.0, 94.0, 43.0],
- [94.0, 205.0, 256.0, 133.0],
- [148.0, 331.0, 418.0, 223.0],
- [202.0, 457.0, 580.0, 313.0],
- [256.0, 583.0, 742.0, 403.0],
- [310.0, 709.0, 904.0, 493.0]
- ]
- ]
->
iex> input = Nx.iota({1, 3, 3}, type: {:f, 32})
+iex> kernel = Nx.iota({6, 3, 2}, type: {:f, 32})
+iex> bias = Nx.tensor(1.0, type: {:f, 32})
+iex> Axon.Layers.conv_transpose(input, kernel, bias, channels: :first)
+#Nx.Tensor<
+ f32[1][6][4]
+ [
+ [
+ [40.0, 79.0, 94.0, 43.0],
+ [94.0, 205.0, 256.0, 133.0],
+ [148.0, 331.0, 418.0, 223.0],
+ [202.0, 457.0, 580.0, 313.0],
+ [256.0, 583.0, 742.0, 403.0],
+ [310.0, 709.0, 904.0, 493.0]
+ ]
+ ]
+>
references
diff --git a/Axon.Loop.State.html b/Axon.Loop.State.html index f7ff00b6..974fffdb 100644 --- a/Axon.Loop.State.html +++ b/Axon.Loop.State.html @@ -112,16 +112,16 @@Accumulated state in an Axon.Loop.
Loop state is a struct:
%State{
- epoch: integer(),
- max_epoch: integer(),
- iteration: integer(),
- max_iteration: integer(),
- metrics: map(string(), container()),
- times: map(integer(), integer()),
- step_state: container(),
- handler_metadata: container()
-}
epoch
is the current epoch, starting at 0, of the nested loop.
+
Accumulated state in an Axon.Loop.
Loop state is a struct:
%State{
+ epoch: integer(),
+ max_epoch: integer(),
+ iteration: integer(),
+ max_iteration: integer(),
+ metrics: map(string(), container()),
+ times: map(integer(), integer()),
+ step_state: container(),
+ handler_metadata: container()
+}
epoch
is the current epoch, starting at 0, of the nested loop.
Defaults to 0.
max_epoch
is the maximum number of epochs the loop should run
for. Defaults to 1.
iteration
is the current iteration of the inner loop. In supervised
settings, this will be the current batch. Defaults to 0.
max_iteration
is the maximum number of iterations the loop should
diff --git a/Axon.Loop.html b/Axon.Loop.html
index 0e34fe69..1a4403ba 100644
--- a/Axon.Loop.html
+++ b/Axon.Loop.html
@@ -114,66 +114,66 @@
Abstraction for modeling a reduction of a dataset with an accumulated state for a number of epochs.
Inspired heavily by PyTorch Ignite.
The main abstraction is the %Axon.Loop{}
struct, which controls a nested
-reduction of the form:
Enum.reduce(1..max_epochs, state, fn epoch, state ->
- Enum.reduce(data, state, &batch_step/2)
-end)
data
is assumed to be an Enumerable
or Stream
of input data which is
+reduction of the form:
Enum.reduce(1..max_epochs, state, fn epoch, state ->
+ Enum.reduce(data, state, &batch_step/2)
+end)
data
is assumed to be an Enumerable
or Stream
of input data which is
handled by a processing function, batch_step
. The purpose of the loop
abstraction is to take away much of the boilerplate code used in solving machine
learning tasks. Tasks such as normalizing a dataset, hyperparameter optimization,
-or training machine learning models boil down to writing one function:
defn batch_step(batch, state) do
+or training machine learning models boil down to writing one function:defn batch_step(batch, state) do
# ...do something with batch...
updated_state
-end
For tasks such as training a neural network, state
will encapsulate things
+
end
For tasks such as training a neural network, state
will encapsulate things
such as model and optimizer state. For supervised learning tasks, batch_step
-might look something like:
defn batch_step({inputs, targets}, state) do
- %{parameters: params, optimizer_state: optim_state} = state
+might look something like:defn batch_step({inputs, targets}, state) do
+ %{parameters: params, optimizer_state: optim_state} = state
- gradients = grad(params, objective_fn.(&1, inputs, targets))
- {updates, new_optim_state} = optimizer.(optim_state, params, gradients)
+ gradients = grad(params, objective_fn.(&1, inputs, targets))
+ {updates, new_optim_state} = optimizer.(optim_state, params, gradients)
- new_params = apply_updates(params, updates)
+ new_params = apply_updates(params, updates)
- %{parameters: new_params, optimizer_state: optim_state}
-end
batch_step
takes a batch of {input, target}
pairs and the current state,
+
%{parameters: new_params, optimizer_state: optim_state}
+end
batch_step
takes a batch of {input, target}
pairs and the current state,
and updates the model parameters based on the gradients received from some arbitrary
objective function. This function will run in a nested loop, iterating over the entire
dataset for N
epochs before finally returning the trained model state. By defining
1 function, we've created a training loop that works for most machine learning models.
In actuality, the loop abstraction accumulates a struct, %Axon.Loop.State{}
, which looks
-like (assuming container
is a generic Elixir container of tensors, e.g. map, tuple, etc.):
%Axon.Loop.State{
- epoch: integer(),
- max_epoch: integer(),
- iteration: integer(),
- max_iteration: integer(),
- metrics: map(string(), container()),
- times: map(integer(), integer()),
- step_state: container()
-}
batch_step
takes in the batch and the step state field and returns a step_state
,
+like (assuming container
is a generic Elixir container of tensors, e.g. map, tuple, etc.):
%Axon.Loop.State{
+ epoch: integer(),
+ max_epoch: integer(),
+ iteration: integer(),
+ max_iteration: integer(),
+ metrics: map(string(), container()),
+ times: map(integer(), integer()),
+ step_state: container()
+}
batch_step
takes in the batch and the step state field and returns a step_state
,
which is a generic container of state accumulated at each iteration. The rest of the fields
in the state struct are updated automatically behind the scenes.
The loop must start from some initial step state, thus most tasks must also provide an additional initialization function to provide some starting point for the step state. For machine learning tasks, the initialization function will return things like initial model parameters and optimizer state.
Typically, the final output of the loop is the accumulated final state; however, you
may optionally apply an output transform to extract specific values at the end of the
-loop. For example, Axon.Loop.trainer/4
by default extracts trained model state:
output_transform = fn state ->
- state.step_state[:model_state]
-end
Axon.Loop.trainer/4
by default extracts trained model state:output_transform = fn state ->
+ state.step_state[:model_state]
+end
initialize-and-step
Initialize and StepThe core of the Axon loop are the init and step functions. The initialization is an -arity-0 function which provides an initial step state:
init = fn ->
- %{params: Axon.init(model)}
-end
While the step function is the batch_step
function mentioned earlier:
step = fn data, state ->
+arity-0 function which provides an initial step state:init = fn ->
+ %{params: Axon.init(model)}
+end
While the step function is the batch_step
function mentioned earlier:
step = fn data, state ->
new_state = # ...do something...
new_state
-end
Note that any optimization and training anonymous functions that need to be used in the
-batch_step
function can be passed as extra arguments. For example:
step_with_training_arguments = fn data, state, optimizer_update_fn, state_update_fn ->
+end
Note that any optimization and training anonymous functions that need to be used in the
+batch_step
function can be passed as extra arguments. For example:
step_with_training_arguments = fn data, state, optimizer_update_fn, state_update_fn ->
# ...do something...
-end
+end
-step = &(step_with_training_arguments.(&1, &2, actual_optimizer_update_fn, actual_state_update_fn))
+
step = &(step_with_training_arguments.(&1, &2, actual_optimizer_update_fn, actual_state_update_fn))
metrics
@@ -181,27 +181,27 @@Often times you want to compute metrics associated with your training iterations.
To accomplish this, you can attach metrics to each Axon.Loop
. Assuming a batch_step
-function which looks like:
defn batch_step({inputs, targets}, state) do
- %{parameters: params, optimizer_state: optim_state} = state
+function which looks like:defn batch_step({inputs, targets}, state) do
+ %{parameters: params, optimizer_state: optim_state} = state
- gradients = grad(params, objective_fn.(&1, inputs, targets))
- {updates, new_optim_state} = optimizer.(optim_state, params, gradients)
+ gradients = grad(params, objective_fn.(&1, inputs, targets))
+ {updates, new_optim_state} = optimizer.(optim_state, params, gradients)
- new_params = apply_updates(params, updates)
+ new_params = apply_updates(params, updates)
# Shown for simplicity, you can optimize this by calculating preds
# along with the gradient calculation
- preds = model_fn.(params, inputs)
+ preds = model_fn.(params, inputs)
- %{
+ %{
y_true: targets,
y_pred: preds,
parameters: new_params,
optimizer_state: optim_state
- }
-end
You can attach metrics to this by using Axon.Loop.metric/4
:
Axon.Loop.loop(&batch_step/2)
-|> Axon.Loop.metric("Accuracy", :accuracy, fn %{y_true: y_, y_pred: y} -> [y_, y] end)
-|> Axon.Loop.run(data)
Because metrics work directly on step_state
, you typically need to provide an output
+
}
+end
You can attach metrics to this by using Axon.Loop.metric/4
:
Axon.Loop.loop(&batch_step/2)
+|> Axon.Loop.metric("Accuracy", :accuracy, fn %{y_true: y_, y_pred: y} -> [y_, y] end)
+|> Axon.Loop.run(data)
Because metrics work directly on step_state
, you typically need to provide an output
transform to indicate which values should be passed to your metric function. By default,
Axon assumes a supervised training task with the fields :y_true
and :y_pred
present
in the step state. See Axon.Loop.metric/4
for more information.
Metrics will be tracked in the loop state using the user-provided key. Metrics integrate @@ -213,24 +213,24 @@
You can instrument several points in the loop using event handlers. By default, several events -are fired when running a loop:
events = [
+are fired when running a loop:events = [
:started, # After loop state initialization
:epoch_started, # On epoch start
:iteration_started, # On iteration start
:iteration_completed, # On iteration complete
:epoch_completed, # On epoch complete
:epoch_halted, # On epoch halt, if early halted
-]
You can attach event handlers to events using Axon.Loop.handle_event/4
:
loop
-|> Axon.Loop.handle_event(:iteration_completed, &log_metrics/1, every: 100)
-|> Axon.Loop.run(data)
The above will trigger log_metrics/1
every 100 times the :iteration_completed
event
+
]
You can attach event handlers to events using Axon.Loop.handle_event/4
:
loop
+|> Axon.Loop.handle_event(:iteration_completed, &log_metrics/1, every: 100)
+|> Axon.Loop.run(data)
The above will trigger log_metrics/1
every 100 times the :iteration_completed
event
is fired. Event handlers must return a tuple {status, state}
, where status
is an
atom with one of the following values:
:continue # Continue epoch, continue looping
:halt_epoch # Halt the epoch, continue looping
:halt_loop # Halt looping
And state
is an updated Axon.Loop.State
struct. Handler functions take as input
the current loop state.
It's important to note that event handlers are triggered in the order they are attached to the loop. If you have two handlers on the same event, they will trigger in order:
loop
-|> Axon.Loop.handle_event(:epoch_completed, &normalize_state/1) # Runs first
-|> Axon.Loop.handle_event(:epoch_completed, &log_state/1) # Runs second
You may provide filters to filter when event handlers trigger. See Axon.Loop.handle_event/4
+|> Axon.Loop.handle_event(:epoch_completed, &normalize_state/1) # Runs first
+|> Axon.Loop.handle_event(:epoch_completed, &log_state/1) # Runs second
You may provide filters to filter when event handlers trigger. See Axon.Loop.handle_event/4
for more details on valid filters.
factories
@@ -250,7 +250,7 @@In order to execute a loop, you should use Axon.Loop.run/3
:
Axon.Loop.run(loop, data, epochs: 10)
In order to execute a loop, you should use Axon.Loop.run/3
:
Axon.Loop.run(loop, data, epochs: 10)
resuming-loops
@@ -258,8 +258,8 @@At times you may want to resume a loop from some previous state. You can accomplish this
with Axon.Loop.from_state/2
:
loop
-|> Axon.Loop.from_state(state)
-|> Axon.Loop.run(data)
+|> Axon.Loop.from_state(state)
+|> Axon.Loop.run(data)
Axon.Loop.serialize_state/2
. Serialization
options will be forwarded to Axon.Loop.serialize_state/2
.You can customize checkpoint events by passing :event
and :filter
options:
loop
-|> Axon.Loop.checkpoint(event: :iteration_completed, filter: [every: 50])
Checkpoints are saved under the checkpoint/
directory with a pattern
+|> Axon.Loop.checkpoint(event: :iteration_completed, filter: [every: 50])
Checkpoints are saved under the checkpoint/
directory with a pattern
of checkpoint_{epoch}.ckpt
. You can customize the path and pattern
with the :path
and :file_pattern
options:
my_file_pattern =
- fn %Axon.Loop.State{epoch: epoch, iteration: iter} ->
- "checkpoint_#{epoch}_#{iter}"
- end
+ fn %Axon.Loop.State{epoch: epoch, iteration: iter} ->
+ "checkpoint_#{epoch}_#{iter}"
+ end
loop
-|> Axon.Loop.checkpoint(path: "my_checkpoints", file_pattern: my_file_pattern)
If you'd like to only save checkpoints based on some metric criteria, +|> Axon.Loop.checkpoint(path: "my_checkpoints", file_pattern: my_file_pattern)
If you'd like to only save checkpoints based on some metric criteria,
you can specify the :criteria
option. :criteria
must be a valid key
in metrics:
loop
-|> Axon.Loop.checkpoint(criteria: "validation_loss")
The default criteria mode is :min
, meaning the min score metric will
+|> Axon.Loop.checkpoint(criteria: "validation_loss")
The default criteria mode is :min
, meaning the min score metric will
be considered "best" when deciding to save on a given event. Valid modes
are :min
and :max
:
loop
-|> Axon.Loop.checkpoint(criteria: "validation_accuracy", mode: :max)
options
@@ -596,18 +596,18 @@You must specify a metric to monitor and the metric must be present in the loop state. Typically, this will be a validation metric:
model
-|> Axon.Loop.trainer(loss, optim)
-|> Axon.Loop.metric(:accuracy)
-|> Axon.Loop.validate(val_data)
-|> Axon.Loop.early_stop("validation_accuracy")
It's important to remember that handlers are executed in the +|> Axon.Loop.trainer(loss, optim) +|> Axon.Loop.metric(:accuracy) +|> Axon.Loop.validate(val_data) +|> Axon.Loop.early_stop("validation_accuracy")
It's important to remember that handlers are executed in the order they are added to the loop. For example, if you'd like to checkpoint a loop after every epoch and use early stopping, most likely you want to add the checkpoint handler before the early stopping handler:
model
-|> Axon.Loop.trainer(loss, optim)
-|> Axon.Loop.metric(:accuracy)
-|> Axon.Loop.checkpoint()
-|> Axon.Loop.early_stop("accuracy")
That will ensure checkpoint is always fired, even if the loop +|> Axon.Loop.trainer(loss, optim) +|> Axon.Loop.metric(:accuracy) +|> Axon.Loop.checkpoint() +|> Axon.Loop.early_stop("accuracy")
That will ensure checkpoint is always fired, even if the loop exited early.
@@ -658,18 +658,18 @@Creates a supervised evaluator from a model.
An evaluator can be used for things such as testing and validation of models
after or during training. It assumes model
is an Axon struct, container of
structs, or a tuple of init
/ apply
functions. model_state
must be a
-container usable from within model
.
The evaluator returns a step state of the form:
%{
+container usable from within model
.The evaluator returns a step state of the form:
%{
y_true: labels,
y_pred: predictions
-}
Such that you can attach any number of supervised metrics to the evaluation
+
}
Such that you can attach any number of supervised metrics to the evaluation loop:
model
-|> Axon.Loop.evaluator()
-|> Axon.Loop.metric("Accuracy", :accuracy)
You must pass a compatible trained model state to Axon.Loop.run/4
when using
+|> Axon.Loop.evaluator()
+|> Axon.Loop.metric("Accuracy", :accuracy)
You must pass a compatible trained model state to Axon.Loop.run/4
when using
supervised evaluation loops. For example, if you've binded the result of a training
run to trained_model_state
, you can run the trained model through an evaluation
run like this:
model
-|> Axon.Loop.evaluator()
-|> Axon.Loop.run(data, trained_model_state, compiler: EXLA)
This function applies an output transform which returns the map of metrics accumulated +|> Axon.Loop.evaluator() +|> Axon.Loop.run(data, trained_model_state, compiler: EXLA)
This function applies an output transform which returns the map of metrics accumulated over the given loop.
@@ -694,7 +694,7 @@Attaches state
to the given loop in order to resume looping
from a previous state.
It's important to note that a loop's attached state takes precedence -over defined initialization functions. Given initialization function:
defn init_state(), do: %{foo: 1, bar: 2}
And an attached state:
state = %State{step_state: %{foo: 2, bar: 3}}
init_state/0
will never execute, and instead the initial step state
+over defined initialization functions. Given initialization function:
defn init_state(), do: %{foo: 1, bar: 2}
And an attached state:
state = %State{step_state: %{foo: 2, bar: 3}}
init_state/0
will never execute, and instead the initial step state
of %{foo: 2, bar: 3}
will be used.
Adds a handler function to the loop which will be triggered on event
with an optional filter.
Events take place at different points during loop execution. The default -events are:
events = [
+events are:events = [
:started, # After loop state initialization
:epoch_started, # On epoch start
:iteration_started, # On iteration start
:iteration_completed, # On iteration complete
:epoch_completed, # On epoch complete
:epoch_halted, # On epoch halt, if early halted
-]
Generally, event handlers are side-effecting operations which provide some
+
]
Generally, event handlers are side-effecting operations which provide some sort of inspection into the loop's progress. It's important to note that if you define multiple handlers to be triggered on the same event, they will execute in order from when they were attached to the training loop:
loop
-|> Axon.Loop.handle_event(:epoch_started, &normalize_step_state/1) # executes first
-|> Axon.Loop.handle_event(:epoch_started, &log_step_state/1) # executes second
Thus, if you have separate handlers which alter or depend on loop state, +|> Axon.Loop.handle_event(:epoch_started, &normalize_step_state/1) # executes first +|> Axon.Loop.handle_event(:epoch_started, &log_step_state/1) # executes second
Thus, if you have separate handlers which alter or depend on loop state, you need to ensure they are ordered correctly, or combined into a single event handler for maximum control over execution.
event
must be an atom representing the event to trigger handler
or a
list of atoms indicating handler
should be triggered on multiple events.
@@ -775,16 +775,16 @@
Adds a handler function which updates a Kino.VegaLite
plot.
By default, this will run after every iteration.
You must specify a plot to push to and a metric to track. The :x
axis will be the iteration count, labeled "step"
. The metric must match the name given to the :y
axis in your VegaLite
plot:
plot =
- Vl.new()
- |> Vl.mark(:line)
- |> Vl.encode_field(:x, "step", type: :quantitative)
- |> Vl.encode_field(:y, "loss", type: :quantitative)
- |> Kino.VegaLite.new()
- |> Kino.render()
+ Vl.new()
+ |> Vl.mark(:line)
+ |> Vl.encode_field(:x, "step", type: :quantitative)
+ |> Vl.encode_field(:y, "loss", type: :quantitative)
+ |> Kino.VegaLite.new()
+ |> Kino.render()
model
-|> Axon.Loop.trainer(loss, optim)
-|> Axon.Loop.kino_vega_lite_plot(plot, "loss")
options
@@ -849,13 +849,13 @@Creates a loop from step_fn
, an optional init_fn
, and an
optional output_transform
.
step_fn
is an arity-2 function which takes a batch and state
-and returns an updated step state:
defn batch_step(batch, step_state) do
+and returns an updated step state:defn batch_step(batch, step_state) do
step_state + 1
-end
init_fn
by default is an identity function which forwards its
+
end
init_fn
by default is an identity function which forwards its
initial arguments as the model state. You should define a custom
-initialization function if you require a different behavior:
defn init_step_state(state) do
- Map.merge(%{foo: 1}, state)
-end
You may use state
in conjunction with initialization functions in
+initialization function if you require a different behavior:
defn init_step_state(state) do
+ Map.merge(%{foo: 1}, state)
+end
You may use state
in conjunction with initialization functions in
init_fn
. For example, train_step/3
uses initial state as initial
model parameters to allow initializing models from partial parameterizations.
step_batch/2
and init_step_state/1
are typically called from
within Nx.Defn.jit/3
. While JIT-compilation will work with anonymous functions,
@@ -893,20 +893,20 @@
Adds a metric of the given name to the loop.
A metric is a function which tracks or measures some value with respect to values in the step state. For example, when training classification models, it's common to track the model's accuracy during training:
loop
-|> Axon.Loop.metric(:accuracy, "Accuracy")
By default, metrics assume a supervised learning task and extract the fields +|> Axon.Loop.metric(:accuracy, "Accuracy")
By default, metrics assume a supervised learning task and extract the fields
[:y_true, :y_pred]
from the step state. If you wish to work on a different
value, you can use an output transform. An output transform is a list of keys
to extract from the output state, or a function which returns a flattened list
of values to pass to the given metric function. Values received from output
-transforms are passed to the given metric using:
value = output_transform.(step_state)
-apply(metric, value)
Thus, even if you want your metric to work on a container, your output transform +transforms are passed to the given metric using:
value = output_transform.(step_state)
+apply(metric, value)
Thus, even if you want your metric to work on a container, your output transform must return a list.
metric
must be an atom which matches the name of a metric in Axon.Metrics
, or
an arbitrary function which returns a tensor or container.
name
must be a string or atom used to store the computed metric in the loop
state. If names conflict, the last attached metric will take precedence:
loop
-|> Axon.Loop.metric(:mean_squared_error, "Error") # Will be overwritten
-|> Axon.Loop.metric(:mean_absolute_error, "Error") # Will be used
By default, metrics keep a running average of the metric calculation. You can +|> Axon.Loop.metric(:mean_squared_error, "Error") # Will be overwritten +|> Axon.Loop.metric(:mean_absolute_error, "Error") # Will be used
By default, metrics keep a running average of the metric calculation. You can
override this behavior by changing accumulate
:
loop
-|> Axon.Loop.metric(:true_negatives, "tn", :running_sum)
Accumulation function can be one of the accumulation combinators in Axon.Metrics +|> Axon.Loop.metric(:true_negatives, "tn", :running_sum)
Accumulation function can be one of the accumulation combinators in Axon.Metrics
or an arity-3 function of the form: accumulate(acc, obs, i) :: new_acc
.
You must specify a metric to monitor and the metric must be present in the loop state. Typically, this will be a validation metric:
model
-|> Axon.Loop.trainer(loss, optim)
-|> Axon.Loop.metric(:accuracy)
-|> Axon.Loop.validate(model, val_data)
-|> Axon.Loop.reduce_lr_on_plateau("accuracy", mode: :max)
options
@@ -1170,13 +1170,13 @@Axon.Updates
for more information on building
optimizers.This function creates a step function which outputs a map consisting of the following
-fields for step_state
:
%{
- y_pred: tensor() | container(tensor()), # Model predictions for use in metrics
- y_true: tensor() | container(tensor()), # True labels for use in metrics
- loss: tensor(), # Running average of loss over epoch
- model_state: container(tensor()), # Model parameters and state
- optimizer_state: container(tensor()) # Optimizer state associated with each parameter
-}
step_state
:%{
+ y_pred: tensor() | container(tensor()), # Model predictions for use in metrics
+ y_true: tensor() | container(tensor()), # True labels for use in metrics
+ loss: tensor(), # Running average of loss over epoch
+ model_state: container(tensor()), # Model parameters and state
+ optimizer_state: container(tensor()) # Optimizer state associated with each parameter
+}
examples
@@ -1188,42 +1188,42 @@data = Stream.zip(input, target)
+data = Stream.zip(input, target)
-model = Axon.input("input", shape: {nil, 32}) |> Axon.dense(1, activation: :sigmoid)
+model = Axon.input("input", shape: {nil, 32}) |> Axon.dense(1, activation: :sigmoid)
model
-|> Axon.Loop.trainer(:binary_cross_entropy, :adam)
-|> Axon.Loop.run(data)
+
|> Axon.Loop.trainer(:binary_cross_entropy, :adam)
+|> Axon.Loop.run(data)
customizing-optimizer
Customizing Optimizermodel
-|> Axon.Loop.trainer(:binary_cross_entropy, Axon.Optimizers.adam(0.05))
-|> Axon.Loop.run(data)
custom-loss
Custom lossloss_fn = fn y_true, y_pred -> Nx.cos(y_true, y_pred) end
+loss_fn = fn y_true, y_pred -> Nx.cos(y_true, y_pred) end
model
-|> Axon.Loop.trainer(loss_fn, Axon.Optimizers.rmsprop(0.01))
-|> Axon.Loop.run(data)
+
|> Axon.Loop.trainer(loss_fn, Axon.Optimizers.rmsprop(0.01))
+|> Axon.Loop.run(data)
multiple-objectives-with-multi-output-model
Multiple objectives with multi-output modelmodel = {Axon.input("input_0", shape: {nil, 1}), Axon.input("input_1", shape: {nil, 2})}
-loss_weights = [mean_squared_error: 0.5, mean_absolute_error: 0.5]
+model = {Axon.input("input_0", shape: {nil, 1}), Axon.input("input_1", shape: {nil, 2})}
+loss_weights = [mean_squared_error: 0.5, mean_absolute_error: 0.5]
model
-|> Axon.Loop.trainer(loss_weights, :sgd)
-|> Axon.Loop.run(data)
+
|> Axon.Loop.trainer(loss_weights, :sgd)
+|> Axon.Loop.run(data)
options
@@ -1264,25 +1264,25 @@This handler assumes the loop state matches the state initialized in a supervised training loop. Typically, you'd call this immediately after creating a supervised training loop:
model
-|> Axon.Loop.trainer(:mean_squared_error, :sgd)
-|> Axon.Loop.validate(model, validation_data)
Please note that you must pass the same (or an equivalent) model +|> Axon.Loop.trainer(:mean_squared_error, :sgd) +|> Axon.Loop.validate(model, validation_data)
Please note that you must pass the same (or an equivalent) model into this method so it can be used during the validation loop. The metrics which are computed are those which are present BEFORE the validation handler was added to the loop. For the following loop:
model
-|> Axon.Loop.trainer(:mean_squared_error, :sgd)
-|> Axon.Loop.metric(:mean_absolute_error)
-|> Axon.Loop.validate(model, validation_data)
-|> Axon.Loop.metric(:binary_cross_entropy)
only :mean_absolute_error
will be computed at validation time.
The returned loop state is altered to contain validation +|> Axon.Loop.trainer(:mean_squared_error, :sgd) +|> Axon.Loop.metric(:mean_absolute_error) +|> Axon.Loop.validate(model, validation_data) +|> Axon.Loop.metric(:binary_cross_entropy)
only :mean_absolute_error
will be computed at validation time.
The returned loop state is altered to contain validation metrics for use in later handlers such as early stopping and model checkpoints. Since the order of execution of event handlers is in the same order they are declared in the training loop, you MUST call this method before any other handler which expects or may use validation metrics.
By default the validation loop runs after every epoch; however, you can customize it by overriding the default event and event filters:
model
-|> Axon.Loop.trainer(:mean_squared_error, :sgd)
-|> Axon.Loop.metric(:mean_absolute_error)
-|> Axon.Loop.validate(model, validation_data, event: :iteration_completed, filter: [every: 10_000])
-|> Axon.Loop.metric(:binary_cross_entropy)
+|> Axon.Loop.trainer(:mean_squared_error, :sgd)
+|> Axon.Loop.metric(:mean_absolute_error)
+|> Axon.Loop.validate(model, validation_data, event: :iteration_completed, filter: [every: 10_000])
+|> Axon.Loop.metric(:binary_cross_entropy)
diff --git a/Axon.LossScale.html b/Axon.LossScale.html
index f62710ba..e210e6cd 100644
--- a/Axon.LossScale.html
+++ b/Axon.LossScale.html
@@ -115,7 +115,7 @@ Implementations of loss-scalers for use in mixed precision training.
Loss scaling is used to prevent underflow when using mixed precision during the model training process. Each loss-scale -implementation here returns a 3-tuple of the functions:
{init_fn, scale_fn, unscale_fn, adjust_fn} = Axon.LossScale.static(Nx.pow(2, 15))
You can use these to scale/unscale loss and gradients as well +implementation here returns a 3-tuple of the functions:
{init_fn, scale_fn, unscale_fn, adjust_fn} = Axon.LossScale.static(Nx.pow(2, 15))
You can use these to scale/unscale loss and gradients as well as adjust the loss scale state.
Axon.Loop.trainer/3
builds loss-scaling in by default. You
can reference the Axon.Loop.train_step/3
implementation to
see how loss-scaling is applied in practice.
y_true
and input prediction y_pred
. As an example, the mean_squared_error/2
loss function produces a tensor whose values are the mean squared
-error between targets and predictions:iex> y_true = Nx.tensor([[0.0, 1.0], [0.0, 0.0]], type: {:f, 32})
-iex> y_pred = Nx.tensor([[1.0, 1.0], [1.0, 0.0]], type: {:f, 32})
-iex> Axon.Losses.mean_squared_error(y_true, y_pred)
-#Nx.Tensor<
- f32[2]
- [0.5, 0.5]
->
It's common to compute the loss across an entire minibatch. +error between targets and predictions:
iex> y_true = Nx.tensor([[0.0, 1.0], [0.0, 0.0]], type: {:f, 32})
+iex> y_pred = Nx.tensor([[1.0, 1.0], [1.0, 0.0]], type: {:f, 32})
+iex> Axon.Losses.mean_squared_error(y_true, y_pred)
+#Nx.Tensor<
+ f32[2]
+ [0.5, 0.5]
+>
It's common to compute the loss across an entire minibatch.
You can easily do so by specifying a :reduction
mode, or
-by composing one of these with an Nx
reduction method:
iex> y_true = Nx.tensor([[0.0, 1.0], [0.0, 0.0]], type: {:f, 32})
-iex> y_pred = Nx.tensor([[1.0, 1.0], [1.0, 0.0]], type: {:f, 32})
-iex> Axon.Losses.mean_squared_error(y_true, y_pred, reduction: :mean)
-#Nx.Tensor<
+by composing one of these with an Nx
reduction method:iex> y_true = Nx.tensor([[0.0, 1.0], [0.0, 0.0]], type: {:f, 32})
+iex> y_pred = Nx.tensor([[1.0, 1.0], [1.0, 0.0]], type: {:f, 32})
+iex> Axon.Losses.mean_squared_error(y_true, y_pred, reduction: :mean)
+#Nx.Tensor<
f32
0.5
->
You can even compose loss functions:
defn my_strange_loss(y_true, y_pred) do
+>
You can even compose loss functions:
defn my_strange_loss(y_true, y_pred) do
y_true
- |> Axon.Losses.mean_squared_error(y_pred)
- |> Axon.Losses.binary_cross_entropy(y_pred)
- |> Nx.sum()
-end
Or, more commonly, you can combine loss functions with penalties for
-regularization:
defn regularized_loss(params, y_true, y_pred) do
- loss = Axon.mean_squared_error(y_true, y_pred)
- penalty = l2_penalty(params)
- Nx.sum(loss) + penalty
-end
All of the functions in this module are implemented as
+
|> Axon.Losses.mean_squared_error(y_pred)
+ |> Axon.Losses.binary_cross_entropy(y_pred)
+ |> Nx.sum()
+end
Or, more commonly, you can combine loss functions with penalties for +regularization:
defn regularized_loss(params, y_true, y_pred) do
+ loss = Axon.mean_squared_error(y_true, y_pred)
+ penalty = l2_penalty(params)
+ Nx.sum(loss) + penalty
+end
All of the functions in this module are implemented as
numerical functions and can be JIT or AOT compiled with
any supported Nx
compiler.
iex> y_true = Nx.tensor([[0, 1], [1, 0], [1, 0]])
-iex> y_pred = Nx.tensor([[0.6811, 0.5565], [0.6551, 0.4551], [0.5422, 0.2648]])
-iex> Axon.Losses.binary_cross_entropy(y_true, y_pred)
-#Nx.Tensor<
- f32[3]
- [0.8644826412200928, 0.5150600075721741, 0.45986634492874146]
->
-
-iex> y_true = Nx.tensor([[0, 1], [1, 0], [1, 0]])
-iex> y_pred = Nx.tensor([[0.6811, 0.5565], [0.6551, 0.4551], [0.5422, 0.2648]])
-iex> Axon.Losses.binary_cross_entropy(y_true, y_pred, reduction: :mean)
-#Nx.Tensor<
+iex> y_true = Nx.tensor([[0, 1], [1, 0], [1, 0]])
+iex> y_pred = Nx.tensor([[0.6811, 0.5565], [0.6551, 0.4551], [0.5422, 0.2648]])
+iex> Axon.Losses.binary_cross_entropy(y_true, y_pred)
+#Nx.Tensor<
+ f32[3]
+ [0.8644826412200928, 0.5150600075721741, 0.45986634492874146]
+>
+
+iex> y_true = Nx.tensor([[0, 1], [1, 0], [1, 0]])
+iex> y_pred = Nx.tensor([[0.6811, 0.5565], [0.6551, 0.4551], [0.5422, 0.2648]])
+iex> Axon.Losses.binary_cross_entropy(y_true, y_pred, reduction: :mean)
+#Nx.Tensor<
f32
0.613136351108551
->
+>
-iex> y_true = Nx.tensor([[0, 1], [1, 0], [1, 0]])
-iex> y_pred = Nx.tensor([[0.6811, 0.5565], [0.6551, 0.4551], [0.5422, 0.2648]])
-iex> Axon.Losses.binary_cross_entropy(y_true, y_pred, reduction: :sum)
-#Nx.Tensor<
+iex> y_true = Nx.tensor([[0, 1], [1, 0], [1, 0]])
+iex> y_pred = Nx.tensor([[0.6811, 0.5565], [0.6551, 0.4551], [0.5422, 0.2648]])
+iex> Axon.Losses.binary_cross_entropy(y_true, y_pred, reduction: :sum)
+#Nx.Tensor<
f32
1.8394089937210083
->
+>
Categorical cross-entropy loss function.
$$l_i = -\sum_i^C \hat{y_i} \cdot \log(y_i)$$
Categorical cross-entropy is typically used for multi-class classifcation problems.
By default, it expects y_pred
to encode a probability distribution along the last
axis. You can specify from_logits: true
to indicate y_pred
is a logits tensor.
# Batch size of 3 with 3 target classes
-y_true = Nx.tensor([0, 2, 1])
-y_pred = Nx.tensor([[0.2, 0.8, 0.0], [0.1, 0.2, 0.7], [0.1, 0.2, 0.7]])
argument-shapes
@@ -497,37 +497,37 @@iex> y_true = Nx.tensor([[0, 1, 0], [0, 0, 1]], type: {:s, 8})
-iex> y_pred = Nx.tensor([[0.05, 0.95, 0], [0.1, 0.8, 0.1]])
-iex> Axon.Losses.categorical_cross_entropy(y_true, y_pred)
-#Nx.Tensor<
- f32[2]
- [0.051293306052684784, 2.3025851249694824]
->
-
-iex> y_true = Nx.tensor([[0, 1, 0], [0, 0, 1]], type: {:s, 8})
-iex> y_pred = Nx.tensor([[0.05, 0.95, 0], [0.1, 0.8, 0.1]])
-iex> Axon.Losses.categorical_cross_entropy(y_true, y_pred, reduction: :mean)
-#Nx.Tensor<
+iex> y_true = Nx.tensor([[0, 1, 0], [0, 0, 1]], type: {:s, 8})
+iex> y_pred = Nx.tensor([[0.05, 0.95, 0], [0.1, 0.8, 0.1]])
+iex> Axon.Losses.categorical_cross_entropy(y_true, y_pred)
+#Nx.Tensor<
+ f32[2]
+ [0.051293306052684784, 2.3025851249694824]
+>
+
+iex> y_true = Nx.tensor([[0, 1, 0], [0, 0, 1]], type: {:s, 8})
+iex> y_pred = Nx.tensor([[0.05, 0.95, 0], [0.1, 0.8, 0.1]])
+iex> Axon.Losses.categorical_cross_entropy(y_true, y_pred, reduction: :mean)
+#Nx.Tensor<
f32
1.1769392490386963
->
+>
-iex> y_true = Nx.tensor([[0, 1, 0], [0, 0, 1]], type: {:s, 8})
-iex> y_pred = Nx.tensor([[0.05, 0.95, 0], [0.1, 0.8, 0.1]])
-iex> Axon.Losses.categorical_cross_entropy(y_true, y_pred, reduction: :sum)
-#Nx.Tensor<
+iex> y_true = Nx.tensor([[0, 1, 0], [0, 0, 1]], type: {:s, 8})
+iex> y_pred = Nx.tensor([[0.05, 0.95, 0], [0.1, 0.8, 0.1]])
+iex> Axon.Losses.categorical_cross_entropy(y_true, y_pred, reduction: :sum)
+#Nx.Tensor<
f32
2.3538784980773926
->
+>
-iex> y_true = Nx.tensor([1, 2], type: {:s, 8})
-iex> y_pred = Nx.tensor([[0.05, 0.95, 0], [0.1, 0.8, 0.1]])
-iex> Axon.Losses.categorical_cross_entropy(y_true, y_pred, reduction: :sum, sparse: true)
-#Nx.Tensor<
+iex> y_true = Nx.tensor([1, 2], type: {:s, 8})
+iex> y_pred = Nx.tensor([[0.05, 0.95, 0], [0.1, 0.8, 0.1]])
+iex> Axon.Losses.categorical_cross_entropy(y_true, y_pred, reduction: :sum, sparse: true)
+#Nx.Tensor<
f32
2.3538784980773926
->
+>
iex> y_true = Nx.tensor([[1, 0, 0], [0, 0, 1]], type: {:s, 8})
-iex> y_pred = Nx.tensor([[0.05300799, 0.21617081, 0.68642382], [0.3754382 , 0.08494169, 0.13442067]])
-iex> Axon.Losses.categorical_hinge(y_true, y_pred)
-#Nx.Tensor<
- f32[2]
- [1.6334158182144165, 1.2410175800323486]
->
-
-iex> y_true = Nx.tensor([[1, 0, 0], [0, 0, 1]], type: {:s, 8})
-iex> y_pred = Nx.tensor([[0.05300799, 0.21617081, 0.68642382], [0.3754382 , 0.08494169, 0.13442067]])
-iex> Axon.Losses.categorical_hinge(y_true, y_pred, reduction: :mean)
-#Nx.Tensor<
+iex> y_true = Nx.tensor([[1, 0, 0], [0, 0, 1]], type: {:s, 8})
+iex> y_pred = Nx.tensor([[0.05300799, 0.21617081, 0.68642382], [0.3754382 , 0.08494169, 0.13442067]])
+iex> Axon.Losses.categorical_hinge(y_true, y_pred)
+#Nx.Tensor<
+ f32[2]
+ [1.6334158182144165, 1.2410175800323486]
+>
+
+iex> y_true = Nx.tensor([[1, 0, 0], [0, 0, 1]], type: {:s, 8})
+iex> y_pred = Nx.tensor([[0.05300799, 0.21617081, 0.68642382], [0.3754382 , 0.08494169, 0.13442067]])
+iex> Axon.Losses.categorical_hinge(y_true, y_pred, reduction: :mean)
+#Nx.Tensor<
f32
1.4372167587280273
->
+>
-iex> y_true = Nx.tensor([[1, 0, 0], [0, 0, 1]], type: {:s, 8})
-iex> y_pred = Nx.tensor([[0.05300799, 0.21617081, 0.68642382], [0.3754382 , 0.08494169, 0.13442067]])
-iex> Axon.Losses.categorical_hinge(y_true, y_pred, reduction: :sum)
-#Nx.Tensor<
+iex> y_true = Nx.tensor([[1, 0, 0], [0, 0, 1]], type: {:s, 8})
+iex> y_pred = Nx.tensor([[0.05300799, 0.21617081, 0.68642382], [0.3754382 , 0.08494169, 0.13442067]])
+iex> Axon.Losses.categorical_hinge(y_true, y_pred, reduction: :sum)
+#Nx.Tensor<
f32
2.8744335174560547
->
+>
iex> y_pred = Nx.tensor([[1.0, 0.0], [1.0, 1.0]])
-iex> y_true = Nx.tensor([[0.0, 1.0], [1.0, 1.0]])
-iex> Axon.Losses.cosine_similarity(y_true, y_pred)
-#Nx.Tensor<
- f32[2]
- [0.0, 1.0000001192092896]
->
+iex> y_pred = Nx.tensor([[1.0, 0.0], [1.0, 1.0]])
+iex> y_true = Nx.tensor([[0.0, 1.0], [1.0, 1.0]])
+iex> Axon.Losses.cosine_similarity(y_true, y_pred)
+#Nx.Tensor<
+ f32[2]
+ [0.0, 1.0000001192092896]
+>
iex> y_true = Nx.tensor([[ 1, 1, -1], [ 1, 1, -1]], type: {:s, 8})
-iex> y_pred = Nx.tensor([[0.45440044, 0.31470688, 0.67920924], [0.24311459, 0.93466766, 0.10914676]])
-iex> Axon.Losses.hinge(y_true, y_pred)
-#Nx.Tensor<
- f32[2]
- [0.9700339436531067, 0.6437881588935852]
->
-
-iex> y_true = Nx.tensor([[ 1, 1, -1], [ 1, 1, -1]], type: {:s, 8})
-iex> y_pred = Nx.tensor([[0.45440044, 0.31470688, 0.67920924], [0.24311459, 0.93466766, 0.10914676]])
-iex> Axon.Losses.hinge(y_true, y_pred, reduction: :mean)
-#Nx.Tensor<
+iex> y_true = Nx.tensor([[ 1, 1, -1], [ 1, 1, -1]], type: {:s, 8})
+iex> y_pred = Nx.tensor([[0.45440044, 0.31470688, 0.67920924], [0.24311459, 0.93466766, 0.10914676]])
+iex> Axon.Losses.hinge(y_true, y_pred)
+#Nx.Tensor<
+ f32[2]
+ [0.9700339436531067, 0.6437881588935852]
+>
+
+iex> y_true = Nx.tensor([[ 1, 1, -1], [ 1, 1, -1]], type: {:s, 8})
+iex> y_pred = Nx.tensor([[0.45440044, 0.31470688, 0.67920924], [0.24311459, 0.93466766, 0.10914676]])
+iex> Axon.Losses.hinge(y_true, y_pred, reduction: :mean)
+#Nx.Tensor<
f32
0.806911051273346
->
+>
-iex> y_true = Nx.tensor([[ 1, 1, -1], [ 1, 1, -1]], type: {:s, 8})
-iex> y_pred = Nx.tensor([[0.45440044, 0.31470688, 0.67920924], [0.24311459, 0.93466766, 0.10914676]])
-iex> Axon.Losses.hinge(y_true, y_pred, reduction: :sum)
-#Nx.Tensor<
+iex> y_true = Nx.tensor([[ 1, 1, -1], [ 1, 1, -1]], type: {:s, 8})
+iex> y_pred = Nx.tensor([[0.45440044, 0.31470688, 0.67920924], [0.24311459, 0.93466766, 0.10914676]])
+iex> Axon.Losses.hinge(y_true, y_pred, reduction: :sum)
+#Nx.Tensor<
f32
1.613822102546692
->
+>
iex> y_true = Nx.tensor([[1], [1.5], [2.0]])
-iex> y_pred = Nx.tensor([[0.8], [1.8], [2.1]])
-iex> Axon.Losses.huber(y_true, y_pred)
-#Nx.Tensor<
- f32[3][1]
- [
- [0.019999997690320015],
- [0.04499998688697815],
- [0.004999990575015545]
- ]
->
-
-iex> y_true = Nx.tensor([[1], [1.5], [2.0]])
-iex> y_pred = Nx.tensor([[0.8], [1.8], [2.1]])
-iex> Axon.Losses.huber(y_true, y_pred, reduction: :mean)
-#Nx.Tensor<
+iex> y_true = Nx.tensor([[1], [1.5], [2.0]])
+iex> y_pred = Nx.tensor([[0.8], [1.8], [2.1]])
+iex> Axon.Losses.huber(y_true, y_pred)
+#Nx.Tensor<
+ f32[3][1]
+ [
+ [0.019999997690320015],
+ [0.04499998688697815],
+ [0.004999990575015545]
+ ]
+>
+
+iex> y_true = Nx.tensor([[1], [1.5], [2.0]])
+iex> y_pred = Nx.tensor([[0.8], [1.8], [2.1]])
+iex> Axon.Losses.huber(y_true, y_pred, reduction: :mean)
+#Nx.Tensor<
f32
0.02333332598209381
->
+>
iex> y_true = Nx.tensor([[0, 1], [0, 0]], type: {:u, 8})
-iex> y_pred = Nx.tensor([[0.6, 0.4], [0.4, 0.6]])
-iex> Axon.Losses.kl_divergence(y_true, y_pred)
-#Nx.Tensor<
- f32[2]
- [0.916289210319519, -3.080907390540233e-6]
->
-
-iex> y_true = Nx.tensor([[0, 1], [0, 0]], type: {:u, 8})
-iex> y_pred = Nx.tensor([[0.6, 0.4], [0.4, 0.6]])
-iex> Axon.Losses.kl_divergence(y_true, y_pred, reduction: :mean)
-#Nx.Tensor<
+iex> y_true = Nx.tensor([[0, 1], [0, 0]], type: {:u, 8})
+iex> y_pred = Nx.tensor([[0.6, 0.4], [0.4, 0.6]])
+iex> Axon.Losses.kl_divergence(y_true, y_pred)
+#Nx.Tensor<
+ f32[2]
+ [0.916289210319519, -3.080907390540233e-6]
+>
+
+iex> y_true = Nx.tensor([[0, 1], [0, 0]], type: {:u, 8})
+iex> y_pred = Nx.tensor([[0.6, 0.4], [0.4, 0.6]])
+iex> Axon.Losses.kl_divergence(y_true, y_pred, reduction: :mean)
+#Nx.Tensor<
f32
0.45814305543899536
->
+>
-iex> y_true = Nx.tensor([[0, 1], [0, 0]], type: {:u, 8})
-iex> y_pred = Nx.tensor([[0.6, 0.4], [0.4, 0.6]])
-iex> Axon.Losses.kl_divergence(y_true, y_pred, reduction: :sum)
-#Nx.Tensor<
+iex> y_true = Nx.tensor([[0, 1], [0, 0]], type: {:u, 8})
+iex> y_pred = Nx.tensor([[0.6, 0.4], [0.4, 0.6]])
+iex> Axon.Losses.kl_divergence(y_true, y_pred, reduction: :sum)
+#Nx.Tensor<
f32
0.9162861108779907
->
+>
iex> y_true = Nx.tensor([[0.0, 1.0], [0.0, 0.0]])
-iex> y_pred = Nx.tensor([[1.0, 1.0], [0.0, 0.0]])
-iex> Axon.Losses.log_cosh(y_true, y_pred)
-#Nx.Tensor<
- f32[2]
- [0.2168903946876526, 0.0]
->
-
-iex> y_true = Nx.tensor([[0.0, 1.0], [0.0, 0.0]])
-iex> y_pred = Nx.tensor([[1.0, 1.0], [0.0, 0.0]])
-iex> Axon.Losses.log_cosh(y_true, y_pred, reduction: :mean)
-#Nx.Tensor<
+iex> y_true = Nx.tensor([[0.0, 1.0], [0.0, 0.0]])
+iex> y_pred = Nx.tensor([[1.0, 1.0], [0.0, 0.0]])
+iex> Axon.Losses.log_cosh(y_true, y_pred)
+#Nx.Tensor<
+ f32[2]
+ [0.2168903946876526, 0.0]
+>
+
+iex> y_true = Nx.tensor([[0.0, 1.0], [0.0, 0.0]])
+iex> y_pred = Nx.tensor([[1.0, 1.0], [0.0, 0.0]])
+iex> Axon.Losses.log_cosh(y_true, y_pred, reduction: :mean)
+#Nx.Tensor<
f32
0.1084451973438263
->
+>
-iex> y_true = Nx.tensor([[0.0, 1.0], [0.0, 0.0]])
-iex> y_pred = Nx.tensor([[1.0, 1.0], [0.0, 0.0]])
-iex> Axon.Losses.log_cosh(y_true, y_pred, reduction: :sum)
-#Nx.Tensor<
+iex> y_true = Nx.tensor([[0.0, 1.0], [0.0, 0.0]])
+iex> y_pred = Nx.tensor([[1.0, 1.0], [0.0, 0.0]])
+iex> Axon.Losses.log_cosh(y_true, y_pred, reduction: :sum)
+#Nx.Tensor<
f32
0.2168903946876526
->
+>
iex> y_true = Nx.tensor([1.0, 1.0, 1.0], type: {:f, 32})
-iex> y_pred1 = Nx.tensor([0.6934, -0.7239, 1.1954], type: {:f, 32})
-iex> y_pred2 = Nx.tensor([-0.4691, 0.2670, -1.7452], type: {:f, 32})
-iex> Axon.Losses.margin_ranking(y_true, {y_pred1, y_pred2})
-#Nx.Tensor<
- f32[3]
- [0.0, 0.9909000396728516, 0.0]
->
-
-iex> y_true = Nx.tensor([1.0, 1.0, 1.0], type: {:f, 32})
-iex> y_pred1 = Nx.tensor([0.6934, -0.7239, 1.1954], type: {:f, 32})
-iex> y_pred2 = Nx.tensor([-0.4691, 0.2670, -1.7452], type: {:f, 32})
-iex> Axon.Losses.margin_ranking(y_true, {y_pred1, y_pred2}, reduction: :mean)
-#Nx.Tensor<
+iex> y_true = Nx.tensor([1.0, 1.0, 1.0], type: {:f, 32})
+iex> y_pred1 = Nx.tensor([0.6934, -0.7239, 1.1954], type: {:f, 32})
+iex> y_pred2 = Nx.tensor([-0.4691, 0.2670, -1.7452], type: {:f, 32})
+iex> Axon.Losses.margin_ranking(y_true, {y_pred1, y_pred2})
+#Nx.Tensor<
+ f32[3]
+ [0.0, 0.9909000396728516, 0.0]
+>
+
+iex> y_true = Nx.tensor([1.0, 1.0, 1.0], type: {:f, 32})
+iex> y_pred1 = Nx.tensor([0.6934, -0.7239, 1.1954], type: {:f, 32})
+iex> y_pred2 = Nx.tensor([-0.4691, 0.2670, -1.7452], type: {:f, 32})
+iex> Axon.Losses.margin_ranking(y_true, {y_pred1, y_pred2}, reduction: :mean)
+#Nx.Tensor<
f32
0.3303000032901764
->
+>
-iex> y_true = Nx.tensor([1.0, 1.0, 1.0], type: {:f, 32})
-iex> y_pred1 = Nx.tensor([0.6934, -0.7239, 1.1954], type: {:f, 32})
-iex> y_pred2 = Nx.tensor([-0.4691, 0.2670, -1.7452], type: {:f, 32})
-iex> Axon.Losses.margin_ranking(y_true, {y_pred1, y_pred2}, reduction: :sum)
-#Nx.Tensor<
+iex> y_true = Nx.tensor([1.0, 1.0, 1.0], type: {:f, 32})
+iex> y_pred1 = Nx.tensor([0.6934, -0.7239, 1.1954], type: {:f, 32})
+iex> y_pred2 = Nx.tensor([-0.4691, 0.2670, -1.7452], type: {:f, 32})
+iex> Axon.Losses.margin_ranking(y_true, {y_pred1, y_pred2}, reduction: :sum)
+#Nx.Tensor<
f32
0.9909000396728516
->
+>
iex> y_true = Nx.tensor([[0.0, 1.0], [0.0, 0.0]], type: {:f, 32})
-iex> y_pred = Nx.tensor([[1.0, 1.0], [1.0, 0.0]], type: {:f, 32})
-iex> Axon.Losses.mean_absolute_error(y_true, y_pred)
-#Nx.Tensor<
- f32[2]
- [0.5, 0.5]
->
-
-iex> y_true = Nx.tensor([[0.0, 1.0], [0.0, 0.0]], type: {:f, 32})
-iex> y_pred = Nx.tensor([[1.0, 1.0], [1.0, 0.0]], type: {:f, 32})
-iex> Axon.Losses.mean_absolute_error(y_true, y_pred, reduction: :mean)
-#Nx.Tensor<
+iex> y_true = Nx.tensor([[0.0, 1.0], [0.0, 0.0]], type: {:f, 32})
+iex> y_pred = Nx.tensor([[1.0, 1.0], [1.0, 0.0]], type: {:f, 32})
+iex> Axon.Losses.mean_absolute_error(y_true, y_pred)
+#Nx.Tensor<
+ f32[2]
+ [0.5, 0.5]
+>
+
+iex> y_true = Nx.tensor([[0.0, 1.0], [0.0, 0.0]], type: {:f, 32})
+iex> y_pred = Nx.tensor([[1.0, 1.0], [1.0, 0.0]], type: {:f, 32})
+iex> Axon.Losses.mean_absolute_error(y_true, y_pred, reduction: :mean)
+#Nx.Tensor<
f32
0.5
->
+>
-iex> y_true = Nx.tensor([[0.0, 1.0], [0.0, 0.0]], type: {:f, 32})
-iex> y_pred = Nx.tensor([[1.0, 1.0], [1.0, 0.0]], type: {:f, 32})
-iex> Axon.Losses.mean_absolute_error(y_true, y_pred, reduction: :sum)
-#Nx.Tensor<
+iex> y_true = Nx.tensor([[0.0, 1.0], [0.0, 0.0]], type: {:f, 32})
+iex> y_pred = Nx.tensor([[1.0, 1.0], [1.0, 0.0]], type: {:f, 32})
+iex> Axon.Losses.mean_absolute_error(y_true, y_pred, reduction: :sum)
+#Nx.Tensor<
f32
1.0
->
+>
iex> y_true = Nx.tensor([[0.0, 1.0], [0.0, 0.0]], type: {:f, 32})
-iex> y_pred = Nx.tensor([[1.0, 1.0], [1.0, 0.0]], type: {:f, 32})
-iex> Axon.Losses.mean_squared_error(y_true, y_pred)
-#Nx.Tensor<
- f32[2]
- [0.5, 0.5]
->
-
-iex> y_true = Nx.tensor([[0.0, 1.0], [0.0, 0.0]], type: {:f, 32})
-iex> y_pred = Nx.tensor([[1.0, 1.0], [1.0, 0.0]], type: {:f, 32})
-iex> Axon.Losses.mean_squared_error(y_true, y_pred, reduction: :mean)
-#Nx.Tensor<
+iex> y_true = Nx.tensor([[0.0, 1.0], [0.0, 0.0]], type: {:f, 32})
+iex> y_pred = Nx.tensor([[1.0, 1.0], [1.0, 0.0]], type: {:f, 32})
+iex> Axon.Losses.mean_squared_error(y_true, y_pred)
+#Nx.Tensor<
+ f32[2]
+ [0.5, 0.5]
+>
+
+iex> y_true = Nx.tensor([[0.0, 1.0], [0.0, 0.0]], type: {:f, 32})
+iex> y_pred = Nx.tensor([[1.0, 1.0], [1.0, 0.0]], type: {:f, 32})
+iex> Axon.Losses.mean_squared_error(y_true, y_pred, reduction: :mean)
+#Nx.Tensor<
f32
0.5
->
+>
-iex> y_true = Nx.tensor([[0.0, 1.0], [0.0, 0.0]], type: {:f, 32})
-iex> y_pred = Nx.tensor([[1.0, 1.0], [1.0, 0.0]], type: {:f, 32})
-iex> Axon.Losses.mean_squared_error(y_true, y_pred, reduction: :sum)
-#Nx.Tensor<
+iex> y_true = Nx.tensor([[0.0, 1.0], [0.0, 0.0]], type: {:f, 32})
+iex> y_pred = Nx.tensor([[1.0, 1.0], [1.0, 0.0]], type: {:f, 32})
+iex> Axon.Losses.mean_squared_error(y_true, y_pred, reduction: :sum)
+#Nx.Tensor<
f32
1.0
->
+>
iex> y_true = Nx.tensor([[0.0, 1.0], [0.0, 0.0]], type: {:f, 32})
-iex> y_pred = Nx.tensor([[1.0, 1.0], [0.0, 0.0]], type: {:f, 32})
-iex> Axon.Losses.poisson(y_true, y_pred)
-#Nx.Tensor<
- f32[2]
- [0.9999999403953552, 0.0]
->
-
-iex> y_true = Nx.tensor([[0.0, 1.0], [0.0, 0.0]], type: {:f, 32})
-iex> y_pred = Nx.tensor([[1.0, 1.0], [0.0, 0.0]], type: {:f, 32})
-iex> Axon.Losses.poisson(y_true, y_pred, reduction: :mean)
-#Nx.Tensor<
+iex> y_true = Nx.tensor([[0.0, 1.0], [0.0, 0.0]], type: {:f, 32})
+iex> y_pred = Nx.tensor([[1.0, 1.0], [0.0, 0.0]], type: {:f, 32})
+iex> Axon.Losses.poisson(y_true, y_pred)
+#Nx.Tensor<
+ f32[2]
+ [0.9999999403953552, 0.0]
+>
+
+iex> y_true = Nx.tensor([[0.0, 1.0], [0.0, 0.0]], type: {:f, 32})
+iex> y_pred = Nx.tensor([[1.0, 1.0], [0.0, 0.0]], type: {:f, 32})
+iex> Axon.Losses.poisson(y_true, y_pred, reduction: :mean)
+#Nx.Tensor<
f32
0.4999999701976776
->
+>
-iex> y_true = Nx.tensor([[0.0, 1.0], [0.0, 0.0]], type: {:f, 32})
-iex> y_pred = Nx.tensor([[1.0, 1.0], [0.0, 0.0]], type: {:f, 32})
-iex> Axon.Losses.poisson(y_true, y_pred, reduction: :sum)
-#Nx.Tensor<
+iex> y_true = Nx.tensor([[0.0, 1.0], [0.0, 0.0]], type: {:f, 32})
+iex> y_pred = Nx.tensor([[1.0, 1.0], [0.0, 0.0]], type: {:f, 32})
+iex> Axon.Losses.poisson(y_true, y_pred, reduction: :sum)
+#Nx.Tensor<
f32
0.9999999403953552
->
+>
iex> y_true = Nx.tensor([[-1.0, 1.0, 1.0]], type: {:f, 32})
-iex> y_pred = Nx.tensor([[0.2953, -0.1709, 0.9486]], type: {:f, 32})
-iex> Axon.Losses.soft_margin(y_true, y_pred)
-#Nx.Tensor<
- f32[3]
- [0.851658046245575, 0.7822436094284058, 0.3273470401763916]
->
-
-iex> y_true = Nx.tensor([[-1.0, 1.0, 1.0]], type: {:f, 32})
-iex> y_pred = Nx.tensor([[0.2953, -0.1709, 0.9486]], type: {:f, 32})
-iex> Axon.Losses.soft_margin(y_true, y_pred, reduction: :mean)
-#Nx.Tensor<
+iex> y_true = Nx.tensor([[-1.0, 1.0, 1.0]], type: {:f, 32})
+iex> y_pred = Nx.tensor([[0.2953, -0.1709, 0.9486]], type: {:f, 32})
+iex> Axon.Losses.soft_margin(y_true, y_pred)
+#Nx.Tensor<
+ f32[3]
+ [0.851658046245575, 0.7822436094284058, 0.3273470401763916]
+>
+
+iex> y_true = Nx.tensor([[-1.0, 1.0, 1.0]], type: {:f, 32})
+iex> y_pred = Nx.tensor([[0.2953, -0.1709, 0.9486]], type: {:f, 32})
+iex> Axon.Losses.soft_margin(y_true, y_pred, reduction: :mean)
+#Nx.Tensor<
f32
0.6537495255470276
->
+>
-iex> y_true = Nx.tensor([[-1.0, 1.0, 1.0]], type: {:f, 32})
-iex> y_pred = Nx.tensor([[0.2953, -0.1709, 0.9486]], type: {:f, 32})
-iex> Axon.Losses.soft_margin(y_true, y_pred, reduction: :sum)
-#Nx.Tensor<
+iex> y_true = Nx.tensor([[-1.0, 1.0, 1.0]], type: {:f, 32})
+iex> y_pred = Nx.tensor([[0.2953, -0.1709, 0.9486]], type: {:f, 32})
+iex> Axon.Losses.soft_margin(y_true, y_pred, reduction: :sum)
+#Nx.Tensor<
f32
1.9612486362457275
->
+>
iex> Axon.Metrics.accuracy(Nx.tensor([[1], [0], [0]]), Nx.tensor([[1], [1], [1]]))
-#Nx.Tensor<
+iex> Axon.Metrics.accuracy(Nx.tensor([[1], [0], [0]]), Nx.tensor([[1], [1], [1]]))
+#Nx.Tensor<
f32
0.3333333432674408
->
+>
-iex> Axon.Metrics.accuracy(Nx.tensor([[0, 1], [1, 0], [1, 0]]), Nx.tensor([[0, 1], [1, 0], [0, 1]]))
-#Nx.Tensor<
+iex> Axon.Metrics.accuracy(Nx.tensor([[0, 1], [1, 0], [1, 0]]), Nx.tensor([[0, 1], [1, 0], [0, 1]]))
+#Nx.Tensor<
f32
0.6666666865348816
->
+>
-iex> Axon.Metrics.accuracy(Nx.tensor([[0, 1, 0], [1, 0, 0]]), Nx.tensor([[0, 1, 0], [0, 1, 0]]))
-#Nx.Tensor<
+iex> Axon.Metrics.accuracy(Nx.tensor([[0, 1, 0], [1, 0, 0]]), Nx.tensor([[0, 1, 0], [0, 1, 0]]))
+#Nx.Tensor<
f32
0.5
->
+>
iex> y_true = Nx.tensor([1, 0, 1, 1, 0, 1, 0])
-iex> y_pred = Nx.tensor([0.8, 0.6, 0.4, 0.2, 0.8, 0.2, 0.2])
-iex> Axon.Metrics.false_negatives(y_true, y_pred)
-#Nx.Tensor<
+iex> y_true = Nx.tensor([1, 0, 1, 1, 0, 1, 0])
+iex> y_pred = Nx.tensor([0.8, 0.6, 0.4, 0.2, 0.8, 0.2, 0.2])
+iex> Axon.Metrics.false_negatives(y_true, y_pred)
+#Nx.Tensor<
u64
3
->
+>
iex> y_true = Nx.tensor([1, 0, 1, 1, 0, 1, 0])
-iex> y_pred = Nx.tensor([0.8, 0.6, 0.4, 0.2, 0.8, 0.2, 0.2])
-iex> Axon.Metrics.false_positives(y_true, y_pred)
-#Nx.Tensor<
+iex> y_true = Nx.tensor([1, 0, 1, 1, 0, 1, 0])
+iex> y_pred = Nx.tensor([0.8, 0.6, 0.4, 0.2, 0.8, 0.2, 0.2])
+iex> Axon.Metrics.false_positives(y_true, y_pred)
+#Nx.Tensor<
u64
2
->
+>
iex> y_true = Nx.tensor([[0.0, 1.0], [0.0, 0.0]], type: {:f, 32})
-iex> y_pred = Nx.tensor([[1.0, 1.0], [1.0, 0.0]], type: {:f, 32})
-iex> Axon.Metrics.mean_absolute_error(y_true, y_pred)
-#Nx.Tensor<
+iex> y_true = Nx.tensor([[0.0, 1.0], [0.0, 0.0]], type: {:f, 32})
+iex> y_pred = Nx.tensor([[1.0, 1.0], [1.0, 0.0]], type: {:f, 32})
+iex> Axon.Metrics.mean_absolute_error(y_true, y_pred)
+#Nx.Tensor<
f32
0.5
->
+>
iex> Axon.Metrics.precision(Nx.tensor([0, 1, 1, 1]), Nx.tensor([1, 0, 1, 1]))
-#Nx.Tensor<
+iex> Axon.Metrics.precision(Nx.tensor([0, 1, 1, 1]), Nx.tensor([1, 0, 1, 1]))
+#Nx.Tensor<
f32
0.6666666865348816
->
+>
iex> Axon.Metrics.recall(Nx.tensor([0, 1, 1, 1]), Nx.tensor([1, 0, 1, 1]))
-#Nx.Tensor<
+iex> Axon.Metrics.recall(Nx.tensor([0, 1, 1, 1]), Nx.tensor([1, 0, 1, 1]))
+#Nx.Tensor<
f32
0.6666666865348816
->
+>
iex> cur_avg = 0.5
iex> iteration = 1
-iex> y_true = Nx.tensor([[0, 1], [1, 0], [1, 0]])
-iex> y_pred = Nx.tensor([[0, 1], [1, 0], [1, 0]])
-iex> avg_acc = Axon.Metrics.running_average(&Axon.Metrics.accuracy/2)
-iex> avg_acc.(cur_avg, [y_true, y_pred], iteration)
-#Nx.Tensor<
+iex> y_true = Nx.tensor([[0, 1], [1, 0], [1, 0]])
+iex> y_pred = Nx.tensor([[0, 1], [1, 0], [1, 0]])
+iex> avg_acc = Axon.Metrics.running_average(&Axon.Metrics.accuracy/2)
+iex> avg_acc.(cur_avg, [y_true, y_pred], iteration)
+#Nx.Tensor<
f32
0.75
->
+>
iex> cur_sum = 12
iex> iteration = 2
-iex> y_true = Nx.tensor([0, 1, 0, 1])
-iex> y_pred = Nx.tensor([1, 1, 0, 1])
-iex> fps = Axon.Metrics.running_sum(&Axon.Metrics.false_positives/2)
-iex> fps.(cur_sum, [y_true, y_pred], iteration)
-#Nx.Tensor<
+iex> y_true = Nx.tensor([0, 1, 0, 1])
+iex> y_pred = Nx.tensor([1, 1, 0, 1])
+iex> fps = Axon.Metrics.running_sum(&Axon.Metrics.false_positives/2)
+iex> fps.(cur_sum, [y_true, y_pred], iteration)
+#Nx.Tensor<
s64
13
->
+>
iex> Axon.Metrics.sensitivity(Nx.tensor([0, 1, 1, 1]), Nx.tensor([1, 0, 1, 1]))
-#Nx.Tensor<
+iex> Axon.Metrics.sensitivity(Nx.tensor([0, 1, 1, 1]), Nx.tensor([1, 0, 1, 1]))
+#Nx.Tensor<
f32
0.6666666865348816
->
+>
iex> Axon.Metrics.specificity(Nx.tensor([0, 1, 1, 1]), Nx.tensor([1, 0, 1, 1]))
-#Nx.Tensor<
+iex> Axon.Metrics.specificity(Nx.tensor([0, 1, 1, 1]), Nx.tensor([1, 0, 1, 1]))
+#Nx.Tensor<
f32
0.0
->
+>
iex> Axon.Metrics.top_k_categorical_accuracy(Nx.tensor([0, 1, 0, 0, 0]), Nx.tensor([0.1, 0.4, 0.3, 0.7, 0.1]), k: 2)
-#Nx.Tensor<
+iex> Axon.Metrics.top_k_categorical_accuracy(Nx.tensor([0, 1, 0, 0, 0]), Nx.tensor([0.1, 0.4, 0.3, 0.7, 0.1]), k: 2)
+#Nx.Tensor<
f32
1.0
->
+>
-iex> Axon.Metrics.top_k_categorical_accuracy(Nx.tensor([[0, 1, 0], [1, 0, 0]]), Nx.tensor([[0.1, 0.4, 0.7], [0.1, 0.4, 0.7]]), k: 2)
-#Nx.Tensor<
+iex> Axon.Metrics.top_k_categorical_accuracy(Nx.tensor([[0, 1, 0], [1, 0, 0]]), Nx.tensor([[0.1, 0.4, 0.7], [0.1, 0.4, 0.7]]), k: 2)
+#Nx.Tensor<
f32
0.5
->
+>
-iex> Axon.Metrics.top_k_categorical_accuracy(Nx.tensor([[0], [2]]), Nx.tensor([[0.1, 0.4, 0.7], [0.1, 0.4, 0.7]]), k: 2, sparse: true)
-#Nx.Tensor<
+iex> Axon.Metrics.top_k_categorical_accuracy(Nx.tensor([[0], [2]]), Nx.tensor([[0.1, 0.4, 0.7], [0.1, 0.4, 0.7]]), k: 2, sparse: true)
+#Nx.Tensor<
f32
0.5
->
+>
iex> y_true = Nx.tensor([1, 0, 1, 1, 0, 1, 0])
-iex> y_pred = Nx.tensor([0.8, 0.6, 0.4, 0.2, 0.8, 0.2, 0.2])
-iex> Axon.Metrics.true_negatives(y_true, y_pred)
-#Nx.Tensor<
+iex> y_true = Nx.tensor([1, 0, 1, 1, 0, 1, 0])
+iex> y_pred = Nx.tensor([0.8, 0.6, 0.4, 0.2, 0.8, 0.2, 0.2])
+iex> Axon.Metrics.true_negatives(y_true, y_pred)
+#Nx.Tensor<
u64
1
->
+>
iex> y_true = Nx.tensor([1, 0, 1, 1, 0, 1, 0])
-iex> y_pred = Nx.tensor([0.8, 0.6, 0.4, 0.2, 0.8, 0.2, 0.2])
-iex> Axon.Metrics.true_positives(y_true, y_pred)
-#Nx.Tensor<
+iex> y_true = Nx.tensor([1, 0, 1, 1, 0, 1, 0])
+iex> y_pred = Nx.tensor([0.8, 0.6, 0.4, 0.2, 0.8, 0.2, 0.2])
+iex> Axon.Metrics.true_positives(y_true, y_pred)
+#Nx.Tensor<
u64
1
->
+>
output
policy dictates what type the model should output.Here's an example of creating a mixed precision policy and applying it to a model:
model =
- Axon.input("input", shape: {nil, 784})
- |> Axon.dense(128, activation: :relu)
- |> Axon.batch_norm()
- |> Axon.dropout(rate: 0.5)
- |> Axon.dense(64, activation: :relu)
- |> Axon.batch_norm()
- |> Axon.dropout(rate: 0.5)
- |> Axon.dense(10, activation: :softmax)
-
-policy = Axon.MixedPrecision.create_policy(
- params: {:f, 32},
- compute: {:f, 16},
- output: {:f, 32}
-)
+ Axon.input("input", shape: {nil, 784})
+ |> Axon.dense(128, activation: :relu)
+ |> Axon.batch_norm()
+ |> Axon.dropout(rate: 0.5)
+ |> Axon.dense(64, activation: :relu)
+ |> Axon.batch_norm()
+ |> Axon.dropout(rate: 0.5)
+ |> Axon.dense(10, activation: :softmax)
+
+policy = Axon.MixedPrecision.create_policy(
+ params: {:f, 32},
+ compute: {:f, 16},
+ output: {:f, 32}
+)
mp_model =
model
- |> Axon.MixedPrecision.apply_policy(policy, except: [:batch_norm])
The example above applies the mixed precision policy to every layer in + |> Axon.MixedPrecision.apply_policy(policy, except: [:batch_norm])
The example above applies the mixed precision policy to every layer in
the model except Batch Normalization layers. The policy will cast parameters
and inputs to {:f, 16}
for intermediate computations in the model's forward
pass before casting the output back to {:f, 32}
.
iex> Axon.MixedPrecision.create_policy(params: {:f, 16}, output: {:f, 16})
-%Policy{params: {:f, 16}, compute: {:f, 32}, output: {:f, 16}}
+iex> Axon.MixedPrecision.create_policy(params: {:f, 16}, output: {:f, 16})
+%Policy{params: {:f, 16}, compute: {:f, 32}, output: {:f, 16}}
-iex> Axon.MixedPrecision.create_policy(compute: {:bf, 16})
-%Policy{params: {:f, 32}, compute: {:bf, 16}, output: {:f, 32}}
+iex> Axon.MixedPrecision.create_policy(compute: {:bf, 16})
+%Policy{params: {:f, 32}, compute: {:bf, 16}, output: {:f, 32}}
diff --git a/Axon.Optimizers.html b/Axon.Optimizers.html
index 2ffa7bd1..7247d7c3 100644
--- a/Axon.Optimizers.html
+++ b/Axon.Optimizers.html
@@ -114,7 +114,7 @@ Implementations of common gradient-based optimization algorithms.
All of the methods in this module are written in terms of
the update methods defined in Axon.Updates
. Axon treats
-optimizers as the tuple:
{init_fn, update_fn}
where init_fn
returns an initial optimizer state and update_fn
+optimizers as the tuple:
{init_fn, update_fn}
where init_fn
returns an initial optimizer state and update_fn
scales input gradients. init_fn
accepts a model's parameters
and attaches state to each parameter. update_fn
accepts
gradients, optimizer state, and current model parameters and
@@ -126,31 +126,31 @@
Consider the following usage of the Adam optimizer in a basic
update function (assuming objective
and the dataset
are
-defined elsewhere):
defmodule Learning do
+defined elsewhere):defmodule Learning do
import Nx.Defn
- defn init(params, init_fn) do
- init_fn.(params)
- end
+ defn init(params, init_fn) do
+ init_fn.(params)
+ end
- defn update(params, optimizer_state, inputs, targets, update_fn) do
- {loss, gradient} = value_and_grad(params, &objective(&1, inputs, targets))
- {scaled_updates, new_optimizer_state} = update_fn.(gradient, optimizer_state, params)
- {Axon.Updates.apply_updates(params, scaled_updates), new_optimizer_state, loss}
- end
-end
+ defn update(params, optimizer_state, inputs, targets, update_fn) do
+ {loss, gradient} = value_and_grad(params, &objective(&1, inputs, targets))
+ {scaled_updates, new_optimizer_state} = update_fn.(gradient, optimizer_state, params)
+ {Axon.Updates.apply_updates(params, scaled_updates), new_optimizer_state, loss}
+ end
+end
-{model_params, _key} = Nx.Random.uniform(key, shape: {784, 10})
-{init_fn, update_fn} = Axon.Optimizers.adam(0.005)
+{model_params, _key} = Nx.Random.uniform(key, shape: {784, 10})
+{init_fn, update_fn} = Axon.Optimizers.adam(0.005)
optimizer_state =
- Learning.init(params, init_fn)
+ Learning.init(params, init_fn)
-{new_params, new_optimizer_state, loss} =
- Learning.update(params, optimizer_state, inputs, targets, update_fn)
For a simpler approach, you can also use optimizers with the training API:
model
- |> Axon.Loop.trainer(:categorical_cross_entropy, Axon.Optimizers.adam(0.005))
- |> Axon.Loop.run(data, epochs: 10, compiler: EXLA)
+{new_params, new_optimizer_state, loss} =
+ Learning.update(params, optimizer_state, inputs, targets, update_fn)
For a simpler approach, you can also use optimizers with the training API:
model
+ |> Axon.Loop.trainer(:categorical_cross_entropy, Axon.Optimizers.adam(0.005))
+ |> Axon.Loop.run(data, epochs: 10, compiler: EXLA)
{init_fn, update_fn}
Which represent a state initialization and state update +or Adam. Each update returns a tuple:
{init_fn, update_fn}
Which represent a state initialization and state update
function respectively. While each method in the Updates
API is a regular Elixir function, the two methods they
return are implemented as defn
, so they can be accelerated
using any Nx backend or compiler.
Update methods are just combinators that can be arbitrarily composed to create complex optimizers. For example, the Adam -optimizer in Axon.Optimizers is implemented as:
def adam(learning_rate, opts \\ []) do
- Updates.scale_by_adam(opts)
- |> Updates.scale(-learning_rate)
-end
Updates are maps of updates, often associated with parameters of +optimizer in Axon.Optimizers is implemented as:
def adam(learning_rate, opts \\ []) do
+ Updates.scale_by_adam(opts)
+ |> Updates.scale(-learning_rate)
+end
Updates are maps of updates, often associated with parameters of
the same names. Using Axon.Updates.apply_updates/3
will merge updates
and parameters by adding associated parameters and updates, and
ensuring any given model state is preserved.
You can create your own combinators using the stateless/2
and
stateful/3
primitives. Every update method in this module is
-implemented in terms of one of these two primitives.
stateless/2
represents a stateless update:
def scale(combinator \\ Axon.Updates.identity(), step_size) do
- stateless(combinator, &apply_scale(&1, &2, step_size))
-end
+implemented in terms of one of these two primitives.stateless/2
represents a stateless update:
def scale(combinator \\ Axon.Updates.identity(), step_size) do
+ stateless(combinator, &apply_scale(&1, &2, step_size))
+end
-defnp apply_scale(x, _params, step) do
- deep_new(updates, fn x -> Nx.multiply(x, step) end)
-end
Notice how the function given to stateless/2
is defined within defn
.
+
defnp apply_scale(x, _params, step) do
+ deep_new(updates, fn x -> Nx.multiply(x, step) end)
+end
Notice how the function given to stateless/2
is defined within defn
.
This is what allows the anonymous functions returned by Axon.Updates
-to be used inside defn
.
stateful/3
represents a stateful update and follows the same pattern:
def my_stateful_update(updates) do
- Axon.Updates.stateful(updates, &init_my_update/1, &apply_my_update/2)
-end
-
-defnp init_my_update(params) do
- state = zeros_like(params, type: :f32)
- %{state: state}
-end
-
-defnp apply_my_update(updates, state) do
- new_state = deep_new(state, fn v -> Nx.add(v, 0.01) end)
- updates = deep_merge(updates, state, fn g, z -> Nx.multiply(g, z) end)
- {updates, %{state: new_state}}
-end
State associated with individual parameters should have keys that match the
+to be used inside defn
.
stateful/3
represents a stateful update and follows the same pattern:
def my_stateful_update(updates) do
+ Axon.Updates.stateful(updates, &init_my_update/1, &apply_my_update/2)
+end
+
+defnp init_my_update(params) do
+ state = zeros_like(params, type: :f32)
+ %{state: state}
+end
+
+defnp apply_my_update(updates, state) do
+ new_state = deep_new(state, fn v -> Nx.add(v, 0.01) end)
+ updates = deep_merge(updates, state, fn g, z -> Nx.multiply(g, z) end)
+ {updates, %{state: new_state}}
+end
State associated with individual parameters should have keys that match the
keys of the parameter. For example, if you have parameters %{kernel: kernel}
with associated states mu
and nu
representing the first and second moments,
-your state should look something like:
%{
- mu: %{kernel: kernel_mu}
- nu: %{kernel: kernel_nu}
-}
+your state should look something like:%{
+ mu: %{kernel: kernel_mu}
+ nu: %{kernel: kernel_nu}
+}
@@ -857,8 +857,8 @@ import Axon.Updates
-Axon.Updates.compose(Axon.Updates.centralize(), Axon.Optimizers.rmsprop())
This is equivalent to:
Axon.Updates.centralize()
-|> Axon.Updates.scale_by_rms()
+Axon.Updates.compose(Axon.Updates.centralize(), Axon.Optimizers.rmsprop())This is equivalent to:
Axon.Updates.centralize()
+|> Axon.Updates.scale_by_rms()
* [Adaptive Methods for Nonconvex Optimization](https://proceedings.neurips.cc/paper/2018/file/90365351ccc7437a1309dc64e4db32a3-Paper.pdf)
+* [Adaptive Methods for Nonconvex Optimization](https://proceedings.neurips.cc/paper/2018/file/90365351ccc7437a1309dc64e4db32a3-Paper.pdf)
All Axon models start with an input layer, optionally specifying -the expected shape of the input data:
input = Axon.input("input", shape: {nil, 784})
Notice you can specify some dimensions as nil
, indicating
+the expected shape of the input data:
input = Axon.input("input", shape: {nil, 784})
Notice you can specify some dimensions as nil
, indicating
that the dimension size will be filled in at model runtime.
You can then compose inputs with other layers:
model =
input
- |> Axon.dense(128, activation: :relu)
- |> Axon.batch_norm()
- |> Axon.dropout(rate: 0.8)
- |> Axon.dense(64)
- |> Axon.tanh()
- |> Axon.dense(10)
- |> Axon.activation(:softmax)
You can inspect the model for a nice summary:
IO.inspect(model)
-
-#Axon<
- inputs: %{"input" => {nil, 784}}
+ |> Axon.dense(128, activation: :relu)
+ |> Axon.batch_norm()
+ |> Axon.dropout(rate: 0.8)
+ |> Axon.dense(64)
+ |> Axon.tanh()
+ |> Axon.dense(10)
+ |> Axon.activation(:softmax)
You can inspect the model for a nice summary:
IO.inspect(model)
+
+#Axon<
+ inputs: %{"input" => {nil, 784}}
outputs: "softmax_0"
nodes: 9
->
Or use the Axon.Display
module to see more in-depth summaries:
Axon.Display.as_table(model, Nx.template({1, 784}, :f32)) |> IO.puts
+>
Or use the Axon.Display
module to see more in-depth summaries:
Axon.Display.as_table(model, Nx.template({1, 784}, :f32)) |> IO.puts
+----------------------------------------------------------------------------------------------------------------+
| Model |
+=======================================+=============+==============+===================+=======================+
| Layer | Input Shape | Output Shape | Options | Parameters |
+=======================================+=============+==============+===================+=======================+
-| input ( input ) | [] | {1, 784} | shape: {nil, 784} | |
+| input ( input ) | [] | {1, 784} | shape: {nil, 784} | |
| | | | optional: false | |
+---------------------------------------+-------------+--------------+-------------------+-----------------------+
-| dense_0 ( dense["input"] ) | [{1, 784}] | {1, 128} | | kernel: f32[784][128] |
-| | | | | bias: f32[128] |
+| dense_0 ( dense["input"] ) | [{1, 784}] | {1, 128} | | kernel: f32[784][128] |
+| | | | | bias: f32[128] |
+---------------------------------------+-------------+--------------+-------------------+-----------------------+
-| relu_0 ( relu["dense_0"] ) | [{1, 128}] | {1, 128} | | |
+| relu_0 ( relu["dense_0"] ) | [{1, 128}] | {1, 128} | | |
+---------------------------------------+-------------+--------------+-------------------+-----------------------+
-| batch_norm_0 ( batch_norm["relu_0"] ) | [{1, 128}] | {1, 128} | epsilon: 1.0e-5 | gamma: f32[128] |
-| | | | channel_index: 1 | beta: f32[128] |
-| | | | momentum: 0.1 | mean: f32[128] |
-| | | | | var: f32[128] |
+| batch_norm_0 ( batch_norm["relu_0"] ) | [{1, 128}] | {1, 128} | epsilon: 1.0e-5 | gamma: f32[128] |
+| | | | channel_index: 1 | beta: f32[128] |
+| | | | momentum: 0.1 | mean: f32[128] |
+| | | | | var: f32[128] |
+---------------------------------------+-------------+--------------+-------------------+-----------------------+
-| dropout_0 ( dropout["batch_norm_0"] ) | [{1, 128}] | {1, 128} | rate: 0.8 | |
+| dropout_0 ( dropout["batch_norm_0"] ) | [{1, 128}] | {1, 128} | rate: 0.8 | |
+---------------------------------------+-------------+--------------+-------------------+-----------------------+
-| dense_1 ( dense["dropout_0"] ) | [{1, 128}] | {1, 64} | | kernel: f32[128][64] |
-| | | | | bias: f32[64] |
+| dense_1 ( dense["dropout_0"] ) | [{1, 128}] | {1, 64} | | kernel: f32[128][64] |
+| | | | | bias: f32[64] |
+---------------------------------------+-------------+--------------+-------------------+-----------------------+
-| tanh_0 ( tanh["dense_1"] ) | [{1, 64}] | {1, 64} | | |
+| tanh_0 ( tanh["dense_1"] ) | [{1, 64}] | {1, 64} | | |
+---------------------------------------+-------------+--------------+-------------------+-----------------------+
-| dense_2 ( dense["tanh_0"] ) | [{1, 64}] | {1, 10} | | kernel: f32[64][10] |
-| | | | | bias: f32[10] |
+| dense_2 ( dense["tanh_0"] ) | [{1, 64}] | {1, 10} | | kernel: f32[64][10] |
+| | | | | bias: f32[10] |
+---------------------------------------+-------------+--------------+-------------------+-----------------------+
-| softmax_0 ( softmax["dense_2"] ) | [{1, 10}] | {1, 10} | | |
+| softmax_0 ( softmax["dense_2"] ) | [{1, 10}] | {1, 10} | | |
+---------------------------------------+-------------+--------------+-------------------+-----------------------+
multiple-inputs
@@ -179,28 +179,28 @@Creating a model with multiple inputs is as easy as declaring an additional input in your Axon graph. Every input layer present in the final Axon graph will be required to be passed as input at the -time of model execution.
inp1 = Axon.input("input_0", shape: {nil, 1})
-inp2 = Axon.input("input_1", shape: {nil, 1})
+time of model execution.inp1 = Axon.input("input_0", shape: {nil, 1})
+inp2 = Axon.input("input_1", shape: {nil, 1})
# Both inputs will be used
-model1 = Axon.add(inp1, inp2)
+model1 = Axon.add(inp1, inp2)
# Only inp2 will be used
-model2 = Axon.add(inp2, inp2)
Axon graphs are immutable, which means composing and manipulating
+
model2 = Axon.add(inp2, inp2)
Axon graphs are immutable, which means composing and manipulating an Axon graph creates an entirely new graph. Additionally, layer names are lazily generated at model execution time. To avoid non-deterministic input orderings and names, Axon requires each input to have a unique binary identifier. You can then reference -inputs by name when passing to models at execution time:
inp1 = Axon.input("input_0", shape: {nil, 1})
-inp2 = Axon.input("input_1", shape: {nil, 1})
+inputs by name when passing to models at execution time:inp1 = Axon.input("input_0", shape: {nil, 1})
+inp2 = Axon.input("input_1", shape: {nil, 1})
-model1 = Axon.add(inp1, inp2)
+model1 = Axon.add(inp1, inp2)
-{init_fn, predict_fn} = Axon.build(model1)
+{init_fn, predict_fn} = Axon.build(model1)
-params1 = init_fn.(Nx.template({1, 1}, {:f, 32}), %{})
+params1 = init_fn.(Nx.template({1, 1}, {:f, 32}), %{})
# Inputs are referenced by name
-predict_fn.(params1, %{"input_0" => x, "input_1" => y})
+
predict_fn.(params1, %{"input_0" => x, "input_1" => y})
multiple-outputs
@@ -208,13 +208,13 @@Nx offers robust container support which is extended to Axon. Axon allows you to wrap any valid Nx container -in a layer. Containers are most commonly used to structure outputs:
inp1 = Axon.input("input_0", shape: {nil, 1})
-inp2 = Axon.input("input_1", shape: {nil, 1})
-model = Axon.container(%{foo: inp1, bar: inp2})
Containers can be arbitrarily nested:
inp1 = Axon.input("input_0", shape: {nil, 1})
-inp2 = Axon.input("input_1", shape: {nil, 1})
-model = Axon.container({%{foo: {inp1, %{bar: inp2}}}})
You can even use custom structs which implement the container protocol:
inp1 = Axon.input("input_0", shape: {nil, 1})
-inp2 = Axon.input("input_1", shape: {nil, 1})
-model = Axon.container(%MyStruct{foo: inp1, bar: inp2})
inp1 = Axon.input("input_0", shape: {nil, 1})
+inp2 = Axon.input("input_1", shape: {nil, 1})
+model = Axon.container(%{foo: inp1, bar: inp2})
Containers can be arbitrarily nested:
inp1 = Axon.input("input_0", shape: {nil, 1})
+inp2 = Axon.input("input_1", shape: {nil, 1})
+model = Axon.container({%{foo: {inp1, %{bar: inp2}}}})
You can even use custom structs which implement the container protocol:
inp1 = Axon.input("input_0", shape: {nil, 1})
+inp2 = Axon.input("input_1", shape: {nil, 1})
+model = Axon.container(%MyStruct{foo: inp1, bar: inp2})
custom-layers
@@ -225,18 +225,18 @@input
, constant
, and container
)
make use of this same API.Axon layers are really just placeholders for Nx computations with trainable
parameters and possibly state. To define a custom layer, you just need to
-define a defn
implementation:
defn my_layer(x, weight, _opts \ []) do
- Nx.atan2(x, weight)
-end
Notice the only stipulation is that your custom layer implementation must
+define a defn
implementation:
defn my_layer(x, weight, _opts \ []) do
+ Nx.atan2(x, weight)
+end
Notice the only stipulation is that your custom layer implementation must
accept at least 1 input and a list of options. At execution time, every
layer will be passed a :mode
option which can be used to control behavior
at training and inference time.
Inputs to your custom layer can be either Axon graph inputs or trainable
parameters. You can pass Axon graph inputs as-is to a custom layer. To
-declare trainable parameters, use Axon.param/3
:
weight = Axon.param("weight", param_shape)
To create a custom layer, you "wrap" your implementation and inputs into
-a layer using Axon.layer
. You'll notice the API mirrors Elixir's apply
:
def atan2_layer(%Axon{} = input) do
- weight = Axon.param("weight", param_shape)
- Axon.layer(&my_layer/3, [input, weight])
-end
Axon.param/3
:weight = Axon.param("weight", param_shape)
To create a custom layer, you "wrap" your implementation and inputs into
+a layer using Axon.layer
. You'll notice the API mirrors Elixir's apply
:
def atan2_layer(%Axon{} = input) do
+ weight = Axon.param("weight", param_shape)
+ Axon.layer(&my_layer/3, [input, weight])
+end
model-execution
@@ -245,16 +245,16 @@Under the hood, Axon models are represented as Elixir structs. You
can initialize and apply models by building or compiling them with
Axon.build/2
or Axon.compile/4
and then calling the produced
-initialization and predict functions:
{init_fn, predict_fn} = Axon.build(model)
+initialization and predict functions:{init_fn, predict_fn} = Axon.build(model)
-init_fn.(Nx.template({1, 1}, {:f, 32}), %{})
-predict_fn.(params, inputs)
You may either set the default JIT compiler or backend globally, or
-pass a specific compiler to Axon.build/2
:
EXLA.set_as_nx_default([:tpu, :cuda, :rocm, :host])
+init_fn.(Nx.template({1, 1}, {:f, 32}), %{})
+predict_fn.(params, inputs)
You may either set the default JIT compiler or backend globally, or
+pass a specific compiler to Axon.build/2
:
EXLA.set_as_nx_default([:tpu, :cuda, :rocm, :host])
-{init_fn, predict_fn} = Axon.build(model, compiler: EXLA, mode: :train)
+{init_fn, predict_fn} = Axon.build(model, compiler: EXLA, mode: :train)
-params = init_fn.(Nx.template({1, 1}, {:f, 32}), %{})
-predict_fn.(params, inputs)
predict_fn
by default runs in inference mode, which performs certain
+
params = init_fn.(Nx.template({1, 1}, {:f, 32}), %{})
+predict_fn.(params, inputs)
predict_fn
by default runs in inference mode, which performs certain
optimizations and removes layers such as dropout layers. If constructing
a training step using Axon.predict/4
or Axon.build/2
, be sure to specify
mode: :train
.
Combining the Axon model creation API with the optimization and training APIs, you can create and train neural networks with ease:
model =
- Axon.input("input_0", shape: {nil, 784})
- |> Axon.dense(128, activation: :relu)
- |> Axon.layer_norm()
- |> Axon.dropout()
- |> Axon.dense(10, activation: :softmax)
+ Axon.input("input_0", shape: {nil, 784})
+ |> Axon.dense(128, activation: :relu)
+ |> Axon.layer_norm()
+ |> Axon.dropout()
+ |> Axon.dense(10, activation: :softmax)
IO.inspect model
model_state =
model
- |> Axon.Loop.trainer(:categorical_cross_entropy, Axon.Optimizers.adamw(0.005))
- |> Axon.Loop.run(train_data, epochs: 10, compiler: EXLA)
See Axon.Updates
and Axon.Loop
for a more in-depth treatment of
+ |> Axon.Loop.trainer(:categorical_cross_entropy, Axon.Optimizers.adamw(0.005))
+ |> Axon.Loop.run(train_data, epochs: 10, compiler: EXLA)
See Axon.Updates
and Axon.Loop
for a more in-depth treatment of
model optimization and model training.
using-with-nx-serving
@@ -287,44 +287,44 @@Nx
already has an abstraction for this task in the
form of Nx.Serving
. Here's how you could define a serving for an Axon
-model:def build_serving() do
+model:def build_serving() do
# Configuration
batch_size = 4
- defn_options = [compiler: EXLA]
+ defn_options = [compiler: EXLA]
- Nx.Serving.new(
+ Nx.Serving.new(
# This function runs on the serving startup
- fn ->
+ fn ->
# Build the Axon model and load params (usually from file)
- model = build_model()
- params = load_params()
+ model = build_model()
+ params = load_params()
# Build the prediction defn function
- {_init_fun, predict_fun} = Axon.build(model)
+ {_init_fun, predict_fun} = Axon.build(model)
- inputs_template = %{"pixel_values" => Nx.template({batch_size, 224, 224, 3}, :f32)}
- template_args = [Nx.to_template(params), inputs_template]
+ inputs_template = %{"pixel_values" => Nx.template({batch_size, 224, 224, 3}, :f32)}
+ template_args = [Nx.to_template(params), inputs_template]
# Compile the prediction function upfront for the configured batch_size
- predict_fun = Nx.Defn.compile(predict_fun, template_args, defn_options)
+ predict_fun = Nx.Defn.compile(predict_fun, template_args, defn_options)
# The returned function is called for every accumulated batch
- fn inputs ->
- inputs = Nx.Batch.pad(inputs, batch_size - inputs.size)
- predict_fun.(params, inputs)
- end
- end,
+ fn inputs ->
+ inputs = Nx.Batch.pad(inputs, batch_size - inputs.size)
+ predict_fun.(params, inputs)
+ end
+ end,
batch_size: batch_size
- )
-end
Then you would start the serving server as part of your application's
-supervision tree:
children = [
+ )
+end
Then you would start the serving server as part of your application's
+supervision tree:
children = [
...,
- {Nx.Serving, serving: build_serving(), name: MyApp.Serving, batch_timeout: 100}
-]
With that in place, you can now ask serving for predictions all across
+
{Nx.Serving, serving: build_serving(), name: MyApp.Serving, batch_timeout: 100}
+]
With that in place, you can now ask serving for predictions all across your application (controllers, live views, async jobs, etc.). Having a -tensor input you would do:
inputs = %{"pixel_values" => ...}
-batch = Nx.Batch.concatenate([inputs])
-result = Nx.Serving.batched_run(MyApp.Serving, batch)
Usually you also want to do pre/post-processing of the model input/output. +tensor input you would do:
inputs = %{"pixel_values" => ...}
+batch = Nx.Batch.concatenate([inputs])
+result = Nx.Serving.batched_run(MyApp.Serving, batch)
Usually you also want to do pre/post-processing of the model input/output.
You could make those preparations directly before/after Nx.Serving.batched_run/2
,
however you can also make use of Nx.Serving.client_preprocessing/2
and
Nx.Serving.client_postprocessing/2
to encapsulate that logic as part of
@@ -1560,9 +1560,9 @@
Adds a constant layer to the network.
Constant layers encapsulate Nx tensors in an Axon layer for ease of use with other Axon layers. They can be used interchangeably -with other Axon layers:
inp = Axon.input("input", shape: {nil, 32})
-my_constant = Axon.constant(Nx.iota({1, 32}))
-model = Axon.add(inp, my_constant)
Constant layers will be cast according to the mixed precision policy. +with other Axon layers:
inp = Axon.input("input", shape: {nil, 32})
+my_constant = Axon.constant(Nx.iota({1, 32}))
+model = Axon.add(inp, my_constant)
Constant layers will be cast according to the mixed precision policy. If it's important for your constant to retain it's type during the computation, you will need to set the mixed precision policy to ignore constant layers.
iex> inp1 = Axon.input("input_0", shape: {nil, 1})
-iex> inp2 = Axon.input("input_1", shape: {nil, 2})
-iex> model = Axon.container(%{a: inp1, b: inp2})
-iex> %{a: a, b: b} = Axon.predict(model, %{}, %{
-...> "input_0" => Nx.tensor([[1.0]]),
-...> "input_1" => Nx.tensor([[1.0, 2.0]])
-...> })
+iex> inp1 = Axon.input("input_0", shape: {nil, 1})
+iex> inp2 = Axon.input("input_1", shape: {nil, 2})
+iex> model = Axon.container(%{a: inp1, b: inp2})
+iex> %{a: a, b: b} = Axon.predict(model, %{}, %{
+...> "input_0" => Nx.tensor([[1.0]]),
+...> "input_1" => Nx.tensor([[1.0, 2.0]])
+...> })
iex> a
-#Nx.Tensor<
- f32[1][1]
- [
- [1.0]
- ]
->
+#Nx.Tensor<
+ f32[1][1]
+ [
+ [1.0]
+ ]
+>
iex> b
-#Nx.Tensor<
- f32[1][2]
- [
- [1.0, 2.0]
- ]
->
+#Nx.Tensor<
+ f32[1][2]
+ [
+ [1.0, 2.0]
+ ]
+>
:name
- layer name.:op_name
- layer operation for inspection and building parameter map.:mode
- if the layer should run only on :inference
or :train
. Defaults to :both
Note this means your layer should not use these as input options, as they will always be dropped during inference compilation.
Axon's compiler will additionally forward the following options to every layer at inference time:
:mode
- :inference
or :train
. To control layer behavior
-based on inference or train time.op
is a function of the form:
fun = fn input, weight, bias, _opts ->
+based on inference or train time.op
is a function of the form:
fun = fn input, weight, bias, _opts ->
input * weight + bias
-end
+end
{base, resnet_params} = resnet()
-base = base |> Axon.namespace("resnet")
+fine-tuning:{base, resnet_params} = resnet()
+base = base |> Axon.namespace("resnet")
-model = base |> Axon.dense(1)
-{init_fn, predict_fn} = Axon.build(model)
+model = base |> Axon.dense(1)
+{init_fn, predict_fn} = Axon.build(model)
-init_fn.(Nx.template({1, 3, 224, 224}, {:f, 32}), %{"resnset" => resnet_params})
Notice you can use init_fn
in conjunction with namespaces
+
init_fn.(Nx.template({1, 3, 224, 224}, {:f, 32}), %{"resnset" => resnet_params})
Notice you can use init_fn
in conjunction with namespaces
to specify which portion of a model you'd like to initialize
from a fixed starting point.
Namespaces have fixed names, which means it's easy to run into namespace collisions. Re-using namespaces, re-using inner parts of a namespace, @@ -1764,8 +1764,8 @@
Applies the given Nx
expression to the input.
Nx layers are meant for quick applications of functions without trainable parameters. For example, they are useful for applying -functions which apply accessors to containers:
model = Axon.container({foo, bar})
-Axon.nx(model, &elem(&1, 0))
model = Axon.container({foo, bar})
+Axon.nx(model, &elem(&1, 0))
options
@@ -1796,38 +1796,38 @@Wraps an Axon model in an optional node.
By default, when an optional input is missing, all subsequent layers -are nullified. For example, consider this model:
values = Axon.input("values")
-mask = Axon.input("mask", optional: true)
+are nullified. For example, consider this model:values = Axon.input("values")
+mask = Axon.input("mask", optional: true)
model =
values
- |> Axon.dense(10)
- |> Axon.multiply(mask)
- |> Axon.dense(1)
- |> Axon.sigmoid()
In case the mask is not provided, the input node will resolve to
+
|> Axon.dense(10)
+ |> Axon.multiply(mask)
+ |> Axon.dense(1)
+ |> Axon.sigmoid()
In case the mask is not provided, the input node will resolve to
%Axon.None{}
and so will all the layers that depend on it. By
using optional/2
a layer may opt-in to receive %Axon.None{}
.
To fix our example, we could define a custom layer to apply the
-mask only when present
def apply_optional_mask(%Axon{} = x, %Axon{} = mask) do
- Axon.layer(
- fn x, mask, _opts ->
- case mask do
- %Axon.None{} -> x
- mask -> Nx.multiply(x, mask)
- end
- end,
- [x, Axon.optional(mask)]
- )
-end
+mask only when presentdef apply_optional_mask(%Axon{} = x, %Axon{} = mask) do
+ Axon.layer(
+ fn x, mask, _opts ->
+ case mask do
+ %Axon.None{} -> x
+ mask -> Nx.multiply(x, mask)
+ end
+ end,
+ [x, Axon.optional(mask)]
+ )
+end
# ...
model =
values
- |> Axon.dense(10)
- |> apply_optional_mask(mask)
- |> Axon.dense(1)
- |> Axon.sigmoid()
+
|> Axon.dense(10)
+ |> apply_optional_mask(mask)
+ |> Axon.dense(1)
+ |> Axon.sigmoid()
options
@@ -2671,7 +2671,7 @@Adds a bilinear layer to the network.
The bilinear layer implements:
output = activation(dot(dot(input1, kernel), input2) + bias)
where activation
is given by the :activation
option and both
+
Adds a bilinear layer to the network.
The bilinear layer implements:
output = activation(dot(dot(input1, kernel), input2) + bias)
where activation
is given by the :activation
option and both
kernel
and bias
are layer parameters. units
specifies the
number of output units.
All dimensions but the last of input1
and input2
must match. The
batch sizes of both inputs must also match or at least one must be nil
.
@@ -2708,7 +2708,7 @@
Adds a dense layer to the network.
The dense layer implements:
output = activation(dot(input, kernel) + bias)
where activation
is given by the :activation
option and both
+
Adds a dense layer to the network.
The dense layer implements:
output = activation(dot(input, kernel) + bias)
where activation
is given by the :activation
option and both
kernel
and bias
are layer parameters. units
specifies the
number of output units.
Compiles to Axon.Layers.dense/4
.
ConvLSTMs apply Axon.Layers.conv_lstm_cell/5
over an entire input
-sequence and return:
{{new_cell, new_hidden}, output_sequence}
You can use the output state as the hidden state of another +sequence and return:
{{new_cell, new_hidden}, output_sequence}
You can use the output state as the hidden state of another ConvLSTM layer.
options
@@ -3726,7 +3726,7 @@Adds a gated recurrent unit (GRU) layer to the network with the given initial hidden state.
GRUs apply Axon.Layers.gru_cell/7
over an entire input
-sequence and return:
{{new_hidden}, output_sequence}
You can use the output state as the hidden state of another +sequence and return:
{{new_hidden}, output_sequence}
You can use the output state as the hidden state of another GRU layer.
options
@@ -3813,7 +3813,7 @@LSTMs apply Axon.Layers.lstm_cell/7
over an entire input
-sequence and return:
{output_sequence, {new_cell, new_hidden}}
You can use the output state as the hidden state of another +sequence and return:
{output_sequence, {new_cell, new_hidden}}
You can use the output state as the hidden state of another LSTM layer.
options
@@ -4244,16 +4244,16 @@init_fn
The init_fn
receives two arguments, the input template and
-an optional map with initial parameters for layers or namespaces:
{init_fn, predict_fn} = Axon.build(model)
-init_fn.(Nx.template({1, 1}, {:f, 32}), %{"dense_0" => dense_params})
{init_fn, predict_fn} = Axon.build(model)
+init_fn.(Nx.template({1, 1}, {:f, 32}), %{"dense_0" => dense_params})
predict_fn
predict_fn
The predict_fn
receives two arguments, the trained parameters
-and the actual inputs:
{_init_fn, predict_fn} = Axon.build(model, opts)
-predict_fn.(params, input)
{_init_fn, predict_fn} = Axon.build(model, opts)
+predict_fn.(params, input)
options
@@ -4334,19 +4334,19 @@iex> model = Axon.input("input", shape: {nil, 2}) |> Axon.dense(1, kernel_initializer: :zeros, activation: :relu)
-iex> {init_fn, _} = Axon.build(model)
-iex> params = init_fn.(Nx.template({1, 2}, :f32), %{})
-iex> serialized = Axon.serialize(model, params)
-iex> {saved_model, saved_params} = Axon.deserialize(serialized)
-iex> {_, predict_fn} = Axon.build(saved_model)
-iex> predict_fn.(saved_params, Nx.tensor([[1.0, 1.0]]))
-#Nx.Tensor<
- f32[1][1]
- [
- [0.0]
- ]
->
+iex> model = Axon.input("input", shape: {nil, 2}) |> Axon.dense(1, kernel_initializer: :zeros, activation: :relu)
+iex> {init_fn, _} = Axon.build(model)
+iex> params = init_fn.(Nx.template({1, 2}, :f32), %{})
+iex> serialized = Axon.serialize(model, params)
+iex> {saved_model, saved_params} = Axon.deserialize(serialized)
+iex> {_, predict_fn} = Axon.build(saved_model)
+iex> predict_fn.(saved_params, Nx.tensor([[1.0, 1.0]]))
+#Nx.Tensor<
+ f32[1][1]
+ [
+ [0.0]
+ ]
+>
cnn_base = get_pretrained_cnn_base()
+in code here:cnn_base = get_pretrained_cnn_base()
model =
cnn_base
- |> Axon.freeze()
- |> Axon.flatten()
- |> Axon.dense(1024, activation: :relu)
- |> Axon.dropout()
- |> Axon.dense(1000, activation: :softmax)
+ |> Axon.freeze()
+ |> Axon.flatten()
+ |> Axon.dense(1024, activation: :relu)
+ |> Axon.dropout()
+ |> Axon.dense(1000, activation: :softmax)
model
-|> Axon.Loop.trainer(:categorical_cross_entropy, Axon.Optimizers.adam(0.005))
-|> Axon.Loop.run(data, epochs: 10)
When compiled, frozen parameters are wrapped in Nx.Defn.Kernel.stop_grad/1
,
+
|> Axon.Loop.trainer(:categorical_cross_entropy, Axon.Optimizers.adam(0.005))
+|> Axon.Loop.run(data, epochs: 10)
When compiled, frozen parameters are wrapped in Nx.Defn.Kernel.stop_grad/1
,
which zeros out the gradient with respect to the frozen parameter. Gradients
of frozen parameters will return 0.0
, meaning they won't be changed during
the update process.
iex> model = Axon.input("input", shape: {nil, 2}) |> Axon.dense(1, kernel_initializer: :zeros, activation: :relu)
-iex> {init_fn, _} = Axon.build(model)
-iex> params = init_fn.(Nx.template({1, 2}, :f32), %{})
-iex> serialized = Axon.serialize(model, params)
-iex> {saved_model, saved_params} = Axon.deserialize(serialized)
-iex> {_, predict_fn} = Axon.build(saved_model)
-iex> predict_fn.(saved_params, Nx.tensor([[1.0, 1.0]]))
-#Nx.Tensor<
- f32[1][1]
- [
- [0.0]
- ]
->
+iex> model = Axon.input("input", shape: {nil, 2}) |> Axon.dense(1, kernel_initializer: :zeros, activation: :relu)
+iex> {init_fn, _} = Axon.build(model)
+iex> params = init_fn.(Nx.template({1, 2}, :f32), %{})
+iex> serialized = Axon.serialize(model, params)
+iex> {saved_model, saved_params} = Axon.deserialize(serialized)
+iex> {_, predict_fn} = Axon.build(saved_model)
+iex> predict_fn.(saved_params, Nx.tensor([[1.0, 1.0]]))
+#Nx.Tensor<
+ f32[1][1]
+ [
+ [0.0]
+ ]
+>
true
if a parameter should be unfrozen or false
otherwise.Unfreezing parameters is useful when fine tuning a model which you have previously frozen and performed transfer learning on. You may want to unfreeze some of the later frozen layers in a model and -fine tune them specifically for your application:
cnn_base = get_pretrained_cnn_base()
+fine tune them specifically for your application:cnn_base = get_pretrained_cnn_base()
model =
frozen_model
- |> Axon.unfreeze(up: 25)
+ |> Axon.unfreeze(up: 25)
model
-|> Axon.Loop.trainer(:categorical_cross_entropy, Axon.Optimizers.adam(0.0005))
-|> Axon.Loop.run(data, epochs: 10)
When compiled, frozen parameters are wrapped in Nx.Defn.Kernel.stop_grad/1
,
+
|> Axon.Loop.trainer(:categorical_cross_entropy, Axon.Optimizers.adam(0.0005))
+|> Axon.Loop.run(data, epochs: 10)
When compiled, frozen parameters are wrapped in Nx.Defn.Kernel.stop_grad/1
,
which zeros out the gradient with respect to the frozen parameter. Gradients
of frozen parameters will return 0.0
, meaning they won't be changed during
the update process.
iex> model = Axon.input("input", shape: {nil, 1}) |> Axon.dense(2)
-iex> Axon.get_op_counts(model)
-%{input: 1, dense: 1}
+iex> model = Axon.input("input", shape: {nil, 1}) |> Axon.dense(2)
+iex> Axon.get_op_counts(model)
+%{input: 1, dense: 1}
-iex> model = Axon.input("input", shape: {nil, 1}) |> Axon.tanh() |> Axon.tanh()
-iex> Axon.get_op_counts(model)
-%{input: 1, tanh: 2}
+iex> model = Axon.input("input", shape: {nil, 1}) |> Axon.tanh() |> Axon.tanh()
+iex> Axon.get_op_counts(model)
+%{input: 1, tanh: 2}
instrumented_model = Axon. (model, fn
- %Axon{op: :conv} = graph ->
- Axon.attach_hook(graph, &visualize_activations/1)
+of all convolutional layers in a model:instrumented_model = Axon. (model, fn
+ %Axon{op: :conv} = graph ->
+ Axon.attach_hook(graph, &visualize_activations/1)
graph ->
graph
-end)
Another use case is to replace entire classes of layers
+
end)
Another use case is to replace entire classes of layers with another. For example, you may want to replace all -relu layers with tanh layers:
new_model = Axon.map_nodes(model, fn
- %Axon{op: :relu} = graph ->
+relu layers with tanh layers:new_model = Axon.map_nodes(model, fn
+ %Axon{op: :relu} = graph ->
# Get nodes immediate parent
- parent = Axon.get_parent(graph)
+ parent = Axon.get_parent(graph)
# Replace node with a tanh
- Axon.tanh(parent)
+ Axon.tanh(parent)
graph ->
graph
-end)
+end)
Pops the top node off of the graph.
This returns the popped node and the updated graph:
{_node, model} = Axon.pop_node(model)
+Pops the top node off of the graph.
This returns the popped node and the updated graph:
{_node, model} = Axon.pop_node(model)
Internally this function is used in several places to accumulate graph metadata. For example, you can use it to count the number -of a certain type of operation in the graph:
Axon.reduce_nodes(model, 0, fn
- %Axon.Nodes{op: :relu}, acc -> acc + 1
+of a certain type of operation in the graph:Axon.reduce_nodes(model, 0, fn
+ %Axon.Nodes{op: :relu}, acc -> acc + 1
_, acc -> acc
-end)
+end)
Attaches a hook to the given Axon model.
Hooks compile down to Nx.Defn.Kernel.hook/3
and provide the same
functionality for adding side-effecting operations to a compiled
model. For example, you can use hooks to inspect intermediate activations,
-send data to an external service, and more.
Hooks can be configured to be invoked on the following events:
:initialize
- on model initialization.:pre_forward
- before layer forward pass is invoked.:forward
- after layer forward pass is invoked.:backward
- after layer backward pass is invoked.To invoke a hook on every single event, you may pass :all
to on:
.
Axon.input("input", shape: {nil, 1}) |> Axon.attach_hook(&IO.inspect/1, on: :all)
The default event is :forward
, assuming you want a hook invoked
+send data to an external service, and more.
Hooks can be configured to be invoked on the following events:
:initialize
- on model initialization.:pre_forward
- before layer forward pass is invoked.:forward
- after layer forward pass is invoked.:backward
- after layer backward pass is invoked.To invoke a hook on every single event, you may pass :all
to on:
.
Axon.input("input", shape: {nil, 1}) |> Axon.attach_hook(&IO.inspect/1, on: :all)
The default event is :forward
, assuming you want a hook invoked
on the layers forward pass.
You may configure hooks to run in one of only training or inference
mode using the :mode
option. The default mode is :both
to be invoked
-during both train and inference mode.
Axon.input("input", shape: {nil, 1}) |> Axon.attach_hook(&IO.inspect/1, on: :forward, mode: :train)
You can also attach multiple hooks to a single layer. Hooks are invoked in +during both train and inference mode.
Axon.input("input", shape: {nil, 1}) |> Axon.attach_hook(&IO.inspect/1, on: :forward, mode: :train)
You can also attach multiple hooks to a single layer. Hooks are invoked in the order in which they are declared. If order is important, you should attach -hooks in the order you want them to be executed:
Axon.input("input", shape: {nil, 1})
+hooks in the order you want them to be executed:Axon.input("input", shape: {nil, 1})
# I will be executed first
-|> Axon.attach_hook(&IO.inspect/1)
+|> Axon.attach_hook(&IO.inspect/1)
# I will be executed second
-|> Axon.attach_hook(fn _ -> IO.write("HERE") end)
Hooks are executed at their point of attachment. You must insert hooks at each point
-you want a hook to execute during model execution.
Axon.input("input", shape: {nil, 1})
-|> Axon.attach_hook(&IO.inspect/1)
-|> Axon.relu()
-|> Axon.attach_hook(&IO.inspect/1)
+|> Axon.attach_hook(fn _ -> IO.write("HERE") end)
Hooks are executed at their point of attachment. You must insert hooks at each point +you want a hook to execute during model execution.
Axon.input("input", shape: {nil, 1})
+|> Axon.attach_hook(&IO.inspect/1)
+|> Axon.relu()
+|> Axon.attach_hook(&IO.inspect/1)
The returned expression is an Nx expression which can be traversed and lowered to an IR or inspected for debugging purposes.
You may optionally specify initial parameters for some layers or -namespaces by passing a partial parameter map:
Axon.trace_init(model, %{"dense_0" => dense_params})
The parameter map will be merged with the initialized model +namespaces by passing a partial parameter map:
Axon.trace_init(model, %{"dense_0" => dense_params})
The parameter map will be merged with the initialized model parameters.
options
diff --git a/accelerating_axon.html b/accelerating_axon.html index fe424b21..c7f0134a 100644 --- a/accelerating_axon.html +++ b/accelerating_axon.html @@ -115,81 +115,81 @@Mix.install([
- {:axon, github: "elixir-nx/axon"},
- {:exla, "~> 0.3.0", github: "elixir-nx/nx", sparse: "exla"},
- {:torchx, github: "elixir-nx/nx", sparse: "torchx"},
- {:nx, "~> 0.3.0", github: "elixir-nx/nx", sparse: "nx", override: true},
- {:benchee, github: "akoutmos/benchee", branch: :adding_table_support},
- {:kino_benchee, github: "livebook-dev/kino_benchee"},
- {:kino, "~> 0.7.0", override: true}
-])
:ok
Mix.install([
+ {:axon, github: "elixir-nx/axon"},
+ {:exla, "~> 0.3.0", github: "elixir-nx/nx", sparse: "exla"},
+ {:torchx, github: "elixir-nx/nx", sparse: "torchx"},
+ {:nx, "~> 0.3.0", github: "elixir-nx/nx", sparse: "nx", override: true},
+ {:benchee, github: "akoutmos/benchee", branch: :adding_table_support},
+ {:kino_benchee, github: "livebook-dev/kino_benchee"},
+ {:kino, "~> 0.7.0", override: true}
+])
:ok
using-nx-compilers-in-axon
Using Nx Compilers in AxonAxon is built entirely on top of Nx's numerical definitions defn
. Functions declared with defn
tell Nx to use just-in-time compilation to compile and execute the given numerical definition with an available Nx compiler. Numerical definitions enable acceleration on CPU/GPU/TPU via pluggable compilers. At the time of this writing, Nx has 2 officially supported compiler/backends on top of the default BinaryBackend
:
By default, Nx and Axon run all computations using the BinaryBackend
which is a pure Elixir implementation of various numerical routines. The BinaryBackend
is guaranteed to run wherever an Elixir installation runs; however, it is very slow. Due to the computational expense of neural networks, you should basically never use the BinaryBackend
and instead opt for one of the available accelerated libraries.
There are several ways to make use of Nx compilers from within Axon. First, create a simple model for benchmarking purposes:
model =
- Axon.input("data")
- |> Axon.dense(32)
- |> Axon.relu()
- |> Axon.dense(1)
- |> Axon.softmax()
#Axon<
- inputs: %{"data" => nil}
+ Axon.input("data")
+ |> Axon.dense(32)
+ |> Axon.relu()
+ |> Axon.dense(1)
+ |> Axon.softmax()
#Axon<
+ inputs: %{"data" => nil}
outputs: "softmax_0"
nodes: 5
->
By default, Axon will respect the default defn
compilation options. You can set compilation options globally or per-process:
# Sets the global compilation options
-Nx.Defn.global_default_options(compiler: EXLA)
+>
By default, Axon will respect the default defn
compilation options. You can set compilation options globally or per-process:
# Sets the global compilation options
+Nx.Defn.global_default_options(compiler: EXLA)
# Sets the process-level compilation options
-Nx.Defn.default_options(compiler: EXLA)
[compiler: EXLA]
When you call Axon.build/2
, Axon automatically marks your initialization and forward functions as JIT compiled functions. When you invoke them, they will compile a specialized version of the function using your default compiler options:
inputs = Nx.random_uniform({2, 128})
-{init_fn, predict_fn} = Axon.build(model)
-params = init_fn.(inputs, %{})
-predict_fn.(params, inputs)
-10:34:02.503 [info] XLA service 0x7fbd5468c170 initialized for platform Host (this does not guarantee that XLA will be used). Devices:
-
-10:34:02.785 [info] StreamExecutor device (0): Host, Default Version
-
#Nx.Tensor<
- f32[2][1]
- EXLA.Backend<host:0, 0.184501844.1168769032.259095>
- [
- [1.0],
- [1.0]
- ]
+Nx.Defn.default_options(compiler: EXLA)
[compiler: EXLA]
When you call Axon.build/2
, Axon automatically marks your initialization and forward functions as JIT compiled functions. When you invoke them, they will compile a specialized version of the function using your default compiler options:
inputs = Nx.random_uniform({2, 128})
+{init_fn, predict_fn} = Axon.build(model)
+params = init_fn.(inputs, %{})
+predict_fn.(params, inputs)
+10:34:02.503 [info] XLA service 0x7fbd5468c170 initialized for platform Host (this does not guarantee that XLA will be used). Devices:
+
+10:34:02.785 [info] StreamExecutor device (0): Host, Default Version
+
#Nx.Tensor<
+ f32[2][1]
+ EXLA.Backend<host:0, 0.184501844.1168769032.259095>
+ [
+ [1.0],
+ [1.0]
+ ]
>
Notice that the inspected tensor indicates the computation has been dispatched to EXLA and the tensor's data points to an EXLA buffer.
If you feel like setting the global or process-level compilation options is too intrusive, you can opt for more explicit behavior in a few ways. First, you can specify the JIT compiler when you build the model:
# Set back to defaults
-Nx.Defn.global_default_options([])
-Nx.Defn.default_options([])
[compiler: EXLA]
{init_fn, predict_fn} = Axon.build(model, compiler: EXLA)
-params = init_fn.(inputs, %{})
-predict_fn.(params, inputs)
#Nx.Tensor<
- f32[2][1]
- EXLA.Backend<host:0, 0.184501844.1168769032.259101>
- [
- [1.0],
- [1.0]
- ]
->
You can also instead JIT compile functions explicitly via the Nx.Defn.jit
or compiler-specific JIT APIs. This is useful when running benchmarks against various backends:
{init_fn, predict_fn} = Axon.build(model)
+Nx.Defn.global_default_options([])
+Nx.Defn.default_options([])
[compiler: EXLA]
{init_fn, predict_fn} = Axon.build(model, compiler: EXLA)
+params = init_fn.(inputs, %{})
+predict_fn.(params, inputs)
#Nx.Tensor<
+ f32[2][1]
+ EXLA.Backend<host:0, 0.184501844.1168769032.259101>
+ [
+ [1.0],
+ [1.0]
+ ]
+>
You can also instead JIT compile functions explicitly via the Nx.Defn.jit
or compiler-specific JIT APIs. This is useful when running benchmarks against various backends:
{init_fn, predict_fn} = Axon.build(model)
# These will both JIT compile with EXLA
-exla_init_fn = Nx.Defn.jit(init_fn, compiler: EXLA)
-exla_predict_fn = EXLA.jit(predict_fn)
#Function<136.40088443/2 in Nx.Defn.wrap_arity/2>
Benchee.run(
- %{
- "elixir init" => fn -> init_fn.(inputs, %{}) end,
- "exla init" => fn -> exla_init_fn.(inputs, %{}) end
- },
+exla_init_fn = Nx.Defn.jit(init_fn, compiler: EXLA)
+exla_predict_fn = EXLA.jit(predict_fn)
#Function<136.40088443/2 in Nx.Defn.wrap_arity/2>
Benchee.run(
+ %{
+ "elixir init" => fn -> init_fn.(inputs, %{}) end,
+ "exla init" => fn -> exla_init_fn.(inputs, %{}) end
+ },
time: 10,
memory_time: 5,
warmup: 2
-)
Warning: the benchmark elixir init is using an evaluated function.
+)
Warning: the benchmark elixir init is using an evaluated function.
Evaluated functions perform slower than compiled functions.
- You can move the Benchee caller to a function in a module and invoke `Mod.fun()` instead.
+ You can move the Benchee caller to a function in a module and invoke `Mod.fun()` instead.
Alternatively, you can move the benchmark into a benchmark.exs file and run mix run benchmark.exs
Warning: the benchmark exla init is using an evaluated function.
Evaluated functions perform slower than compiled functions.
- You can move the Benchee caller to a function in a module and invoke `Mod.fun()` instead.
+ You can move the Benchee caller to a function in a module and invoke `Mod.fun()` instead.
Alternatively, you can move the benchmark into a benchmark.exs file and run mix run benchmark.exs
Operating System: Linux
-CPU Information: Intel(R) Core(TM) i7-7600U CPU @ 2.80GHz
+CPU Information: Intel(R) Core(TM) i7-7600U CPU @ 2.80GHz
Number of Available Cores: 4
Available memory: 24.95 GB
Elixir 1.13.4
@@ -221,26 +221,26 @@
exla init 9.80 KB
elixir init 644.63 KB - 65.80x memory usage +634.83 KB
-**All measurements for memory usage were the same**
Benchee.run(
- %{
- "elixir predict" => fn -> predict_fn.(params, inputs) end,
- "exla predict" => fn -> exla_predict_fn.(params, inputs) end
- },
+**All measurements for memory usage were the same**
Benchee.run(
+ %{
+ "elixir predict" => fn -> predict_fn.(params, inputs) end,
+ "exla predict" => fn -> exla_predict_fn.(params, inputs) end
+ },
time: 10,
memory_time: 5,
warmup: 2
-)
Warning: the benchmark elixir predict is using an evaluated function.
+)
Warning: the benchmark elixir predict is using an evaluated function.
Evaluated functions perform slower than compiled functions.
- You can move the Benchee caller to a function in a module and invoke `Mod.fun()` instead.
+ You can move the Benchee caller to a function in a module and invoke `Mod.fun()` instead.
Alternatively, you can move the benchmark into a benchmark.exs file and run mix run benchmark.exs
Warning: the benchmark exla predict is using an evaluated function.
Evaluated functions perform slower than compiled functions.
- You can move the Benchee caller to a function in a module and invoke `Mod.fun()` instead.
+ You can move the Benchee caller to a function in a module and invoke `Mod.fun()` instead.
Alternatively, you can move the benchmark into a benchmark.exs file and run mix run benchmark.exs
Operating System: Linux
-CPU Information: Intel(R) Core(TM) i7-7600U CPU @ 2.80GHz
+CPU Information: Intel(R) Core(TM) i7-7600U CPU @ 2.80GHz
Number of Available Cores: 4
Available memory: 24.95 GB
Elixir 1.13.4
@@ -279,29 +279,29 @@
Using Nx Backends in Axon
In addition to JIT-compilation, Axon also supports the usage of Nx backends. Nx backends are slightly different than Nx compilers in the sense that they do not fuse calls within numerical definitions. Backends are more eager, sacrificing a bit of performance for convenience. Torchx and EXLA both support running via backends.
Again, Axon will respect the global and process-level Nx backend configuration options. You can set the default backend using:
# Global default backend
-Nx.global_default_backend(Torchx.Backend)
+Nx.global_default_backend(Torchx.Backend)
# Process default backend
-Nx.default_backend(Torchx.Backend)
{Nx.BinaryBackend, []}
Now when you invoke model functions, it will run them with the given backend:
{init_fn, predict_fn} = Axon.build(model)
-params = init_fn.(inputs, %{})
-predict_fn.(params, inputs)
#Nx.Tensor<
- f32[2][1]
- Torchx.Backend(cpu)
- [
- [1.0],
- [1.0]
- ]
->
# Global default backend
-Nx.global_default_backend(EXLA.Backend)
+Nx.default_backend(Torchx.Backend)
{Nx.BinaryBackend, []}
Now when you invoke model functions, it will run them with the given backend:
{init_fn, predict_fn} = Axon.build(model)
+params = init_fn.(inputs, %{})
+predict_fn.(params, inputs)
#Nx.Tensor<
+ f32[2][1]
+ Torchx.Backend(cpu)
+ [
+ [1.0],
+ [1.0]
+ ]
+>
# Global default backend
+Nx.global_default_backend(EXLA.Backend)
# Process default backend
-Nx.default_backend(EXLA.Backend)
{Torchx.Backend, []}
{init_fn, predict_fn} = Axon.build(model)
-params = init_fn.(inputs, %{})
-predict_fn.(params, inputs)
#Nx.Tensor<
- f32[2][1]
- EXLA.Backend<host:0, 0.184501844.1169293320.110725>
- [
- [1.0],
- [1.0]
- ]
+Nx.default_backend(EXLA.Backend)
{Torchx.Backend, []}
{init_fn, predict_fn} = Axon.build(model)
+params = init_fn.(inputs, %{})
+predict_fn.(params, inputs)
#Nx.Tensor<
+ f32[2][1]
+ EXLA.Backend<host:0, 0.184501844.1169293320.110725>
+ [
+ [1.0],
+ [1.0]
+ ]
>
Unlike with JIT-compilation, you must set the backend at the top-level in order to invoke it. You should be careful using multiple backends in the same project as attempting to mix tensors between backends may result in strange performance bugs or errors.
With most larger models, using a JIT compiler will be more performant than using a backend.
a-note-on-cpus-gpus-tpus
diff --git a/complex_models.html b/complex_models.html
index fa6364e5..a85aa5eb 100644
--- a/complex_models.html
+++ b/complex_models.html
@@ -115,27 +115,27 @@
-Mix.install([
- {:axon, github: "elixir-nx/axon"},
- {:nx, "~> 0.3.0", github: "elixir-nx/nx", sparse: "nx", override: true},
- {:kino, "~> 0.7.0"}
-])
:ok
+Mix.install([
+ {:axon, github: "elixir-nx/axon"},
+ {:nx, "~> 0.3.0", github: "elixir-nx/nx", sparse: "nx", override: true},
+ {:kino, "~> 0.7.0"}
+])
:ok
creating-more-complex-models
Creating more complex models
-
Not all models you'd want to create fit cleanly in the sequential paradigm. Some models require a more flexible API. Fortunately, because Axon models are just Elixir data structures, you can manipulate them and decompose architectures as you would any other Elixir program:
input = Axon.input("data")
+Not all models you'd want to create fit cleanly in the sequential paradigm. Some models require a more flexible API. Fortunately, because Axon models are just Elixir data structures, you can manipulate them and decompose architectures as you would any other Elixir program:
input = Axon.input("data")
-x1 = input |> Axon.dense(32)
-x2 = input |> Axon.dense(64) |> Axon.relu() |> Axon.dense(32)
+x1 = input |> Axon.dense(32)
+x2 = input |> Axon.dense(64) |> Axon.relu() |> Axon.dense(32)
-out = Axon.add(x1, x2)
#Axon<
- inputs: %{"data" => nil}
+out = Axon.add(x1, x2)
#Axon<
+ inputs: %{"data" => nil}
outputs: "add_0"
nodes: 7
->
In the snippet above, your model branches input
into x1
and x2
. Each branch performs a different set of transformations; however, at the end the branches are merged with an Axon.add/3
. You might sometimes see layers like Axon.add/3
called combinators. Really they're just layers that operate on multiple Axon models at once - typically to merge some branches together.
out
represents your final Axon model.
If you visualize this model, you can see the full effect of the branching in this model:
template = Nx.template({2, 8}, :f32)
-Axon.Display.as_graph(out, template)
graph TD;
+
>
In the snippet above, your model branches input
into x1
and x2
. Each branch performs a different set of transformations; however, at the end the branches are merged with an Axon.add/3
. You might sometimes see layers like Axon.add/3
called combinators. Really they're just layers that operate on multiple Axon models at once - typically to merge some branches together.
out
represents your final Axon model.
If you visualize this model, you can see the full effect of the branching in this model:
template = Nx.template({2, 8}, :f32)
+Axon.Display.as_graph(out, template)
graph TD;
3[/"data (:input) {2, 8}"/];
6["dense_0 (:dense) {2, 32}"];
9["dense_1 (:dense) {2, 64}"];
@@ -149,43 +149,43 @@
10 --> 13;
9 --> 10;
3 --> 9;
-3 --> 6;
And you can use Axon.build/2
on out
as you would any other Axon model:
{init_fn, predict_fn} = Axon.build(out)
{#Function<135.51955502/2 in Nx.Defn.Compiler.fun/2>,
- #Function<135.51955502/2 in Nx.Defn.Compiler.fun/2>}
params = init_fn.(template, %{})
-predict_fn.(params, Nx.iota({2, 8}, type: :f32))
#Nx.Tensor<
- f32[2][32]
- [
- [-3.4256787300109863, -0.866683840751648, -0.2629307508468628, 3.2555718421936035, 2.2740533351898193, 3.0403499603271484, -2.7904915809631348, 3.4799132347106934, -4.16396951675415, -4.545778274536133, 3.146249532699585, -3.0786540508270264, 3.4500746726989746, 1.1419837474822998, -0.7993628978729248, 2.3798861503601074, 4.787802696228027, 1.290929913520813, 1.8274409770965576, -1.5016275644302368, 3.441028118133545, -1.8077948093414307, 0.25549376010894775, -2.555987596511841, -4.643674850463867, 2.164360523223877, -0.30402517318725586, -2.54134464263916, -2.699089527130127, 4.074007511138916, -0.7711544036865234, -3.988246202468872],
- [-11.235082626342773, -1.5991168022155762, -4.076810836791992, 11.091293334960938, 4.669280052185059, 12.756690979003906, -1.4954360723495483, 4.8143310546875, -14.211947441101074, -11.360504150390625, 6.239661693572998, -0.9994411468505859, 8.645132064819336, -0.5422897338867188, -1.4019453525543213, 9.633858680725098, 10.077424049377441, -0.3623824119567871, ...]
- ]
->
As your architectures grow in complexity, you might find yourself reaching for better abstractions to organize your model creation code. For example, PyTorch models are often organized into nn.Module
. The equivalent of an nn.Module
in Axon is a regular Elixir function. If you're translating models from PyTorch to Axon, it's natural to create one Elixir function per nn.Module
.
You should write your models as you would write any other Elixir code - you don't need to worry about any framework specific constructs:
defmodule MyModel do
- def model() do
- Axon.input("data")
- |> conv_block()
- |> Axon.flatten()
- |> dense_block()
- |> dense_block()
- |> Axon.dense(1)
- end
-
- defp conv_block(input) do
+3 --> 6;
And you can use Axon.build/2
on out
as you would any other Axon model:
{init_fn, predict_fn} = Axon.build(out)
{#Function<135.51955502/2 in Nx.Defn.Compiler.fun/2>,
+ #Function<135.51955502/2 in Nx.Defn.Compiler.fun/2>}
params = init_fn.(template, %{})
+predict_fn.(params, Nx.iota({2, 8}, type: :f32))
#Nx.Tensor<
+ f32[2][32]
+ [
+ [-3.4256787300109863, -0.866683840751648, -0.2629307508468628, 3.2555718421936035, 2.2740533351898193, 3.0403499603271484, -2.7904915809631348, 3.4799132347106934, -4.16396951675415, -4.545778274536133, 3.146249532699585, -3.0786540508270264, 3.4500746726989746, 1.1419837474822998, -0.7993628978729248, 2.3798861503601074, 4.787802696228027, 1.290929913520813, 1.8274409770965576, -1.5016275644302368, 3.441028118133545, -1.8077948093414307, 0.25549376010894775, -2.555987596511841, -4.643674850463867, 2.164360523223877, -0.30402517318725586, -2.54134464263916, -2.699089527130127, 4.074007511138916, -0.7711544036865234, -3.988246202468872],
+ [-11.235082626342773, -1.5991168022155762, -4.076810836791992, 11.091293334960938, 4.669280052185059, 12.756690979003906, -1.4954360723495483, 4.8143310546875, -14.211947441101074, -11.360504150390625, 6.239661693572998, -0.9994411468505859, 8.645132064819336, -0.5422897338867188, -1.4019453525543213, 9.633858680725098, 10.077424049377441, -0.3623824119567871, ...]
+ ]
+>
As your architectures grow in complexity, you might find yourself reaching for better abstractions to organize your model creation code. For example, PyTorch models are often organized into nn.Module
. The equivalent of an nn.Module
in Axon is a regular Elixir function. If you're translating models from PyTorch to Axon, it's natural to create one Elixir function per nn.Module
.
You should write your models as you would write any other Elixir code - you don't need to worry about any framework specific constructs:
defmodule MyModel do
+ def model() do
+ Axon.input("data")
+ |> conv_block()
+ |> Axon.flatten()
+ |> dense_block()
+ |> dense_block()
+ |> Axon.dense(1)
+ end
+
+ defp conv_block(input) do
residual = input
- x = input |> Axon.conv(3, padding: :same) |> Axon.mish()
+ x = input |> Axon.conv(3, padding: :same) |> Axon.mish()
x
- |> Axon.add(residual)
- |> Axon.max_pool(kernel_size: {2, 2})
- end
-
- defp dense_block(input) do
- input |> Axon.dense(32) |> Axon.relu()
- end
-end
{:module, MyModel, <<70, 79, 82, 49, 0, 0, 8, ...>>, {:dense_block, 1}}
model = MyModel.model()
#Axon<
- inputs: %{"data" => nil}
+ |> Axon.add(residual)
+ |> Axon.max_pool(kernel_size: {2, 2})
+ end
+
+ defp dense_block(input) do
+ input |> Axon.dense(32) |> Axon.relu()
+ end
+end
{:module, MyModel, <<70, 79, 82, 49, 0, 0, 8, ...>>, {:dense_block, 1}}
model = MyModel.model()
#Axon<
+ inputs: %{"data" => nil}
outputs: "dense_2"
nodes: 12
->
template = Nx.template({1, 28, 28, 3}, :f32)
-Axon.Display.as_graph(model, template)
graph TD;
+
>
template = Nx.template({1, 28, 28, 3}, :f32)
+Axon.Display.as_graph(model, template)
graph TD;
16[/"data (:input) {1, 28, 28, 3}"/];
19["conv_0 (:conv) {1, 28, 28, 3}"];
20["mish_0 (:mish) {1, 28, 28, 3}"];
diff --git a/credit_card_fraud.html b/credit_card_fraud.html
index b22da571..07832b38 100644
--- a/credit_card_fraud.html
+++ b/credit_card_fraud.html
@@ -115,18 +115,18 @@
-Mix.install([
- {:axon, "~> 0.3.0"},
- {:nx, "~> 0.4.0", override: true},
- {:exla, "~> 0.4.0"},
- {:explorer, "~> 0.3.1"},
- {:kino, "~> 0.7.0"}
-])
-
-Nx.Defn.default_options(compiler: EXLA)
-Nx.global_default_backend(EXLA.Backend)
-
-alias Explorer.{DataFrame, Series}
+Mix.install([
+ {:axon, "~> 0.3.0"},
+ {:nx, "~> 0.4.0", override: true},
+ {:exla, "~> 0.4.0"},
+ {:explorer, "~> 0.3.1"},
+ {:kino, "~> 0.7.0"}
+])
+
+Nx.Defn.default_options(compiler: EXLA)
+Nx.global_default_backend(EXLA.Backend)
+
+alias Explorer.{DataFrame, Series}
introduction
@@ -138,58 +138,58 @@
Data processing
-
The first step is to prepare the data for training and evaluation. Please download the dataset in the CSV format from https://www.kaggle.com/mlg-ulb/creditcardfraud (this requires a Kaggla account). Once done, put the file path in the input below.
data_path_input = Kino.Input.text("Data path (CSV)")
Now, let's read the data into an Explorer.Dataframe
:
data_path = Kino.Input.read(data_path_input)
+The first step is to prepare the data for training and evaluation. Please download the dataset in the CSV format from https://www.kaggle.com/mlg-ulb/creditcardfraud (this requires a Kaggla account). Once done, put the file path in the input below.
data_path_input = Kino.Input.text("Data path (CSV)")
Now, let's read the data into an Explorer.Dataframe
:
data_path = Kino.Input.read(data_path_input)
-df = DataFrame.from_csv!(data_path, dtypes: [{"Time", :float}])
For further processing, we will need a couple helper functions. We will group them in a module for convenience.
defmodule CredidCard.Data do
+df = DataFrame.from_csv!(data_path, dtypes: [{"Time", :float}])
For further processing, we will need a couple helper functions. We will group them in a module for convenience.
defmodule CredidCard.Data do
import Nx.Defn
- def split_train_test(df, portion) do
- num_examples = DataFrame.n_rows(df)
- num_train = ceil(portion * num_examples)
+ def split_train_test(df, portion) do
+ num_examples = DataFrame.n_rows(df)
+ num_train = ceil(portion * num_examples)
num_test = num_examples - num_train
- train = DataFrame.slice(df, 0, num_train)
- test = DataFrame.slice(df, num_train, num_test)
- {train, test}
- end
+ train = DataFrame.slice(df, 0, num_train)
+ test = DataFrame.slice(df, num_train, num_test)
+ {train, test}
+ end
- def split_features_targets(df) do
- features = DataFrame.select(df, &(&1 == "Class"), :drop)
- targets = DataFrame.select(df, &(&1 == "Class"), :keep)
- {features, targets}
- end
+ def split_features_targets(df) do
+ features = DataFrame.select(df, &(&1 == "Class"), :drop)
+ targets = DataFrame.select(df, &(&1 == "Class"), :keep)
+ {features, targets}
+ end
- def df_to_tensor(df) do
+ def df_to_tensor(df) do
df
- |> DataFrame.names()
- |> Enum.map(&Series.to_tensor(df[&1]))
- |> Nx.stack(axis: 1)
- end
+ |> DataFrame.names()
+ |> Enum.map(&Series.to_tensor(df[&1]))
+ |> Nx.stack(axis: 1)
+ end
- defn normalize_features(tensor) do
+ defn normalize_features(tensor) do
max =
tensor
- |> Nx.abs()
- |> Nx.reduce_max(axes: [0], keep_axes: true)
+ |> Nx.abs()
+ |> Nx.reduce_max(axes: [0], keep_axes: true)
tensor / max
- end
-end
With that, we can start converting the data into the desired format. First, we split the data into training and test data (in proportion 80% into a training set and 20% into a test set).
{train_df, test_df} = CredidCard.Data.split_train_test(df, 0.8)
-{DataFrame.n_rows(train_df), DataFrame.n_rows(test_df)}
Next, we separate features from labels and convert both to tensors. In case of features we additionally normalize each of them, dividing by the maximum absolute value of that feature.
{train_features, train_targets} = CredidCard.Data.split_features_targets(train_df)
-{test_features, test_targets} = CredidCard.Data.split_features_targets(test_df)
+ end
+end
With that, we can start converting the data into the desired format. First, we split the data into training and test data (in proportion 80% into a training set and 20% into a test set).
{train_df, test_df} = CredidCard.Data.split_train_test(df, 0.8)
+{DataFrame.n_rows(train_df), DataFrame.n_rows(test_df)}
Next, we separate features from labels and convert both to tensors. In case of features we additionally normalize each of them, dividing by the maximum absolute value of that feature.
{train_features, train_targets} = CredidCard.Data.split_features_targets(train_df)
+{test_features, test_targets} = CredidCard.Data.split_features_targets(test_df)
train_inputs =
train_features
- |> CredidCard.Data.df_to_tensor()
- |> CredidCard.Data.normalize_features()
+ |> CredidCard.Data.df_to_tensor()
+ |> CredidCard.Data.normalize_features()
test_inputs =
test_features
- |> CredidCard.Data.df_to_tensor()
- |> CredidCard.Data.normalize_features()
+ |> CredidCard.Data.df_to_tensor()
+ |> CredidCard.Data.normalize_features()
-train_targets = CredidCard.Data.df_to_tensor(train_targets)
-test_targets = CredidCard.Data.df_to_tensor(test_targets)
+train_targets = CredidCard.Data.df_to_tensor(train_targets)
+test_targets = CredidCard.Data.df_to_tensor(test_targets)
:ok
@@ -198,43 +198,43 @@
Building the model
Our model for predicting whether a transaction was fraudulent or not is a dense neural network. It consists of two dense layers with 256 neurons, ReLU activation functions, one dropout layer, and a dense layer with one neuron (since the problem is a binary prediction) followed by a sigmoid activation function.
model =
- Axon.input("input")
- |> Axon.dense(256)
- |> Axon.relu()
- |> Axon.dense(256)
- |> Axon.relu()
- |> Axon.dropout(rate: 0.3)
- |> Axon.dense(1)
- |> Axon.sigmoid()
+
Axon.input("input")
+ |> Axon.dense(256)
+ |> Axon.relu()
+ |> Axon.dense(256)
+ |> Axon.relu()
+ |> Axon.dropout(rate: 0.3)
+ |> Axon.dense(1)
+ |> Axon.sigmoid()
training-our-model
Training our model
-Now we have both data and model architecture prepared, it's time to train!
Note the disproportion in the data samples:
fraud = Nx.sum(train_targets) |> Nx.to_number()
-legit = Nx.size(train_targets) - fraud
+Now we have both data and model architecture prepared, it's time to train!
Note the disproportion in the data samples:
fraud = Nx.sum(train_targets) |> Nx.to_number()
+legit = Nx.size(train_targets) - fraud
-batched_train_inputs = Nx.to_batched(train_inputs, 2048)
-batched_train_targets = Nx.to_batched(train_targets, 2048)
-batched_train = Stream.zip(batched_train_inputs, batched_train_targets)
+batched_train_inputs = Nx.to_batched(train_inputs, 2048)
+batched_train_targets = Nx.to_batched(train_targets, 2048)
+batched_train = Stream.zip(batched_train_inputs, batched_train_targets)
-IO.puts("# of legit transactions (train): #{legit}")
-IO.puts("# of fraudulent transactions (train): #{fraud}")
-IO.puts("% fraudlent transactions (train): #{100 * (fraud / (legit + fraud))}%")
As always, we define our train loop. We are using binary cross-entropy as our loss function and Adam as the optimizer with a learning rate of 0.01. Then we immediately start the training passing our train portion of the dataset.
loss =
- &Axon.Losses.binary_cross_entropy(
+IO.puts("# of legit transactions (train): #{legit}")
+IO.puts("# of fraudulent transactions (train): #{fraud}")
+IO.puts("% fraudlent transactions (train): #{100 * (fraud / (legit + fraud))}%")
As always, we define our train loop. We are using binary cross-entropy as our loss function and Adam as the optimizer with a learning rate of 0.01. Then we immediately start the training passing our train portion of the dataset.
loss =
+ &Axon.Losses.binary_cross_entropy(
&1,
&2,
negative_weight: 1 / legit,
positive_weight: 1 / fraud,
reduction: :mean
- )
+ )
-optimizer = Axon.Optimizers.adam(1.0e-2)
+optimizer = Axon.Optimizers.adam(1.0e-2)
params =
model
- |> Axon.Loop.trainer(loss, optimizer)
- |> Axon.Loop.run(batched_train, %{}, epochs: 30, compiler: EXLA)
+ |> Axon.Loop.trainer(loss, optimizer)
+ |> Axon.Loop.run(batched_train, %{}, epochs: 30, compiler: EXLA)
:ok
@@ -242,39 +242,39 @@
Model evaluation
-After the training, there is only one thing left: testing. Here, we will focus on the number of true positive, true negative, false positive, and false negative values, but also on the likelihood of denying legit and fraudulent transactions.
batched_test_inputs = Nx.to_batched(test_inputs, 2048)
-batched_test_targets = Nx.to_batched(test_targets, 2048)
-batched_test = Stream.zip(batched_test_inputs, batched_test_targets)
-
-summarize = fn %Axon.Loop.State{metrics: metrics} = state ->
- legit_transactions_declined = Nx.to_number(metrics["fp"])
- legit_transactions_accepted = Nx.to_number(metrics["tn"])
- fraud_transactions_accepted = Nx.to_number(metrics["fn"])
- fraud_transactions_declined = Nx.to_number(metrics["tp"])
+After the training, there is only one thing left: testing. Here, we will focus on the number of true positive, true negative, false positive, and false negative values, but also on the likelihood of denying legit and fraudulent transactions.
batched_test_inputs = Nx.to_batched(test_inputs, 2048)
+batched_test_targets = Nx.to_batched(test_targets, 2048)
+batched_test = Stream.zip(batched_test_inputs, batched_test_targets)
+
+summarize = fn %Axon.Loop.State{metrics: metrics} = state ->
+ legit_transactions_declined = Nx.to_number(metrics["fp"])
+ legit_transactions_accepted = Nx.to_number(metrics["tn"])
+ fraud_transactions_accepted = Nx.to_number(metrics["fn"])
+ fraud_transactions_declined = Nx.to_number(metrics["tp"])
total_fraud = fraud_transactions_declined + fraud_transactions_accepted
total_legit = legit_transactions_declined + legit_transactions_accepted
- fraud_denial_percent = 100 * (fraud_transactions_declined / total_fraud)
- legit_denial_percent = 100 * (legit_transactions_declined / total_legit)
+ fraud_denial_percent = 100 * (fraud_transactions_declined / total_fraud)
+ legit_denial_percent = 100 * (legit_transactions_declined / total_legit)
- IO.write("\n")
- IO.puts("Legit Transactions Declined: #{legit_transactions_declined}")
- IO.puts("Fraudulent Transactions Caught: #{fraud_transactions_declined}")
- IO.puts("Fraudulent Transactions Missed: #{fraud_transactions_accepted}")
- IO.puts("Likelihood of catching fraud: #{fraud_denial_percent}%")
- IO.puts("Likelihood of denying legit transaction: #{legit_denial_percent}%")
+ IO.write("\n")
+ IO.puts("Legit Transactions Declined: #{legit_transactions_declined}")
+ IO.puts("Fraudulent Transactions Caught: #{fraud_transactions_declined}")
+ IO.puts("Fraudulent Transactions Missed: #{fraud_transactions_accepted}")
+ IO.puts("Likelihood of catching fraud: #{fraud_denial_percent}%")
+ IO.puts("Likelihood of denying legit transaction: #{legit_denial_percent}%")
- {:continue, state}
-end
+ {:continue, state}
+end
model
-|> Axon.Loop.evaluator()
-|> Axon.Loop.metric(:true_positives, "tp", :running_sum)
-|> Axon.Loop.metric(:true_negatives, "tn", :running_sum)
-|> Axon.Loop.metric(:false_positives, "fp", :running_sum)
-|> Axon.Loop.metric(:false_negatives, "fn", :running_sum)
-|> Axon.Loop.handle(:epoch_completed, summarize)
-|> Axon.Loop.run(batched_test, params, compiler: EXLA)
+|> Axon.Loop.evaluator()
+|> Axon.Loop.metric(:true_positives, "tp", :running_sum)
+|> Axon.Loop.metric(:true_negatives, "tn", :running_sum)
+|> Axon.Loop.metric(:false_positives, "fp", :running_sum)
+|> Axon.Loop.metric(:false_negatives, "fn", :running_sum)
+|> Axon.Loop.handle(:epoch_completed, summarize)
+|> Axon.Loop.run(batched_test, params, compiler: EXLA)
:ok
diff --git a/custom_layers.html b/custom_layers.html
index 1f5f555b..b506a69a 100644
--- a/custom_layers.html
+++ b/custom_layers.html
@@ -115,104 +115,104 @@
-Mix.install([
- {:axon, github: "elixir-nx/axon"},
- {:nx, "~> 0.3.0", github: "elixir-nx/nx", sparse: "nx", override: true},
- {:kino, "~> 0.7.0"}
-])
:ok
+Mix.install([
+ {:axon, github: "elixir-nx/axon"},
+ {:nx, "~> 0.3.0", github: "elixir-nx/nx", sparse: "nx", override: true},
+ {:kino, "~> 0.7.0"}
+])
:ok
creating-custom-layers
Creating custom layers
-
While Axon has a plethora of built-in layers, more than likely you'll run into a case where you need something not provided by the framework. In these instances, you can use custom layers.
To Axon, layers are really just defn
implementations with special Axon inputs. Every layer in Axon (including the built-in layers), are implemented with the Axon.layer/3
function. The API of Axon.layer/3
intentionally mirrors the API of Kernel.apply/2
. To declare a custom layer you need 2 things:
- A
defn
implementation - Inputs
The defn
implementation looks like any other defn
you'd write; however, it must always account for additional opts
as an argument:
defmodule CustomLayers do
+While Axon has a plethora of built-in layers, more than likely you'll run into a case where you need something not provided by the framework. In these instances, you can use custom layers.
To Axon, layers are really just defn
implementations with special Axon inputs. Every layer in Axon (including the built-in layers), are implemented with the Axon.layer/3
function. The API of Axon.layer/3
intentionally mirrors the API of Kernel.apply/2
. To declare a custom layer you need 2 things:
- A
defn
implementation - Inputs
The defn
implementation looks like any other defn
you'd write; however, it must always account for additional opts
as an argument:
defmodule CustomLayers do
import Nx.Defn
- defn my_layer(input, opts \\ []) do
- opts = keyword!(opts, mode: :train, alpha: 1.0)
+ defn my_layer(input, opts \\ []) do
+ opts = keyword!(opts, mode: :train, alpha: 1.0)
input
- |> Nx.sin()
- |> Nx.multiply(opts[:alpha])
- end
-end
{:module, CustomLayers, <<70, 79, 82, 49, 0, 0, 11, ...>>, {:my_layer, 2}}
Regardless of the options you configure your layer to accept, the defn
implementation will always receive a :mode
option indicating whether or not the model is running in training or inference mode. You can customize the behavior of your layer depending on the mode.
With an implementation defined, you need only to call Axon.layer/3
to apply our custom layer to an Axon input:
input = Axon.input("data")
+ |> Nx.sin()
+ |> Nx.multiply(opts[:alpha])
+ end
+end
{:module, CustomLayers, <<70, 79, 82, 49, 0, 0, 11, ...>>, {:my_layer, 2}}
Regardless of the options you configure your layer to accept, the defn
implementation will always receive a :mode
option indicating whether or not the model is running in training or inference mode. You can customize the behavior of your layer depending on the mode.
With an implementation defined, you need only to call Axon.layer/3
to apply our custom layer to an Axon input:
input = Axon.input("data")
-out = Axon.layer(&CustomLayers.my_layer/2, [input])
#Axon<
- inputs: %{"data" => nil}
+out = Axon.layer(&CustomLayers.my_layer/2, [input])
#Axon<
+ inputs: %{"data" => nil}
outputs: "custom_0"
nodes: 2
->
Now you can inspect and execute your model as normal:
template = Nx.template({2, 8}, :f32)
-Axon.Display.as_graph(out, template)
graph TD;
+
>
Now you can inspect and execute your model as normal:
template = Nx.template({2, 8}, :f32)
+Axon.Display.as_graph(out, template)
graph TD;
3[/"data (:input) {2, 8}"/];
4["custom_0 (:custom) {2, 8}"];
-3 --> 4;
Notice that by default custom layers render with a default operation marked as :custom
. This can make it difficult to determine which layer is which during inspection. You can control the rendering by passing :op_name
to Axon.layer/3
:
out = Axon.layer(&CustomLayers.my_layer/2, [input], op_name: :my_layer)
+3 --> 4;
Notice that by default custom layers render with a default operation marked as :custom
. This can make it difficult to determine which layer is which during inspection. You can control the rendering by passing :op_name
to Axon.layer/3
:
out = Axon.layer(&CustomLayers.my_layer/2, [input], op_name: :my_layer)
-Axon.Display.as_graph(out, template)
graph TD;
+
Axon.Display.as_graph(out, template)
graph TD;
3[/"data (:input) {2, 8}"/];
5["my_layer_0 (:my_layer) {2, 8}"];
3 --> 5;
You can also control the name of your layer via the :name
option. All other options are forwarded to the layer implementation function:
out =
- Axon.layer(&CustomLayers.my_layer/2, [input],
+ Axon.layer(&CustomLayers.my_layer/2, [input],
name: "layer",
op_name: :my_layer,
alpha: 2.0
- )
+ )
-Axon.Display.as_graph(out, template)
graph TD;
+
Axon.Display.as_graph(out, template)
graph TD;
3[/"data (:input) {2, 8}"/];
6["layer (:my_layer) {2, 8}"];
-3 --> 6;
{init_fn, predict_fn} = Axon.build(out)
-params = init_fn.(template, %{})
%{}
predict_fn.(params, Nx.iota({2, 8}, type: :f32))
#Nx.Tensor<
- f32[2][8]
- [
- [0.0, 1.6829419136047363, 1.8185948133468628, 0.28224000334739685, -1.513604998588562, -1.9178485870361328, -0.558830976486206, 1.3139731884002686],
- [1.978716492652893, 0.8242369890213013, -1.0880422592163086, -1.9999804496765137, -1.073145866394043, 0.8403340578079224, 1.9812147617340088, 1.3005757331848145]
- ]
->
Notice that this model does not have any trainable parameters because none of the layers have trainable parameters. You can introduce trainable parameters by passing inputs created with Axon.param/3
to Axon.layer/3
. For example, you can modify your original custom layer to take an additional trainable parameter:
defmodule CustomLayers do
+3 --> 6;
{init_fn, predict_fn} = Axon.build(out)
+params = init_fn.(template, %{})
%{}
predict_fn.(params, Nx.iota({2, 8}, type: :f32))
#Nx.Tensor<
+ f32[2][8]
+ [
+ [0.0, 1.6829419136047363, 1.8185948133468628, 0.28224000334739685, -1.513604998588562, -1.9178485870361328, -0.558830976486206, 1.3139731884002686],
+ [1.978716492652893, 0.8242369890213013, -1.0880422592163086, -1.9999804496765137, -1.073145866394043, 0.8403340578079224, 1.9812147617340088, 1.3005757331848145]
+ ]
+>
Notice that this model does not have any trainable parameters because none of the layers have trainable parameters. You can introduce trainable parameters by passing inputs created with Axon.param/3
to Axon.layer/3
. For example, you can modify your original custom layer to take an additional trainable parameter:
defmodule CustomLayers do
import Nx.Defn
- defn my_layer(input, alpha, _opts \\ []) do
+ defn my_layer(input, alpha, _opts \\ []) do
input
- |> Nx.sin()
- |> Nx.multiply(alpha)
- end
-end
{:module, CustomLayers, <<70, 79, 82, 49, 0, 0, 11, ...>>, {:my_layer, 3}}
And then construct the layer with a regular Axon input and a trainable parameter:
alpha = Axon.param("alpha", fn _ -> {} end)
+ |> Nx.sin()
+ |> Nx.multiply(alpha)
+ end
+end
{:module, CustomLayers, <<70, 79, 82, 49, 0, 0, 11, ...>>, {:my_layer, 3}}
And then construct the layer with a regular Axon input and a trainable parameter:
alpha = Axon.param("alpha", fn _ -> {} end)
-out = Axon.layer(&CustomLayers.my_layer/3, [input, alpha], op_name: :my_layer)
#Axon<
- inputs: %{"data" => nil}
+out = Axon.layer(&CustomLayers.my_layer/3, [input, alpha], op_name: :my_layer)
#Axon<
+ inputs: %{"data" => nil}
outputs: "my_layer_0"
nodes: 2
->
{init_fn, predict_fn} = Axon.build(out)
-params = init_fn.(template, %{})
%{
- "my_layer_0" => %{
- "alpha" => #Nx.Tensor<
+>
{init_fn, predict_fn} = Axon.build(out)
+params = init_fn.(template, %{})
%{
+ "my_layer_0" => %{
+ "alpha" => #Nx.Tensor<
f32
1.194254994392395
- >
- }
-}
Notice how your model now initializes with a trainable parameter "alpha"
for your custom layer. Each parameter requires a unique (per-layer) string name and a function which determines the parameter's shape from the layer's input shapes.
If you plan on re-using custom layers in many locations, it's recommended that you wrap them in an Elixir function as an interface:
defmodule CustomLayers do
+ >
+ }
+}
Notice how your model now initializes with a trainable parameter "alpha"
for your custom layer. Each parameter requires a unique (per-layer) string name and a function which determines the parameter's shape from the layer's input shapes.
If you plan on re-using custom layers in many locations, it's recommended that you wrap them in an Elixir function as an interface:
defmodule CustomLayers do
import Nx.Defn
- def my_layer(%Axon{} = input, opts \\ []) do
- opts = Keyword.validate!(opts, [:name])
- alpha = Axon.param("alpha", fn _ -> {} end)
+ def my_layer(%Axon{} = input, opts \\ []) do
+ opts = Keyword.validate!(opts, [:name])
+ alpha = Axon.param("alpha", fn _ -> {} end)
- Axon.layer(&my_layer_impl/3, [input, alpha], name: opts[:name], op_name: :my_layer)
- end
+ Axon.layer(&my_layer_impl/3, [input, alpha], name: opts[:name], op_name: :my_layer)
+ end
- defnp my_layer_impl(input, alpha, _opts \\ []) do
+ defnp my_layer_impl(input, alpha, _opts \\ []) do
input
- |> Nx.sin()
- |> Nx.multiply(alpha)
- end
-end
{:module, CustomLayers, <<70, 79, 82, 49, 0, 0, 13, ...>>, {:my_layer_impl, 3}}
out =
+ |> Nx.sin()
+ |> Nx.multiply(alpha)
+ end
+end
{:module, CustomLayers, <<70, 79, 82, 49, 0, 0, 13, ...>>, {:my_layer_impl, 3}}
out =
input
- |> CustomLayers.my_layer()
- |> CustomLayers.my_layer()
- |> Axon.dense(1)
#Axon<
- inputs: %{"data" => nil}
+ |> CustomLayers.my_layer()
+ |> CustomLayers.my_layer()
+ |> Axon.dense(1)
#Axon<
+ inputs: %{"data" => nil}
outputs: "dense_0"
nodes: 4
->
Axon.Display.as_graph(out, template)
graph TD;
+>
Axon.Display.as_graph(out, template)
graph TD;
3[/"data (:input) {2, 8}"/];
10["my_layer_0 (:my_layer) {2, 8}"];
12["my_layer_1 (:my_layer) {2, 8}"];
diff --git a/custom_models_loss_optimizers.html b/custom_models_loss_optimizers.html
index 9d7e9b0e..a326757f 100644
--- a/custom_models_loss_optimizers.html
+++ b/custom_models_loss_optimizers.html
@@ -115,320 +115,320 @@
-Mix.install([
- {:axon, github: "elixir-nx/axon"},
- {:nx, "~> 0.3.0", github: "elixir-nx/nx", sparse: "nx", override: true}
-])
:ok
+Mix.install([
+ {:axon, github: "elixir-nx/axon"},
+ {:nx, "~> 0.3.0", github: "elixir-nx/nx", sparse: "nx", override: true}
+])
:ok
using-custom-models-in-training-loops
Using custom models in training loops
In the Your first training loop, you learned how to declare a supervised training loop using Axon.Loop.trainer/3
with a model, loss function, and optimizer. Your overall model and loop declaration looked something like this:
model =
- Axon.input("data")
- |> Axon.dense(8)
- |> Axon.relu()
- |> Axon.dense(4)
- |> Axon.relu()
- |> Axon.dense(1)
-
-loop = Axon.Loop.trainer(model, :mean_squared_error, :sgd)
This example uses an %Axon{}
struct to represent your model
to train, and atoms to represent your loss function and optimizer. Some of your problems will require a bit more flexibility than this example affords. Fortunately, Axon.Loop.trainer/3
is designed for flexibility.
For example, if your model cannot be cleanly represented as an %Axon{}
model, you can instead opt instead to define custom initialization and forward functions to pass to Axon.Loop.trainer/3
. Actually, Axon.Loop.trainer/3
is doing this for you under the hood - the ability to pass an %Axon{}
struct directly is just a convenience:
model =
- Axon.input("data")
- |> Axon.dense(8)
- |> Axon.relu()
- |> Axon.dense(4)
- |> Axon.relu()
- |> Axon.dense(1)
-
-lowered_model = {init_fn, predict_fn} = Axon.build(model)
-
-loop = Axon.Loop.trainer(lowered_model, :mean_squared_error, :sgd)
#Axon.Loop<
- handlers: %{
- completed: [],
- epoch_completed: [
- {#Function<23.20267452/1 in Axon.Loop.log/5>,
- #Function<5.20267452/1 in Axon.Loop.build_filter_fn/1>}
- ],
- epoch_halted: [],
- epoch_started: [],
- halted: [],
- iteration_completed: [
- {#Function<23.20267452/1 in Axon.Loop.log/5>,
- #Function<3.20267452/1 in Axon.Loop.build_filter_fn/1>}
- ],
- iteration_started: [],
- started: []
- },
- metrics: %{
- "loss" => {#Function<12.6031754/3 in Axon.Metrics.running_average/1>,
- #Function<6.20267452/2 in Axon.Loop.build_loss_fn/1>}
- },
+ Axon.input("data")
+ |> Axon.dense(8)
+ |> Axon.relu()
+ |> Axon.dense(4)
+ |> Axon.relu()
+ |> Axon.dense(1)
+
+loop = Axon.Loop.trainer(model, :mean_squared_error, :sgd)
This example uses an %Axon{}
struct to represent your model
to train, and atoms to represent your loss function and optimizer. Some of your problems will require a bit more flexibility than this example affords. Fortunately, Axon.Loop.trainer/3
is designed for flexibility.
For example, if your model cannot be cleanly represented as an %Axon{}
model, you can instead opt instead to define custom initialization and forward functions to pass to Axon.Loop.trainer/3
. Actually, Axon.Loop.trainer/3
is doing this for you under the hood - the ability to pass an %Axon{}
struct directly is just a convenience:
model =
+ Axon.input("data")
+ |> Axon.dense(8)
+ |> Axon.relu()
+ |> Axon.dense(4)
+ |> Axon.relu()
+ |> Axon.dense(1)
+
+lowered_model = {init_fn, predict_fn} = Axon.build(model)
+
+loop = Axon.Loop.trainer(lowered_model, :mean_squared_error, :sgd)
#Axon.Loop<
+ handlers: %{
+ completed: [],
+ epoch_completed: [
+ {#Function<23.20267452/1 in Axon.Loop.log/5>,
+ #Function<5.20267452/1 in Axon.Loop.build_filter_fn/1>}
+ ],
+ epoch_halted: [],
+ epoch_started: [],
+ halted: [],
+ iteration_completed: [
+ {#Function<23.20267452/1 in Axon.Loop.log/5>,
+ #Function<3.20267452/1 in Axon.Loop.build_filter_fn/1>}
+ ],
+ iteration_started: [],
+ started: []
+ },
+ metrics: %{
+ "loss" => {#Function<12.6031754/3 in Axon.Metrics.running_average/1>,
+ #Function<6.20267452/2 in Axon.Loop.build_loss_fn/1>}
+ },
...
->
Notice that Axon.Loop.trainer/3
handles the "lowered" form of an Axon model without issue. When you pass an %Axon{}
struct, the trainer factory converts it to a lowered representation for you. With this construct, you can build custom models entirely with Nx defn
, or readily mix your Axon models into custom workflows without worrying about compatibility with the Axon.Loop
API:
defmodule CustomModel do
+>
Notice that Axon.Loop.trainer/3
handles the "lowered" form of an Axon model without issue. When you pass an %Axon{}
struct, the trainer factory converts it to a lowered representation for you. With this construct, you can build custom models entirely with Nx defn
, or readily mix your Axon models into custom workflows without worrying about compatibility with the Axon.Loop
API:
defmodule CustomModel do
import Nx.Defn
- defn custom_predict_fn(model_predict_fn, params, input) do
- %{prediction: preds} = out = model_predict_fn.(params, input)
- %{out | prediction: Nx.cos(preds)}
- end
-end
{:module, CustomModel, <<70, 79, 82, 49, 0, 0, 9, ...>>, {:custom_predict_fn, 3}}
train_data =
- Stream.repeatedly(fn ->
- xs = Nx.random_normal({8, 1})
- ys = Nx.sin(xs)
- {xs, ys}
- end)
-
-{init_fn, predict_fn} = Axon.build(model, mode: :train)
-custom_predict_fn = &CustomModel.custom_predict_fn(predict_fn, &1, &2)
-
-loop = Axon.Loop.trainer({init_fn, custom_predict_fn}, :mean_squared_error, :sgd)
-
-Axon.Loop.run(loop, train_data, %{}, iterations: 500)
Epoch: 0, Batch: 500, loss: 0.3053460
%{
- "dense_0" => %{
- "bias" => #Nx.Tensor<
- f32[8]
- [-0.06573846191167831, 0.37533989548683167, -0.014221129938960075, -0.0056641618721187115, -0.013241665437817574, -0.04930500313639641, 0.03238297998905182, 0.019304191693663597]
- >,
- "kernel" => #Nx.Tensor<
- f32[1][8]
- [
- [-0.3132522702217102, -0.9284062385559082, 0.5041953921318054, 0.09051526337862015, 0.003381401300430298, -0.22686156630516052, 0.506594181060791, 0.46744370460510254]
- ]
- >
- },
- "dense_1" => %{
- "bias" => #Nx.Tensor<
- f32[4]
- [0.008441010490059853, 0.0, 0.5370790958404541, 0.03584281727671623]
- >,
- "kernel" => #Nx.Tensor<
- f32[8][4]
- [
- [-0.3442431688308716, -0.33131587505340576, -0.03751888871192932, -0.5497395396232605],
- [-0.4568001925945282, -0.5024663805961609, 0.8712142109870911, -0.13484779000282288],
- [0.7310590744018555, -0.34318023920059204, 0.3977772295475006, -0.6045383214950562],
- [-0.5255699157714844, -0.2829623818397522, -0.45367464423179626, -0.157784566283226],
- [-0.47948920726776123, 0.2930692136287689, -0.3784458339214325, -0.69244384765625],
- [0.7052943706512451, 0.015830136835575104, -0.02979498915374279, 0.6160839796066284],
- [0.3201732933521271, -0.1367085874080658, -0.17100055515766144, 0.7335636019706726],
- [-0.2825513482093811, -0.424674928188324, -0.3110836148262024, 0.46001508831977844]
- ]
- >
- },
- "dense_2" => %{
- "bias" => #Nx.Tensor<
- f32[1]
- [0.6889857649803162]
- >,
- "kernel" => #Nx.Tensor<
- f32[4][1]
- [
- [-0.7191283106803894],
- [-0.4222411513328552],
- [1.122635006904602],
- [-0.7385509014129639]
- ]
- >
- }
-}
+ defn custom_predict_fn(model_predict_fn, params, input) do
+ %{prediction: preds} = out = model_predict_fn.(params, input)
+ %{out | prediction: Nx.cos(preds)}
+ end
+end
{:module, CustomModel, <<70, 79, 82, 49, 0, 0, 9, ...>>, {:custom_predict_fn, 3}}
train_data =
+ Stream.repeatedly(fn ->
+ xs = Nx.random_normal({8, 1})
+ ys = Nx.sin(xs)
+ {xs, ys}
+ end)
+
+{init_fn, predict_fn} = Axon.build(model, mode: :train)
+custom_predict_fn = &CustomModel.custom_predict_fn(predict_fn, &1, &2)
+
+loop = Axon.Loop.trainer({init_fn, custom_predict_fn}, :mean_squared_error, :sgd)
+
+Axon.Loop.run(loop, train_data, %{}, iterations: 500)
Epoch: 0, Batch: 500, loss: 0.3053460
%{
+ "dense_0" => %{
+ "bias" => #Nx.Tensor<
+ f32[8]
+ [-0.06573846191167831, 0.37533989548683167, -0.014221129938960075, -0.0056641618721187115, -0.013241665437817574, -0.04930500313639641, 0.03238297998905182, 0.019304191693663597]
+ >,
+ "kernel" => #Nx.Tensor<
+ f32[1][8]
+ [
+ [-0.3132522702217102, -0.9284062385559082, 0.5041953921318054, 0.09051526337862015, 0.003381401300430298, -0.22686156630516052, 0.506594181060791, 0.46744370460510254]
+ ]
+ >
+ },
+ "dense_1" => %{
+ "bias" => #Nx.Tensor<
+ f32[4]
+ [0.008441010490059853, 0.0, 0.5370790958404541, 0.03584281727671623]
+ >,
+ "kernel" => #Nx.Tensor<
+ f32[8][4]
+ [
+ [-0.3442431688308716, -0.33131587505340576, -0.03751888871192932, -0.5497395396232605],
+ [-0.4568001925945282, -0.5024663805961609, 0.8712142109870911, -0.13484779000282288],
+ [0.7310590744018555, -0.34318023920059204, 0.3977772295475006, -0.6045383214950562],
+ [-0.5255699157714844, -0.2829623818397522, -0.45367464423179626, -0.157784566283226],
+ [-0.47948920726776123, 0.2930692136287689, -0.3784458339214325, -0.69244384765625],
+ [0.7052943706512451, 0.015830136835575104, -0.02979498915374279, 0.6160839796066284],
+ [0.3201732933521271, -0.1367085874080658, -0.17100055515766144, 0.7335636019706726],
+ [-0.2825513482093811, -0.424674928188324, -0.3110836148262024, 0.46001508831977844]
+ ]
+ >
+ },
+ "dense_2" => %{
+ "bias" => #Nx.Tensor<
+ f32[1]
+ [0.6889857649803162]
+ >,
+ "kernel" => #Nx.Tensor<
+ f32[4][1]
+ [
+ [-0.7191283106803894],
+ [-0.4222411513328552],
+ [1.122635006904602],
+ [-0.7385509014129639]
+ ]
+ >
+ }
+}
using-custom-loss-functions-in-training-loops
Using custom loss functions in training loops
-Just as Axon.Loop.trainer/3
allows more flexibility with models, it also supports more flexible loss functions. In most cases, you can get away with using one of Axon's built-in loss functions by specifying an atom. Atoms map directly to a loss-function defined in Axon.Losses
. Under the hood, Axon.Loop.trainer/3
is doing something like:
loss_fn = &apply(Axon.Losses, loss_atom, [&1, &2])
Rather than pass an atom, you can pass your own custom arity-2 function to Axon.Loop.trainer/3
. This arises most often in cases where you want to control some parameters of the loss function, such as the batch-level reduction:
loss_fn = &Axon.Losses.mean_squared_error(&1, &2, reduction: :sum)
-
-loop = Axon.Loop.trainer(model, loss_fn, :sgd)
#Axon.Loop<
- handlers: %{
- completed: [],
- epoch_completed: [
- {#Function<23.20267452/1 in Axon.Loop.log/5>,
- #Function<5.20267452/1 in Axon.Loop.build_filter_fn/1>}
- ],
- epoch_halted: [],
- epoch_started: [],
- halted: [],
- iteration_completed: [
- {#Function<23.20267452/1 in Axon.Loop.log/5>,
- #Function<3.20267452/1 in Axon.Loop.build_filter_fn/1>}
- ],
- iteration_started: [],
- started: []
- },
- metrics: %{
- "loss" => {#Function<12.6031754/3 in Axon.Metrics.running_average/1>,
- #Function<41.3316493/2 in :erl_eval.expr/6>}
- },
+Just as Axon.Loop.trainer/3
allows more flexibility with models, it also supports more flexible loss functions. In most cases, you can get away with using one of Axon's built-in loss functions by specifying an atom. Atoms map directly to a loss-function defined in Axon.Losses
. Under the hood, Axon.Loop.trainer/3
is doing something like:
loss_fn = &apply(Axon.Losses, loss_atom, [&1, &2])
Rather than pass an atom, you can pass your own custom arity-2 function to Axon.Loop.trainer/3
. This arises most often in cases where you want to control some parameters of the loss function, such as the batch-level reduction:
loss_fn = &Axon.Losses.mean_squared_error(&1, &2, reduction: :sum)
+
+loop = Axon.Loop.trainer(model, loss_fn, :sgd)
#Axon.Loop<
+ handlers: %{
+ completed: [],
+ epoch_completed: [
+ {#Function<23.20267452/1 in Axon.Loop.log/5>,
+ #Function<5.20267452/1 in Axon.Loop.build_filter_fn/1>}
+ ],
+ epoch_halted: [],
+ epoch_started: [],
+ halted: [],
+ iteration_completed: [
+ {#Function<23.20267452/1 in Axon.Loop.log/5>,
+ #Function<3.20267452/1 in Axon.Loop.build_filter_fn/1>}
+ ],
+ iteration_started: [],
+ started: []
+ },
+ metrics: %{
+ "loss" => {#Function<12.6031754/3 in Axon.Metrics.running_average/1>,
+ #Function<41.3316493/2 in :erl_eval.expr/6>}
+ },
...
->
You can also define your own custom loss functions, so long as they match the following spec:
loss(
- y_true :: tensor[batch, ...] | container(tensor),
- y_preds :: tensor[batch, ...] | container(tensor)
- ) :: scalar
This is useful for constructing loss functions when dealing with multi-output scenarios. For example, it's very easy to construct a custom loss function which is a weighted average of several loss functions on multiple inputs:
train_data =
- Stream.repeatedly(fn ->
- xs = Nx.random_normal({8, 1})
- y1 = Nx.sin(xs)
- y2 = Nx.cos(xs)
- {xs, {y1, y2}}
- end)
+>
You can also define your own custom loss functions, so long as they match the following spec:
loss(
+ y_true :: tensor[batch, ...] | container(tensor),
+ y_preds :: tensor[batch, ...] | container(tensor)
+ ) :: scalar
This is useful for constructing loss functions when dealing with multi-output scenarios. For example, it's very easy to construct a custom loss function which is a weighted average of several loss functions on multiple inputs:
train_data =
+ Stream.repeatedly(fn ->
+ xs = Nx.random_normal({8, 1})
+ y1 = Nx.sin(xs)
+ y2 = Nx.cos(xs)
+ {xs, {y1, y2}}
+ end)
shared =
- Axon.input("data")
- |> Axon.dense(8)
- |> Axon.relu()
- |> Axon.dense(4)
- |> Axon.relu()
+ Axon.input("data")
+ |> Axon.dense(8)
+ |> Axon.relu()
+ |> Axon.dense(4)
+ |> Axon.relu()
-y1 = Axon.dense(shared, 1)
-y2 = Axon.dense(shared, 1)
+y1 = Axon.dense(shared, 1)
+y2 = Axon.dense(shared, 1)
-model = Axon.container({y1, y2})
+model = Axon.container({y1, y2})
-custom_loss_fn = fn {y_true1, y_true2}, {y_pred1, y_pred2} ->
- loss1 = Axon.Losses.mean_squared_error(y_true1, y_pred1, reduction: :mean)
- loss2 = Axon.Losses.mean_squared_error(y_true2, y_pred2, reduction: :mean)
+custom_loss_fn = fn {y_true1, y_true2}, {y_pred1, y_pred2} ->
+ loss1 = Axon.Losses.mean_squared_error(y_true1, y_pred1, reduction: :mean)
+ loss2 = Axon.Losses.mean_squared_error(y_true2, y_pred2, reduction: :mean)
loss1
- |> Nx.multiply(0.4)
- |> Nx.add(Nx.multiply(loss2, 0.6))
-end
+ |> Nx.multiply(0.4)
+ |> Nx.add(Nx.multiply(loss2, 0.6))
+end
model
-|> Axon.Loop.trainer(custom_loss_fn, :sgd)
-|> Axon.Loop.run(train_data, %{}, iterations: 1000)
Epoch: 0, Batch: 1000, loss: 0.1098235
%{
- "dense_0" => %{
- "bias" => #Nx.Tensor<
- f32[8]
- [0.07738334685564041, 0.04548311233520508, 0.049238916486501694, 0.38714033365249634, -0.030310271307826042, -0.07575170695781708, 0.02918776497244835, 0.15639683604240417]
- >,
- "kernel" => #Nx.Tensor<
- f32[1][8]
- [
- [-0.5250527858734131, 0.9252119660377502, -0.7720071077346802, 0.3685735762119293, -0.15688209235668182, -0.41163918375968933, 0.7827479839324951, 0.07295594364404678]
- ]
- >
- },
- "dense_1" => %{
- "bias" => #Nx.Tensor<
- f32[4]
- [0.012770675122737885, 0.6008449792861938, 0.29370757937431335, -0.05354489013552666]
- >,
- "kernel" => #Nx.Tensor<
- f32[8][4]
- [
- [-0.08783119916915894, 0.4296257495880127, 0.07153885811567307, -0.6921477317810059],
- [0.15848888456821442, -0.4663836658000946, 0.7126847505569458, 0.0693722814321518],
- [-0.24852830171585083, -0.7588720321655273, -0.5033655166625977, 0.6524038314819336],
- [0.2933746874332428, 0.6656989455223083, -0.046741705387830734, 0.44998466968536377],
- [0.17215801775455475, -0.3072860836982727, 0.2046997845172882, -0.7001357078552246],
- [0.6354788541793823, -0.12706635892391205, -0.18666459619998932, -0.26693975925445557],
- [-0.3737913966178894, -0.07344938814640045, 0.22658668458461761, -0.37110695242881775],
- [0.01989569514989853, 0.39410898089408875, -0.30496707558631897, -0.4945743680000305]
- ]
- >
- },
- "dense_2" => %{
- "bias" => #Nx.Tensor<
- f32[1]
- [-0.5888826251029968]
- >,
- "kernel" => #Nx.Tensor<
- f32[4][1]
- [
- [1.0239059925079346],
- [0.25252565741539],
- [0.8877795338630676],
- [-0.13882321119308472]
- ]
- >
- },
- "dense_3" => %{
- "bias" => #Nx.Tensor<
- f32[1]
- [0.2557465434074402]
- >,
- "kernel" => #Nx.Tensor<
- f32[4][1]
- [
- [-0.6269392371177673],
- [1.1281259059906006],
- [-0.503214418888092],
- [-0.5435869693756104]
- ]
- >
- }
-}
+
|> Axon.Loop.trainer(custom_loss_fn, :sgd)
+|> Axon.Loop.run(train_data, %{}, iterations: 1000)
Epoch: 0, Batch: 1000, loss: 0.1098235
%{
+ "dense_0" => %{
+ "bias" => #Nx.Tensor<
+ f32[8]
+ [0.07738334685564041, 0.04548311233520508, 0.049238916486501694, 0.38714033365249634, -0.030310271307826042, -0.07575170695781708, 0.02918776497244835, 0.15639683604240417]
+ >,
+ "kernel" => #Nx.Tensor<
+ f32[1][8]
+ [
+ [-0.5250527858734131, 0.9252119660377502, -0.7720071077346802, 0.3685735762119293, -0.15688209235668182, -0.41163918375968933, 0.7827479839324951, 0.07295594364404678]
+ ]
+ >
+ },
+ "dense_1" => %{
+ "bias" => #Nx.Tensor<
+ f32[4]
+ [0.012770675122737885, 0.6008449792861938, 0.29370757937431335, -0.05354489013552666]
+ >,
+ "kernel" => #Nx.Tensor<
+ f32[8][4]
+ [
+ [-0.08783119916915894, 0.4296257495880127, 0.07153885811567307, -0.6921477317810059],
+ [0.15848888456821442, -0.4663836658000946, 0.7126847505569458, 0.0693722814321518],
+ [-0.24852830171585083, -0.7588720321655273, -0.5033655166625977, 0.6524038314819336],
+ [0.2933746874332428, 0.6656989455223083, -0.046741705387830734, 0.44998466968536377],
+ [0.17215801775455475, -0.3072860836982727, 0.2046997845172882, -0.7001357078552246],
+ [0.6354788541793823, -0.12706635892391205, -0.18666459619998932, -0.26693975925445557],
+ [-0.3737913966178894, -0.07344938814640045, 0.22658668458461761, -0.37110695242881775],
+ [0.01989569514989853, 0.39410898089408875, -0.30496707558631897, -0.4945743680000305]
+ ]
+ >
+ },
+ "dense_2" => %{
+ "bias" => #Nx.Tensor<
+ f32[1]
+ [-0.5888826251029968]
+ >,
+ "kernel" => #Nx.Tensor<
+ f32[4][1]
+ [
+ [1.0239059925079346],
+ [0.25252565741539],
+ [0.8877795338630676],
+ [-0.13882321119308472]
+ ]
+ >
+ },
+ "dense_3" => %{
+ "bias" => #Nx.Tensor<
+ f32[1]
+ [0.2557465434074402]
+ >,
+ "kernel" => #Nx.Tensor<
+ f32[4][1]
+ [
+ [-0.6269392371177673],
+ [1.1281259059906006],
+ [-0.503214418888092],
+ [-0.5435869693756104]
+ ]
+ >
+ }
+}
using-custom-optimizers-in-training-loops
Using custom optimizers in training loops
As you might expect, it's also possible to customize the optimizer passed to Axon.Loop.trainer/3
. If you read the Axon.Updates
documentation, you'll learn that optimizers are actually represented as the tuple {init_fn, update_fn}
where init_fn
initializes optimizer state from model state and update_fn
scales gradients from optimizer state, gradients, and model state.
You likely won't have to implement a custom optimizer; however, you should know how to construct optimizers with different hyperparameters and how to apply different modifiers to different optimizers to customize the optimization process.
When you specify an optimizer as an atom in Axon.Loop.trainer/3
, it maps directly to an optimizer declared in Axon.Optimizers
. You can instead opt to declare your optimizer directly. This is most useful for controlling things like the learning rate and various optimizer hyperparameters:
train_data =
- Stream.repeatedly(fn ->
- xs = Nx.random_normal({8, 1})
- ys = Nx.sin(xs)
- {xs, ys}
- end)
+ Stream.repeatedly(fn ->
+ xs = Nx.random_normal({8, 1})
+ ys = Nx.sin(xs)
+ {xs, ys}
+ end)
model =
- Axon.input("data")
- |> Axon.dense(8)
- |> Axon.relu()
- |> Axon.dense(4)
- |> Axon.relu()
- |> Axon.dense(1)
+ Axon.input("data")
+ |> Axon.dense(8)
+ |> Axon.relu()
+ |> Axon.dense(4)
+ |> Axon.relu()
+ |> Axon.dense(1)
-optimizer = {_init_optimizer_fn, _update_fn} = Axon.Optimizers.sgd(1.0e-3)
+optimizer = {_init_optimizer_fn, _update_fn} = Axon.Optimizers.sgd(1.0e-3)
model
-|> Axon.Loop.trainer(:mean_squared_error, optimizer)
-|> Axon.Loop.run(train_data, %{}, iterations: 1000)
Epoch: 0, Batch: 1000, loss: 0.0992607
%{
- "dense_0" => %{
- "bias" => #Nx.Tensor<
- f32[8]
- [0.06136200204491615, -0.08278193324804306, -0.07280997931957245, 0.08740464597940445, 0.08663233369588852, -0.06915996968746185, 0.03753892332315445, 0.06512840837240219]
- >,
- "kernel" => #Nx.Tensor<
- f32[1][8]
- [
- [0.622833251953125, 0.24778570234775543, 0.4959430694580078, -0.604946494102478, -0.31578049063682556, 0.09977878630161285, 0.776294469833374, 0.5804685950279236]
- ]
- >
- },
- "dense_1" => %{
- "bias" => #Nx.Tensor<
- f32[4]
- [-0.012786266393959522, 0.01057625561952591, 0.10597240924835205, 0.13692162930965424]
- >,
- "kernel" => #Nx.Tensor<
- f32[8][4]
- [
- [-0.46233609318733215, -0.7435348033905029, -0.10738609731197357, 0.09911829978227615],
- [0.5295257568359375, 0.48769527673721313, -0.23950818181037903, -0.26084062457084656],
- [-0.5117107033729553, 0.2039143443107605, -0.12630638480186462, -0.41089773178100586],
- [-0.6043668985366821, 0.3961969316005707, 0.5120400190353394, -0.6773409247398376],
- [0.22123000025749207, 0.7197521924972534, 0.2679356038570404, -0.12402179092168808],
- [0.4830038249492645, 0.3629038631916046, 0.49994897842407227, -0.25865232944488525],
- [0.29824453592300415, 0.29333528876304626, -0.05371938645839691, 0.5230391621589661],
- [0.5483304262161255, 0.08283360302448273, -0.6959219574928284, 0.6471460461616516]
- ]
- >
- },
- "dense_2" => %{
- "bias" => #Nx.Tensor<
- f32[1]
- [0.07759959995746613]
- >,
- "kernel" => #Nx.Tensor<
- f32[4][1]
- [
- [-0.036170706152915955],
- [-0.5362256765365601],
- [-0.6853286027908325],
- [0.6693617701530457]
- ]
- >
- }
-}
+|> Axon.Loop.trainer(:mean_squared_error, optimizer)
+|> Axon.Loop.run(train_data, %{}, iterations: 1000)
Epoch: 0, Batch: 1000, loss: 0.0992607
%{
+ "dense_0" => %{
+ "bias" => #Nx.Tensor<
+ f32[8]
+ [0.06136200204491615, -0.08278193324804306, -0.07280997931957245, 0.08740464597940445, 0.08663233369588852, -0.06915996968746185, 0.03753892332315445, 0.06512840837240219]
+ >,
+ "kernel" => #Nx.Tensor<
+ f32[1][8]
+ [
+ [0.622833251953125, 0.24778570234775543, 0.4959430694580078, -0.604946494102478, -0.31578049063682556, 0.09977878630161285, 0.776294469833374, 0.5804685950279236]
+ ]
+ >
+ },
+ "dense_1" => %{
+ "bias" => #Nx.Tensor<
+ f32[4]
+ [-0.012786266393959522, 0.01057625561952591, 0.10597240924835205, 0.13692162930965424]
+ >,
+ "kernel" => #Nx.Tensor<
+ f32[8][4]
+ [
+ [-0.46233609318733215, -0.7435348033905029, -0.10738609731197357, 0.09911829978227615],
+ [0.5295257568359375, 0.48769527673721313, -0.23950818181037903, -0.26084062457084656],
+ [-0.5117107033729553, 0.2039143443107605, -0.12630638480186462, -0.41089773178100586],
+ [-0.6043668985366821, 0.3961969316005707, 0.5120400190353394, -0.6773409247398376],
+ [0.22123000025749207, 0.7197521924972534, 0.2679356038570404, -0.12402179092168808],
+ [0.4830038249492645, 0.3629038631916046, 0.49994897842407227, -0.25865232944488525],
+ [0.29824453592300415, 0.29333528876304626, -0.05371938645839691, 0.5230391621589661],
+ [0.5483304262161255, 0.08283360302448273, -0.6959219574928284, 0.6471460461616516]
+ ]
+ >
+ },
+ "dense_2" => %{
+ "bias" => #Nx.Tensor<
+ f32[1]
+ [0.07759959995746613]
+ >,
+ "kernel" => #Nx.Tensor<
+ f32[4][1]
+ [
+ [-0.036170706152915955],
+ [-0.5362256765365601],
+ [-0.6853286027908325],
+ [0.6693617701530457]
+ ]
+ >
+ }
+}
Mix.install([
- {:axon, "~> 0.3.0"},
- {:nx, "~> 0.4.0", override: true},
- {:exla, "~> 0.4.0"},
- {:scidata, "~> 0.1.9"}
-])
-
-Nx.Defn.default_options(compiler: EXLA)
Mix.install([
+ {:axon, "~> 0.3.0"},
+ {:nx, "~> 0.4.0", override: true},
+ {:exla, "~> 0.4.0"},
+ {:scidata, "~> 0.1.9"}
+])
+
+Nx.Defn.default_options(compiler: EXLA)
introduction
@@ -135,29 +135,29 @@To train and test how our model works, we use one of the most popular data sets: Fashion MNIST. It consists of small black and white images of clothes. Loading this data set is very simple with the help of Scidata
.
{image_data, _label_data} = Scidata.FashionMNIST.download()
-{bin, type, shape} = image_data
We get the data in a raw format, but this is exactly the information we need to build an Nx tensor.
train_images =
+To train and test how our model works, we use one of the most popular data sets: Fashion MNIST. It consists of small black and white images of clothes. Loading this data set is very simple with the help of Scidata
.
{image_data, _label_data} = Scidata.FashionMNIST.download()
+{bin, type, shape} = image_data
We get the data in a raw format, but this is exactly the information we need to build an Nx tensor.
train_images =
bin
- |> Nx.from_binary(type)
- |> Nx.reshape(shape)
- |> Nx.divide(255.0)
We also normalize pixel values into the range $[0, 1]$.
We can visualize one of the images by looking at the tensor heatmap:
Nx.to_heatmap(train_images[1])
+
|> Nx.from_binary(type)
+ |> Nx.reshape(shape)
+ |> Nx.divide(255.0)
We also normalize pixel values into the range $[0, 1]$.
We can visualize one of the images by looking at the tensor heatmap:
Nx.to_heatmap(train_images[1])
encoder-and-decoder
Encoder and decoderFirst we need to define the encoder and decoder. Both are one-layer neural networks.
In the encoder, we start by flattening the input, so we get from shape {batch_size, 1, 28, 28}
to {batch_size, 784}
and we pass the input into a dense layer. Our dense layer has only latent_dim
number of neurons. The latent_dim
(or the latent space) is a compressed representation of data. Remember, we want our encoder to compress the input data into a lower-dimensional representation, so we choose a latent_dim
which is less than the dimensionality of the input.
encoder = fn x, latent_dim ->
+First we need to define the encoder and decoder. Both are one-layer neural networks.
In the encoder, we start by flattening the input, so we get from shape {batch_size, 1, 28, 28}
to {batch_size, 784}
and we pass the input into a dense layer. Our dense layer has only latent_dim
number of neurons. The latent_dim
(or the latent space) is a compressed representation of data. Remember, we want our encoder to compress the input data into a lower-dimensional representation, so we choose a latent_dim
which is less than the dimensionality of the input.
encoder = fn x, latent_dim ->
x
- |> Axon.flatten()
- |> Axon.dense(latent_dim, activation: :relu)
-end
Next, we pass the output of the encoder to the decoder and try to reconstruct the compressed data into its original form. Since our original input had a dimensionality of 784, we use a dense layer with 784 neurons. Because our original data was normalized to have pixel values between 0 and 1, we use a :sigmoid
activation in our dense layer to squeeze output values between 0 and 1. Our original input shape was 28x28, so we use Axon.reshape
to convert the flattened representation of the outputs into an image with correct the width and height.
decoder = fn x ->
+ |> Axon.flatten()
+ |> Axon.dense(latent_dim, activation: :relu)
+end
Next, we pass the output of the encoder to the decoder and try to reconstruct the compressed data into its original form. Since our original input had a dimensionality of 784, we use a dense layer with 784 neurons. Because our original data was normalized to have pixel values between 0 and 1, we use a :sigmoid
activation in our dense layer to squeeze output values between 0 and 1. Our original input shape was 28x28, so we use Axon.reshape
to convert the flattened representation of the outputs into an image with correct the width and height.
decoder = fn x ->
x
- |> Axon.dense(784, activation: :sigmoid)
- |> Axon.reshape({:batch, 1, 28, 28})
-end
If we just bind the encoder and decoder sequentially, we'll get the desired model. This was pretty smooth, wasn't it?
model =
- Axon.input("input", shape: {nil, 1, 28, 28})
- |> encoder.(64)
- |> decoder.()
+
|> Axon.dense(784, activation: :sigmoid)
+ |> Axon.reshape({:batch, 1, 28, 28})
+end
If we just bind the encoder and decoder sequentially, we'll get the desired model. This was pretty smooth, wasn't it?
model =
+ Axon.input("input", shape: {nil, 1, 28, 28})
+ |> encoder.(64)
+ |> decoder.()
training-the-model
@@ -166,14 +166,14 @@Finally, we can train the model. We'll use the :adam
and :mean_squared_error
loss with Axon.Loop.trainer
. Our loss function will measure the aggregate error between pixels of original images and the model's reconstructed images. We'll also :mean_absolute_error
using Axon.Loop.metric
. Axon.Loop.run
trains the model with the given training data.
batch_size = 32
epochs = 5
-batched_images = Nx.to_batched(train_images, batch_size)
-train_batches = Stream.zip(batched_images, batched_images)
+batched_images = Nx.to_batched(train_images, batch_size)
+train_batches = Stream.zip(batched_images, batched_images)
params =
model
- |> Axon.Loop.trainer(:mean_squared_error, :adam)
- |> Axon.Loop.metric(:mean_absolute_error, "Error")
- |> Axon.Loop.run(train_batches, %{}, epochs: epochs, compiler: EXLA)
extra-losses
@@ -181,46 +181,46 @@To better understand what is mean absolute error (MAE) and mean square error (MSE) let's go through an example.
# Error definitions for a single sample
-mean_square_error = fn y_pred, y ->
+mean_square_error = fn y_pred, y ->
y_pred
- |> Nx.subtract(y)
- |> Nx.power(2)
- |> Nx.mean()
-end
+ |> Nx.subtract(y)
+ |> Nx.power(2)
+ |> Nx.mean()
+end
-mean_absolute_erorr = fn y_pred, y ->
+mean_absolute_erorr = fn y_pred, y ->
y_pred
- |> Nx.subtract(y)
- |> Nx.abs()
- |> Nx.mean()
-end
We will work with a sample image of a shoe, a slightly noised version of that image, and also an entirely different image from the dataset.
shoe_image = train_images[0]
-noised_shoe_image = Nx.add(shoe_image, Nx.random_normal(shoe_image, 0.0, 0.05))
-other_image = train_images[1]
-:ok
For the same image both errors should be 0, because when we have two exact copies, there is no pixel difference.
{
- mean_square_error.(shoe_image, shoe_image),
- mean_absolute_erorr.(shoe_image, shoe_image)
-}
Now the noised image:
{
- mean_square_error.(shoe_image, noised_shoe_image),
- mean_absolute_erorr.(shoe_image, noised_shoe_image)
-}
And a different image:
{
- mean_square_error.(shoe_image, other_image),
- mean_absolute_erorr.(shoe_image, other_image)
-}
As we can see, the noised image has a non-zero MSE and MAE but is much smaller than the error of two completely different pictures. In other words, both of these error types measure the level of similarity between images. A small error implies decent prediction values. On the other hand, a large error value suggests poor quality of predictions.
If you look at our implementation of MAE and MSE, you will notice that they are very similar. MAE and MSE can also be called the $L_1$ and $L_2$ loss respectively for the $L_1$ and $L_2$ norm. The $L_2$ loss (MSE) is typically preferred because it's a smoother function whereas $L_1$ is often difficult to optimize with stochastic gradient descent (SGD).
We will work with a sample image of a shoe, a slightly noised version of that image, and also an entirely different image from the dataset.
shoe_image = train_images[0]
+noised_shoe_image = Nx.add(shoe_image, Nx.random_normal(shoe_image, 0.0, 0.05))
+other_image = train_images[1]
+:ok
For the same image both errors should be 0, because when we have two exact copies, there is no pixel difference.
{
+ mean_square_error.(shoe_image, shoe_image),
+ mean_absolute_erorr.(shoe_image, shoe_image)
+}
Now the noised image:
{
+ mean_square_error.(shoe_image, noised_shoe_image),
+ mean_absolute_erorr.(shoe_image, noised_shoe_image)
+}
And a different image:
{
+ mean_square_error.(shoe_image, other_image),
+ mean_absolute_erorr.(shoe_image, other_image)
+}
As we can see, the noised image has a non-zero MSE and MAE but is much smaller than the error of two completely different pictures. In other words, both of these error types measure the level of similarity between images. A small error implies decent prediction values. On the other hand, a large error value suggests poor quality of predictions.
If you look at our implementation of MAE and MSE, you will notice that they are very similar. MAE and MSE can also be called the $L_1$ and $L_2$ loss respectively for the $L_1$ and $L_2$ norm. The $L_2$ loss (MSE) is typically preferred because it's a smoother function whereas $L_1$ is often difficult to optimize with stochastic gradient descent (SGD).
inference
InferenceNow, let's see how our model is doing! We will compare a sample image before and after compression.
sample_image = train_images[0..0//1]
-compressed_image = Axon.predict(model, params, sample_image, compiler: EXLA)
+Now, let's see how our model is doing! We will compare a sample image before and after compression.
sample_image = train_images[0..0//1]
+compressed_image = Axon.predict(model, params, sample_image, compiler: EXLA)
sample_image
-|> Nx.to_heatmap()
-|> IO.inspect(label: "Original")
+|> Nx.to_heatmap()
+|> IO.inspect(label: "Original")
compressed_image
-|> Nx.to_heatmap()
-|> IO.inspect(label: "Compressed")
+|> Nx.to_heatmap()
+|> IO.inspect(label: "Compressed")
:ok
As we can see, the generated image is similar to the input image. The only difference between them is the absence of a sign in the middle of the second shoe. The model treated the sign as noise and bled this into the plain shoe.
diff --git a/fashionmnist_vae.html b/fashionmnist_vae.html
index 8e8573a0..e73e4e88 100644
--- a/fashionmnist_vae.html
+++ b/fashionmnist_vae.html
@@ -115,23 +115,23 @@
-Mix.install([
- {:exla, "~> 0.4.0"},
- {:nx, "~> 0.4.0", override: true},
- {:axon, "~> 0.3.0"},
- {:req, "~> 0.3.1"},
- {:kino, "~> 0.7.0"},
- {:scidata, "~> 0.1.9"},
- {:stb_image, "~> 0.5.2"},
- {:kino_vega_lite, "~> 0.1.6"},
- {:vega_lite, "~> 0.1.6"},
- {:table_rex, "~> 3.1.1"}
-])
+Mix.install([
+ {:exla, "~> 0.4.0"},
+ {:nx, "~> 0.4.0", override: true},
+ {:axon, "~> 0.3.0"},
+ {:req, "~> 0.3.1"},
+ {:kino, "~> 0.7.0"},
+ {:scidata, "~> 0.1.9"},
+ {:stb_image, "~> 0.5.2"},
+ {:kino_vega_lite, "~> 0.1.6"},
+ {:vega_lite, "~> 0.1.6"},
+ {:table_rex, "~> 3.1.1"}
+])
alias VegaLite, as: Vl
# This speeds up all our `Nx` operations without having to use `defn`
-Nx.global_default_backend(EXLA.Backend)
+Nx.global_default_backend(EXLA.Backend)
:ok
@@ -145,7 +145,7 @@
Training a simple autoencoder
-This section will proceed without much explanation as most of it is extracted from denoising autoencoder example. If anything here doesn't make sense, take a look at that notebook for an explanation.
defmodule Data do
+This section will proceed without much explanation as most of it is extracted from denoising autoencoder example. If anything here doesn't make sense, take a look at that notebook for an explanation.
defmodule Data do
@moduledoc """
A module to hold useful data processing utilities,
mostly extracted from the previous notebook
@@ -157,182 +157,182 @@
`image` must be a single channel `Nx` tensor with pixel values between 0 and 1.
`height` and `width` are the output size in pixels
"""
- def image_to_kino(image, height \\ 200, width \\ 200) do
+ def image_to_kino(image, height \\ 200, width \\ 200) do
image
- |> Nx.multiply(255)
- |> Nx.as_type(:u8)
- |> Nx.transpose(axes: [:height, :width, :channels])
- |> StbImage.from_nx()
- |> StbImage.resize(height, width)
- |> StbImage.to_binary(:png)
- |> Kino.Image.new(:png)
- end
+ |> Nx.multiply(255)
+ |> Nx.as_type(:u8)
+ |> Nx.transpose(axes: [:height, :width, :channels])
+ |> StbImage.from_nx()
+ |> StbImage.resize(height, width)
+ |> StbImage.to_binary(:png)
+ |> Kino.Image.new(:png)
+ end
@doc """
Converts image data from `Scidata.MNIST` into an `Nx` tensor and normalizes it.
"""
- def preprocess_data(data) do
- {image_data, _labels} = data
- {images_binary, type, shape} = image_data
+ def preprocess_data(data) do
+ {image_data, _labels} = data
+ {images_binary, type, shape} = image_data
images_binary
- |> Nx.from_binary(type)
+ |> Nx.from_binary(type)
# Since pixels are organized row-wise, reshape into rows x columns
- |> Nx.reshape(shape, names: [:images, :channels, :height, :width])
+ |> Nx.reshape(shape, names: [:images, :channels, :height, :width])
# Normalize the pixel values to be between 0 and 1
- |> Nx.divide(255)
- end
+ |> Nx.divide(255)
+ end
@doc """
Converts a tensor of images into random batches of paired images for model training
"""
- def prepare_training_data(images, batch_size) do
- Stream.flat_map([nil], fn nil ->
- images |> Nx.shuffle(axis: :images) |> Nx.to_batched(batch_size)
- end)
- |> Stream.map(fn batch -> {batch, batch} end)
- end
-end
train_images = Data.preprocess_data(Scidata.FashionMNIST.download())
-test_images = Data.preprocess_data(Scidata.FashionMNIST.download_test())
-
-Kino.render(train_images[[images: 0]] |> Data.image_to_kino())
-Kino.render(test_images[[images: 0]] |> Data.image_to_kino())
-
-:ok
Now for our simple autoencoder model. We won't be using a denoising autoencoder here.
Note that we're giving each of the layers a name - the reason for this will be apparent later.
I'm also using a small custom layer to shift and scale the output of the sigmoid layer slightly so it can hit the 0 and 1 targets. I noticed the gradients tend to explode without this.
defmodule CustomLayer do
+ def prepare_training_data(images, batch_size) do
+ Stream.flat_map([nil], fn nil ->
+ images |> Nx.shuffle(axis: :images) |> Nx.to_batched(batch_size)
+ end)
+ |> Stream.map(fn batch -> {batch, batch} end)
+ end
+end
train_images = Data.preprocess_data(Scidata.FashionMNIST.download())
+test_images = Data.preprocess_data(Scidata.FashionMNIST.download_test())
+
+Kino.render(train_images[[images: 0]] |> Data.image_to_kino())
+Kino.render(test_images[[images: 0]] |> Data.image_to_kino())
+
+:ok
Now for our simple autoencoder model. We won't be using a denoising autoencoder here.
Note that we're giving each of the layers a name - the reason for this will be apparent later.
I'm also using a small custom layer to shift and scale the output of the sigmoid layer slightly so it can hit the 0 and 1 targets. I noticed the gradients tend to explode without this.
defmodule CustomLayer do
import Nx.Defn
- def scaling_layer(%Axon{} = input, _opts \\ []) do
- Axon.layer(&scaling_layer_impl/2, [input])
- end
+ def scaling_layer(%Axon{} = input, _opts \\ []) do
+ Axon.layer(&scaling_layer_impl/2, [input])
+ end
- defnp scaling_layer_impl(x, _opts \\ []) do
+ defnp scaling_layer_impl(x, _opts \\ []) do
x
- |> Nx.subtract(0.05)
- |> Nx.multiply(1.2)
- end
-end
model =
- Axon.input("image", shape: {nil, 1, 28, 28})
+ |> Nx.subtract(0.05)
+ |> Nx.multiply(1.2)
+ end
+end
model =
+ Axon.input("image", shape: {nil, 1, 28, 28})
# This is now 28*28*1 = 784
- |> Axon.flatten()
+ |> Axon.flatten()
# The encoder
- |> Axon.dense(256, activation: :relu, name: "encoder_layer_1")
- |> Axon.dense(128, activation: :relu, name: "encoder_layer_2")
- |> Axon.dense(64, activation: :relu, name: "encoder_layer_3")
+ |> Axon.dense(256, activation: :relu, name: "encoder_layer_1")
+ |> Axon.dense(128, activation: :relu, name: "encoder_layer_2")
+ |> Axon.dense(64, activation: :relu, name: "encoder_layer_3")
# Bottleneck layer
- |> Axon.dense(10, activation: :relu, name: "bottleneck_layer")
+ |> Axon.dense(10, activation: :relu, name: "bottleneck_layer")
# The decoder
- |> Axon.dense(64, activation: :relu, name: "decoder_layer_1")
- |> Axon.dense(128, activation: :relu, name: "decoder_layer_2")
- |> Axon.dense(256, activation: :relu, name: "decoder_layer_3")
- |> Axon.dense(784, activation: :sigmoid, name: "decoder_layer_4")
- |> CustomLayer.scaling_layer()
+ |> Axon.dense(64, activation: :relu, name: "decoder_layer_1")
+ |> Axon.dense(128, activation: :relu, name: "decoder_layer_2")
+ |> Axon.dense(256, activation: :relu, name: "decoder_layer_3")
+ |> Axon.dense(784, activation: :sigmoid, name: "decoder_layer_4")
+ |> CustomLayer.scaling_layer()
# Turn it back into a 28x28 single channel image
- |> Axon.reshape({:auto, 1, 28, 28})
+ |> Axon.reshape({:auto, 1, 28, 28})
# We can use Axon.Display to show us what each of the layers would look like
# assuming we send in a batch of 4 images
-Axon.Display.as_table(model, Nx.template({4, 1, 28, 28}, :f32)) |> IO.puts()
batch_size = 128
+Axon.Display.as_table(model, Nx.template({4, 1, 28, 28}, :f32)) |> IO.puts()
batch_size = 128
-train_data = Data.prepare_training_data(train_images, 128)
-test_data = Data.prepare_training_data(test_images, 128)
+train_data = Data.prepare_training_data(train_images, 128)
+test_data = Data.prepare_training_data(test_images, 128)
-{input_batch, target_batch} = Enum.at(train_data, 0)
-Kino.render(input_batch[[images: 0]] |> Data.image_to_kino())
-Kino.render(target_batch[[images: 0]] |> Data.image_to_kino())
+{input_batch, target_batch} = Enum.at(train_data, 0)
+Kino.render(input_batch[[images: 0]] |> Data.image_to_kino())
+Kino.render(target_batch[[images: 0]] |> Data.image_to_kino())
-:ok
When training, it can be useful to stop execution early - either when you see it's failing and you don't want to waste time waiting for the remaining epochs to finish, or if it's good enough and you want to start experimenting with it.
The kino_early_stop/1
function below is a handy handler to give us a Kino.Control.button
that will stop the training loop when clicked.
We also have plot_losses/1
function to visualize our train and validation losses using VegaLite
.
defmodule KinoAxon do
+:ok
When training, it can be useful to stop execution early - either when you see it's failing and you don't want to waste time waiting for the remaining epochs to finish, or if it's good enough and you want to start experimenting with it.
The kino_early_stop/1
function below is a handy handler to give us a Kino.Control.button
that will stop the training loop when clicked.
We also have plot_losses/1
function to visualize our train and validation losses using VegaLite
.
defmodule KinoAxon do
@doc """
Adds handler function which adds a frame with a "stop" button
to the cell with the training loop.
Clicking "stop" will halt the training loop.
"""
- def kino_early_stop(loop) do
- frame = Kino.Frame.new() |> Kino.render()
- stop_button = Kino.Control.button("stop")
- Kino.Frame.render(frame, stop_button)
+ def kino_early_stop(loop) do
+ frame = Kino.Frame.new() |> Kino.render()
+ stop_button = Kino.Control.button("stop")
+ Kino.Frame.render(frame, stop_button)
- {:ok, button_agent} = Agent.start_link(fn -> nil end)
+ {:ok, button_agent} = Agent.start_link(fn -> nil end)
stop_button
- |> Kino.Control.stream()
- |> Kino.listen(fn _event ->
- Agent.update(button_agent, fn _ -> :stop end)
- end)
-
- handler = fn state ->
- stop_state = Agent.get(button_agent, & &1)
-
- if stop_state == :stop do
- Agent.stop(button_agent)
- Kino.Frame.render(frame, "stopped")
- {:halt_loop, state}
- else
- {:continue, state}
- end
- end
-
- Axon.Loop.handle(loop, :iteration_completed, handler)
- end
+ |> Kino.Control.stream()
+ |> Kino.listen(fn _event ->
+ Agent.update(button_agent, fn _ -> :stop end)
+ end)
+
+ handler = fn state ->
+ stop_state = Agent.get(button_agent, & &1)
+
+ if stop_state == :stop do
+ Agent.stop(button_agent)
+ Kino.Frame.render(frame, "stopped")
+ {:halt_loop, state}
+ else
+ {:continue, state}
+ end
+ end
+
+ Axon.Loop.handle(loop, :iteration_completed, handler)
+ end
@doc """
Plots the training and validation losses using Kino and VegaLite.
This *must* come after `Axon.Loop.validate`.
"""
- def plot_losses(loop) do
+ def plot_losses(loop) do
vl_widget =
- Vl.new(width: 600, height: 400)
- |> Vl.mark(:point, tooltip: true)
- |> Vl.encode_field(:x, "epoch", type: :ordinal)
- |> Vl.encode_field(:y, "loss", type: :quantitative)
- |> Vl.encode_field(:color, "dataset", type: :nominal)
- |> Kino.VegaLite.new()
- |> Kino.render()
-
- handler = fn state ->
- %Axon.Loop.State{metrics: metrics, epoch: epoch} = state
- loss = metrics["loss"] |> Nx.to_number()
- val_loss = metrics["validation_loss"] |> Nx.to_number()
-
- points = [
- %{epoch: epoch, loss: loss, dataset: "train"},
- %{epoch: epoch, loss: val_loss, dataset: "validation"}
- ]
-
- Kino.VegaLite.push_many(vl_widget, points)
- {:continue, state}
- end
-
- Axon.Loop.handle(loop, :epoch_completed, handler)
- end
-end
# A helper function to display the input and output side by side
-combined_input_output = fn params, image_index ->
- test_image = test_images[[images: image_index]]
- reconstructed_image = Axon.predict(model, params, test_image) |> Nx.squeeze(axes: [0])
- Nx.concatenate([test_image, reconstructed_image], axis: :width)
-end
-
-frame = Kino.Frame.new() |> Kino.render()
-
-render_example_handler = fn state ->
+ Vl.new(width: 600, height: 400)
+ |> Vl.mark(:point, tooltip: true)
+ |> Vl.encode_field(:x, "epoch", type: :ordinal)
+ |> Vl.encode_field(:y, "loss", type: :quantitative)
+ |> Vl.encode_field(:color, "dataset", type: :nominal)
+ |> Kino.VegaLite.new()
+ |> Kino.render()
+
+ handler = fn state ->
+ %Axon.Loop.State{metrics: metrics, epoch: epoch} = state
+ loss = metrics["loss"] |> Nx.to_number()
+ val_loss = metrics["validation_loss"] |> Nx.to_number()
+
+ points = [
+ %{epoch: epoch, loss: loss, dataset: "train"},
+ %{epoch: epoch, loss: val_loss, dataset: "validation"}
+ ]
+
+ Kino.VegaLite.push_many(vl_widget, points)
+ {:continue, state}
+ end
+
+ Axon.Loop.handle(loop, :epoch_completed, handler)
+ end
+end
# A helper function to display the input and output side by side
+combined_input_output = fn params, image_index ->
+ test_image = test_images[[images: image_index]]
+ reconstructed_image = Axon.predict(model, params, test_image) |> Nx.squeeze(axes: [0])
+ Nx.concatenate([test_image, reconstructed_image], axis: :width)
+end
+
+frame = Kino.Frame.new() |> Kino.render()
+
+render_example_handler = fn state ->
# state.step_state[:model_state] contains the model params when this event is fired
- params = state.step_state[:model_state]
- image_index = Enum.random(0..(Nx.axis_size(test_images, :images) - 1))
- image = combined_input_output.(params, image_index) |> Data.image_to_kino(200, 400)
- Kino.Frame.render(frame, image)
- Kino.Frame.append(frame, "Epoch: #{state.epoch}, Iteration: #{state.iteration}")
- {:continue, state}
-end
+ params = state.step_state[:model_state]
+ image_index = Enum.random(0..(Nx.axis_size(test_images, :images) - 1))
+ image = combined_input_output.(params, image_index) |> Data.image_to_kino(200, 400)
+ Kino.Frame.render(frame, image)
+ Kino.Frame.append(frame, "Epoch: #{state.epoch}, Iteration: #{state.iteration}")
+ {:continue, state}
+end
params =
model
- |> Axon.Loop.trainer(:mean_squared_error, Axon.Optimizers.adamw(0.001))
- |> KinoAxon.kino_early_stop()
- |> Axon.Loop.handle(:iteration_completed, render_example_handler, every: 450)
- |> Axon.Loop.validate(model, test_data)
- |> KinoAxon.plot_losses()
- |> Axon.Loop.run(train_data, %{}, epochs: 40, compiler: EXLA)
+ |> Axon.Loop.trainer(:mean_squared_error, Axon.Optimizers.adamw(0.001))
+ |> KinoAxon.kino_early_stop()
+ |> Axon.Loop.handle(:iteration_completed, render_example_handler, every: 450)
+ |> Axon.Loop.validate(model, test_data)
+ |> KinoAxon.plot_losses()
+ |> Axon.Loop.run(train_data, %{}, epochs: 40, compiler: EXLA)
:ok
@@ -341,191 +341,191 @@
Splitting up the model
Cool! We now have the parameters for a trained, simple autoencoder. Our next step is to split up the model so we can use the encoder and decoder separately. By doing that, we'll be able to take an image and encode it to get the model's compressed image representation (the latent vector). We can then manipulate the latent vector and run the manipulated latent vector through the decoder to get a new image.
Let's start by defining the encoder and decoder separately as two different models.
encoder =
- Axon.input("image", shape: {nil, 1, 28, 28})
+ Axon.input("image", shape: {nil, 1, 28, 28})
# This is now 28*28*1 = 784
- |> Axon.flatten()
+ |> Axon.flatten()
# The encoder
- |> Axon.dense(256, activation: :relu, name: "encoder_layer_1")
- |> Axon.dense(128, activation: :relu, name: "encoder_layer_2")
- |> Axon.dense(64, activation: :relu, name: "encoder_layer_3")
+ |> Axon.dense(256, activation: :relu, name: "encoder_layer_1")
+ |> Axon.dense(128, activation: :relu, name: "encoder_layer_2")
+ |> Axon.dense(64, activation: :relu, name: "encoder_layer_3")
# Bottleneck layer
- |> Axon.dense(10, activation: :relu, name: "bottleneck_layer")
+ |> Axon.dense(10, activation: :relu, name: "bottleneck_layer")
# The output from the encoder
decoder =
- Axon.input("latent", shape: {nil, 10})
+ Axon.input("latent", shape: {nil, 10})
# The decoder
- |> Axon.dense(64, activation: :relu, name: "decoder_layer_1")
- |> Axon.dense(128, activation: :relu, name: "decoder_layer_2")
- |> Axon.dense(256, activation: :relu, name: "decoder_layer_3")
- |> Axon.dense(784, activation: :sigmoid, name: "decoder_layer_4")
- |> CustomLayer.scaling_layer()
+ |> Axon.dense(64, activation: :relu, name: "decoder_layer_1")
+ |> Axon.dense(128, activation: :relu, name: "decoder_layer_2")
+ |> Axon.dense(256, activation: :relu, name: "decoder_layer_3")
+ |> Axon.dense(784, activation: :sigmoid, name: "decoder_layer_4")
+ |> CustomLayer.scaling_layer()
# Turn it back into a 28x28 single channel image
- |> Axon.reshape({:auto, 1, 28, 28})
+ |> Axon.reshape({:auto, 1, 28, 28})
-Axon.Display.as_table(encoder, Nx.template({4, 1, 28, 28}, :f32)) |> IO.puts()
-Axon.Display.as_table(decoder, Nx.template({4, 10}, :f32)) |> IO.puts()
We have the two models, but the problem is these are untrained models so we don't have the corresponding set of parameters. We'd like to use the parameters from the autoencoder we just trained and apply them to our split up models.
Let's first take a look at what params actually are:
params
Params are just a Map
with the layer name as the key identifying which parameters to use. We can easily match up the layer names with the output from the Axon.Display.as_table/2
call for the autoencoder model.
So all we need to do is create a new Map that plucks out the right layers from our autoencoder params
for each model and use that to run inference on our split up models.
Fortunately, since we gave each of the layers names, this requires no work at all - we can use the Map as it is since the layer names match up! Axon will ignore any extra keys so those won't be a problem.
Note that naming the layers wasn't required, if the layers didn't have names we would have some renaming to do to get the names to match between the models. But giving them names made it very convenient :)
Let's try encoding an image, printing the latent and then decoding the latent using our split up model to make sure it's working.
image = test_images[[images: 0]]
+Axon.Display.as_table(encoder, Nx.template({4, 1, 28, 28}, :f32)) |> IO.puts()
+Axon.Display.as_table(decoder, Nx.template({4, 10}, :f32)) |> IO.puts()
We have the two models, but the problem is these are untrained models so we don't have the corresponding set of parameters. We'd like to use the parameters from the autoencoder we just trained and apply them to our split up models.
Let's first take a look at what params actually are:
params
Params are just a Map
with the layer name as the key identifying which parameters to use. We can easily match up the layer names with the output from the Axon.Display.as_table/2
call for the autoencoder model.
So all we need to do is create a new Map that plucks out the right layers from our autoencoder params
for each model and use that to run inference on our split up models.
Fortunately, since we gave each of the layers names, this requires no work at all - we can use the Map as it is since the layer names match up! Axon will ignore any extra keys so those won't be a problem.
Note that naming the layers wasn't required, if the layers didn't have names we would have some renaming to do to get the names to match between the models. But giving them names made it very convenient :)
Let's try encoding an image, printing the latent and then decoding the latent using our split up model to make sure it's working.
image = test_images[[images: 0]]
# Encode the image
-latent = Axon.predict(encoder, params, image)
-IO.inspect(latent, label: "Latent")
+latent = Axon.predict(encoder, params, image)
+IO.inspect(latent, label: "Latent")
# Decode the image
-reconstructed_image = Axon.predict(decoder, params, latent) |> Nx.squeeze(axes: [0])
+reconstructed_image = Axon.predict(decoder, params, latent) |> Nx.squeeze(axes: [0])
-combined_image = Nx.concatenate([image, reconstructed_image], axis: :width)
-Data.image_to_kino(combined_image, 200, 400)
Perfect! Seems like the split up models are working as expected. Now let's try to generate some new images using our autoencoder. To do this, we'll manipulate the latent so it's slightly different from what the encoder gave us. Specifically, we'll try to interpolate between two images, showing 100 steps from our starting image to our final image.
num_steps = 100
+combined_image = Nx.concatenate([image, reconstructed_image], axis: :width)
+Data.image_to_kino(combined_image, 200, 400)
Perfect! Seems like the split up models are working as expected. Now let's try to generate some new images using our autoencoder. To do this, we'll manipulate the latent so it's slightly different from what the encoder gave us. Specifically, we'll try to interpolate between two images, showing 100 steps from our starting image to our final image.
num_steps = 100
# Get our latents, image at index 0 is our starting point
# index 1 is where we'll end
-latents = Axon.predict(encoder, params, test_images[[images: 0..1]])
+latents = Axon.predict(encoder, params, test_images[[images: 0..1]])
# Latents is a {2, 10} tensor
# The step we'll add to our latent to move it towards image[1]
-step = Nx.subtract(latents[1], latents[0]) |> Nx.divide(num_steps)
+step = Nx.subtract(latents[1], latents[0]) |> Nx.divide(num_steps)
# We can make a batch of all our new latents
-new_latents = Nx.multiply(Nx.iota({num_steps + 1, 1}), step) |> Nx.add(latents[0])
+new_latents = Nx.multiply(Nx.iota({num_steps + 1, 1}), step) |> Nx.add(latents[0])
-reconstructed_images = Axon.predict(decoder, params, new_latents)
+reconstructed_images = Axon.predict(decoder, params, new_latents)
reconstructed_images =
- Nx.reshape(
+ Nx.reshape(
reconstructed_images,
- Nx.shape(reconstructed_images),
- names: [:images, :channels, :height, :width]
- )
-
-Stream.interval(div(5000, num_steps))
-|> Stream.take(num_steps + 1)
-|> Kino.animate(fn i ->
- Data.image_to_kino(reconstructed_images[i])
-end)
Cool! We have interpolation! But did you notice that some of the intermediate frames don't look fashionable at all? Autoencoders don't generally return good results for random vectors in their latent space. That's where a VAE can help.
+
Nx.shape(reconstructed_images),
+ names: [:images, :channels, :height, :width]
+ )
+
+Stream.interval(div(5000, num_steps))
+|> Stream.take(num_steps + 1)
+|> Kino.animate(fn i ->
+ Data.image_to_kino(reconstructed_images[i])
+end)
Cool! We have interpolation! But did you notice that some of the intermediate frames don't look fashionable at all? Autoencoders don't generally return good results for random vectors in their latent space. That's where a VAE can help.
making-it-variational
Making it variational
-In a VAE, instead of outputting a latent vector, our encoder will output a distribution. Essentially this means instead of 10 outputs we'll have 20. 10 of them will represent the mean and 10 will represent the log of the variance of the latent. We'll have to sample from this distribution to get our latent vector. Finally, we'll have to modify our loss function to also compute the KL Divergence between the latent distribution and a standard normal distribution (this acts as a regularizer of the latent space).
We'll start by defining our model:
defmodule Vae do
+In a VAE, instead of outputting a latent vector, our encoder will output a distribution. Essentially this means instead of 10 outputs we'll have 20. 10 of them will represent the mean and 10 will represent the log of the variance of the latent. We'll have to sample from this distribution to get our latent vector. Finally, we'll have to modify our loss function to also compute the KL Divergence between the latent distribution and a standard normal distribution (this acts as a regularizer of the latent space).
We'll start by defining our model:
defmodule Vae do
import Nx.Defn
@latent_features 10
- defp sampling_layer(%Axon{} = input, _opts \\ []) do
- Axon.layer(&sampling_layer_impl/2, [input], name: "sampling_layer", op_name: :sample)
- end
+ defp sampling_layer(%Axon{} = input, _opts \\ []) do
+ Axon.layer(&sampling_layer_impl/2, [input], name: "sampling_layer", op_name: :sample)
+ end
- defnp sampling_layer_impl(x, _opts \\ []) do
- mu = x[[0..-1//1, 0, 0..-1//1]]
- log_var = x[[0..-1//1, 1, 0..-1//1]]
- std_dev = Nx.exp(0.5 * log_var)
- eps = Nx.random_normal(std_dev)
+ defnp sampling_layer_impl(x, _opts \\ []) do
+ mu = x[[0..-1//1, 0, 0..-1//1]]
+ log_var = x[[0..-1//1, 1, 0..-1//1]]
+ std_dev = Nx.exp(0.5 * log_var)
+ eps = Nx.random_normal(std_dev)
sample = mu + std_dev * eps
- Nx.stack([sample, mu, std_dev], axis: 1)
- end
+ Nx.stack([sample, mu, std_dev], axis: 1)
+ end
- defp encoder_partial() do
- Axon.input("image", shape: {nil, 1, 28, 28})
+ defp encoder_partial() do
+ Axon.input("image", shape: {nil, 1, 28, 28})
# This is now 28*28*1 = 784
- |> Axon.flatten()
+ |> Axon.flatten()
# The encoder
- |> Axon.dense(256, activation: :relu, name: "encoder_layer_1")
- |> Axon.dense(128, activation: :relu, name: "encoder_layer_2")
- |> Axon.dense(64, activation: :relu, name: "encoder_layer_3")
+ |> Axon.dense(256, activation: :relu, name: "encoder_layer_1")
+ |> Axon.dense(128, activation: :relu, name: "encoder_layer_2")
+ |> Axon.dense(64, activation: :relu, name: "encoder_layer_3")
# Bottleneck layer
- |> Axon.dense(@latent_features * 2, name: "bottleneck_layer")
+ |> Axon.dense(@latent_features * 2, name: "bottleneck_layer")
# Split up the mu and logvar
- |> Axon.reshape({:auto, 2, @latent_features})
- |> sampling_layer()
- end
+ |> Axon.reshape({:auto, 2, @latent_features})
+ |> sampling_layer()
+ end
- def encoder() do
- encoder_partial()
+ def encoder() do
+ encoder_partial()
# Grab only the sample (ie. the sampled latent)
- |> Axon.nx(fn x -> x[[0..-1//1, 0]] end)
- end
+ |> Axon.nx(fn x -> x[[0..-1//1, 0]] end)
+ end
- def decoder(input_latent) do
+ def decoder(input_latent) do
input_latent
- |> Axon.dense(64, activation: :relu, name: "decoder_layer_1")
- |> Axon.dense(128, activation: :relu, name: "decoder_layer_2")
- |> Axon.dense(256, activation: :relu, name: "decoder_layer_3")
- |> Axon.dense(784, activation: :sigmoid, name: "decoder_layer_4")
- |> CustomLayer.scaling_layer()
+ |> Axon.dense(64, activation: :relu, name: "decoder_layer_1")
+ |> Axon.dense(128, activation: :relu, name: "decoder_layer_2")
+ |> Axon.dense(256, activation: :relu, name: "decoder_layer_3")
+ |> Axon.dense(784, activation: :sigmoid, name: "decoder_layer_4")
+ |> CustomLayer.scaling_layer()
# Turn it back into a 28x28 single channel image
- |> Axon.reshape({:auto, 1, 28, 28})
- end
-
- def autoencoder() do
- encoder_partial = encoder_partial()
- encoder = encoder()
- autoencoder = decoder(encoder)
- Axon.container(%{mu_sigma: encoder_partial, reconstruction: autoencoder})
- end
-end
There's a few interesting things going on here. First, since our model has become more complex, we've used a module to keep it organized. We also built a custom layer to do the sampling and output the sampled latent vector as well as the distribution parameters (mu and sigma).
Finally, we need the distribution itself so we can calculate the KL Divergence in our loss function. To make the model output the distribution parameters (mu and sigma), we use Axon.container/1
to produce two outputs from our model instead of one. Now, instead of getting a tensor as an output, we'll get a map with the two tensors we need for our loss function.
Our loss function also has to be modified so be the sum of the KL divergence and MSE. Here's our custom loss function:
defmodule CustomLoss do
+ |> Axon.reshape({:auto, 1, 28, 28})
+ end
+
+ def autoencoder() do
+ encoder_partial = encoder_partial()
+ encoder = encoder()
+ autoencoder = decoder(encoder)
+ Axon.container(%{mu_sigma: encoder_partial, reconstruction: autoencoder})
+ end
+end
There's a few interesting things going on here. First, since our model has become more complex, we've used a module to keep it organized. We also built a custom layer to do the sampling and output the sampled latent vector as well as the distribution parameters (mu and sigma).
Finally, we need the distribution itself so we can calculate the KL Divergence in our loss function. To make the model output the distribution parameters (mu and sigma), we use Axon.container/1
to produce two outputs from our model instead of one. Now, instead of getting a tensor as an output, we'll get a map with the two tensors we need for our loss function.
Our loss function also has to be modified so be the sum of the KL divergence and MSE. Here's our custom loss function:
defmodule CustomLoss do
import Nx.Defn
- defn loss(y_true, %{reconstruction: reconstruction, mu_sigma: mu_sigma}) do
- mu = mu_sigma[[0..-1//1, 1, 0..-1//1]]
- sigma = mu_sigma[[0..-1//1, 2, 0..-1//1]]
- kld = Nx.sum(-Nx.log(sigma) - 0.5 + Nx.multiply(sigma, sigma) + Nx.multiply(mu, mu))
- kld * 0.1 + Axon.Losses.mean_squared_error(y_true, reconstruction, reduction: :sum)
- end
-end
With all our pieces ready, we can pretty much use the same training loop as we did earlier. The only modifications needed are to account for the fact that the model outputs a map with two values instead of a single tensor and telling the trainer to use our custom loss.
model = Vae.autoencoder()
+ defn loss(y_true, %{reconstruction: reconstruction, mu_sigma: mu_sigma}) do
+ mu = mu_sigma[[0..-1//1, 1, 0..-1//1]]
+ sigma = mu_sigma[[0..-1//1, 2, 0..-1//1]]
+ kld = Nx.sum(-Nx.log(sigma) - 0.5 + Nx.multiply(sigma, sigma) + Nx.multiply(mu, mu))
+ kld * 0.1 + Axon.Losses.mean_squared_error(y_true, reconstruction, reduction: :sum)
+ end
+end
With all our pieces ready, we can pretty much use the same training loop as we did earlier. The only modifications needed are to account for the fact that the model outputs a map with two values instead of a single tensor and telling the trainer to use our custom loss.
model = Vae.autoencoder()
# A helper function to display the input and output side by side
-combined_input_output = fn params, image_index ->
- test_image = test_images[[images: image_index]]
- %{reconstruction: reconstructed_image} = Axon.predict(model, params, test_image)
- reconstructed_image = reconstructed_image |> Nx.squeeze(axes: [0])
- Nx.concatenate([test_image, reconstructed_image], axis: :width)
-end
+combined_input_output = fn params, image_index ->
+ test_image = test_images[[images: image_index]]
+ %{reconstruction: reconstructed_image} = Axon.predict(model, params, test_image)
+ reconstructed_image = reconstructed_image |> Nx.squeeze(axes: [0])
+ Nx.concatenate([test_image, reconstructed_image], axis: :width)
+end
-frame = Kino.Frame.new() |> Kino.render()
+frame = Kino.Frame.new() |> Kino.render()
-render_example_handler = fn state ->
+render_example_handler = fn state ->
# state.step_state[:model_state] contains the model params when this event is fired
- params = state.step_state[:model_state]
- image_index = Enum.random(0..(Nx.axis_size(test_images, :images) - 1))
- image = combined_input_output.(params, image_index) |> Data.image_to_kino(200, 400)
- Kino.Frame.render(frame, image)
- Kino.Frame.append(frame, "Epoch: #{state.epoch}, Iteration: #{state.iteration}")
- {:continue, state}
-end
+ params = state.step_state[:model_state]
+ image_index = Enum.random(0..(Nx.axis_size(test_images, :images) - 1))
+ image = combined_input_output.(params, image_index) |> Data.image_to_kino(200, 400)
+ Kino.Frame.render(frame, image)
+ Kino.Frame.append(frame, "Epoch: #{state.epoch}, Iteration: #{state.iteration}")
+ {:continue, state}
+end
params =
model
- |> Axon.Loop.trainer(&CustomLoss.loss/2, Axon.Optimizers.adam(0.001))
- |> KinoAxon.kino_early_stop()
- |> Axon.Loop.handle(:epoch_completed, render_example_handler)
- |> Axon.Loop.validate(model, test_data)
- |> KinoAxon.plot_losses()
- |> Axon.Loop.run(train_data, %{}, epochs: 40, compiler: EXLA)
+ |> Axon.Loop.trainer(&CustomLoss.loss/2, Axon.Optimizers.adam(0.001))
+ |> KinoAxon.kino_early_stop()
+ |> Axon.Loop.handle(:epoch_completed, render_example_handler)
+ |> Axon.Loop.validate(model, test_data)
+ |> KinoAxon.plot_losses()
+ |> Axon.Loop.run(train_data, %{}, epochs: 40, compiler: EXLA)
:ok
Finally, we can try our interpolation again:
num_steps = 100
# Get our latents, image at index 0 is our starting point
# index 1 is where we'll end
-latents = Axon.predict(Vae.encoder(), params, test_images[[images: 0..1]])
+latents = Axon.predict(Vae.encoder(), params, test_images[[images: 0..1]])
# Latents is a {2, 10} tensor
# The step we'll add to our latent to move it towards image[1]
-step = Nx.subtract(latents[1], latents[0]) |> Nx.divide(num_steps)
+step = Nx.subtract(latents[1], latents[0]) |> Nx.divide(num_steps)
# We can make a batch of all our new latents
-new_latents = Nx.multiply(Nx.iota({num_steps + 1, 1}), step) |> Nx.add(latents[0])
+new_latents = Nx.multiply(Nx.iota({num_steps + 1, 1}), step) |> Nx.add(latents[0])
-decoder = Axon.input("latent", shape: {nil, 10}) |> Vae.decoder()
+decoder = Axon.input("latent", shape: {nil, 10}) |> Vae.decoder()
-reconstructed_images = Axon.predict(decoder, params, new_latents)
+reconstructed_images = Axon.predict(decoder, params, new_latents)
reconstructed_images =
- Nx.reshape(
+ Nx.reshape(
reconstructed_images,
- Nx.shape(reconstructed_images),
- names: [:images, :channels, :height, :width]
- )
-
-Stream.interval(div(5000, num_steps))
-|> Stream.take(num_steps + 1)
-|> Kino.animate(fn i ->
- Data.image_to_kino(reconstructed_images[i])
-end)
Did you notice the difference? Every step in our interpolation looks similar to items in our dataset! This is the benefit of the VAE: we can generate new items by using random latents. In contrast, in the simple autoencoder, for the most part only latents we got from our encoder were likely to produce sensible outputs.
+ Nx.shape(reconstructed_images),
+ names: [:images, :channels, :height, :width]
+ )
+
+Stream.interval(div(5000, num_steps))
+|> Stream.take(num_steps + 1)
+|> Kino.animate(fn i ->
+ Data.image_to_kino(reconstructed_images[i])
+end)
Did you notice the difference? Every step in our interpolation looks similar to items in our dataset! This is the benefit of the VAE: we can generate new items by using random latents. In contrast, in the simple autoencoder, for the most part only latents we got from our encoder were likely to produce sensible outputs.
diff --git a/horses_or_humans.html b/horses_or_humans.html
index 8e1e4913..be9c688c 100644
--- a/horses_or_humans.html
+++ b/horses_or_humans.html
@@ -115,17 +115,17 @@
-Mix.install([
- {:axon, "~> 0.3.0"},
- {:nx, "~> 0.4.0", sparse: "nx", override: true},
- {:exla, "~> 0.4.0", sparse: "exla", override: true},
- {:stb_image, "~> 0.5.2"},
- {:req, "~> 0.3.1"},
- {:kino, "~> 0.7.0"}
-])
-
-Nx.global_default_backend(EXLA.Backend)
-Nx.Defn.global_default_options(compiler: EXLA)
+Mix.install([
+ {:axon, "~> 0.3.0"},
+ {:nx, "~> 0.4.0", sparse: "nx", override: true},
+ {:exla, "~> 0.4.0", sparse: "exla", override: true},
+ {:stb_image, "~> 0.5.2"},
+ {:req, "~> 0.3.1"},
+ {:kino, "~> 0.7.0"}
+])
+
+Nx.global_default_backend(EXLA.Backend)
+Nx.Defn.global_default_options(compiler: EXLA)
introduction
@@ -137,151 +137,151 @@
Loading the data
-
We will be using the Horses or Humans Dataset. The dataset is available as a ZIP with image files, we will download it using req
. Conveniently, req
will unzip the files for us, we just need to convert the filenames from strings.
%{body: files} =
- Req.get!("https://storage.googleapis.com/laurencemoroney-blog.appspot.com/horse-or-human.zip")
+We will be using the Horses or Humans Dataset. The dataset is available as a ZIP with image files, we will download it using req
. Conveniently, req
will unzip the files for us, we just need to convert the filenames from strings.
%{body: files} =
+ Req.get!("https://storage.googleapis.com/laurencemoroney-blog.appspot.com/horse-or-human.zip")
-files = for {name, binary} <- files, do: {List.to_string(name), binary}
+
files = for {name, binary} <- files, do: {List.to_string(name), binary}
note-on-batching
Note on batching
We need to know how many images to include in a batch. A batch is a group of images to load into the GPU at a time. If the batch size is too big for your GPU, it will run out of memory, in such case you can reduce the batch size. It is generally optimal to utilize almost all of the GPU memory during training. It will take more time to train with a lower batch size.
batch_size = 32
-batches_per_epoch = div(length(files), batch_size)
+batches_per_epoch = div(length(files), batch_size)
a-look-at-the-data
A look at the data
-
We'll have a really quick look at our data. Let's see what we are dealing with:
{name, binary} = Enum.random(files)
-Kino.Markdown.new(name) |> Kino.render()
-Kino.Image.new(binary, :png)
Reevaluate the cell a couple times to view different images. Note that the file names are either horse[N]-[M].png
or human[N]-[M].png
, so we can derive the expected class from that.
While we are at it, look at this beautiful animation:
names_to_animate = ["horse01", "horse05", "human01", "human05"]
+We'll have a really quick look at our data. Let's see what we are dealing with:
{name, binary} = Enum.random(files)
+Kino.Markdown.new(name) |> Kino.render()
+Kino.Image.new(binary, :png)
Reevaluate the cell a couple times to view different images. Note that the file names are either horse[N]-[M].png
or human[N]-[M].png
, so we can derive the expected class from that.
While we are at it, look at this beautiful animation:
names_to_animate = ["horse01", "horse05", "human01", "human05"]
images_to_animate =
- for {name, binary} <- files, Enum.any?(names_to_animate, &String.contains?(name, &1)) do
- Kino.Image.new(binary, :png)
- end
-
-Kino.animate(50, images_to_animate, fn
- _i, [image | images] -> {:cont, image, images}
- _i, [] -> :halt
-end)
How many images are there?
length(files)
How many images will not be used for training? The remainder of the integer division will be ignored.
files
-|> length()
-|> rem(batch_size)
+
for {name, binary} <- files, Enum.any?(names_to_animate, &String.contains?(name, &1)) do
+ Kino.Image.new(binary, :png)
+ end
+
+Kino.animate(50, images_to_animate, fn
+ _i, [image | images] -> {:cont, image, images}
+ _i, [] -> :halt
+end)
How many images are there?
length(files)
How many images will not be used for training? The remainder of the integer division will be ignored.
files
+|> length()
+|> rem(batch_size)
data-processing
Data processing
-First, we need to preprocess the data for our CNN. At the beginning of the process, we chunk images into batches. Then, we use the parse_file/1
function to load images and label them accurately. Finally, we "augment" the input, which means that we normalize data and flip the images along one of the axes. The last procedure helps a neural network to make predictions regardless of the orientation of the image.
defmodule HorsesHumans.DataProcessing do
+First, we need to preprocess the data for our CNN. At the beginning of the process, we chunk images into batches. Then, we use the parse_file/1
function to load images and label them accurately. Finally, we "augment" the input, which means that we normalize data and flip the images along one of the axes. The last procedure helps a neural network to make predictions regardless of the orientation of the image.
defmodule HorsesHumans.DataProcessing do
import Nx.Defn
- def data_stream(files, batch_size) do
+ def data_stream(files, batch_size) do
files
- |> Enum.shuffle()
- |> Stream.chunk_every(batch_size, batch_size, :discard)
- |> Task.async_stream(
- fn batch ->
- {images, labels} = batch |> Enum.map(&parse_file/1) |> Enum.unzip()
- {Nx.stack(images), Nx.stack(labels)}
- end,
+ |> Enum.shuffle()
+ |> Stream.chunk_every(batch_size, batch_size, :discard)
+ |> Task.async_stream(
+ fn batch ->
+ {images, labels} = batch |> Enum.map(&parse_file/1) |> Enum.unzip()
+ {Nx.stack(images), Nx.stack(labels)}
+ end,
timeout: :infinity
- )
- |> Stream.map(fn {:ok, {images, labels}} -> {augment(images), labels} end)
- |> Stream.cycle()
- end
+ )
+ |> Stream.map(fn {:ok, {images, labels}} -> {augment(images), labels} end)
+ |> Stream.cycle()
+ end
- defp parse_file({filename, binary}) do
+ defp parse_file({filename, binary}) do
label =
- if String.starts_with?(filename, "horses/"),
- do: Nx.tensor([1, 0], type: {:u, 8}),
- else: Nx.tensor([0, 1], type: {:u, 8})
+ if String.starts_with?(filename, "horses/"),
+ do: Nx.tensor([1, 0], type: {:u, 8}),
+ else: Nx.tensor([0, 1], type: {:u, 8})
- image = binary |> StbImage.read_binary!() |> StbImage.to_nx()
+ image = binary |> StbImage.read_binary!() |> StbImage.to_nx()
- {image, label}
- end
+ {image, label}
+ end
- defnp augment(images) do
+ defnp augment(images) do
# Normalize
images = images / 255.0
# Optional vertical/horizontal flip
- u = Nx.random_uniform({})
+ u = Nx.random_uniform({})
- cond do
+ cond do
u < 0.25 -> images
- u < 0.5 -> Nx.reverse(images, axes: [2])
- u < 0.75 -> Nx.reverse(images, axes: [3])
- true -> Nx.reverse(images, axes: [2, 3])
- end
- end
-end
+
u < 0.5 -> Nx.reverse(images, axes: [2])
+ u < 0.75 -> Nx.reverse(images, axes: [3])
+ true -> Nx.reverse(images, axes: [2, 3])
+ end
+ end
+end
building-the-model
Building the model
The next step is creating our model. In this notebook, we choose the classic Convolutional Neural Network architecture. Let's dive in to the core components of a CNN.
Axon.conv/3
adds a convolutional layer, which is at the core of a CNN. A convolutional layer applies a filter function throughout the image, sliding a window with shape :kernel_size
. As opposed to dense layers, a convolutional layer exploits weight sharing to better model data where locality matters. This feature is a natural fit for images.

Figure 1: A step-by-step visualization of a convolution layer for kernel_size: {3, 3}
Axon.max_pool/2
adds a downscaling operation that takes the maximum value from a subtensor according to :kernel_size
.

Figure 2: Max pooling operation for kernel_size: {2, 2}
Axon.dropout/2
and Axon.spatial_dropout/2
add dropout layers which prevent a neural network from overfitting. Standard dropout drops a given rate of randomly chosen neurons during the training process. On the other hand, spatial dropout gets rid of whole feature maps. The graphical difference between dropout and spatial dropout is presented in a picture below.
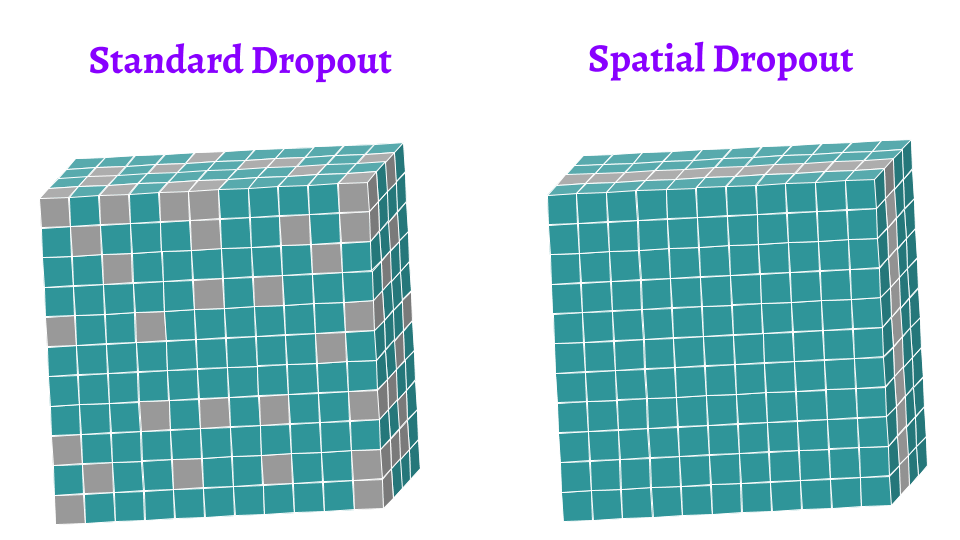
Figure 3: The difference between standard dropout and spatial dropout
Knowing the relevant building blocks, let's build our network! It will have a convolutional part, composed of convolutional and pooling layers, this part should capture the spatial features of an image. Then at the end, we will add a dense layer with 512 neurons fed with all the spatial features, and a final two-neuron layer for as our classification output.
model =
- Axon.input("input", shape: {nil, 300, 300, 4})
- |> Axon.conv(16, kernel_size: {3, 3}, activation: :relu)
- |> Axon.max_pool(kernel_size: {2, 2})
- |> Axon.conv(32, kernel_size: {3, 3}, activation: :relu)
- |> Axon.spatial_dropout(rate: 0.5)
- |> Axon.max_pool(kernel_size: {2, 2})
- |> Axon.conv(64, kernel_size: {3, 3}, activation: :relu)
- |> Axon.spatial_dropout(rate: 0.5)
- |> Axon.max_pool(kernel_size: {2, 2})
- |> Axon.conv(64, kernel_size: {3, 3}, activation: :relu)
- |> Axon.max_pool(kernel_size: {2, 2})
- |> Axon.conv(64, kernel_size: {3, 3}, activation: :relu)
- |> Axon.max_pool(kernel_size: {2, 2})
- |> Axon.flatten()
- |> Axon.dropout(rate: 0.5)
- |> Axon.dense(512, activation: :relu)
- |> Axon.dense(2, activation: :softmax)
+ Axon.input("input", shape: {nil, 300, 300, 4})
+ |> Axon.conv(16, kernel_size: {3, 3}, activation: :relu)
+ |> Axon.max_pool(kernel_size: {2, 2})
+ |> Axon.conv(32, kernel_size: {3, 3}, activation: :relu)
+ |> Axon.spatial_dropout(rate: 0.5)
+ |> Axon.max_pool(kernel_size: {2, 2})
+ |> Axon.conv(64, kernel_size: {3, 3}, activation: :relu)
+ |> Axon.spatial_dropout(rate: 0.5)
+ |> Axon.max_pool(kernel_size: {2, 2})
+ |> Axon.conv(64, kernel_size: {3, 3}, activation: :relu)
+ |> Axon.max_pool(kernel_size: {2, 2})
+ |> Axon.conv(64, kernel_size: {3, 3}, activation: :relu)
+ |> Axon.max_pool(kernel_size: {2, 2})
+ |> Axon.flatten()
+ |> Axon.dropout(rate: 0.5)
+ |> Axon.dense(512, activation: :relu)
+ |> Axon.dense(2, activation: :softmax)
training-the-model
Training the model
-
It's time to train our model. We specify the loss, optimizer and choose accuracy as our metric. We also set log: 1
to frequently update the training progress. We manually specify the number of iterations, such that each epoch goes through all of the baches once.
data = HorsesHumans.DataProcessing.data_stream(files, batch_size)
+It's time to train our model. We specify the loss, optimizer and choose accuracy as our metric. We also set log: 1
to frequently update the training progress. We manually specify the number of iterations, such that each epoch goes through all of the baches once.
data = HorsesHumans.DataProcessing.data_stream(files, batch_size)
-optimizer = Axon.Optimizers.adam(1.0e-4)
+optimizer = Axon.Optimizers.adam(1.0e-4)
params =
model
- |> Axon.Loop.trainer(:categorical_cross_entropy, optimizer, :identity, log: 1)
- |> Axon.Loop.metric(:accuracy)
- |> Axon.Loop.run(data, %{}, epochs: 10, iterations: batches_per_epoch)
+
|> Axon.Loop.trainer(:categorical_cross_entropy, optimizer, :identity, log: 1)
+ |> Axon.Loop.metric(:accuracy)
+ |> Axon.Loop.run(data, %{}, epochs: 10, iterations: batches_per_epoch)
extra-gradient-centralization
Extra: gradient centralization
-We can improve the training by applying gradient centralization. It is a technique with a similar purpose to batch normalization. For each loss gradient, we subtract a mean value to have a gradient with mean equal to zero. This process prevents gradients from exploding.
centralized_optimizer = Axon.Updates.compose(Axon.Updates.centralize(), optimizer)
+We can improve the training by applying gradient centralization. It is a technique with a similar purpose to batch normalization. For each loss gradient, we subtract a mean value to have a gradient with mean equal to zero. This process prevents gradients from exploding.
centralized_optimizer = Axon.Updates.compose(Axon.Updates.centralize(), optimizer)
model
-|> Axon.Loop.trainer(:categorical_cross_entropy, centralized_optimizer, :identity, log: 1)
-|> Axon.Loop.metric(:accuracy)
-|> Axon.Loop.run(data, %{}, epochs: 10, iterations: batches_per_epoch)
+
|> Axon.Loop.trainer(:categorical_cross_entropy, centralized_optimizer, :identity, log: 1)
+|> Axon.Loop.metric(:accuracy)
+|> Axon.Loop.run(data, %{}, epochs: 10, iterations: batches_per_epoch)
inference
Inference
-We can now use our trained model, let's try a couple examples.
{name, binary} = Enum.random(files)
-Kino.Markdown.new(name) |> Kino.render()
-Kino.Image.new(binary, :png) |> Kino.render()
+We can now use our trained model, let's try a couple examples.
{name, binary} = Enum.random(files)
+Kino.Markdown.new(name) |> Kino.render()
+Kino.Image.new(binary, :png) |> Kino.render()
input =
binary
- |> StbImage.read_binary!()
- |> StbImage.to_nx()
- |> Nx.new_axis(0)
- |> Nx.divide(255.0)
+ |> StbImage.read_binary!()
+ |> StbImage.to_nx()
+ |> Nx.new_axis(0)
+ |> Nx.divide(255.0)
-Axon.predict(model, params, input)
Note: the model output refers to the probability that the image presents a horse and a human respectively.
The website from where we loaded the dataset also includes a validation set, in case you want to experiment further!
+Axon.predict(model, params, input)
Note: the model output refers to the probability that the image presents a horse and a human respectively.
The website from where we loaded the dataset also includes a validation set, in case you want to experiment further!
diff --git a/instrumenting_loops_with_metrics.html b/instrumenting_loops_with_metrics.html
index 4a6d42ee..fd8b901d 100644
--- a/instrumenting_loops_with_metrics.html
+++ b/instrumenting_loops_with_metrics.html
@@ -115,205 +115,205 @@
-Mix.install([
- {:axon, github: "elixir-nx/axon"},
- {:nx, "~> 0.3.0", github: "elixir-nx/nx", sparse: "nx", override: true}
-])
:ok
+Mix.install([
+ {:axon, github: "elixir-nx/axon"},
+ {:nx, "~> 0.3.0", github: "elixir-nx/nx", sparse: "nx", override: true}
+])
:ok
adding-metrics-to-training-loops
Adding metrics to training loops
Often times when executing a loop you want to keep track of various metrics such as accuracy or precision. For training loops, Axon by default only tracks loss; however, you can instrument the loop with additional built-in metrics. For example, you might want to track mean-absolute error on top of a mean-squared error loss:
model =
- Axon.input("data")
- |> Axon.dense(8)
- |> Axon.relu()
- |> Axon.dense(4)
- |> Axon.relu()
- |> Axon.dense(1)
+ Axon.input("data")
+ |> Axon.dense(8)
+ |> Axon.relu()
+ |> Axon.dense(4)
+ |> Axon.relu()
+ |> Axon.dense(1)
loop =
model
- |> Axon.Loop.trainer(:mean_squared_error, :sgd)
- |> Axon.Loop.metric(:mean_absolute_error)
#Axon.Loop<
- handlers: %{
- completed: [],
- epoch_completed: [
- {#Function<23.20267452/1 in Axon.Loop.log/5>,
- #Function<5.20267452/1 in Axon.Loop.build_filter_fn/1>}
- ],
- epoch_halted: [],
- epoch_started: [],
- halted: [],
- iteration_completed: [
- {#Function<23.20267452/1 in Axon.Loop.log/5>,
- #Function<3.20267452/1 in Axon.Loop.build_filter_fn/1>}
- ],
- iteration_started: [],
- started: []
- },
- metrics: %{
- "loss" => {#Function<12.6031754/3 in Axon.Metrics.running_average/1>,
- #Function<6.20267452/2 in Axon.Loop.build_loss_fn/1>},
- "mean_absolute_error" => {#Function<12.6031754/3 in Axon.Metrics.running_average/1>,
- :mean_absolute_error}
- },
+ |> Axon.Loop.trainer(:mean_squared_error, :sgd)
+ |> Axon.Loop.metric(:mean_absolute_error)
#Axon.Loop<
+ handlers: %{
+ completed: [],
+ epoch_completed: [
+ {#Function<23.20267452/1 in Axon.Loop.log/5>,
+ #Function<5.20267452/1 in Axon.Loop.build_filter_fn/1>}
+ ],
+ epoch_halted: [],
+ epoch_started: [],
+ halted: [],
+ iteration_completed: [
+ {#Function<23.20267452/1 in Axon.Loop.log/5>,
+ #Function<3.20267452/1 in Axon.Loop.build_filter_fn/1>}
+ ],
+ iteration_started: [],
+ started: []
+ },
+ metrics: %{
+ "loss" => {#Function<12.6031754/3 in Axon.Metrics.running_average/1>,
+ #Function<6.20267452/2 in Axon.Loop.build_loss_fn/1>},
+ "mean_absolute_error" => {#Function<12.6031754/3 in Axon.Metrics.running_average/1>,
+ :mean_absolute_error}
+ },
...
->
When specifying a metric, you can specify an atom which maps to any of the metrics defined in Axon.Metrics
. You can also define custom metrics. For more information on custom metrics, see Writing custom metrics.
When you run a loop with metrics, Axon will aggregate that metric over the course of the loop execution. For training loops, Axon will also report the aggregate metric in the training logs:
train_data =
- Stream.repeatedly(fn ->
- xs = Nx.random_normal({8, 1})
- ys = Nx.sin(xs)
- {xs, ys}
- end)
-
-Axon.Loop.run(loop, train_data, %{}, iterations: 1000)
Epoch: 0, Batch: 1000, loss: 0.0646209 mean_absolute_error: 0.1720028
%{
- "dense_0" => %{
- "bias" => #Nx.Tensor<
- f32[8]
- [-0.2462722808122635, 0.18984302878379822, 0.0016971784643828869, 0.19568635523319244, 0.33571094274520874, 0.07703055441379547, 0.29576605558395386, 0.14511419832706451]
- >,
- "kernel" => #Nx.Tensor<
- f32[1][8]
- [
- [-0.7807592749595642, -0.17303702235221863, 0.43004679679870605, -0.46043306589126587, -0.6577866077423096, 0.7490359544754028, -0.5164405703544617, -0.77418452501297]
- ]
- >
- },
- "dense_1" => %{
- "bias" => #Nx.Tensor<
- f32[4]
- [0.027583779767155647, 0.4279942214488983, -0.10632428526878357, -0.05149337649345398]
- >,
- "kernel" => #Nx.Tensor<
- f32[8][4]
- [
- [-0.5688502192497253, -0.49978527426719666, 0.0660838857293129, 0.30804139375686646],
- [0.21578946709632874, 0.4183472990989685, 0.530754566192627, 0.1742597073316574],
- [-0.17872463166713715, -0.08955764025449753, -0.7048909664154053, 0.053243234753608704],
- [-0.41064000129699707, 0.3491946756839752, 0.3753710091114044, 0.6630277037620544],
- [-0.1781950145959854, 0.5766432881355286, 0.5829672813415527, -0.34879636764526367],
- [-0.026939965784549713, -0.44429031014442444, -0.12619371712207794, 0.0030224998481571674],
- [0.411702424287796, 0.3330642879009247, -0.5062007308006287, -0.0731467455625534],
- [-0.41474586725234985, 0.23881299793720245, 0.3847745358943939, -0.5769480466842651]
- ]
- >
- },
- "dense_2" => %{
- "bias" => #Nx.Tensor<
- f32[1]
- [0.8004998564720154]
- >,
- "kernel" => #Nx.Tensor<
- f32[4][1]
- [
- [-0.40993982553482056],
- [-1.0208697319030762],
- [0.18116380274295807],
- [-0.8320646286010742]
- ]
- >
- }
-}
By default, the metric will have a name which matches the string form of the given metric. You can give metrics semantic meaning by providing an explicit name:
model
-|> Axon.Loop.trainer(:mean_squared_error, :sgd)
-|> Axon.Loop.metric(:mean_absolute_error, "model error")
-|> Axon.Loop.run(train_data, %{}, iterations: 1000)
Epoch: 0, Batch: 1000, loss: 0.0559179 model error: 0.1430965
%{
- "dense_0" => %{
- "bias" => #Nx.Tensor<
- f32[8]
- [-0.2884136438369751, -0.016403740271925926, 0.30548375844955444, 0.2799474000930786, -0.017874717712402344, 0.3168976306915283, -0.10385002940893173, -0.18653006851673126]
- >,
- "kernel" => #Nx.Tensor<
- f32[1][8]
- [
- [-0.44000443816185, 0.6495574712753296, -0.5427255034446716, -0.795007050037384, -0.0035864184610545635, -0.5102121233940125, 0.10152970999479294, -0.3913733959197998]
- ]
- >
- },
- "dense_1" => %{
- "bias" => #Nx.Tensor<
- f32[4]
- [-0.24588409066200256, -0.05674195662140846, -0.08545850962400436, 0.27886852622032166]
- >,
- "kernel" => #Nx.Tensor<
- f32[8][4]
- [
- [0.6334101557731628, -0.44550418853759766, 0.34385600686073303, 0.24886265397071838],
- [-0.5474148988723755, 0.09881290793418884, 0.14616712927818298, 0.8087677359580994],
- [-0.15381869673728943, 0.5322079658508301, -0.6275551915168762, -0.4207017421722412],
- [0.4673740863800049, 0.5706797242164612, 0.44344833493232727, -0.5382705926895142],
- [0.6662552356719971, -0.3875215947628021, -0.5359503626823425, -0.6198058724403381],
- [-0.2842515707015991, 0.2379448264837265, 0.581102728843689, -0.5942302346229553],
- [0.039275627583265305, 0.6341984272003174, -0.10589496046304703, -0.3522306978702545],
- [0.4015151560306549, -0.15162920951843262, -0.3449919819831848, 0.21970798075199127]
- ]
- >
- },
- "dense_2" => %{
- "bias" => #Nx.Tensor<
- f32[1]
- [0.26691529154777527]
- >,
- "kernel" => #Nx.Tensor<
- f32[4][1]
- [
- [0.7088357210159302],
- [-0.9271859526634216],
- [-0.1610293984413147],
- [0.6011591553688049]
- ]
- >
- }
-}
Axon's default aggregation behavior is to aggregate metrics with a running average; however, you can customize this behavior by specifying an explicit accumulation function. Built-in accumulation functions are :running_average
and :running_sum
:
model
-|> Axon.Loop.trainer(:mean_squared_error, :sgd)
-|> Axon.Loop.metric(:mean_absolute_error, "total error", :running_sum)
-|> Axon.Loop.run(train_data, %{}, iterations: 1000)
Epoch: 0, Batch: 1000, loss: 0.0645265 total error: 158.5873566
%{
- "dense_0" => %{
- "bias" => #Nx.Tensor<
- f32[8]
- [0.013307658955454826, 0.08766761422157288, -0.0048030223697423935, -0.07024712860584259, 0.261692613363266, 0.0028863451443612576, -0.12552864849567413, 0.10552618652582169]
- >,
- "kernel" => #Nx.Tensor<
- f32[1][8]
- [
- [-0.1647171825170517, -0.4144238233566284, -0.09969457238912582, -0.6063833832740784, 0.7182243466377258, -0.3485015034675598, -0.29005324840545654, -0.5282242298126221]
- ]
- >
- },
- "dense_1" => %{
- "bias" => #Nx.Tensor<
- f32[4]
- [0.021465059369802475, -0.16003911197185516, 0.6696521043777466, -0.15482725203037262]
- >,
- "kernel" => #Nx.Tensor<
- f32[8][4]
- [
- [0.3359515964984894, -0.21561087667942047, -0.48400720953941345, -0.3186679184436798],
- [-0.08509980887174606, -0.031951334327459335, -0.6084564924240112, -0.39506790041923523],
- [0.003889488521963358, -0.12886928021907806, 0.5679722428321838, 0.22699925303459167],
- [-0.315458744764328, 0.5626247525215149, -0.4241454303264618, -0.11212264746427536],
- [0.6759291291236877, -0.6508319973945618, 0.3511318564414978, 0.17946019768714905],
- [-0.7148906588554382, 0.45404312014579773, 0.4150676727294922, 0.33603984117507935],
- [0.398037314414978, 0.5080180764198303, 0.6770725250244141, -0.5274750590324402],
- [0.5072763562202454, -0.7351003289222717, -0.583225429058075, -0.2974703013896942]
- ]
- >
- },
- "dense_2" => %{
- "bias" => #Nx.Tensor<
- f32[1]
- [-0.8310347199440002]
- >,
- "kernel" => #Nx.Tensor<
- f32[4][1]
- [
- [0.28011587262153625],
- [0.542819082736969],
- [1.2814348936080933],
- [-0.5193246603012085]
- ]
- >
- }
-}
+>When specifying a metric, you can specify an atom which maps to any of the metrics defined in Axon.Metrics
. You can also define custom metrics. For more information on custom metrics, see Writing custom metrics.
When you run a loop with metrics, Axon will aggregate that metric over the course of the loop execution. For training loops, Axon will also report the aggregate metric in the training logs:
train_data =
+ Stream.repeatedly(fn ->
+ xs = Nx.random_normal({8, 1})
+ ys = Nx.sin(xs)
+ {xs, ys}
+ end)
+
+Axon.Loop.run(loop, train_data, %{}, iterations: 1000)
Epoch: 0, Batch: 1000, loss: 0.0646209 mean_absolute_error: 0.1720028
%{
+ "dense_0" => %{
+ "bias" => #Nx.Tensor<
+ f32[8]
+ [-0.2462722808122635, 0.18984302878379822, 0.0016971784643828869, 0.19568635523319244, 0.33571094274520874, 0.07703055441379547, 0.29576605558395386, 0.14511419832706451]
+ >,
+ "kernel" => #Nx.Tensor<
+ f32[1][8]
+ [
+ [-0.7807592749595642, -0.17303702235221863, 0.43004679679870605, -0.46043306589126587, -0.6577866077423096, 0.7490359544754028, -0.5164405703544617, -0.77418452501297]
+ ]
+ >
+ },
+ "dense_1" => %{
+ "bias" => #Nx.Tensor<
+ f32[4]
+ [0.027583779767155647, 0.4279942214488983, -0.10632428526878357, -0.05149337649345398]
+ >,
+ "kernel" => #Nx.Tensor<
+ f32[8][4]
+ [
+ [-0.5688502192497253, -0.49978527426719666, 0.0660838857293129, 0.30804139375686646],
+ [0.21578946709632874, 0.4183472990989685, 0.530754566192627, 0.1742597073316574],
+ [-0.17872463166713715, -0.08955764025449753, -0.7048909664154053, 0.053243234753608704],
+ [-0.41064000129699707, 0.3491946756839752, 0.3753710091114044, 0.6630277037620544],
+ [-0.1781950145959854, 0.5766432881355286, 0.5829672813415527, -0.34879636764526367],
+ [-0.026939965784549713, -0.44429031014442444, -0.12619371712207794, 0.0030224998481571674],
+ [0.411702424287796, 0.3330642879009247, -0.5062007308006287, -0.0731467455625534],
+ [-0.41474586725234985, 0.23881299793720245, 0.3847745358943939, -0.5769480466842651]
+ ]
+ >
+ },
+ "dense_2" => %{
+ "bias" => #Nx.Tensor<
+ f32[1]
+ [0.8004998564720154]
+ >,
+ "kernel" => #Nx.Tensor<
+ f32[4][1]
+ [
+ [-0.40993982553482056],
+ [-1.0208697319030762],
+ [0.18116380274295807],
+ [-0.8320646286010742]
+ ]
+ >
+ }
+}
By default, the metric will have a name which matches the string form of the given metric. You can give metrics semantic meaning by providing an explicit name:
model
+|> Axon.Loop.trainer(:mean_squared_error, :sgd)
+|> Axon.Loop.metric(:mean_absolute_error, "model error")
+|> Axon.Loop.run(train_data, %{}, iterations: 1000)
Epoch: 0, Batch: 1000, loss: 0.0559179 model error: 0.1430965
%{
+ "dense_0" => %{
+ "bias" => #Nx.Tensor<
+ f32[8]
+ [-0.2884136438369751, -0.016403740271925926, 0.30548375844955444, 0.2799474000930786, -0.017874717712402344, 0.3168976306915283, -0.10385002940893173, -0.18653006851673126]
+ >,
+ "kernel" => #Nx.Tensor<
+ f32[1][8]
+ [
+ [-0.44000443816185, 0.6495574712753296, -0.5427255034446716, -0.795007050037384, -0.0035864184610545635, -0.5102121233940125, 0.10152970999479294, -0.3913733959197998]
+ ]
+ >
+ },
+ "dense_1" => %{
+ "bias" => #Nx.Tensor<
+ f32[4]
+ [-0.24588409066200256, -0.05674195662140846, -0.08545850962400436, 0.27886852622032166]
+ >,
+ "kernel" => #Nx.Tensor<
+ f32[8][4]
+ [
+ [0.6334101557731628, -0.44550418853759766, 0.34385600686073303, 0.24886265397071838],
+ [-0.5474148988723755, 0.09881290793418884, 0.14616712927818298, 0.8087677359580994],
+ [-0.15381869673728943, 0.5322079658508301, -0.6275551915168762, -0.4207017421722412],
+ [0.4673740863800049, 0.5706797242164612, 0.44344833493232727, -0.5382705926895142],
+ [0.6662552356719971, -0.3875215947628021, -0.5359503626823425, -0.6198058724403381],
+ [-0.2842515707015991, 0.2379448264837265, 0.581102728843689, -0.5942302346229553],
+ [0.039275627583265305, 0.6341984272003174, -0.10589496046304703, -0.3522306978702545],
+ [0.4015151560306549, -0.15162920951843262, -0.3449919819831848, 0.21970798075199127]
+ ]
+ >
+ },
+ "dense_2" => %{
+ "bias" => #Nx.Tensor<
+ f32[1]
+ [0.26691529154777527]
+ >,
+ "kernel" => #Nx.Tensor<
+ f32[4][1]
+ [
+ [0.7088357210159302],
+ [-0.9271859526634216],
+ [-0.1610293984413147],
+ [0.6011591553688049]
+ ]
+ >
+ }
+}
Axon's default aggregation behavior is to aggregate metrics with a running average; however, you can customize this behavior by specifying an explicit accumulation function. Built-in accumulation functions are :running_average
and :running_sum
:
model
+|> Axon.Loop.trainer(:mean_squared_error, :sgd)
+|> Axon.Loop.metric(:mean_absolute_error, "total error", :running_sum)
+|> Axon.Loop.run(train_data, %{}, iterations: 1000)
Epoch: 0, Batch: 1000, loss: 0.0645265 total error: 158.5873566
%{
+ "dense_0" => %{
+ "bias" => #Nx.Tensor<
+ f32[8]
+ [0.013307658955454826, 0.08766761422157288, -0.0048030223697423935, -0.07024712860584259, 0.261692613363266, 0.0028863451443612576, -0.12552864849567413, 0.10552618652582169]
+ >,
+ "kernel" => #Nx.Tensor<
+ f32[1][8]
+ [
+ [-0.1647171825170517, -0.4144238233566284, -0.09969457238912582, -0.6063833832740784, 0.7182243466377258, -0.3485015034675598, -0.29005324840545654, -0.5282242298126221]
+ ]
+ >
+ },
+ "dense_1" => %{
+ "bias" => #Nx.Tensor<
+ f32[4]
+ [0.021465059369802475, -0.16003911197185516, 0.6696521043777466, -0.15482725203037262]
+ >,
+ "kernel" => #Nx.Tensor<
+ f32[8][4]
+ [
+ [0.3359515964984894, -0.21561087667942047, -0.48400720953941345, -0.3186679184436798],
+ [-0.08509980887174606, -0.031951334327459335, -0.6084564924240112, -0.39506790041923523],
+ [0.003889488521963358, -0.12886928021907806, 0.5679722428321838, 0.22699925303459167],
+ [-0.315458744764328, 0.5626247525215149, -0.4241454303264618, -0.11212264746427536],
+ [0.6759291291236877, -0.6508319973945618, 0.3511318564414978, 0.17946019768714905],
+ [-0.7148906588554382, 0.45404312014579773, 0.4150676727294922, 0.33603984117507935],
+ [0.398037314414978, 0.5080180764198303, 0.6770725250244141, -0.5274750590324402],
+ [0.5072763562202454, -0.7351003289222717, -0.583225429058075, -0.2974703013896942]
+ ]
+ >
+ },
+ "dense_2" => %{
+ "bias" => #Nx.Tensor<
+ f32[1]
+ [-0.8310347199440002]
+ >,
+ "kernel" => #Nx.Tensor<
+ f32[4][1]
+ [
+ [0.28011587262153625],
+ [0.542819082736969],
+ [1.2814348936080933],
+ [-0.5193246603012085]
+ ]
+ >
+ }
+}
diff --git a/lstm_generation.html b/lstm_generation.html
index 3cec316b..abd3b621 100644
--- a/lstm_generation.html
+++ b/lstm_generation.html
@@ -115,15 +115,15 @@
-Mix.install([
- {:axon, "~> 0.3.0"},
- {:nx, "~> 0.4.0", override: true},
- {:exla, "~> 0.4.0"},
- {:req, "~> 0.3.1"}
-])
-
-Nx.Defn.default_options(compiler: EXLA)
-Nx.global_default_backend(EXLA.Backend)
+Mix.install([
+ {:axon, "~> 0.3.0"},
+ {:nx, "~> 0.4.0", override: true},
+ {:exla, "~> 0.4.0"},
+ {:req, "~> 0.3.1"}
+])
+
+Nx.Defn.default_options(compiler: EXLA)
+Nx.global_default_backend(EXLA.Backend)
introduction
@@ -138,43 +138,43 @@
Using Project Gutenburg we can download a text books that are no longer protected under copywrite, so we can experiment with them.
The one that we will use for this experiment is Alice's Adventures in Wonderland by Lewis Carroll. You can choose any other text or book that you like for this experiment.
# Change the URL if you'd like to experiment with other books
download_url = "https://www.gutenberg.org/files/11/11-0.txt"
-book_text = Req.get!(download_url).body
First of all, we need to normalize the content of the book. We are only interested in the sequence of English characters, periods and new lines. Also currently we don't care about the capitalization and things like apostrophe so we can remove all other unknown characters and downcase everything. We can use a regular expression for that.
We can also convert the string into a list of characters so we can handle them easier. You will understand exactly why a bit further.
normalized_book_text =
+book_text = Req.get!(download_url).body
First of all, we need to normalize the content of the book. We are only interested in the sequence of English characters, periods and new lines. Also currently we don't care about the capitalization and things like apostrophe so we can remove all other unknown characters and downcase everything. We can use a regular expression for that.
We can also convert the string into a list of characters so we can handle them easier. You will understand exactly why a bit further.
normalized_book_text =
book_text
- |> String.downcase()
- |> String.replace(~r/[^a-z \.\n]/, "")
- |> String.to_charlist()
We converted the text to a list of characters, where each character is a number (specifically, a Unicode code point). Lowercase English characters are represented with numbers between 97 = a
and 122 = z
, a space is 32 = [ ]
, a new line is 10 = \n
and the period is 46 = .
.
So we should have 26 + 3 (= 29) characters in total. Let's see if that's true.
normalized_book_text |> Enum.uniq() |> Enum.count()
Since we want to use this 29 characters as possible values for each input in our neural network, we can re-map them to values between 0 and 28. So each specific neuron will indicate a specific character.
# Extract all then unique characters we have and sort them for clarity
-characters = normalized_book_text |> Enum.uniq() |> Enum.sort()
-characters_count = Enum.count(characters)
+ |> String.downcase()
+ |> String.replace(~r/[^a-z \.\n]/, "")
+ |> String.to_charlist()
We converted the text to a list of characters, where each character is a number (specifically, a Unicode code point). Lowercase English characters are represented with numbers between 97 = a
and 122 = z
, a space is 32 = [ ]
, a new line is 10 = \n
and the period is 46 = .
.
So we should have 26 + 3 (= 29) characters in total. Let's see if that's true.
normalized_book_text |> Enum.uniq() |> Enum.count()
Since we want to use this 29 characters as possible values for each input in our neural network, we can re-map them to values between 0 and 28. So each specific neuron will indicate a specific character.
# Extract all then unique characters we have and sort them for clarity
+characters = normalized_book_text |> Enum.uniq() |> Enum.sort()
+characters_count = Enum.count(characters)
# Create a mapping for every character
-char_to_idx = characters |> Enum.with_index() |> Map.new()
+char_to_idx = characters |> Enum.with_index() |> Map.new()
# And a reverse mapping to convert back to characters
-idx_to_char = characters |> Enum.with_index(&{&2, &1}) |> Map.new()
+idx_to_char = characters |> Enum.with_index(&{&2, &1}) |> Map.new()
-IO.puts("Total book characters: #{Enum.count(normalized_book_text)}")
-IO.puts("Total unique characters: #{characters_count}")
Now we need to create our training and testing data sets. But how?
Our goal is to teach the machine what comes after a sequence of characters (usually). For example given the following sequence "Hello, My name i" the computer should be able to guess that the next character is probably "s".
graph LR;
+IO.puts("Total book characters: #{Enum.count(normalized_book_text)}")
+IO.puts("Total unique characters: #{characters_count}")
Now we need to create our training and testing data sets. But how?
Our goal is to teach the machine what comes after a sequence of characters (usually). For example given the following sequence "Hello, My name i" the computer should be able to guess that the next character is probably "s".
graph LR;
A[Input: Hello my name i]-->NN[Neural Network]-->B[Output: s];
Let's choose an arbitrary sequence length and create a data set from the book text. All we need to do is read X amount of characters from the book as the input and then read 1 more as the designated output.
After doing all that, we also want to convert every character to it's index using the char_to_idx
mapping that we have created before.
Neural networks work best if you scale your inputs and outputs. In this case we are going to scale everything between 0 and 1 by dividing them by the number of unique characters that we have.
And for the final step we will reshape it so we can use the data in our LSTM model.
sequence_length = 100
train_data =
normalized_book_text
- |> Enum.map(&Map.fetch!(char_to_idx, &1))
- |> Enum.chunk_every(sequence_length, 1, :discard)
+ |> Enum.map(&Map.fetch!(char_to_idx, &1))
+ |> Enum.chunk_every(sequence_length, 1, :discard)
# We don't want the last chunk since we don't have a prediction for it.
- |> Enum.drop(-1)
- |> Nx.tensor()
- |> Nx.divide(characters_count)
- |> Nx.reshape({:auto, sequence_length, 1})
For our train results, We will do the same. Drop the first sequence_length
characters and then convert them to the mapping. Additionally, we will do one-hot encoding.
The reason we want to use one-hot encoding is that in our model we don't want to only return a character as the output. We want it to return the probability of each character for the output. This way we can decide if certain probability is good or not or even we can decide between multiple possible outputs or even discard everything if the network is not confident enough.
In Nx, you can achieve this encoding by using this snippet
Nx.tensor([
- [0],
- [1],
- [2]
-])
-|> Nx.equal(Nx.iota({1, 3}))
To sum it up, Here is how we generate the train results.
train_results =
+ |> Enum.drop(-1)
+ |> Nx.tensor()
+ |> Nx.divide(characters_count)
+ |> Nx.reshape({:auto, sequence_length, 1})
For our train results, We will do the same. Drop the first sequence_length
characters and then convert them to the mapping. Additionally, we will do one-hot encoding.
The reason we want to use one-hot encoding is that in our model we don't want to only return a character as the output. We want it to return the probability of each character for the output. This way we can decide if certain probability is good or not or even we can decide between multiple possible outputs or even discard everything if the network is not confident enough.
In Nx, you can achieve this encoding by using this snippet
Nx.tensor([
+ [0],
+ [1],
+ [2]
+])
+|> Nx.equal(Nx.iota({1, 3}))
To sum it up, Here is how we generate the train results.
train_results =
normalized_book_text
- |> Enum.drop(sequence_length)
- |> Enum.map(&Map.fetch!(char_to_idx, &1))
- |> Nx.tensor()
- |> Nx.reshape({:auto, 1})
- |> Nx.equal(Nx.iota({1, characters_count}))
+ |> Enum.drop(sequence_length)
+ |> Enum.map(&Map.fetch!(char_to_idx, &1))
+ |> Nx.tensor()
+ |> Nx.reshape({:auto, 1})
+ |> Nx.equal(Nx.iota({1, characters_count}))
defining-the-model
@@ -183,34 +183,34 @@
# As the input, we expect the sequence_length characters
model =
- Axon.input("input_chars", shape: {nil, sequence_length, 1})
+ Axon.input("input_chars", shape: {nil, sequence_length, 1})
# The LSTM layer of our network
- |> Axon.lstm(256)
+ |> Axon.lstm(256)
# Selecting only the output from the LSTM Layer
- |> then(fn {out, _} -> out end)
+ |> then(fn {out, _} -> out end)
# Since we only want the last sequence in LSTM we will slice it and
# select the last one
- |> Axon.nx(fn t -> t[[0..-1//1, -1]] end)
+ |> Axon.nx(fn t -> t[[0..-1//1, -1]] end)
# 20% dropout so we will not become too dependent on specific neurons
- |> Axon.dropout(rate: 0.2)
+ |> Axon.dropout(rate: 0.2)
# The output layer. One neuron for each character and using softmax,
# as activation so every node represents a probability
- |> Axon.dense(characters_count, activation: :softmax)
+ |> Axon.dense(characters_count, activation: :softmax)
training-the-network
Training the network
To train the network, we will use Axon's Loop API. It is pretty straightforward.
For the loss function we can use categorical cross-entropy since we are dealing with categories (each character) in our output. For the optimizer we can use Adam.
We will train our network for 20 epochs. Note that we are working with a fair amount data, so it may take a long time unless you run it on a GPU.
batch_size = 128
-train_batches = Nx.to_batched(train_data, batch_size)
-result_batches = Nx.to_batched(train_results, batch_size)
+train_batches = Nx.to_batched(train_data, batch_size)
+result_batches = Nx.to_batched(train_results, batch_size)
-IO.puts("Total batches: #{Enum.count(train_batches)}")
+IO.puts("Total batches: #{Enum.count(train_batches)}")
params =
model
- |> Axon.Loop.trainer(:categorical_cross_entropy, Axon.Optimizers.adam(0.001))
- |> Axon.Loop.run(Stream.zip(train_batches, result_batches), %{}, epochs: 20, compiler: EXLA)
+ |> Axon.Loop.trainer(:categorical_cross_entropy, Axon.Optimizers.adam(0.001))
+ |> Axon.Loop.run(Stream.zip(train_batches, result_batches), %{}, epochs: 20, compiler: EXLA)
:ok
@@ -218,32 +218,32 @@
Generating text
-Now we have a trained neural network, so we can start generating text with it! We just need to pass the initial sequence as the input to the network and select the most probable output. Axon.predict/3
will give us the output layer and then using Nx.argmax/1
we get the most confident neuron index, then simply convert that index back to its Unicode representation.
generate_fn = fn model, params, init_seq ->
+Now we have a trained neural network, so we can start generating text with it! We just need to pass the initial sequence as the input to the network and select the most probable output. Axon.predict/3
will give us the output layer and then using Nx.argmax/1
we get the most confident neuron index, then simply convert that index back to its Unicode representation.
generate_fn = fn model, params, init_seq ->
# The initial sequence that we want the network to complete for us.
init_seq =
init_seq
- |> String.trim()
- |> String.downcase()
- |> String.to_charlist()
- |> Enum.map(&Map.fetch!(char_to_idx, &1))
+ |> String.trim()
+ |> String.downcase()
+ |> String.to_charlist()
+ |> Enum.map(&Map.fetch!(char_to_idx, &1))
- Enum.reduce(1..100, init_seq, fn _, seq ->
+ Enum.reduce(1..100, init_seq, fn _, seq ->
init_seq =
seq
- |> Enum.take(-sequence_length)
- |> Nx.tensor()
- |> Nx.divide(characters_count)
- |> Nx.reshape({1, sequence_length, 1})
+ |> Enum.take(-sequence_length)
+ |> Nx.tensor()
+ |> Nx.divide(characters_count)
+ |> Nx.reshape({1, sequence_length, 1})
char =
- Axon.predict(model, params, init_seq)
- |> Nx.argmax()
- |> Nx.to_number()
+ Axon.predict(model, params, init_seq)
+ |> Nx.argmax()
+ |> Nx.to_number()
- seq ++ [char]
- end)
- |> Enum.map(&Map.fetch!(idx_to_char, &1))
-end
+ seq ++ [char]
+ end)
+ |> Enum.map(&Map.fetch!(idx_to_char, &1))
+end
# The initial sequence that we want the network to complete for us.
init_seq = """
@@ -252,34 +252,34 @@
cupboards as she fell past it.
"""
-generate_fn.(model, params, init_seq) |> IO.puts()
+
generate_fn.(model, params, init_seq) |> IO.puts()
multi-lstm-layers
Multi LSTM layers
We can improve our network by stacking multiple LSTM layers together. We just need to change our model and re-train our network.
new_model =
- Axon.input("input_chars", shape: {nil, sequence_length, 1})
- |> Axon.lstm(256)
- |> then(fn {out, _} -> out end)
- |> Axon.dropout(rate: 0.2)
+ Axon.input("input_chars", shape: {nil, sequence_length, 1})
+ |> Axon.lstm(256)
+ |> then(fn {out, _} -> out end)
+ |> Axon.dropout(rate: 0.2)
# This time we will pass all of the `out` to the next lstm layer.
# We just need to slice the last one.
- |> Axon.lstm(256)
- |> then(fn {out, _} -> out end)
- |> Axon.nx(fn x -> x[[0..-1//1, -1]] end)
- |> Axon.dropout(rate: 0.2)
- |> Axon.dense(characters_count, activation: :softmax)
Then we can train the network using the exact same code as before
# Using a smaller batch size in this case will give the network more opportunity to learn
+ |> Axon.lstm(256)
+ |> then(fn {out, _} -> out end)
+ |> Axon.nx(fn x -> x[[0..-1//1, -1]] end)
+ |> Axon.dropout(rate: 0.2)
+ |> Axon.dense(characters_count, activation: :softmax)
Then we can train the network using the exact same code as before
# Using a smaller batch size in this case will give the network more opportunity to learn
batch_size = 64
-train_batches = Nx.to_batched(train_data, batch_size)
-result_batches = Nx.to_batched(train_results, batch_size)
+train_batches = Nx.to_batched(train_data, batch_size)
+result_batches = Nx.to_batched(train_results, batch_size)
-IO.puts("Total batches: #{Enum.count(train_batches)}")
+IO.puts("Total batches: #{Enum.count(train_batches)}")
new_params =
new_model
- |> Axon.Loop.trainer(:categorical_cross_entropy, Axon.Optimizers.adam(0.001))
- |> Axon.Loop.run(Stream.zip(train_batches, result_batches), %{}, epochs: 50, compiler: EXLA)
+ |> Axon.Loop.trainer(:categorical_cross_entropy, Axon.Optimizers.adam(0.001))
+ |> Axon.Loop.run(Stream.zip(train_batches, result_batches), %{}, epochs: 50, compiler: EXLA)
:ok
@@ -287,7 +287,7 @@
Generate text with the new network
-generate_fn.(new_model, new_params, init_seq) |> IO.puts()
As you may see, it improved a lot with this new model and the extensive training. This time it knows about rules like adding a space after period.
+generate_fn.(new_model, new_params, init_seq) |> IO.puts()
As you may see, it improved a lot with this new model and the extensive training. This time it knows about rules like adding a space after period.
references
diff --git a/mnist.html b/mnist.html
index 8aff6dd4..17dd0abf 100644
--- a/mnist.html
+++ b/mnist.html
@@ -115,12 +115,12 @@
-Mix.install([
- {:axon, "~> 0.3.0"},
- {:nx, "~> 0.4.0", override: true},
- {:exla, "~> 0.4.0"},
- {:req, "~> 0.3.1"}
-])
+Mix.install([
+ {:axon, "~> 0.3.0"},
+ {:nx, "~> 0.4.0", override: true},
+ {:exla, "~> 0.4.0"},
+ {:req, "~> 0.3.1"}
+])
introduction
@@ -133,30 +133,30 @@
Retrieving and exploring the dataset
The MNIST dataset is available for free online. Using Req
we'll download both training images and training labels. Both train_images
and train_labels
are compressed binary data. Fortunately, Req
takes care of the decompression for us.
You can read more about the format of the ubyte files here. Each file starts with a magic number and some metadata. We can use binary pattern matching to extract the information we want. In this case we extract the raw binary images and labels.
base_url = "https://storage.googleapis.com/cvdf-datasets/mnist/"
-%{body: train_images} = Req.get!(base_url <> "train-images-idx3-ubyte.gz")
-%{body: train_labels} = Req.get!(base_url <> "train-labels-idx1-ubyte.gz")
+%{body: train_images} = Req.get!(base_url <> "train-images-idx3-ubyte.gz")
+%{body: train_labels} = Req.get!(base_url <> "train-labels-idx1-ubyte.gz")
-<<_::32, n_images::32, n_rows::32, n_cols::32, images::binary>> = train_images
-<<_::32, n_labels::32, labels::binary>> = train_labels
We can easily read that binary data into a tensor using Nx.from_binary/2
. Nx.from_binary/2
expects a raw binary and a data type. In this case, both images and labels are stored as unsigned 8-bit integers. We can start by parsing our images:
images =
+<<_::32, n_images::32, n_rows::32, n_cols::32, images::binary>> = train_images
+<<_::32, n_labels::32, labels::binary>> = train_labels
We can easily read that binary data into a tensor using Nx.from_binary/2
. Nx.from_binary/2
expects a raw binary and a data type. In this case, both images and labels are stored as unsigned 8-bit integers. We can start by parsing our images:
images =
images
- |> Nx.from_binary({:u, 8})
- |> Nx.reshape({n_images, 1, n_rows, n_cols}, names: [:images, :channels, :height, :width])
- |> Nx.divide(255)
Nx.from_binary/2
returns a flat tensor. Using Nx.reshape/3
we can manipulate this flat tensor into meaningful dimensions. Notice we also normalized the tensor by dividing the input data by 255. This squeezes the data between 0 and 1 which often leads to better behavior when training models. Now, let's see what these images look like:
images[[images: 0..4]] |> Nx.to_heatmap()
In the reshape operation above, we give each dimension of the tensor a name. This makes it much easier to do things like slicing, and helps make your code easier to understand. Here we slice the images
dimension of the images tensor to obtain the first 5 training images. Then, we convert them to a heatmap for easy visualization.
It's common to train neural networks in batches (actually correctly called minibatches, but you'll see batch and minibatch used interchangeably). We can "batch" our images into batches of 32 like this:
images = Nx.to_batched(images, 32)
Now, we'll need to get our labels into batches as well, but first we need to one-hot encode the labels. One-hot encoding converts input data from labels such as 3
, 5
, 7
, etc. into vectors of 0's and a single 1 at the correct labels index. As an example, a label of: 3
gets converted to: [0, 0, 0, 1, 0, 0, 0, 0, 0, 0]
.
targets =
+ |> Nx.from_binary({:u, 8})
+ |> Nx.reshape({n_images, 1, n_rows, n_cols}, names: [:images, :channels, :height, :width])
+ |> Nx.divide(255)
Nx.from_binary/2
returns a flat tensor. Using Nx.reshape/3
we can manipulate this flat tensor into meaningful dimensions. Notice we also normalized the tensor by dividing the input data by 255. This squeezes the data between 0 and 1 which often leads to better behavior when training models. Now, let's see what these images look like:
images[[images: 0..4]] |> Nx.to_heatmap()
In the reshape operation above, we give each dimension of the tensor a name. This makes it much easier to do things like slicing, and helps make your code easier to understand. Here we slice the images
dimension of the images tensor to obtain the first 5 training images. Then, we convert them to a heatmap for easy visualization.
It's common to train neural networks in batches (actually correctly called minibatches, but you'll see batch and minibatch used interchangeably). We can "batch" our images into batches of 32 like this:
images = Nx.to_batched(images, 32)
Now, we'll need to get our labels into batches as well, but first we need to one-hot encode the labels. One-hot encoding converts input data from labels such as 3
, 5
, 7
, etc. into vectors of 0's and a single 1 at the correct labels index. As an example, a label of: 3
gets converted to: [0, 0, 0, 1, 0, 0, 0, 0, 0, 0]
.
targets =
labels
- |> Nx.from_binary({:u, 8})
- |> Nx.new_axis(-1)
- |> Nx.equal(Nx.tensor(Enum.to_list(0..9)))
- |> Nx.to_batched(32)
+ |> Nx.from_binary({:u, 8})
+ |> Nx.new_axis(-1)
+ |> Nx.equal(Nx.tensor(Enum.to_list(0..9)))
+ |> Nx.to_batched(32)
defining-the-model
Defining the model
Let's start by defining a simple model:
model =
- Axon.input("input", shape: {nil, 1, 28, 28})
- |> Axon.flatten()
- |> Axon.dense(128, activation: :relu)
- |> Axon.dense(10, activation: :softmax)
All Axon
models start with an input layer to tell subsequent layers what shapes to expect. We then use Axon.flatten/2
which flattens the previous layer by squeezing all dimensions but the first dimension into a single dimension. Our model consists of 2 fully connected layers with 128 and 10 units respectively. The first layer uses :relu
activation which returns max(0, input)
element-wise. The final layer uses :softmax
activation to return a probability distribution over the 10 labels [0 - 9].
+ Axon.input("input", shape: {nil, 1, 28, 28})
+ |> Axon.flatten()
+ |> Axon.dense(128, activation: :relu)
+ |> Axon.dense(10, activation: :softmax)
All Axon
models start with an input layer to tell subsequent layers what shapes to expect. We then use Axon.flatten/2
which flattens the previous layer by squeezing all dimensions but the first dimension into a single dimension. Our model consists of 2 fully connected layers with 128 and 10 units respectively. The first layer uses :relu
activation which returns max(0, input)
element-wise. The final layer uses :softmax
activation to return a probability distribution over the 10 labels [0 - 9].
training
@@ -164,18 +164,18 @@
In Axon we express the task of training using a declarative loop API. First, we need to specify a loss function and optimizer, there are many built-in variants to choose from. In this example, we'll use categorical cross-entropy and the Adam optimizer. We will also keep track of the accuracy metric. Finally, we run training loop passing our batched images and labels. We'll train for 10 epochs using the EXLA
compiler.
params =
model
- |> Axon.Loop.trainer(:categorical_cross_entropy, :adam)
- |> Axon.Loop.metric(:accuracy, "Accuracy")
- |> Axon.Loop.run(Stream.zip(images, targets), %{}, epochs: 10, compiler: EXLA)
+ |> Axon.Loop.trainer(:categorical_cross_entropy, :adam)
+ |> Axon.Loop.metric(:accuracy, "Accuracy")
+ |> Axon.Loop.run(Stream.zip(images, targets), %{}, epochs: 10, compiler: EXLA)
prediction
Prediction
Now that we have the parameters from the training step, we can use them for predictions.
-For this the Axon.predict
can be used.
first_batch = Enum.at(images, 0)
+For this the Axon.predict
can be used.first_batch = Enum.at(images, 0)
-output = Axon.predict(model, params, first_batch)
For each image, the model outputs probability distribution. This informs us how certain the model is about its prediction. Let's see the most probable digit for each image:
Nx.argmax(output, axis: 1)
If you look at the original images and you will see the predictions match the data!
+output = Axon.predict(model, params, first_batch)
For each image, the model outputs probability distribution. This informs us how certain the model is about its prediction. Let's see the most probable digit for each image:
Nx.argmax(output, axis: 1)
If you look at the original images and you will see the predictions match the data!
diff --git a/mnist_autoencoder_using_kino.html b/mnist_autoencoder_using_kino.html
index 70dbbf6d..206b76cc 100644
--- a/mnist_autoencoder_using_kino.html
+++ b/mnist_autoencoder_using_kino.html
@@ -115,16 +115,16 @@
-Mix.install([
- {:exla, "~> 0.4.0"},
- {:nx, "~> 0.4.0", override: true},
- {:axon, "~> 0.3.0"},
- {:req, "~> 0.3.1"},
- {:kino, "~> 0.7.0"},
- {:scidata, "~> 0.1.9"},
- {:stb_image, "~> 0.5.2"},
- {:table_rex, "~> 3.1.1"}
-])
+Mix.install([
+ {:exla, "~> 0.4.0"},
+ {:nx, "~> 0.4.0", override: true},
+ {:axon, "~> 0.3.0"},
+ {:req, "~> 0.3.1"},
+ {:kino, "~> 0.7.0"},
+ {:scidata, "~> 0.1.9"},
+ {:stb_image, "~> 0.5.2"},
+ {:table_rex, "~> 3.1.1"}
+])
introduction
@@ -137,26 +137,26 @@
Data loading
An autoencoder learns to recreate data it's seen in the dataset. For this notebook, we're going to try something simple: generating images of digits using the MNIST digit recognition dataset.
Following along with the Fashion MNIST Autoencoder example, we'll use Scidata to download the MNIST dataset and then preprocess the data.
# We're not going to use the labels so we'll ignore them
-{train_images, _train_labels} = Scidata.MNIST.download()
-{train_images_binary, type, shape} = train_images
The shape
tells us we have 60,000 images with a single channel of size 28x28.
According to the MNIST website:
Pixels are organized row-wise. Pixel values are 0 to 255. 0 means background (white), 255 means foreground (black).
Let's preprocess and normalize the data accordingly.
train_images =
+{train_images, _train_labels} = Scidata.MNIST.download()
+{train_images_binary, type, shape} = train_images
The shape
tells us we have 60,000 images with a single channel of size 28x28.
According to the MNIST website:
Pixels are organized row-wise. Pixel values are 0 to 255. 0 means background (white), 255 means foreground (black).
Let's preprocess and normalize the data accordingly.
train_images =
train_images_binary
- |> Nx.from_binary(type)
+ |> Nx.from_binary(type)
# Since pixels are organized row-wise, reshape into rows x columns
- |> Nx.reshape(shape, names: [:images, :channels, :height, :width])
+ |> Nx.reshape(shape, names: [:images, :channels, :height, :width])
# Normalize the pixel values to be between 0 and 1
- |> Nx.divide(255)
# Make sure they look like numbers
-train_images[[images: 0..2]] |> Nx.to_heatmap()
That looks right! Let's repeat the process for the test set.
{test_images, _train_labels} = Scidata.MNIST.download_test()
-{test_images_binary, type, shape} = test_images
+ |> Nx.divide(255)
# Make sure they look like numbers
+train_images[[images: 0..2]] |> Nx.to_heatmap()
That looks right! Let's repeat the process for the test set.
{test_images, _train_labels} = Scidata.MNIST.download_test()
+{test_images_binary, type, shape} = test_images
test_images =
test_images_binary
- |> Nx.from_binary(type)
+ |> Nx.from_binary(type)
# Since pixels are organized row-wise, reshape into rows x columns
- |> Nx.reshape(shape, names: [:images, :channels, :height, :width])
+ |> Nx.reshape(shape, names: [:images, :channels, :height, :width])
# Normalize the pixel values to be between 0 and 1
- |> Nx.divide(255)
+ |> Nx.divide(255)
-test_images[[images: 0..2]] |> Nx.to_heatmap()
+test_images[[images: 0..2]] |> Nx.to_heatmap()
building-the-model
@@ -169,79 +169,79 @@
The model
model =
- Axon.input("image", shape: {nil, 1, 28, 28})
+ Axon.input("image", shape: {nil, 1, 28, 28})
# This is now 28*28*1 = 784
- |> Axon.flatten()
+ |> Axon.flatten()
# The encoder
- |> Axon.dense(256, activation: :relu)
- |> Axon.dense(128, activation: :relu)
- |> Axon.dense(64, activation: :relu)
+ |> Axon.dense(256, activation: :relu)
+ |> Axon.dense(128, activation: :relu)
+ |> Axon.dense(64, activation: :relu)
# Bottleneck layer
- |> Axon.dense(10, activation: :relu)
+ |> Axon.dense(10, activation: :relu)
# The decoder
- |> Axon.dense(64, activation: :relu)
- |> Axon.dense(128, activation: :relu)
- |> Axon.dense(256, activation: :relu)
- |> Axon.dense(784, activation: :sigmoid)
+ |> Axon.dense(64, activation: :relu)
+ |> Axon.dense(128, activation: :relu)
+ |> Axon.dense(256, activation: :relu)
+ |> Axon.dense(784, activation: :sigmoid)
# Turn it back into a 28x28 single channel image
- |> Axon.reshape({:auto, 1, 28, 28})
+ |> Axon.reshape({:auto, 1, 28, 28})
# We can use Axon.Display to show us what each of the layers would look like
# assuming we send in a batch of 4 images
-Axon.Display.as_table(model, Nx.template({4, 1, 28, 28}, :f32)) |> IO.puts()
Checking our understanding, since the layers are all dense layers, the number of parameters should be input_features * output_features
parameters for the weights + output_features
parameters for the biases for each layer.
This should match the Total Parameters
output from Axon.Display (486298 parameters)
# encoder
-encoder_parameters = 784 * 256 + 256 + (256 * 128 + 128) + (128 * 64 + 64) + (64 * 10 + 10)
-decoder_parameters = 10 * 64 + 64 + (64 * 128 + 128) + (128 * 256 + 256) + (256 * 784 + 784)
+Axon.Display.as_table(model, Nx.template({4, 1, 28, 28}, :f32)) |> IO.puts()
Checking our understanding, since the layers are all dense layers, the number of parameters should be input_features * output_features
parameters for the weights + output_features
parameters for the biases for each layer.
This should match the Total Parameters
output from Axon.Display (486298 parameters)
# encoder
+encoder_parameters = 784 * 256 + 256 + (256 * 128 + 128) + (128 * 64 + 64) + (64 * 10 + 10)
+decoder_parameters = 10 * 64 + 64 + (64 * 128 + 128) + (128 * 256 + 256) + (256 * 784 + 784)
total_parameters = encoder_parameters + decoder_parameters
training
Training
-With the model set up, we can now try to train the model. We'll use MSE loss to compare our reconstruction with the original
We'll create the training input by turning our image list into batches of size 128 and then using the same image as both the input and the target. However, the input image will have noise added to it that the autoencoder will have to remove.
For validation data, we'll use the test set and look at how the autoencoder does at reconstructing the test set to make sure we're not overfitting
The function below adds some noise to the image by adding the image with gaussian noise scaled by a noise factor. We then have to make sure the pixel values are still within the 0..1.0 range.
We have to define this function using defn
so that Nx
can optimize it. If we don't do this, adding noise will take a really long time, making our training loop very slow. See Nx.defn for more details. defn
can only be used in a module so we'll define a little module to contain it.
defmodule Noiser do
+With the model set up, we can now try to train the model. We'll use MSE loss to compare our reconstruction with the original
We'll create the training input by turning our image list into batches of size 128 and then using the same image as both the input and the target. However, the input image will have noise added to it that the autoencoder will have to remove.
For validation data, we'll use the test set and look at how the autoencoder does at reconstructing the test set to make sure we're not overfitting
The function below adds some noise to the image by adding the image with gaussian noise scaled by a noise factor. We then have to make sure the pixel values are still within the 0..1.0 range.
We have to define this function using defn
so that Nx
can optimize it. If we don't do this, adding noise will take a really long time, making our training loop very slow. See Nx.defn for more details. defn
can only be used in a module so we'll define a little module to contain it.
defmodule Noiser do
import Nx.Defn
@noise_factor 0.4
- defn add_noise(images) do
+ defn add_noise(images) do
@noise_factor
- |> Nx.multiply(Nx.random_normal(images))
- |> Nx.add(images)
- |> Nx.clip(0.0, 1.0)
- end
-end
+ |> Nx.multiply(Nx.random_normal(images))
+ |> Nx.add(images)
+ |> Nx.clip(0.0, 1.0)
+ end
+end
-add_noise = Nx.Defn.jit(&Noiser.add_noise/1, compiler: EXLA)
batch_size = 128
+add_noise = Nx.Defn.jit(&Noiser.add_noise/1, compiler: EXLA)
batch_size = 128
# The original image which is the target the network will trying to match
batched_train_images =
train_images
- |> Nx.to_batched(batch_size)
+ |> Nx.to_batched(batch_size)
batched_noisy_train_images =
train_images
- |> Nx.to_batched(batch_size)
+ |> Nx.to_batched(batch_size)
# goes after to_batched so the noise is different every time
- |> Stream.map(add_noise)
+ |> Stream.map(add_noise)
# The noisy image is the input to the network
# and the original image is the target it's trying to match
-train_data = Stream.zip(batched_noisy_train_images, batched_train_images)
+train_data = Stream.zip(batched_noisy_train_images, batched_train_images)
batched_test_images =
test_images
- |> Nx.to_batched(batch_size)
+ |> Nx.to_batched(batch_size)
batched_noisy_test_images =
test_images
- |> Nx.to_batched(batch_size)
- |> Stream.map(add_noise)
+ |> Nx.to_batched(batch_size)
+ |> Stream.map(add_noise)
-test_data = Stream.zip(batched_noisy_test_images, batched_test_images)
Let's see what an element of the input and target look like
{input_batch, target_batch} = Enum.at(train_data, 0)
-{Nx.to_heatmap(input_batch[images: 0]), Nx.to_heatmap(target_batch[images: 0])}
Looks right (and tricky). Let's see how the model does.
params =
+test_data = Stream.zip(batched_noisy_test_images, batched_test_images)
Let's see what an element of the input and target look like
{input_batch, target_batch} = Enum.at(train_data, 0)
+{Nx.to_heatmap(input_batch[images: 0]), Nx.to_heatmap(target_batch[images: 0])}
Looks right (and tricky). Let's see how the model does.
params =
model
- |> Axon.Loop.trainer(:mean_squared_error, Axon.Optimizers.adamw(0.001))
- |> Axon.Loop.validate(model, test_data)
- |> Axon.Loop.run(train_data, %{}, epochs: 20, compiler: EXLA)
+ |> Axon.Loop.trainer(:mean_squared_error, Axon.Optimizers.adamw(0.001))
+ |> Axon.Loop.validate(model, test_data)
+ |> Axon.Loop.run(train_data, %{}, epochs: 20, compiler: EXLA)
:ok
Now that we have a model that theoretically has learned something, we'll see what it's learned by running it on some images from the test set. We'll use Kino to allow us to select the image from the test set to run the model against. To avoid losing the params that took a while to train, we'll create another branch so we can experiment with the params and stop execution when needed without having to retrain.
@@ -250,70 +250,70 @@
Evaluation
A note on branching
By default, everything in Livebook runs sequentially in a single process. Stopping a running cell aborts that process and consequently all its state is lost. A branching section copies everything from its parent and runs in a separate process. Thanks to this isolation, when we stop a cell in a branching section, only the state within that section is gone.
Since we just spent a bunch of time training the model and don't want to lose that memory state as we continue to experiment, we create a branching section. This does add some memory overhead, but it's worth it so we can experiment without fear!
To use Kino
to give us an interactive tool to evaluate the model, we'll create a Kino.Frame
that we can dynamically update. We'll also create a form using Kino.Control
to allow the user to select which image from the test set they'd like to evaluate the model on. Finally Kino.Control.stream
enables us to respond to changes in the user's selection when the user clicks the "Render" button.
We can use Nx.concatenate
to stack the images side by side for a prettier output.
form =
- Kino.Control.form(
- [
- test_image_index: Kino.Input.number("Test Image Index", default: 0)
- ],
+ Kino.Control.form(
+ [
+ test_image_index: Kino.Input.number("Test Image Index", default: 0)
+ ],
submit: "Render"
- )
+ )
-Kino.render(form)
+Kino.render(form)
form
-|> Kino.Control.stream()
-|> Kino.animate(fn %{data: %{test_image_index: image_index}} ->
- test_image = test_images[[images: image_index]] |> add_noise.()
+|> Kino.Control.stream()
+|> Kino.animate(fn %{data: %{test_image_index: image_index}} ->
+ test_image = test_images[[images: image_index]] |> add_noise.()
reconstructed_image =
model
- |> Axon.predict(params, test_image)
+ |> Axon.predict(params, test_image)
# Get rid of the batch dimension
- |> Nx.squeeze(axes: [0])
+ |> Nx.squeeze(axes: [0])
- combined_image = Nx.concatenate([test_image, reconstructed_image], axis: :width)
- Nx.to_heatmap(combined_image)
-end)
That looks pretty good!
Note we used Kino.animate/2
which runs asynchronously so we don't block execution of the rest of the notebook.
+
combined_image = Nx.concatenate([test_image, reconstructed_image], axis: :width)
+ Nx.to_heatmap(combined_image)
+end)
That looks pretty good!
Note we used Kino.animate/2
which runs asynchronously so we don't block execution of the rest of the notebook.
a-better-training-loop
A better training loop
Note that we branch from the "Building a model" section since we only need the model definition for this section and not the previously trained model.
It'd be nice to see how the model improves as it trains. In this section (also a branch since I plan to experiment and don't want to lose the execution state) we'll improve the training loop to use Kino
to show us how it's doing.
Axon.Loop.handle gives us a hook into various points of the training loop. We'll can use it with the :iteration_completed
event to get a copy of the state of the params after some number of completed iterations of the training loop. By using those params to render an image in the test set, we can get a live view of the autoencoder learning to reconstruct its inputs.
# A helper function to display the input and output side by side
-combined_input_output = fn params, image_index ->
- test_image = test_images[[images: image_index]] |> add_noise.()
- reconstructed_image = Axon.predict(model, params, test_image) |> Nx.squeeze(axes: [0])
- Nx.concatenate([test_image, reconstructed_image], axis: :width)
-end
+combined_input_output = fn params, image_index ->
+ test_image = test_images[[images: image_index]] |> add_noise.()
+ reconstructed_image = Axon.predict(model, params, test_image) |> Nx.squeeze(axes: [0])
+ Nx.concatenate([test_image, reconstructed_image], axis: :width)
+end
-Nx.to_heatmap(combined_input_output.(params, 0))
It'd also be nice to have a prettier version of the output. Let's convert the heatmap to a png to make that happen.
image_to_kino = fn image ->
+Nx.to_heatmap(combined_input_output.(params, 0))
It'd also be nice to have a prettier version of the output. Let's convert the heatmap to a png to make that happen.
image_to_kino = fn image ->
image
- |> Nx.multiply(255)
- |> Nx.as_type(:u8)
- |> Nx.transpose(axes: [:height, :width, :channels])
- |> StbImage.from_nx()
- |> StbImage.resize(200, 400)
- |> StbImage.to_binary(:png)
- |> Kino.Image.new(:png)
-end
-
-image_to_kino.(combined_input_output.(params, 0))
Much nicer!
Once again we'll use Kino.Frame
for dynamically updating output:
frame = Kino.Frame.new() |> Kino.render()
-
-render_example_handler = fn state ->
- Kino.Frame.append(frame, "Epoch: #{state.epoch}, Iteration: #{state.iteration}")
+ |> Nx.multiply(255)
+ |> Nx.as_type(:u8)
+ |> Nx.transpose(axes: [:height, :width, :channels])
+ |> StbImage.from_nx()
+ |> StbImage.resize(200, 400)
+ |> StbImage.to_binary(:png)
+ |> Kino.Image.new(:png)
+end
+
+image_to_kino.(combined_input_output.(params, 0))
Much nicer!
Once again we'll use Kino.Frame
for dynamically updating output:
frame = Kino.Frame.new() |> Kino.render()
+
+render_example_handler = fn state ->
+ Kino.Frame.append(frame, "Epoch: #{state.epoch}, Iteration: #{state.iteration}")
# state.step_state[:model_state] contains the model params when this event is fired
- params = state.step_state[:model_state]
- image_index = Enum.random(0..(Nx.axis_size(test_images, :images) - 1))
- image = combined_input_output.(params, image_index) |> image_to_kino.()
- Kino.Frame.append(frame, image)
- {:continue, state}
-end
+ params = state.step_state[:model_state]
+ image_index = Enum.random(0..(Nx.axis_size(test_images, :images) - 1))
+ image = combined_input_output.(params, image_index) |> image_to_kino.()
+ Kino.Frame.append(frame, image)
+ {:continue, state}
+end
params =
model
- |> Axon.Loop.trainer(:mean_squared_error, Axon.Optimizers.adamw(0.001))
- |> Axon.Loop.handle(:iteration_completed, render_example_handler, every: 450)
- |> Axon.Loop.validate(model, test_data)
- |> Axon.Loop.run(train_data, %{}, epochs: 20, compiler: EXLA)
+ |> Axon.Loop.trainer(:mean_squared_error, Axon.Optimizers.adamw(0.001))
+ |> Axon.Loop.handle(:iteration_completed, render_example_handler, every: 450)
+ |> Axon.Loop.validate(model, test_data)
+ |> Axon.Loop.run(train_data, %{}, epochs: 20, compiler: EXLA)
:ok
Awesome! We have a working denoising autoencoder that we can visualize getting better in 20 epochs!
diff --git a/model_hooks.html b/model_hooks.html
index 491459b1..934b9d0b 100644
--- a/model_hooks.html
+++ b/model_hooks.html
@@ -115,304 +115,304 @@
-Mix.install([
- {:axon, github: "elixir-nx/axon"},
- {:nx, "~> 0.3.0", github: "elixir-nx/nx", sparse: "nx", override: true}
-])
:ok
+Mix.install([
+ {:axon, github: "elixir-nx/axon"},
+ {:nx, "~> 0.3.0", github: "elixir-nx/nx", sparse: "nx", override: true}
+])
:ok
creating-models-with-hooks
Creating models with hooks
Sometimes it's useful to inspect or visualize the values of intermediate layers in your model during the forward or backward pass. For example, it's common to visualize the gradients of activation functions to ensure your model is learning in a stable manner. Axon supports this functionality via model hooks.
Model hooks are a means of unidirectional communication with an executing model. Hooks are unidirectional in the sense that you can only receive information from your model, and not send information back.
Hooks are attached per-layer and can execute at 4 different points in model execution: on the pre-forward, forward, or backward pass of the model or during model initialization. You can also configure the same hook to execute on all 3 events. You can attach hooks to models using Axon.attach_hook/3
:
model =
- Axon.input("data")
- |> Axon.dense(8)
- |> Axon.attach_hook(fn val -> IO.inspect(val, label: :dense_forward) end, on: :forward)
- |> Axon.attach_hook(fn val -> IO.inspect(val, label: :dense_init) end, on: :initialize)
- |> Axon.relu()
- |> Axon.attach_hook(fn val -> IO.inspect(val, label: :relu) end, on: :forward)
-
-{init_fn, predict_fn} = Axon.build(model)
-
-input = Nx.iota({2, 4}, type: :f32)
-params = init_fn.(input, %{})
dense_init: %{
- "bias" => #Nx.Tensor<
- f32[8]
- [0.0, 0.0, 0.0, 0.0, 0.0, 0.0, 0.0, 0.0]
- >,
- "kernel" => #Nx.Tensor<
- f32[4][8]
- [
- [-0.40611347556114197, -0.1551232784986496, 0.08485020697116852, -0.6748610734939575, 0.04797258973121643, -0.059523195028305054, 0.4092640280723572, 0.1300794780254364],
- [-0.3551754057407379, 0.3159058094024658, 0.25394684076309204, 0.22510826587677002, 0.2613920271396637, -0.15213526785373688, -0.15744848549365997, -0.46065202355384827],
- [-0.5224899649620056, 0.3639957010746002, -0.19676287472248077, 0.5423932075500488, -0.4722306430339813, 0.26447463035583496, 0.18534891307353973, -0.6442952752113342],
- [-0.5629043579101562, 0.6370815634727478, -0.43325361609458923, 0.5084872245788574, -0.1424017995595932, 0.4865548312664032, -0.5839526057243347, 0.09811079502105713]
- ]
- >
-}
%{
- "dense_0" => %{
- "bias" => #Nx.Tensor<
- f32[8]
- [0.0, 0.0, 0.0, 0.0, 0.0, 0.0, 0.0, 0.0]
- >,
- "kernel" => #Nx.Tensor<
- f32[4][8]
- [
- [-0.40611347556114197, -0.1551232784986496, 0.08485020697116852, -0.6748610734939575, 0.04797258973121643, -0.059523195028305054, 0.4092640280723572, 0.1300794780254364],
- [-0.3551754057407379, 0.3159058094024658, 0.25394684076309204, 0.22510826587677002, 0.2613920271396637, -0.15213526785373688, -0.15744848549365997, -0.46065202355384827],
- [-0.5224899649620056, 0.3639957010746002, -0.19676287472248077, 0.5423932075500488, -0.4722306430339813, 0.26447463035583496, 0.18534891307353973, -0.6442952752113342],
- [-0.5629043579101562, 0.6370815634727478, -0.43325361609458923, 0.5084872245788574, -0.1424017995595932, 0.4865548312664032, -0.5839526057243347, 0.09811079502105713]
- ]
- >
- }
-}
Notice how during initialization the :dense_init
hook fired and inspected the layer's parameters. Now when executing, you'll see outputs for :dense
and :relu
:
predict_fn.(params, input)
dense_forward: #Nx.Tensor<
- f32[2][8]
- [
- [-3.0888683795928955, 2.955142021179199, -1.4393397569656372, 2.8353562355041504, -1.1102746725082397, 1.8364784717559814, -1.538608431816101, -1.454910159111023],
- [-10.475601196289062, 7.602581024169922, -2.604217529296875, 5.239866733551025, -2.331346035003662, 3.993962526321411, -2.125761032104492, -4.961938381195068]
- ]
->
-relu: #Nx.Tensor<
- f32[2][8]
- [
- [0.0, 2.955142021179199, 0.0, 2.8353562355041504, 0.0, 1.8364784717559814, 0.0, 0.0],
- [0.0, 7.602581024169922, 0.0, 5.239866733551025, 0.0, 3.993962526321411, 0.0, 0.0]
- ]
->
#Nx.Tensor<
- f32[2][8]
- [
- [0.0, 2.955142021179199, 0.0, 2.8353562355041504, 0.0, 1.8364784717559814, 0.0, 0.0],
- [0.0, 7.602581024169922, 0.0, 5.239866733551025, 0.0, 3.993962526321411, 0.0, 0.0]
- ]
->
It's important to note that hooks execute in the order they were attached to a layer. If you attach 2 hooks to the same layer which execute different functions on the same event, they will run in order:
model =
- Axon.input("data")
- |> Axon.dense(8)
- |> Axon.attach_hook(fn val -> IO.inspect(val, label: :hook1) end, on: :forward)
- |> Axon.attach_hook(fn val -> IO.inspect(val, label: :hook2) end, on: :forward)
- |> Axon.relu()
-
-{init_fn, predict_fn} = Axon.build(model)
-params = init_fn.(input, %{})
-
-predict_fn.(params, input)
hook1: #Nx.Tensor<
- f32[2][8]
- [
- [1.3320910930633545, 1.712153673171997, -2.0420351028442383, 2.2541849613189697, -3.1382551193237305, -1.2241677045822144, -1.5477651357650757, -0.2126261293888092],
- [2.1975531578063965, 3.722827911376953, -1.6301460266113281, 5.891226768493652, -10.79372787475586, -2.9982359409332275, -6.589874267578125, 1.5387766361236572]
- ]
->
-hook2: #Nx.Tensor<
- f32[2][8]
- [
- [1.3320910930633545, 1.712153673171997, -2.0420351028442383, 2.2541849613189697, -3.1382551193237305, -1.2241677045822144, -1.5477651357650757, -0.2126261293888092],
- [2.1975531578063965, 3.722827911376953, -1.6301460266113281, 5.891226768493652, -10.79372787475586, -2.9982359409332275, -6.589874267578125, 1.5387766361236572]
- ]
->
#Nx.Tensor<
- f32[2][8]
- [
- [1.3320910930633545, 1.712153673171997, 0.0, 2.2541849613189697, 0.0, 0.0, 0.0, 0.0],
- [2.1975531578063965, 3.722827911376953, 0.0, 5.891226768493652, 0.0, 0.0, 0.0, 1.5387766361236572]
- ]
->
Notice that :hook1
fires before :hook2
.
You can also specify a hook to fire on all events:
model =
- Axon.input("data")
- |> Axon.dense(8)
- |> Axon.attach_hook(&IO.inspect/1, on: :all)
- |> Axon.relu()
- |> Axon.dense(1)
-
-{init_fn, predict_fn} = Axon.build(model)
{#Function<136.40088443/2 in Nx.Defn.wrap_arity/2>,
- #Function<136.40088443/2 in Nx.Defn.wrap_arity/2>}
On initialization:
params = init_fn.(input, %{})
%{
- "bias" => #Nx.Tensor<
- f32[8]
- [0.0, 0.0, 0.0, 0.0, 0.0, 0.0, 0.0, 0.0]
- >,
- "kernel" => #Nx.Tensor<
- f32[4][8]
- [
- [0.6784419417381287, 0.175045907497406, 0.010701040737330914, -0.5537784695625305, -0.010694148950278759, 0.7021086812019348, -0.3290281891822815, -0.6818609237670898],
- [-0.6378231644630432, -0.5675055384635925, 0.031453751027584076, 0.4705190360546112, -0.002226108219474554, 0.48611924052238464, 0.5700677037239075, 0.6729928851127625],
- [0.4596043527126312, -0.6557875871658325, -0.07168347388505936, -0.37926459312438965, -0.20766735076904297, 0.11274437606334686, -0.5166378617286682, -0.5115087032318115],
- [-0.30842259526252747, -0.3418923616409302, 0.3374936282634735, 0.6272460222244263, 0.6156857013702393, 0.6739501357078552, -0.09081890434026718, 0.706954836845398]
- ]
- >
-}
%{
- "dense_0" => %{
- "bias" => #Nx.Tensor<
- f32[8]
- [0.0, 0.0, 0.0, 0.0, 0.0, 0.0, 0.0, 0.0]
- >,
- "kernel" => #Nx.Tensor<
- f32[4][8]
- [
- [0.6784419417381287, 0.175045907497406, 0.010701040737330914, -0.5537784695625305, -0.010694148950278759, 0.7021086812019348, -0.3290281891822815, -0.6818609237670898],
- [-0.6378231644630432, -0.5675055384635925, 0.031453751027584076, 0.4705190360546112, -0.002226108219474554, 0.48611924052238464, 0.5700677037239075, 0.6729928851127625],
- [0.4596043527126312, -0.6557875871658325, -0.07168347388505936, -0.37926459312438965, -0.20766735076904297, 0.11274437606334686, -0.5166378617286682, -0.5115087032318115],
- [-0.30842259526252747, -0.3418923616409302, 0.3374936282634735, 0.6272460222244263, 0.6156857013702393, 0.6739501357078552, -0.09081890434026718, 0.706954836845398]
- ]
- >
- },
- "dense_1" => %{
- "bias" => #Nx.Tensor<
- f32[1]
- [0.0]
- >,
- "kernel" => #Nx.Tensor<
- f32[8][1]
- [
- [-0.7136709690093994],
- [-0.16328231990337372],
- [0.08359552919864655],
- [0.07646285742521286],
- [0.7133787274360657],
- [-0.00617210753262043],
- [0.2241944670677185],
- [-0.055933959782123566]
- ]
- >
- }
-}
On pre-forward and forward:
predict_fn.(params, input)
#Nx.Tensor<
- f32[2][4]
- [
- [0.0, 1.0, 2.0, 3.0],
- [4.0, 5.0, 6.0, 7.0]
- ]
->
-#Nx.Tensor<
- f32[2][8]
- [
- [-0.6438822746276855, -2.9047577381134033, 0.9005677103996277, 1.593727946281433, 1.4294962882995605, 2.7334585189819336, -0.7356647253036499, 1.7708399295806885],
- [0.12331989407539368, -8.465315818786621, 2.132427453994751, 2.2526159286499023, 3.0098886489868164, 10.633148193359375, -2.20133376121521, 2.5171523094177246]
- ]
->
-#Nx.Tensor<
- f32[2][8]
- [
- [-0.6438822746276855, -2.9047577381134033, 0.9005677103996277, 1.593727946281433, 1.4294962882995605, 2.7334585189819336, -0.7356647253036499, 1.7708399295806885],
- [0.12331989407539368, -8.465315818786621, 2.132427453994751, 2.2526159286499023, 3.0098886489868164, 10.633148193359375, -2.20133376121521, 2.5171523094177246]
- ]
->
#Nx.Tensor<
- f32[2][1]
- [
- [1.100995421409607],
- [2.2032604217529297]
- ]
->
And on backwards:
Nx.Defn.grad(fn params -> predict_fn.(params, input) end).(params)
#Nx.Tensor<
- f32[2][4]
- [
- [0.0, 1.0, 2.0, 3.0],
- [4.0, 5.0, 6.0, 7.0]
- ]
->
-#Nx.Tensor<
- f32[2][8]
- [
- [-0.6438822746276855, -2.9047577381134033, 0.9005677103996277, 1.593727946281433, 1.4294962882995605, 2.7334585189819336, -0.7356647253036499, 1.7708399295806885],
- [0.12331989407539368, -8.465315818786621, 2.132427453994751, 2.2526159286499023, 3.0098886489868164, 10.633148193359375, -2.20133376121521, 2.5171523094177246]
- ]
->
-#Nx.Tensor<
- f32[2][8]
- [
- [-0.6438822746276855, -2.9047577381134033, 0.9005677103996277, 1.593727946281433, 1.4294962882995605, 2.7334585189819336, -0.7356647253036499, 1.7708399295806885],
- [0.12331989407539368, -8.465315818786621, 2.132427453994751, 2.2526159286499023, 3.0098886489868164, 10.633148193359375, -2.20133376121521, 2.5171523094177246]
- ]
->
%{
- "dense_0" => %{
- "bias" => #Nx.Tensor<
- f32[8]
- [-0.7136709690093994, 0.0, 0.1671910583972931, 0.15292571485042572, 1.4267574548721313, -0.01234421506524086, 0.0, -0.11186791956424713]
- >,
- "kernel" => #Nx.Tensor<
- f32[4][8]
- [
- [-2.8546838760375977, 0.0, 0.3343821167945862, 0.30585142970085144, 2.8535149097442627, -0.02468843013048172, 0.0, -0.22373583912849426],
- [-3.568354845046997, 0.0, 0.5015732049942017, 0.45877712965011597, 4.280272483825684, -0.03703264519572258, 0.0, -0.3356037735939026],
- [-4.2820258140563965, 0.0, 0.6687642335891724, 0.6117028594017029, 5.707029819488525, -0.04937686026096344, 0.0, -0.4474716782569885],
- [-4.995697021484375, 0.0, 0.8359552621841431, 0.7646285891532898, 7.133787155151367, -0.0617210753262043, 0.0, -0.5593395829200745]
- ]
- >
- },
- "dense_1" => %{
- "bias" => #Nx.Tensor<
- f32[1]
- [2.0]
- >,
- "kernel" => #Nx.Tensor<
- f32[8][1]
- [
- [0.12331989407539368],
- [0.0],
- [3.0329952239990234],
- [3.846343994140625],
- [4.439384937286377],
- [13.366606712341309],
- [0.0],
- [4.287992477416992]
- ]
- >
- }
-}
Finally, you can specify hooks to only run when the model is built in a certain mode such as training and inference mode. You can read more about training and inference mode in Training and inference mode:
model =
- Axon.input("data")
- |> Axon.dense(8)
- |> Axon.attach_hook(&IO.inspect/1, on: :forward, mode: :train)
- |> Axon.relu()
-
-{init_fn, predict_fn} = Axon.build(model, mode: :train)
-params = init_fn.(input, %{})
%{
- "dense_0" => %{
- "bias" => #Nx.Tensor<
- f32[8]
- [0.0, 0.0, 0.0, 0.0, 0.0, 0.0, 0.0, 0.0]
- >,
- "kernel" => #Nx.Tensor<
- f32[4][8]
- [
- [0.13930729031562805, 0.6213980913162231, 0.5555388331413269, -0.18602639436721802, 0.37516212463378906, 0.025288991630077362, 0.5311357378959656, 0.2825106978416443],
- [-0.14007511734962463, -0.1472432166337967, -0.011716545559465885, 0.06804006546735764, 0.4615606963634491, -0.024897094815969467, -0.2336975485086441, 0.10019711405038834],
- [-0.29539188742637634, -0.5487134456634521, 0.41018739342689514, -0.49597275257110596, 0.2970600426197052, 0.4304136335849762, 0.13961079716682434, -0.4316418170928955],
- [0.5435506105422974, -0.056049738079309464, 0.5059406161308289, 0.29488587379455566, 0.5656863451004028, 0.43807661533355713, -0.5058187246322632, -0.6963644623756409]
- ]
- >
- }
-}
The model was built in training mode so the hook will run:
predict_fn.(params, input)
#Nx.Tensor<
- f32[2][8]
- [
- [0.8997929096221924, -1.412819266319275, 2.3264801502227783, -0.039247818291187286, 2.752739906311035, 2.150160074234009, -1.4719321727752686, -2.852180004119873],
- [1.8893564939498901, -1.9352525472640991, 8.166281700134277, -1.3155406713485718, 9.550616264343262, 5.625688552856445, -1.7470110654830933, -5.833373546600342]
- ]
->
%{
- prediction: #Nx.Tensor<
- f32[2][8]
- [
- [0.8997929096221924, 0.0, 2.3264801502227783, 0.0, 2.752739906311035, 2.150160074234009, 0.0, 0.0],
- [1.8893564939498901, 0.0, 8.166281700134277, 0.0, 9.550616264343262, 5.625688552856445, 0.0, 0.0]
- ]
- >,
- state: %{}
-}
{init_fn, predict_fn} = Axon.build(model, mode: :inference)
-params = init_fn.(input, %{})
%{
- "dense_0" => %{
- "bias" => #Nx.Tensor<
- f32[8]
- [0.0, 0.0, 0.0, 0.0, 0.0, 0.0, 0.0, 0.0]
- >,
- "kernel" => #Nx.Tensor<
- f32[4][8]
- [
- [0.4261569678783417, -0.6842133402824402, -0.13853907585144043, 0.6665098667144775, 0.6171062588691711, 0.25513389706611633, -0.4866299033164978, -0.5819953680038452],
- [-0.36037471890449524, -0.21852241456508636, -0.6355746388435364, -0.5705516934394836, -0.35449153184890747, -0.1527744084596634, -0.5036700367927551, -0.4164859354496002],
- [0.6485253572463989, 0.30033791065216064, 0.35249730944633484, -0.31768497824668884, 0.020564774051308632, 0.147691547870636, 0.6939279437065125, 0.6060985922813416],
- [0.006978582590818405, 0.5333927869796753, 0.30155065655708313, -0.09574121236801147, 0.3447912037372589, -0.11081335693597794, 0.5808792114257812, 0.04360806941986084]
- ]
- >
- }
-}
The model was built in inference mode so the hook will not run:
predict_fn.(params, input)
#Nx.Tensor<
- f32[2][8]
- [
- [0.9576117396354675, 1.9823317527770996, 0.9740719795227051, 0.0, 0.7210116386413574, 0.0, 2.6268234252929688, 0.9265354871749878],
- [3.842756509780884, 1.706311583518982, 0.49380895495414734, 0.0, 3.2328944206237793, 0.36711934208869934, 3.764852285385132, 0.0]
- ]
->
+ Axon.input("data")
+ |> Axon.dense(8)
+ |> Axon.attach_hook(fn val -> IO.inspect(val, label: :dense_forward) end, on: :forward)
+ |> Axon.attach_hook(fn val -> IO.inspect(val, label: :dense_init) end, on: :initialize)
+ |> Axon.relu()
+ |> Axon.attach_hook(fn val -> IO.inspect(val, label: :relu) end, on: :forward)
+
+{init_fn, predict_fn} = Axon.build(model)
+
+input = Nx.iota({2, 4}, type: :f32)
+params = init_fn.(input, %{})dense_init: %{
+ "bias" => #Nx.Tensor<
+ f32[8]
+ [0.0, 0.0, 0.0, 0.0, 0.0, 0.0, 0.0, 0.0]
+ >,
+ "kernel" => #Nx.Tensor<
+ f32[4][8]
+ [
+ [-0.40611347556114197, -0.1551232784986496, 0.08485020697116852, -0.6748610734939575, 0.04797258973121643, -0.059523195028305054, 0.4092640280723572, 0.1300794780254364],
+ [-0.3551754057407379, 0.3159058094024658, 0.25394684076309204, 0.22510826587677002, 0.2613920271396637, -0.15213526785373688, -0.15744848549365997, -0.46065202355384827],
+ [-0.5224899649620056, 0.3639957010746002, -0.19676287472248077, 0.5423932075500488, -0.4722306430339813, 0.26447463035583496, 0.18534891307353973, -0.6442952752113342],
+ [-0.5629043579101562, 0.6370815634727478, -0.43325361609458923, 0.5084872245788574, -0.1424017995595932, 0.4865548312664032, -0.5839526057243347, 0.09811079502105713]
+ ]
+ >
+}
%{
+ "dense_0" => %{
+ "bias" => #Nx.Tensor<
+ f32[8]
+ [0.0, 0.0, 0.0, 0.0, 0.0, 0.0, 0.0, 0.0]
+ >,
+ "kernel" => #Nx.Tensor<
+ f32[4][8]
+ [
+ [-0.40611347556114197, -0.1551232784986496, 0.08485020697116852, -0.6748610734939575, 0.04797258973121643, -0.059523195028305054, 0.4092640280723572, 0.1300794780254364],
+ [-0.3551754057407379, 0.3159058094024658, 0.25394684076309204, 0.22510826587677002, 0.2613920271396637, -0.15213526785373688, -0.15744848549365997, -0.46065202355384827],
+ [-0.5224899649620056, 0.3639957010746002, -0.19676287472248077, 0.5423932075500488, -0.4722306430339813, 0.26447463035583496, 0.18534891307353973, -0.6442952752113342],
+ [-0.5629043579101562, 0.6370815634727478, -0.43325361609458923, 0.5084872245788574, -0.1424017995595932, 0.4865548312664032, -0.5839526057243347, 0.09811079502105713]
+ ]
+ >
+ }
+}
Notice how during initialization the :dense_init
hook fired and inspected the layer's parameters. Now when executing, you'll see outputs for :dense
and :relu
:
predict_fn.(params, input)
dense_forward: #Nx.Tensor<
+ f32[2][8]
+ [
+ [-3.0888683795928955, 2.955142021179199, -1.4393397569656372, 2.8353562355041504, -1.1102746725082397, 1.8364784717559814, -1.538608431816101, -1.454910159111023],
+ [-10.475601196289062, 7.602581024169922, -2.604217529296875, 5.239866733551025, -2.331346035003662, 3.993962526321411, -2.125761032104492, -4.961938381195068]
+ ]
+>
+relu: #Nx.Tensor<
+ f32[2][8]
+ [
+ [0.0, 2.955142021179199, 0.0, 2.8353562355041504, 0.0, 1.8364784717559814, 0.0, 0.0],
+ [0.0, 7.602581024169922, 0.0, 5.239866733551025, 0.0, 3.993962526321411, 0.0, 0.0]
+ ]
+>
#Nx.Tensor<
+ f32[2][8]
+ [
+ [0.0, 2.955142021179199, 0.0, 2.8353562355041504, 0.0, 1.8364784717559814, 0.0, 0.0],
+ [0.0, 7.602581024169922, 0.0, 5.239866733551025, 0.0, 3.993962526321411, 0.0, 0.0]
+ ]
+>
It's important to note that hooks execute in the order they were attached to a layer. If you attach 2 hooks to the same layer which execute different functions on the same event, they will run in order:
model =
+ Axon.input("data")
+ |> Axon.dense(8)
+ |> Axon.attach_hook(fn val -> IO.inspect(val, label: :hook1) end, on: :forward)
+ |> Axon.attach_hook(fn val -> IO.inspect(val, label: :hook2) end, on: :forward)
+ |> Axon.relu()
+
+{init_fn, predict_fn} = Axon.build(model)
+params = init_fn.(input, %{})
+
+predict_fn.(params, input)
hook1: #Nx.Tensor<
+ f32[2][8]
+ [
+ [1.3320910930633545, 1.712153673171997, -2.0420351028442383, 2.2541849613189697, -3.1382551193237305, -1.2241677045822144, -1.5477651357650757, -0.2126261293888092],
+ [2.1975531578063965, 3.722827911376953, -1.6301460266113281, 5.891226768493652, -10.79372787475586, -2.9982359409332275, -6.589874267578125, 1.5387766361236572]
+ ]
+>
+hook2: #Nx.Tensor<
+ f32[2][8]
+ [
+ [1.3320910930633545, 1.712153673171997, -2.0420351028442383, 2.2541849613189697, -3.1382551193237305, -1.2241677045822144, -1.5477651357650757, -0.2126261293888092],
+ [2.1975531578063965, 3.722827911376953, -1.6301460266113281, 5.891226768493652, -10.79372787475586, -2.9982359409332275, -6.589874267578125, 1.5387766361236572]
+ ]
+>
#Nx.Tensor<
+ f32[2][8]
+ [
+ [1.3320910930633545, 1.712153673171997, 0.0, 2.2541849613189697, 0.0, 0.0, 0.0, 0.0],
+ [2.1975531578063965, 3.722827911376953, 0.0, 5.891226768493652, 0.0, 0.0, 0.0, 1.5387766361236572]
+ ]
+>
Notice that :hook1
fires before :hook2
.
You can also specify a hook to fire on all events:
model =
+ Axon.input("data")
+ |> Axon.dense(8)
+ |> Axon.attach_hook(&IO.inspect/1, on: :all)
+ |> Axon.relu()
+ |> Axon.dense(1)
+
+{init_fn, predict_fn} = Axon.build(model)
{#Function<136.40088443/2 in Nx.Defn.wrap_arity/2>,
+ #Function<136.40088443/2 in Nx.Defn.wrap_arity/2>}
On initialization:
params = init_fn.(input, %{})
%{
+ "bias" => #Nx.Tensor<
+ f32[8]
+ [0.0, 0.0, 0.0, 0.0, 0.0, 0.0, 0.0, 0.0]
+ >,
+ "kernel" => #Nx.Tensor<
+ f32[4][8]
+ [
+ [0.6784419417381287, 0.175045907497406, 0.010701040737330914, -0.5537784695625305, -0.010694148950278759, 0.7021086812019348, -0.3290281891822815, -0.6818609237670898],
+ [-0.6378231644630432, -0.5675055384635925, 0.031453751027584076, 0.4705190360546112, -0.002226108219474554, 0.48611924052238464, 0.5700677037239075, 0.6729928851127625],
+ [0.4596043527126312, -0.6557875871658325, -0.07168347388505936, -0.37926459312438965, -0.20766735076904297, 0.11274437606334686, -0.5166378617286682, -0.5115087032318115],
+ [-0.30842259526252747, -0.3418923616409302, 0.3374936282634735, 0.6272460222244263, 0.6156857013702393, 0.6739501357078552, -0.09081890434026718, 0.706954836845398]
+ ]
+ >
+}
%{
+ "dense_0" => %{
+ "bias" => #Nx.Tensor<
+ f32[8]
+ [0.0, 0.0, 0.0, 0.0, 0.0, 0.0, 0.0, 0.0]
+ >,
+ "kernel" => #Nx.Tensor<
+ f32[4][8]
+ [
+ [0.6784419417381287, 0.175045907497406, 0.010701040737330914, -0.5537784695625305, -0.010694148950278759, 0.7021086812019348, -0.3290281891822815, -0.6818609237670898],
+ [-0.6378231644630432, -0.5675055384635925, 0.031453751027584076, 0.4705190360546112, -0.002226108219474554, 0.48611924052238464, 0.5700677037239075, 0.6729928851127625],
+ [0.4596043527126312, -0.6557875871658325, -0.07168347388505936, -0.37926459312438965, -0.20766735076904297, 0.11274437606334686, -0.5166378617286682, -0.5115087032318115],
+ [-0.30842259526252747, -0.3418923616409302, 0.3374936282634735, 0.6272460222244263, 0.6156857013702393, 0.6739501357078552, -0.09081890434026718, 0.706954836845398]
+ ]
+ >
+ },
+ "dense_1" => %{
+ "bias" => #Nx.Tensor<
+ f32[1]
+ [0.0]
+ >,
+ "kernel" => #Nx.Tensor<
+ f32[8][1]
+ [
+ [-0.7136709690093994],
+ [-0.16328231990337372],
+ [0.08359552919864655],
+ [0.07646285742521286],
+ [0.7133787274360657],
+ [-0.00617210753262043],
+ [0.2241944670677185],
+ [-0.055933959782123566]
+ ]
+ >
+ }
+}
On pre-forward and forward:
predict_fn.(params, input)
#Nx.Tensor<
+ f32[2][4]
+ [
+ [0.0, 1.0, 2.0, 3.0],
+ [4.0, 5.0, 6.0, 7.0]
+ ]
+>
+#Nx.Tensor<
+ f32[2][8]
+ [
+ [-0.6438822746276855, -2.9047577381134033, 0.9005677103996277, 1.593727946281433, 1.4294962882995605, 2.7334585189819336, -0.7356647253036499, 1.7708399295806885],
+ [0.12331989407539368, -8.465315818786621, 2.132427453994751, 2.2526159286499023, 3.0098886489868164, 10.633148193359375, -2.20133376121521, 2.5171523094177246]
+ ]
+>
+#Nx.Tensor<
+ f32[2][8]
+ [
+ [-0.6438822746276855, -2.9047577381134033, 0.9005677103996277, 1.593727946281433, 1.4294962882995605, 2.7334585189819336, -0.7356647253036499, 1.7708399295806885],
+ [0.12331989407539368, -8.465315818786621, 2.132427453994751, 2.2526159286499023, 3.0098886489868164, 10.633148193359375, -2.20133376121521, 2.5171523094177246]
+ ]
+>
#Nx.Tensor<
+ f32[2][1]
+ [
+ [1.100995421409607],
+ [2.2032604217529297]
+ ]
+>
And on backwards:
Nx.Defn.grad(fn params -> predict_fn.(params, input) end).(params)
#Nx.Tensor<
+ f32[2][4]
+ [
+ [0.0, 1.0, 2.0, 3.0],
+ [4.0, 5.0, 6.0, 7.0]
+ ]
+>
+#Nx.Tensor<
+ f32[2][8]
+ [
+ [-0.6438822746276855, -2.9047577381134033, 0.9005677103996277, 1.593727946281433, 1.4294962882995605, 2.7334585189819336, -0.7356647253036499, 1.7708399295806885],
+ [0.12331989407539368, -8.465315818786621, 2.132427453994751, 2.2526159286499023, 3.0098886489868164, 10.633148193359375, -2.20133376121521, 2.5171523094177246]
+ ]
+>
+#Nx.Tensor<
+ f32[2][8]
+ [
+ [-0.6438822746276855, -2.9047577381134033, 0.9005677103996277, 1.593727946281433, 1.4294962882995605, 2.7334585189819336, -0.7356647253036499, 1.7708399295806885],
+ [0.12331989407539368, -8.465315818786621, 2.132427453994751, 2.2526159286499023, 3.0098886489868164, 10.633148193359375, -2.20133376121521, 2.5171523094177246]
+ ]
+>
%{
+ "dense_0" => %{
+ "bias" => #Nx.Tensor<
+ f32[8]
+ [-0.7136709690093994, 0.0, 0.1671910583972931, 0.15292571485042572, 1.4267574548721313, -0.01234421506524086, 0.0, -0.11186791956424713]
+ >,
+ "kernel" => #Nx.Tensor<
+ f32[4][8]
+ [
+ [-2.8546838760375977, 0.0, 0.3343821167945862, 0.30585142970085144, 2.8535149097442627, -0.02468843013048172, 0.0, -0.22373583912849426],
+ [-3.568354845046997, 0.0, 0.5015732049942017, 0.45877712965011597, 4.280272483825684, -0.03703264519572258, 0.0, -0.3356037735939026],
+ [-4.2820258140563965, 0.0, 0.6687642335891724, 0.6117028594017029, 5.707029819488525, -0.04937686026096344, 0.0, -0.4474716782569885],
+ [-4.995697021484375, 0.0, 0.8359552621841431, 0.7646285891532898, 7.133787155151367, -0.0617210753262043, 0.0, -0.5593395829200745]
+ ]
+ >
+ },
+ "dense_1" => %{
+ "bias" => #Nx.Tensor<
+ f32[1]
+ [2.0]
+ >,
+ "kernel" => #Nx.Tensor<
+ f32[8][1]
+ [
+ [0.12331989407539368],
+ [0.0],
+ [3.0329952239990234],
+ [3.846343994140625],
+ [4.439384937286377],
+ [13.366606712341309],
+ [0.0],
+ [4.287992477416992]
+ ]
+ >
+ }
+}
Finally, you can specify hooks to only run when the model is built in a certain mode such as training and inference mode. You can read more about training and inference mode in Training and inference mode:
model =
+ Axon.input("data")
+ |> Axon.dense(8)
+ |> Axon.attach_hook(&IO.inspect/1, on: :forward, mode: :train)
+ |> Axon.relu()
+
+{init_fn, predict_fn} = Axon.build(model, mode: :train)
+params = init_fn.(input, %{})
%{
+ "dense_0" => %{
+ "bias" => #Nx.Tensor<
+ f32[8]
+ [0.0, 0.0, 0.0, 0.0, 0.0, 0.0, 0.0, 0.0]
+ >,
+ "kernel" => #Nx.Tensor<
+ f32[4][8]
+ [
+ [0.13930729031562805, 0.6213980913162231, 0.5555388331413269, -0.18602639436721802, 0.37516212463378906, 0.025288991630077362, 0.5311357378959656, 0.2825106978416443],
+ [-0.14007511734962463, -0.1472432166337967, -0.011716545559465885, 0.06804006546735764, 0.4615606963634491, -0.024897094815969467, -0.2336975485086441, 0.10019711405038834],
+ [-0.29539188742637634, -0.5487134456634521, 0.41018739342689514, -0.49597275257110596, 0.2970600426197052, 0.4304136335849762, 0.13961079716682434, -0.4316418170928955],
+ [0.5435506105422974, -0.056049738079309464, 0.5059406161308289, 0.29488587379455566, 0.5656863451004028, 0.43807661533355713, -0.5058187246322632, -0.6963644623756409]
+ ]
+ >
+ }
+}
The model was built in training mode so the hook will run:
predict_fn.(params, input)
#Nx.Tensor<
+ f32[2][8]
+ [
+ [0.8997929096221924, -1.412819266319275, 2.3264801502227783, -0.039247818291187286, 2.752739906311035, 2.150160074234009, -1.4719321727752686, -2.852180004119873],
+ [1.8893564939498901, -1.9352525472640991, 8.166281700134277, -1.3155406713485718, 9.550616264343262, 5.625688552856445, -1.7470110654830933, -5.833373546600342]
+ ]
+>
%{
+ prediction: #Nx.Tensor<
+ f32[2][8]
+ [
+ [0.8997929096221924, 0.0, 2.3264801502227783, 0.0, 2.752739906311035, 2.150160074234009, 0.0, 0.0],
+ [1.8893564939498901, 0.0, 8.166281700134277, 0.0, 9.550616264343262, 5.625688552856445, 0.0, 0.0]
+ ]
+ >,
+ state: %{}
+}
{init_fn, predict_fn} = Axon.build(model, mode: :inference)
+params = init_fn.(input, %{})
%{
+ "dense_0" => %{
+ "bias" => #Nx.Tensor<
+ f32[8]
+ [0.0, 0.0, 0.0, 0.0, 0.0, 0.0, 0.0, 0.0]
+ >,
+ "kernel" => #Nx.Tensor<
+ f32[4][8]
+ [
+ [0.4261569678783417, -0.6842133402824402, -0.13853907585144043, 0.6665098667144775, 0.6171062588691711, 0.25513389706611633, -0.4866299033164978, -0.5819953680038452],
+ [-0.36037471890449524, -0.21852241456508636, -0.6355746388435364, -0.5705516934394836, -0.35449153184890747, -0.1527744084596634, -0.5036700367927551, -0.4164859354496002],
+ [0.6485253572463989, 0.30033791065216064, 0.35249730944633484, -0.31768497824668884, 0.020564774051308632, 0.147691547870636, 0.6939279437065125, 0.6060985922813416],
+ [0.006978582590818405, 0.5333927869796753, 0.30155065655708313, -0.09574121236801147, 0.3447912037372589, -0.11081335693597794, 0.5808792114257812, 0.04360806941986084]
+ ]
+ >
+ }
+}
The model was built in inference mode so the hook will not run:
predict_fn.(params, input)
#Nx.Tensor<
+ f32[2][8]
+ [
+ [0.9576117396354675, 1.9823317527770996, 0.9740719795227051, 0.0, 0.7210116386413574, 0.0, 2.6268234252929688, 0.9265354871749878],
+ [3.842756509780884, 1.706311583518982, 0.49380895495414734, 0.0, 3.2328944206237793, 0.36711934208869934, 3.764852285385132, 0.0]
+ ]
+>
diff --git a/multi_input_multi_output_models.html b/multi_input_multi_output_models.html
index d2f50a5a..e7301d3a 100644
--- a/multi_input_multi_output_models.html
+++ b/multi_input_multi_output_models.html
@@ -115,64 +115,64 @@
-Mix.install([
- {:axon, github: "elixir-nx/axon"},
- {:nx, "~> 0.3.0", github: "elixir-nx/nx", sparse: "nx", override: true},
- {:kino, "~> 0.7.0"}
-])
:ok
+Mix.install([
+ {:axon, github: "elixir-nx/axon"},
+ {:nx, "~> 0.3.0", github: "elixir-nx/nx", sparse: "nx", override: true},
+ {:kino, "~> 0.7.0"}
+])
:ok
creating-multi-input-models
Creating multi-input models
-
Sometimes your application necessitates the use of multiple inputs. To use multiple inputs in an Axon model, you just need to declare multiple inputs in your graph:
input_1 = Axon.input("input_1")
-input_2 = Axon.input("input_2")
+Sometimes your application necessitates the use of multiple inputs. To use multiple inputs in an Axon model, you just need to declare multiple inputs in your graph:
input_1 = Axon.input("input_1")
+input_2 = Axon.input("input_2")
-out = Axon.add(input_1, input_2)
#Axon<
- inputs: %{"input_1" => nil, "input_2" => nil}
+out = Axon.add(input_1, input_2)
#Axon<
+ inputs: %{"input_1" => nil, "input_2" => nil}
outputs: "add_0"
nodes: 4
->
Notice when you inspect the model, it tells you what your models inputs are up front. You can also get metadata about your model inputs programmatically with Axon.get_inputs/1
:
Axon.get_inputs(out)
%{"input_1" => nil, "input_2" => nil}
Each input is uniquely named, so you can pass inputs by-name into inspection and execution functions with a map:
inputs = %{
- "input_1" => Nx.template({2, 8}, :f32),
- "input_2" => Nx.template({2, 8}, :f32)
-}
+>
Notice when you inspect the model, it tells you what your models inputs are up front. You can also get metadata about your model inputs programmatically with Axon.get_inputs/1
:
Axon.get_inputs(out)
%{"input_1" => nil, "input_2" => nil}
Each input is uniquely named, so you can pass inputs by-name into inspection and execution functions with a map:
inputs = %{
+ "input_1" => Nx.template({2, 8}, :f32),
+ "input_2" => Nx.template({2, 8}, :f32)
+}
-Axon.Display.as_graph(out, inputs)
graph TD;
+
Axon.Display.as_graph(out, inputs)
graph TD;
3[/"input_1 (:input) {2, 8}"/];
4[/"input_2 (:input) {2, 8}"/];
5["container_0 (:container) {{2, 8}, {2, 8}}"];
6["add_0 (:add) {2, 8}"];
5 --> 6;
4 --> 5;
-3 --> 5;
{init_fn, predict_fn} = Axon.build(out)
-params = init_fn.(inputs, %{})
%{}
inputs = %{
- "input_1" => Nx.iota({2, 8}, type: :f32),
- "input_2" => Nx.iota({2, 8}, type: :f32)
-}
-
-predict_fn.(params, inputs)
#Nx.Tensor<
- f32[2][8]
- [
- [0.0, 2.0, 4.0, 6.0, 8.0, 10.0, 12.0, 14.0],
- [16.0, 18.0, 20.0, 22.0, 24.0, 26.0, 28.0, 30.0]
- ]
->
If you forget a required input, Axon will raise:
predict_fn.(params, %{"input_1" => Nx.iota({2, 8}, type: :f32)})
+3 --> 5;{init_fn, predict_fn} = Axon.build(out)
+params = init_fn.(inputs, %{})
%{}
inputs = %{
+ "input_1" => Nx.iota({2, 8}, type: :f32),
+ "input_2" => Nx.iota({2, 8}, type: :f32)
+}
+
+predict_fn.(params, inputs)
#Nx.Tensor<
+ f32[2][8]
+ [
+ [0.0, 2.0, 4.0, 6.0, 8.0, 10.0, 12.0, 14.0],
+ [16.0, 18.0, 20.0, 22.0, 24.0, 26.0, 28.0, 30.0]
+ ]
+>
If you forget a required input, Axon will raise:
predict_fn.(params, %{"input_1" => Nx.iota({2, 8}, type: :f32)})
creating-multi-output-models
Creating multi-output models
-Depending on your application, you might also want your model to have multiple outputs. You can achieve this by using Axon.container/2
to wrap multiple nodes into any supported Nx container:
inp = Axon.input("data")
+Depending on your application, you might also want your model to have multiple outputs. You can achieve this by using Axon.container/2
to wrap multiple nodes into any supported Nx container:
inp = Axon.input("data")
-x1 = inp |> Axon.dense(32) |> Axon.relu()
-x2 = inp |> Axon.dense(64) |> Axon.relu()
+x1 = inp |> Axon.dense(32) |> Axon.relu()
+x2 = inp |> Axon.dense(64) |> Axon.relu()
-out = Axon.container({x1, x2})
#Axon<
- inputs: %{"data" => nil}
+out = Axon.container({x1, x2})
#Axon<
+ inputs: %{"data" => nil}
outputs: "container_0"
nodes: 6
->
template = Nx.template({2, 8}, :f32)
-Axon.Display.as_graph(out, template)
graph TD;
+
>
template = Nx.template({2, 8}, :f32)
+Axon.Display.as_graph(out, template)
graph TD;
7[/"data (:input) {2, 8}"/];
10["dense_0 (:dense) {2, 32}"];
11["relu_0 (:relu) {2, 32}"];
@@ -184,80 +184,80 @@
14 --> 15;
7 --> 14;
10 --> 11;
-7 --> 10;
When executed, containers will return a data structure which matches their input structure:
{init_fn, predict_fn} = Axon.build(out)
-params = init_fn.(template, %{})
-predict_fn.(params, Nx.iota({2, 8}, type: :f32))
{#Nx.Tensor<
- f32[2][32]
- [
- [0.0, 0.0, 3.111135482788086, 0.48920655250549316, 0.0, 0.5125713348388672, 0.0, 0.0, 1.482532262802124, 0.0, 0.0, 0.0, 0.0, 3.103637933731079, 0.46897295117378235, 2.6465413570404053, 2.837477445602417, 0.6159781217575073, 1.3220927715301514, 0.0, 0.24302834272384644, 3.4662821292877197, 0.40560781955718994, 0.0, 0.0, 0.2682836055755615, 3.5352964401245117, 0.0, 0.6591103672981262, 2.5643503665924072, 0.0, 0.0],
- [0.0, 0.0, 4.642599105834961, 0.0, 0.0, 1.8978865146636963, 2.2522430419921875, 0.0, 1.2110804319381714, 2.5524141788482666, 0.0, 0.742849588394165, 0.0, 8.30776596069336, 5.09386682510376, 4.69991397857666, 5.195588111877441, ...]
- ]
- >,
- #Nx.Tensor<
- f32[2][64]
- [
- [0.0, 0.0, 0.7948622107505798, 0.0, 0.0, 0.0, 0.0, 0.0, 2.3980231285095215, 5.2512712478637695, 1.5820361375808716, 0.0, 2.6624603271484375, 0.0, 0.0, 0.0, 1.6954007148742676, 0.017102837562561035, 0.7754535675048828, 0.0, 1.891753911972046, 0.0, 2.7824556827545166, 0.0, 0.5906356573104858, 0.0, 0.0, 1.288651466369629, 0.6939071416854858, 0.8427785038948059, 1.5664646625518799, 0.38097164034843445, 0.0, 0.0, 0.0, 0.0, 0.0, 0.0, 0.3193289637565613, 0.0, 0.0, 0.35316526889801025, 0.0, 1.2567038536071777, 0.7732977867126465, 0.16440902650356293, 0.0, 1.9872947931289673, ...],
+7 --> 10;
When executed, containers will return a data structure which matches their input structure:
{init_fn, predict_fn} = Axon.build(out)
+params = init_fn.(template, %{})
+predict_fn.(params, Nx.iota({2, 8}, type: :f32))
{#Nx.Tensor<
+ f32[2][32]
+ [
+ [0.0, 0.0, 3.111135482788086, 0.48920655250549316, 0.0, 0.5125713348388672, 0.0, 0.0, 1.482532262802124, 0.0, 0.0, 0.0, 0.0, 3.103637933731079, 0.46897295117378235, 2.6465413570404053, 2.837477445602417, 0.6159781217575073, 1.3220927715301514, 0.0, 0.24302834272384644, 3.4662821292877197, 0.40560781955718994, 0.0, 0.0, 0.2682836055755615, 3.5352964401245117, 0.0, 0.6591103672981262, 2.5643503665924072, 0.0, 0.0],
+ [0.0, 0.0, 4.642599105834961, 0.0, 0.0, 1.8978865146636963, 2.2522430419921875, 0.0, 1.2110804319381714, 2.5524141788482666, 0.0, 0.742849588394165, 0.0, 8.30776596069336, 5.09386682510376, 4.69991397857666, 5.195588111877441, ...]
+ ]
+ >,
+ #Nx.Tensor<
+ f32[2][64]
+ [
+ [0.0, 0.0, 0.7948622107505798, 0.0, 0.0, 0.0, 0.0, 0.0, 2.3980231285095215, 5.2512712478637695, 1.5820361375808716, 0.0, 2.6624603271484375, 0.0, 0.0, 0.0, 1.6954007148742676, 0.017102837562561035, 0.7754535675048828, 0.0, 1.891753911972046, 0.0, 2.7824556827545166, 0.0, 0.5906356573104858, 0.0, 0.0, 1.288651466369629, 0.6939071416854858, 0.8427785038948059, 1.5664646625518799, 0.38097164034843445, 0.0, 0.0, 0.0, 0.0, 0.0, 0.0, 0.3193289637565613, 0.0, 0.0, 0.35316526889801025, 0.0, 1.2567038536071777, 0.7732977867126465, 0.16440902650356293, 0.0, 1.9872947931289673, ...],
...
- ]
- >}
You can output maps as well:
out = Axon.container(%{x1: x1, x2: x2})
#Axon<
- inputs: %{"data" => nil}
+ ]
+ >}
You can output maps as well:
out = Axon.container(%{x1: x1, x2: x2})
#Axon<
+ inputs: %{"data" => nil}
outputs: "container_0"
nodes: 6
->
{init_fn, predict_fn} = Axon.build(out)
-params = init_fn.(template, %{})
-predict_fn.(params, Nx.iota({2, 8}, type: :f32))
%{
- x1: #Nx.Tensor<
- f32[2][32]
- [
- [0.0, 0.0, 0.0, 0.0, 0.0, 0.0, 1.8718442916870117, 0.0, 1.813383936882019, 0.0, 0.0, 0.0, 0.0, 3.0636630058288574, 0.0, 1.1350113153457642, 1.7888737916946411, 0.0658932775259018, 0.0, 0.4498137831687927, 1.1311852931976318, 3.2784717082977295, 0.0, 2.4505443572998047, 3.346879005432129, 0.0, 0.0, 2.614570140838623, 0.0, 0.0, 0.8967163562774658, 0.0],
- [0.0, 0.0, 0.0, 1.9045438766479492, 0.0, 0.0, 7.110898971557617, 0.09859625995159149, 8.149545669555664, 0.0, 0.0, 0.0, 0.0, 4.178244113922119, 0.0, 3.8360297679901123, 6.177351474761963, ...]
- ]
- >,
- x2: #Nx.Tensor<
- f32[2][64]
- [
- [0.41670602560043335, 0.0, 0.0, 0.0, 1.338260531425476, 0.0, 0.5181264877319336, 1.1024510860443115, 0.0, 0.0, 1.485485553741455, 0.0, 0.0, 1.9365136623382568, 0.0, 0.0, 0.0, 0.0, 2.6925604343414307, 0.6202171444892883, 0.0, 0.08886899054050446, 0.0, 1.3045244216918945, 0.0, 0.0545249879360199, 0.0, 1.2294358015060425, 0.0, 0.0, 0.670710563659668, 0.0, 4.161868572235107, 1.880513072013855, 2.6189277172088623, 0.5702207684516907, 0.0, 1.953904151916504, 0.0, 0.0, 1.370330572128296, 0.17245425283908844, 1.9922431707382202, 2.6845364570617676, 0.3711611032485962, 0.7940037250518799, 0.0, 2.12975811958313, ...],
+>
{init_fn, predict_fn} = Axon.build(out)
+params = init_fn.(template, %{})
+predict_fn.(params, Nx.iota({2, 8}, type: :f32))
%{
+ x1: #Nx.Tensor<
+ f32[2][32]
+ [
+ [0.0, 0.0, 0.0, 0.0, 0.0, 0.0, 1.8718442916870117, 0.0, 1.813383936882019, 0.0, 0.0, 0.0, 0.0, 3.0636630058288574, 0.0, 1.1350113153457642, 1.7888737916946411, 0.0658932775259018, 0.0, 0.4498137831687927, 1.1311852931976318, 3.2784717082977295, 0.0, 2.4505443572998047, 3.346879005432129, 0.0, 0.0, 2.614570140838623, 0.0, 0.0, 0.8967163562774658, 0.0],
+ [0.0, 0.0, 0.0, 1.9045438766479492, 0.0, 0.0, 7.110898971557617, 0.09859625995159149, 8.149545669555664, 0.0, 0.0, 0.0, 0.0, 4.178244113922119, 0.0, 3.8360297679901123, 6.177351474761963, ...]
+ ]
+ >,
+ x2: #Nx.Tensor<
+ f32[2][64]
+ [
+ [0.41670602560043335, 0.0, 0.0, 0.0, 1.338260531425476, 0.0, 0.5181264877319336, 1.1024510860443115, 0.0, 0.0, 1.485485553741455, 0.0, 0.0, 1.9365136623382568, 0.0, 0.0, 0.0, 0.0, 2.6925604343414307, 0.6202171444892883, 0.0, 0.08886899054050446, 0.0, 1.3045244216918945, 0.0, 0.0545249879360199, 0.0, 1.2294358015060425, 0.0, 0.0, 0.670710563659668, 0.0, 4.161868572235107, 1.880513072013855, 2.6189277172088623, 0.5702207684516907, 0.0, 1.953904151916504, 0.0, 0.0, 1.370330572128296, 0.17245425283908844, 1.9922431707382202, 2.6845364570617676, 0.3711611032485962, 0.7940037250518799, 0.0, 2.12975811958313, ...],
...
- ]
- >
-}
Containers even support arbitrary nesting:
out = Axon.container({%{x1: {x1, x2}, x2: %{x1: x1, x2: {x2}}}})
#Axon<
- inputs: %{"data" => nil}
+ ]
+ >
+}
Containers even support arbitrary nesting:
out = Axon.container({%{x1: {x1, x2}, x2: %{x1: x1, x2: {x2}}}})
#Axon<
+ inputs: %{"data" => nil}
outputs: "container_0"
nodes: 6
->
{init_fn, predict_fn} = Axon.build(out)
-params = init_fn.(template, %{})
-predict_fn.(params, Nx.iota({2, 8}, type: :f32))
{%{
- x1: {#Nx.Tensor<
- f32[2][32]
- [
- [3.9104199409484863, 0.0, 0.0, 0.0, 0.0, 0.0, 0.0, 0.0, 0.0, 2.051666498184204, 0.0, 1.086042881011963, 0.6107193827629089, 0.5136545896530151, 2.7927842140197754, 0.0, 0.0, 0.0, 0.0, 2.472961902618408, 0.13712915778160095, 0.49807000160217285, 1.7868735790252686, 5.796293258666992, 0.0, 0.0, 4.727283477783203, 0.0, 0.0, 2.129516363143921, 0.0, 0.0],
- [11.746908187866211, 0.0, 0.0, 0.0, 0.0, 0.0, 0.0, 0.0, 0.0, 4.840534687042236, 0.0, 0.0, 4.103122711181641, 1.0597835779190063, 8.971627235412598, ...]
- ]
- >,
- #Nx.Tensor<
- f32[2][64]
- [
- [0.951026439666748, 0.0, 0.6895619034767151, 0.12973949313163757, 3.0561492443084717, 0.0, 0.21812109649181366, 0.0, 0.6377829313278198, 0.0, 0.0, 0.0, 0.0, 1.6837494373321533, 0.0, 0.0, 0.0, 1.3907173871994019, 0.0, 0.0, 0.21352148056030273, 0.0, 1.2145031690597534, 0.0, 3.080430507659912, 0.0, 3.9572620391845703, 2.3347463607788086, 0.5280991196632385, 0.0, 0.0, 0.0, 0.0, 0.0, 0.0, 1.616438627243042, 0.0, 1.1335082054138184, 2.228783369064331, 0.0, 1.0927692651748657, 0.0, 0.0, 0.0, 0.0, 2.7719650268554688, ...],
+>
{init_fn, predict_fn} = Axon.build(out)
+params = init_fn.(template, %{})
+predict_fn.(params, Nx.iota({2, 8}, type: :f32))
{%{
+ x1: {#Nx.Tensor<
+ f32[2][32]
+ [
+ [3.9104199409484863, 0.0, 0.0, 0.0, 0.0, 0.0, 0.0, 0.0, 0.0, 2.051666498184204, 0.0, 1.086042881011963, 0.6107193827629089, 0.5136545896530151, 2.7927842140197754, 0.0, 0.0, 0.0, 0.0, 2.472961902618408, 0.13712915778160095, 0.49807000160217285, 1.7868735790252686, 5.796293258666992, 0.0, 0.0, 4.727283477783203, 0.0, 0.0, 2.129516363143921, 0.0, 0.0],
+ [11.746908187866211, 0.0, 0.0, 0.0, 0.0, 0.0, 0.0, 0.0, 0.0, 4.840534687042236, 0.0, 0.0, 4.103122711181641, 1.0597835779190063, 8.971627235412598, ...]
+ ]
+ >,
+ #Nx.Tensor<
+ f32[2][64]
+ [
+ [0.951026439666748, 0.0, 0.6895619034767151, 0.12973949313163757, 3.0561492443084717, 0.0, 0.21812109649181366, 0.0, 0.6377829313278198, 0.0, 0.0, 0.0, 0.0, 1.6837494373321533, 0.0, 0.0, 0.0, 1.3907173871994019, 0.0, 0.0, 0.21352148056030273, 0.0, 1.2145031690597534, 0.0, 3.080430507659912, 0.0, 3.9572620391845703, 2.3347463607788086, 0.5280991196632385, 0.0, 0.0, 0.0, 0.0, 0.0, 0.0, 1.616438627243042, 0.0, 1.1335082054138184, 2.228783369064331, 0.0, 1.0927692651748657, 0.0, 0.0, 0.0, 0.0, 2.7719650268554688, ...],
...
- ]
- >},
- x2: %{
- x1: #Nx.Tensor<
- f32[2][32]
- [
- [3.9104199409484863, 0.0, 0.0, 0.0, 0.0, 0.0, 0.0, 0.0, 0.0, 2.051666498184204, 0.0, 1.086042881011963, 0.6107193827629089, 0.5136545896530151, 2.7927842140197754, 0.0, 0.0, 0.0, 0.0, 2.472961902618408, 0.13712915778160095, 0.49807000160217285, 1.7868735790252686, 5.796293258666992, 0.0, 0.0, 4.727283477783203, 0.0, 0.0, 2.129516363143921, 0.0, 0.0],
- [11.746908187866211, 0.0, 0.0, 0.0, 0.0, 0.0, 0.0, 0.0, 0.0, 4.840534687042236, 0.0, 0.0, 4.103122711181641, 1.0597835779190063, ...]
- ]
- >,
- x2: {#Nx.Tensor<
- f32[2][64]
- [
- [0.951026439666748, 0.0, 0.6895619034767151, 0.12973949313163757, 3.0561492443084717, 0.0, 0.21812109649181366, 0.0, 0.6377829313278198, 0.0, 0.0, 0.0, 0.0, 1.6837494373321533, 0.0, 0.0, 0.0, 1.3907173871994019, 0.0, 0.0, 0.21352148056030273, 0.0, 1.2145031690597534, 0.0, 3.080430507659912, 0.0, 3.9572620391845703, 2.3347463607788086, 0.5280991196632385, 0.0, 0.0, 0.0, 0.0, 0.0, 0.0, 1.616438627243042, 0.0, 1.1335082054138184, 2.228783369064331, 0.0, 1.0927692651748657, 0.0, 0.0, 0.0, ...],
+ ]
+ >},
+ x2: %{
+ x1: #Nx.Tensor<
+ f32[2][32]
+ [
+ [3.9104199409484863, 0.0, 0.0, 0.0, 0.0, 0.0, 0.0, 0.0, 0.0, 2.051666498184204, 0.0, 1.086042881011963, 0.6107193827629089, 0.5136545896530151, 2.7927842140197754, 0.0, 0.0, 0.0, 0.0, 2.472961902618408, 0.13712915778160095, 0.49807000160217285, 1.7868735790252686, 5.796293258666992, 0.0, 0.0, 4.727283477783203, 0.0, 0.0, 2.129516363143921, 0.0, 0.0],
+ [11.746908187866211, 0.0, 0.0, 0.0, 0.0, 0.0, 0.0, 0.0, 0.0, 4.840534687042236, 0.0, 0.0, 4.103122711181641, 1.0597835779190063, ...]
+ ]
+ >,
+ x2: {#Nx.Tensor<
+ f32[2][64]
+ [
+ [0.951026439666748, 0.0, 0.6895619034767151, 0.12973949313163757, 3.0561492443084717, 0.0, 0.21812109649181366, 0.0, 0.6377829313278198, 0.0, 0.0, 0.0, 0.0, 1.6837494373321533, 0.0, 0.0, 0.0, 1.3907173871994019, 0.0, 0.0, 0.21352148056030273, 0.0, 1.2145031690597534, 0.0, 3.080430507659912, 0.0, 3.9572620391845703, 2.3347463607788086, 0.5280991196632385, 0.0, 0.0, 0.0, 0.0, 0.0, 0.0, 1.616438627243042, 0.0, 1.1335082054138184, 2.228783369064331, 0.0, 1.0927692651748657, 0.0, 0.0, 0.0, ...],
...
- ]
- >}
- }
- }}
+ ]
+ >}
+ }
+ }}
diff --git a/onnx_to_axon.html b/onnx_to_axon.html
index 4e723d71..ea10f3f7 100644
--- a/onnx_to_axon.html
+++ b/onnx_to_axon.html
@@ -115,18 +115,18 @@
-Mix.install(
- [
- {:nx, "~> 0.3"},
- {:axon, "~> 0.2"},
- {:exla, "~> 0.3"},
- {:axon_onnx, "~> 0.2"},
- {:stb_image, "~> 0.5"},
- {:kino, "~> 0.7.0"}
- ],
+Mix.install(
+ [
+ {:nx, "~> 0.3"},
+ {:axon, "~> 0.2"},
+ {:exla, "~> 0.3"},
+ {:axon_onnx, "~> 0.2"},
+ {:stb_image, "~> 0.5"},
+ {:kino, "~> 0.7.0"}
+ ],
# change to "cuda111" for Nvidia GPU
- system_env: %{"XLA_TARGET" => xla_target}
-)
+
system_env: %{"XLA_TARGET" => xla_target}
+)
converting-an-onnx-model-into-axon
@@ -162,11 +162,11 @@
axon_onnx
.
You can find all dependencies in the installation cell at the top of the notebook.
In there, you will also find the XLA_TARGET
environment variable whick you can set
to "cuda111" or "rocm" if you have any of those GPUs available. Let's also configure
-Nx to store tensors in EXLA by default:
Nx.default_backend(EXLA.Backend)
We'll also need local access to ONNX files. For this notebook, the models/onnx folder
+Nx to store tensors in EXLA by default:
Nx.default_backend(EXLA.Backend)
We'll also need local access to ONNX files. For this notebook, the models/onnx folder
contains the ONNX model file. This notebook assumes the output file location will be
in models axon. Copy your ONNX model files into the models/onnx folder.
This opinionated module presents a simple API for loading in an ONNX file and saving
the converted Axon model in the provided directory. This API will allow us to
-save multiple models pretty quickly.
defmodule OnnxToAxon do
+save multiple models pretty quickly.defmodule OnnxToAxon do
@moduledoc """
Helper module from ONNX to Axon.
"""
@@ -179,40 +179,40 @@
iex> OnnxToAxon.onnx_axon(path_to_onnx_file, path_to_axon_dir)
"""
- def onnx_axon(path_to_onnx_file, path_to_axon_dir) do
- axon_name = axon_name_from_onnx_path(path_to_onnx_file)
- path_to_axon = Path.join(path_to_axon_dir, axon_name)
-
- {model, parameters} = AxonOnnx.import(path_to_onnx_file)
- model_bytes = Axon.serialize(model, parameters)
- File.write!(path_to_axon, model_bytes)
- end
-
- defp axon_name_from_onnx_path(onnx_path) do
- model_root = onnx_path |> Path.basename() |> Path.rootname()
- "#{model_root}.axon"
- end
-end
+
def onnx_axon(path_to_onnx_file, path_to_axon_dir) do
+ axon_name = axon_name_from_onnx_path(path_to_onnx_file)
+ path_to_axon = Path.join(path_to_axon_dir, axon_name)
+
+ {model, parameters} = AxonOnnx.import(path_to_onnx_file)
+ model_bytes = Axon.serialize(model, parameters)
+ File.write!(path_to_axon, model_bytes)
+ end
+
+ defp axon_name_from_onnx_path(onnx_path) do
+ model_root = onnx_path |> Path.basename() |> Path.rootname()
+ "#{model_root}.axon"
+ end
+end
onnx-model
ONNX model
-For this example, we'll use a couple ONNX models that have been saved in the Huggingface Hub.
The ONNX models were trained in Fast.ai (PyTorch) using the following notebooks:
- https://github.com/meanderingstream/fastai_course22/blob/main/saving-a-basic-fastai-model-in-onnx.ipynb
- https://github.com/meanderingstream/fastai_course22/blob/main/saving-cat-dog-breed-fastai-model-in-onnx.ipynb
To repeat this notebook, the onnx files for this notebook can be found on huggingface hub. Download the onnx models from:
- https://huggingface.co/ScottMueller/Cats_v_Dogs.ONNX
- https://huggingface.co/ScottMueller/Cat_Dog_Breeds.ONNX
Download the files and place them in a directory of your choice. By default, we will assume you downloaded them to the same directory as the notebook:
File.cd!(__DIR__)
Now let's convert an ONNX model into Axon
path_to_onnx_file = "models/onnx/cats_v_dogs.onnx"
+For this example, we'll use a couple ONNX models that have been saved in the Huggingface Hub.
The ONNX models were trained in Fast.ai (PyTorch) using the following notebooks:
- https://github.com/meanderingstream/fastai_course22/blob/main/saving-a-basic-fastai-model-in-onnx.ipynb
- https://github.com/meanderingstream/fastai_course22/blob/main/saving-cat-dog-breed-fastai-model-in-onnx.ipynb
To repeat this notebook, the onnx files for this notebook can be found on huggingface hub. Download the onnx models from:
- https://huggingface.co/ScottMueller/Cats_v_Dogs.ONNX
- https://huggingface.co/ScottMueller/Cat_Dog_Breeds.ONNX
Download the files and place them in a directory of your choice. By default, we will assume you downloaded them to the same directory as the notebook:
File.cd!(__DIR__)
Now let's convert an ONNX model into Axon
path_to_onnx_file = "models/onnx/cats_v_dogs.onnx"
path_to_axon_dir = "models/axon"
-OnnxToAxon.onnx_axon(path_to_onnx_file, path_to_axon_dir)
path_to_onnx_file = "models/onnx/cat_dog_breeds.onnx"
+OnnxToAxon.onnx_axon(path_to_onnx_file, path_to_axon_dir)
path_to_onnx_file = "models/onnx/cat_dog_breeds.onnx"
path_to_axon_dir = "models/axon"
-OnnxToAxon.onnx_axon(path_to_onnx_file, path_to_axon_dir)
+
OnnxToAxon.onnx_axon(path_to_onnx_file, path_to_axon_dir)
inference-on-onnx-derived-models
Inference on ONNX derived models
-To run inference on the model, you'll need 10 images focused on cats or dogs. You can download the images used in training the model at:
"https://s3.amazonaws.com/fast-ai-imageclas/oxford-iiit-pet.tgz"
Or you can find or use your own images. In this notebook, we are going to use the local copies of the Oxford Pets dataset that was used in training the model.
Let's load the Axon model.
cats_v_dogs = File.read!("models/axon/cats_v_dogs.axon")
-{cats_v_dogs_model, cats_v_dogs_params} = Axon.deserialize(cats_v_dogs)
We need a tensor representation of an image. Let's start by looking at samples of
-our data.
File.read!("data/oxford-iiit-pet/images/havanese_71.jpg")
-|> Kino.Image.new(:jpeg)
To manipulate the images, we will use the StbImage
library:
{:ok, img} = StbImage.read_file("data/oxford-iiit-pet/images/havanese_71.jpg")
-%StbImage{data: binary, shape: shape, type: type} = StbImage.resize(img, 224, 224)
Now let's work on a batch of images and convert them to tensors. Here are the images we will work with:
file_names = [
+To run inference on the model, you'll need 10 images focused on cats or dogs. You can download the images used in training the model at:
"https://s3.amazonaws.com/fast-ai-imageclas/oxford-iiit-pet.tgz"
Or you can find or use your own images. In this notebook, we are going to use the local copies of the Oxford Pets dataset that was used in training the model.
Let's load the Axon model.
cats_v_dogs = File.read!("models/axon/cats_v_dogs.axon")
+{cats_v_dogs_model, cats_v_dogs_params} = Axon.deserialize(cats_v_dogs)
We need a tensor representation of an image. Let's start by looking at samples of
+our data.
File.read!("data/oxford-iiit-pet/images/havanese_71.jpg")
+|> Kino.Image.new(:jpeg)
To manipulate the images, we will use the StbImage
library:
{:ok, img} = StbImage.read_file("data/oxford-iiit-pet/images/havanese_71.jpg")
+%StbImage{data: binary, shape: shape, type: type} = StbImage.resize(img, 224, 224)
Now let's work on a batch of images and convert them to tensors. Here are the images we will work with:
file_names = [
"havanese_71.jpg",
"yorkshire_terrier_9.jpg",
"Sphynx_206.jpg",
@@ -223,18 +223,18 @@
"British_Shorthair_122.jpg",
"Russian_Blue_20.jpg",
"boxer_99.jpg"
-]
Next we resize the images:
resized_images =
- Enum.map(file_names, fn file_name ->
- ("data/oxford-iiit-pet/images/" <> file_name)
- |> IO.inspect(label: file_name)
- |> StbImage.read_file!()
- |> StbImage.resize(224, 224)
- end)
And finally convert them into tensors by using StbImage.to_nx/1
. The created tensor will have three axes, named :height
, :width
, and :channel
respectively. Our goal is to stack the tensors, then normalize and transpose their axes to the order expected by the neural network:
img_tensors =
+]
Next we resize the images:
resized_images =
+ Enum.map(file_names, fn file_name ->
+ ("data/oxford-iiit-pet/images/" <> file_name)
+ |> IO.inspect(label: file_name)
+ |> StbImage.read_file!()
+ |> StbImage.resize(224, 224)
+ end)
And finally convert them into tensors by using StbImage.to_nx/1
. The created tensor will have three axes, named :height
, :width
, and :channel
respectively. Our goal is to stack the tensors, then normalize and transpose their axes to the order expected by the neural network:
img_tensors =
resized_images
- |> Enum.map(&StbImage.to_nx/1)
- |> Nx.stack(name: :index)
- |> Nx.divide(255.0)
- |> Nx.transpose(axes: [:index, :channels, :height, :width])
With our input data, it is finally time to work on predictions. First let's define a helper module:
defmodule Predictions do
+ |> Enum.map(&StbImage.to_nx/1)
+ |> Nx.stack(name: :index)
+ |> Nx.divide(255.0)
+ |> Nx.transpose(axes: [:index, :channels, :height, :width])
With our input data, it is finally time to work on predictions. First let's define a helper module:
defmodule Predictions do
@doc """
When provided a Tensor of single label predictions, returns the best vocabulary match for
each row in the prediction tensor.
@@ -245,26 +245,26 @@
["dog", "cat", "dog"]
"""
- def single_label_classification(predictions_batch, vocabulary) do
- IO.inspect(Nx.shape(predictions_batch), label: "predictions batch shape")
+ def single_label_classification(predictions_batch, vocabulary) do
+ IO.inspect(Nx.shape(predictions_batch), label: "predictions batch shape")
- for prediction_tensor <- Nx.to_batched(predictions_batch) do
- {_prediction_value, prediction_label} =
+ for prediction_tensor <- Nx.to_batched(predictions_batch) do
+ {_prediction_value, prediction_label} =
prediction_tensor
- |> Nx.to_flat_list()
- |> Enum.zip(vocabulary)
- |> Enum.max()
+ |> Nx.to_flat_list()
+ |> Enum.zip(vocabulary)
+ |> Enum.max()
prediction_label
- end
- end
-end
Now we deserialize the model
{cats_v_dogs_model, cats_v_dogs_params} = Axon.deserialize(cats_v_dogs)
run a prediction using the EXLA
compiler for performance
tensor_of_predictions =
- Axon.predict(cats_v_dogs_model, cats_v_dogs_params, img_tensors, compiler: EXLA)
and finally retrieve the predicted label
dog_cat_vocabulary = [
+ end
+ end
+end
Now we deserialize the model
{cats_v_dogs_model, cats_v_dogs_params} = Axon.deserialize(cats_v_dogs)
run a prediction using the EXLA
compiler for performance
tensor_of_predictions =
+ Axon.predict(cats_v_dogs_model, cats_v_dogs_params, img_tensors, compiler: EXLA)
and finally retrieve the predicted label
dog_cat_vocabulary = [
"dog",
"cat"
-]
+]
-Predictions.single_label_classification(tensor_of_predictions, dog_cat_vocabulary)
Let's repeat the above process for the dog and cat breed model.
cat_dog_vocabulary = [
+Predictions.single_label_classification(tensor_of_predictions, dog_cat_vocabulary)
Let's repeat the above process for the dog and cat breed model.
cat_dog_vocabulary = [
"abyssinian",
"american_bulldog",
"american_pit_bull_terrier",
@@ -302,9 +302,9 @@
"staffordshire_bull_terrier",
"wheaten_terrier",
"yorkshire_terrier"
-]
cat_dog_breeds = File.read!("models/axon/cat_dog_breeds.axon")
-{cat_dog_breeds_model, cat_dog_breeds_params} = Axon.deserialize(cat_dog_breeds)
Axon.predict(cat_dog_breeds_model, cat_dog_breeds_params, img_tensors)
-|> Predictions.single_label_classification(cat_dog_vocabulary)
For cat and dog breeds, the model performed pretty well, but it was not perfect.
+]
cat_dog_breeds = File.read!("models/axon/cat_dog_breeds.axon")
+{cat_dog_breeds_model, cat_dog_breeds_params} = Axon.deserialize(cat_dog_breeds)
Axon.predict(cat_dog_breeds_model, cat_dog_breeds_params, img_tensors)
+|> Predictions.single_label_classification(cat_dog_vocabulary)
For cat and dog breeds, the model performed pretty well, but it was not perfect.
diff --git a/sequential_models.html b/sequential_models.html
index 41362aec..72bcee96 100644
--- a/sequential_models.html
+++ b/sequential_models.html
@@ -115,31 +115,31 @@
-Mix.install([
- {:axon, github: "elixir-nx/axon"},
- {:nx, "~> 0.3.0", github: "elixir-nx/nx", sparse: "nx", override: true},
- {:kino, "~> 0.7.0"}
-])
:ok
+Mix.install([
+ {:axon, github: "elixir-nx/axon"},
+ {:nx, "~> 0.3.0", github: "elixir-nx/nx", sparse: "nx", override: true},
+ {:kino, "~> 0.7.0"}
+])
:ok
creating-a-sequential-model
Creating a sequential model
In the last guide, you created a simple identity model which just returned the input. Of course, you would never actually use Axon for such purposes. You want to create real neural networks!
In equivalent frameworks in the Python ecosystem such as Keras and PyTorch, there is a concept of sequential models. Sequential models are named after the sequential nature in which data flows through them. Sequential models transform the input with sequential, successive transformations.
If you're an experienced Elixir programmer, this paradigm of sequential transformations might sound a lot like what happens when using the pipe (|>
) operator. In Elixir, it's common to see code blocks like:
list
-|> Enum.map(fn x -> x + 1 end)
-|> Enum.filter(&rem(&1, 2) == 0)
-|> Enum.count()
The snippet above passes list
through a sequence of transformations. You can apply this same paradigm in Axon to create sequential models. In fact, creating sequential models is so natural with Elixir's pipe operator, that Axon does not need a distinct sequential construct. To create a sequential model, you just pass Axon models through successive transformations in the Axon API:
model =
- Axon.input("data")
- |> Axon.dense(32)
- |> Axon.activation(:relu)
- |> Axon.dropout(rate: 0.5)
- |> Axon.dense(1)
- |> Axon.activation(:softmax)
#Axon<
- inputs: %{"data" => nil}
+|> Enum.map(fn x -> x + 1 end)
+|> Enum.filter(&rem(&1, 2) == 0)
+|> Enum.count()
The snippet above passes list
through a sequence of transformations. You can apply this same paradigm in Axon to create sequential models. In fact, creating sequential models is so natural with Elixir's pipe operator, that Axon does not need a distinct sequential construct. To create a sequential model, you just pass Axon models through successive transformations in the Axon API:
model =
+ Axon.input("data")
+ |> Axon.dense(32)
+ |> Axon.activation(:relu)
+ |> Axon.dropout(rate: 0.5)
+ |> Axon.dense(1)
+ |> Axon.activation(:softmax)
#Axon<
+ inputs: %{"data" => nil}
outputs: "softmax_0"
nodes: 6
->
If you visualize this model, it's easy to see how data flows sequentially through it:
template = Nx.template({2, 16}, :f32)
-Axon.Display.as_graph(model, template)
graph TD;
+>
If you visualize this model, it's easy to see how data flows sequentially through it:
template = Nx.template({2, 16}, :f32)
+Axon.Display.as_graph(model, template)
graph TD;
3[/"data (:input) {2, 16}"/];
6["dense_0 (:dense) {2, 32}"];
7["relu_0 (:relu) {2, 32}"];
@@ -150,72 +150,72 @@
8 --> 11;
7 --> 8;
6 --> 7;
-3 --> 6;
Your model is more involved and as a result so is the execution graph! Now, using the same constructs from the last section, you can build and run your model:
{init_fn, predict_fn} = Axon.build(model)
{#Function<137.55749718/2 in Nx.Defn.wrap_arity/2>,
- #Function<137.55749718/2 in Nx.Defn.wrap_arity/2>}
params = init_fn.(template, %{})
%{
- "dense_0" => %{
- "bias" => #Nx.Tensor<
- f32[32]
- [0.0, 0.0, 0.0, 0.0, 0.0, 0.0, 0.0, 0.0, 0.0, 0.0, 0.0, 0.0, 0.0, 0.0, 0.0, 0.0, 0.0, 0.0, 0.0, 0.0, 0.0, 0.0, 0.0, 0.0, 0.0, 0.0, 0.0, 0.0, 0.0, 0.0, 0.0, 0.0]
- >,
- "kernel" => #Nx.Tensor<
- f32[16][32]
- [
- [-0.25727564096450806, -0.31299564242362976, -0.1557893306016922, -0.3321501314640045, 0.34875044226646423, 0.15635445713996887, 0.25805917382240295, 0.316285640001297, 0.29047688841819763, -0.09108144044876099, 0.2781231701374054, 0.21326711773872375, -0.29581472277641296, -0.3105146288871765, -0.11265464127063751, 0.054490894079208374, -0.22294805943965912, 0.23276928067207336, 0.06426036357879639, 0.12059605121612549, -0.24530324339866638, 0.061366915702819824, 0.17463091015815735, -0.2774006724357605, 0.2621242105960846, 0.19262376427650452, -0.10884760320186615, -0.3156566321849823, 0.104307621717453, -0.22591334581375122, -0.09672778844833374, -0.18450938165187836],
- [-0.32328563928604126, -0.3434811234474182, -0.3464450538158417, 0.14756330847740173, 0.010595977306365967, 0.32808688282966614, -0.3048470616340637, 0.011142522096633911, 0.10394474864006042, 0.04501914978027344, -0.26296690106391907, -0.1051199734210968, -0.0060880184173583984, 0.22103646397590637, -0.3040429651737213, ...],
+3 --> 6;
Your model is more involved and as a result so is the execution graph! Now, using the same constructs from the last section, you can build and run your model:
{init_fn, predict_fn} = Axon.build(model)
{#Function<137.55749718/2 in Nx.Defn.wrap_arity/2>,
+ #Function<137.55749718/2 in Nx.Defn.wrap_arity/2>}
params = init_fn.(template, %{})
%{
+ "dense_0" => %{
+ "bias" => #Nx.Tensor<
+ f32[32]
+ [0.0, 0.0, 0.0, 0.0, 0.0, 0.0, 0.0, 0.0, 0.0, 0.0, 0.0, 0.0, 0.0, 0.0, 0.0, 0.0, 0.0, 0.0, 0.0, 0.0, 0.0, 0.0, 0.0, 0.0, 0.0, 0.0, 0.0, 0.0, 0.0, 0.0, 0.0, 0.0]
+ >,
+ "kernel" => #Nx.Tensor<
+ f32[16][32]
+ [
+ [-0.25727564096450806, -0.31299564242362976, -0.1557893306016922, -0.3321501314640045, 0.34875044226646423, 0.15635445713996887, 0.25805917382240295, 0.316285640001297, 0.29047688841819763, -0.09108144044876099, 0.2781231701374054, 0.21326711773872375, -0.29581472277641296, -0.3105146288871765, -0.11265464127063751, 0.054490894079208374, -0.22294805943965912, 0.23276928067207336, 0.06426036357879639, 0.12059605121612549, -0.24530324339866638, 0.061366915702819824, 0.17463091015815735, -0.2774006724357605, 0.2621242105960846, 0.19262376427650452, -0.10884760320186615, -0.3156566321849823, 0.104307621717453, -0.22591334581375122, -0.09672778844833374, -0.18450938165187836],
+ [-0.32328563928604126, -0.3434811234474182, -0.3464450538158417, 0.14756330847740173, 0.010595977306365967, 0.32808688282966614, -0.3048470616340637, 0.011142522096633911, 0.10394474864006042, 0.04501914978027344, -0.26296690106391907, -0.1051199734210968, -0.0060880184173583984, 0.22103646397590637, -0.3040429651737213, ...],
...
- ]
- >
- },
- "dense_1" => %{
- "bias" => #Nx.Tensor<
- f32[1]
- [0.0]
- >,
- "kernel" => #Nx.Tensor<
- f32[32][1]
- [
- [-0.379288911819458],
- [-0.05532142519950867],
- [-0.07836392521858215],
- [0.41381680965423584],
- [0.33221137523651123],
- [0.23515504598617554],
- [-0.40667685866355896],
- [-0.3503745198249817],
- [0.2631032466888428],
- [-0.13176566362380981],
- [-0.3811171054840088],
- [0.24656128883361816],
- [0.17257028818130493],
- [0.3528350591659546],
- [0.4112042784690857],
- [0.056196123361587524],
- [0.138421893119812],
- [-0.38378745317459106],
- [-0.044070273637771606],
- [0.11507803201675415],
- [-0.3125251233577728],
- [-0.11389034986495972],
- [-0.27444711327552795],
- [-0.30974721908569336],
- [-0.3695589303970337],
- [0.3146793246269226],
- [0.005854517221450806],
- [-0.03735968470573425],
- [0.02763468027114868],
- [-0.10707724094390869],
- [0.10824829339981079],
- [0.29013824462890625]
- ]
- >
- }
-}
Wow! Notice that this model actually has trainable parameters. You can see that the parameter map is just a regular Elixir map. Each top-level entry maps to a layer with a key corresponding to that layer's name and a value corresponding to that layer's trainable parameters. Each layer's individual trainable parameters are given layer-specific names and map directly to Nx tensors.
Now you can use these params
with your predict_fn
:
predict_fn.(params, Nx.iota({2, 16}, type: :f32))
#Nx.Tensor<
- f32[2][1]
- [
- [1.0],
- [1.0]
- ]
->
And voila! You've successfully created and used a sequential model in Axon!
+ ]
+ >
+ },
+ "dense_1" => %{
+ "bias" => #Nx.Tensor<
+ f32[1]
+ [0.0]
+ >,
+ "kernel" => #Nx.Tensor<
+ f32[32][1]
+ [
+ [-0.379288911819458],
+ [-0.05532142519950867],
+ [-0.07836392521858215],
+ [0.41381680965423584],
+ [0.33221137523651123],
+ [0.23515504598617554],
+ [-0.40667685866355896],
+ [-0.3503745198249817],
+ [0.2631032466888428],
+ [-0.13176566362380981],
+ [-0.3811171054840088],
+ [0.24656128883361816],
+ [0.17257028818130493],
+ [0.3528350591659546],
+ [0.4112042784690857],
+ [0.056196123361587524],
+ [0.138421893119812],
+ [-0.38378745317459106],
+ [-0.044070273637771606],
+ [0.11507803201675415],
+ [-0.3125251233577728],
+ [-0.11389034986495972],
+ [-0.27444711327552795],
+ [-0.30974721908569336],
+ [-0.3695589303970337],
+ [0.3146793246269226],
+ [0.005854517221450806],
+ [-0.03735968470573425],
+ [0.02763468027114868],
+ [-0.10707724094390869],
+ [0.10824829339981079],
+ [0.29013824462890625]
+ ]
+ >
+ }
+}Wow! Notice that this model actually has trainable parameters. You can see that the parameter map is just a regular Elixir map. Each top-level entry maps to a layer with a key corresponding to that layer's name and a value corresponding to that layer's trainable parameters. Each layer's individual trainable parameters are given layer-specific names and map directly to Nx tensors.
Now you can use these params
with your predict_fn
:
predict_fn.(params, Nx.iota({2, 16}, type: :f32))
#Nx.Tensor<
+ f32[2][1]
+ [
+ [1.0],
+ [1.0]
+ ]
+>
And voila! You've successfully created and used a sequential model in Axon!
diff --git a/training_and_inference_mode.html b/training_and_inference_mode.html
index 05428018..9ef212e6 100644
--- a/training_and_inference_mode.html
+++ b/training_and_inference_mode.html
@@ -115,87 +115,87 @@
-Mix.install([
- {:axon, github: "elixir-nx/axon"},
- {:nx, "~> 0.3.0", github: "elixir-nx/nx", sparse: "nx", override: true}
-])
:ok
+Mix.install([
+ {:axon, github: "elixir-nx/axon"},
+ {:nx, "~> 0.3.0", github: "elixir-nx/nx", sparse: "nx", override: true}
+])
:ok
executing-models-in-inference-mode
Executing models in inference mode
-
Some layers have different considerations and behavior when running during model training versus model inference. For example dropout layers are intended only to be used during training as a form of model regularization. Certain stateful layers like batch normalization keep a running-internal state which changes during training mode but remains fixed during inference mode. Axon supports mode-dependent execution behavior via the :mode
option passed to all building, compilation, and execution methods. By default, all models build in inference mode. You can see this behavior by adding a dropout layer with a dropout rate of 1. In inference mode this layer will have no affect:
inputs = Nx.iota({2, 8}, type: :f32)
+Some layers have different considerations and behavior when running during model training versus model inference. For example dropout layers are intended only to be used during training as a form of model regularization. Certain stateful layers like batch normalization keep a running-internal state which changes during training mode but remains fixed during inference mode. Axon supports mode-dependent execution behavior via the :mode
option passed to all building, compilation, and execution methods. By default, all models build in inference mode. You can see this behavior by adding a dropout layer with a dropout rate of 1. In inference mode this layer will have no affect:
inputs = Nx.iota({2, 8}, type: :f32)
model =
- Axon.input("data")
- |> Axon.dense(4)
- |> Axon.sigmoid()
- |> Axon.dropout(rate: 0.99)
- |> Axon.dense(1)
-
-{init_fn, predict_fn} = Axon.build(model)
-params = init_fn.(inputs, %{})
-predict_fn.(params, inputs)
#Nx.Tensor<
- f32[2][1]
- [
- [-0.6138466000556946],
- [-0.8409845232963562]
- ]
->
You can also explicitly specify the mode:
{init_fn, predict_fn} = Axon.build(model, mode: :inference)
-params = init_fn.(inputs, %{})
-predict_fn.(params, inputs)
#Nx.Tensor<
- f32[2][1]
- [
- [0.7551136016845703],
- [0.448221355676651]
- ]
->
It's important that you know which mode your model's were compiled for, as running a model built in :inference
mode will behave drastically different than a model built in :train
mode.
+
Axon.input("data")
+ |> Axon.dense(4)
+ |> Axon.sigmoid()
+ |> Axon.dropout(rate: 0.99)
+ |> Axon.dense(1)
+
+{init_fn, predict_fn} = Axon.build(model)
+params = init_fn.(inputs, %{})
+predict_fn.(params, inputs)
#Nx.Tensor<
+ f32[2][1]
+ [
+ [-0.6138466000556946],
+ [-0.8409845232963562]
+ ]
+>
You can also explicitly specify the mode:
{init_fn, predict_fn} = Axon.build(model, mode: :inference)
+params = init_fn.(inputs, %{})
+predict_fn.(params, inputs)
#Nx.Tensor<
+ f32[2][1]
+ [
+ [0.7551136016845703],
+ [0.448221355676651]
+ ]
+>
It's important that you know which mode your model's were compiled for, as running a model built in :inference
mode will behave drastically different than a model built in :train
mode.
executing-models-in-training-mode
Executing models in training mode
-By specifying mode: :train
, you tell your models to execute in training mode. You can see the effects of this behavior here:
{init_fn, predict_fn} = Axon.build(model, mode: :train)
-params = init_fn.(inputs, %{})
-predict_fn.(params, inputs)
%{
- prediction: #Nx.Tensor<
- f32[2][1]
- [
- [0.0],
- [0.0]
- ]
- >,
- state: %{}
-}
First, notice that your model now returns a map with keys :prediction
and :state
. :prediction
contains the actual model prediction, while :state
contains the updated state for any stateful layers such as batch norm. When writing custom training loops, you should extract :state
and use it in conjunction with the updates API to ensure your stateful layers are updated correctly. If your model has stateful layers, :state
will look similar to your model's parameter map:
model =
- Axon.input("data")
- |> Axon.dense(4)
- |> Axon.sigmoid()
- |> Axon.batch_norm()
- |> Axon.dense(1)
-
-{init_fn, predict_fn} = Axon.build(model, mode: :train)
-params = init_fn.(inputs, %{})
-predict_fn.(params, inputs)
%{
- prediction: #Nx.Tensor<
- f32[2][1]
- [
- [0.03675001487135887],
- [-0.03674999624490738]
- ]
- >,
- state: %{
- "batch_norm_0" => %{
- "mean" => #Nx.Tensor<
- f32[4]
- [0.8784151673316956, 0.7386987209320068, 0.663623571395874, 0.8947045803070068]
- >,
- "var" => #Nx.Tensor<
- f32[4]
- [0.10050597041845322, 0.11294332146644592, 0.16061438620090485, 0.10003116726875305]
- >
- }
- }
-}
+By specifying mode: :train
, you tell your models to execute in training mode. You can see the effects of this behavior here:
{init_fn, predict_fn} = Axon.build(model, mode: :train)
+params = init_fn.(inputs, %{})
+predict_fn.(params, inputs)
%{
+ prediction: #Nx.Tensor<
+ f32[2][1]
+ [
+ [0.0],
+ [0.0]
+ ]
+ >,
+ state: %{}
+}
First, notice that your model now returns a map with keys :prediction
and :state
. :prediction
contains the actual model prediction, while :state
contains the updated state for any stateful layers such as batch norm. When writing custom training loops, you should extract :state
and use it in conjunction with the updates API to ensure your stateful layers are updated correctly. If your model has stateful layers, :state
will look similar to your model's parameter map:
model =
+ Axon.input("data")
+ |> Axon.dense(4)
+ |> Axon.sigmoid()
+ |> Axon.batch_norm()
+ |> Axon.dense(1)
+
+{init_fn, predict_fn} = Axon.build(model, mode: :train)
+params = init_fn.(inputs, %{})
+predict_fn.(params, inputs)
%{
+ prediction: #Nx.Tensor<
+ f32[2][1]
+ [
+ [0.03675001487135887],
+ [-0.03674999624490738]
+ ]
+ >,
+ state: %{
+ "batch_norm_0" => %{
+ "mean" => #Nx.Tensor<
+ f32[4]
+ [0.8784151673316956, 0.7386987209320068, 0.663623571395874, 0.8947045803070068]
+ >,
+ "var" => #Nx.Tensor<
+ f32[4]
+ [0.10050597041845322, 0.11294332146644592, 0.16061438620090485, 0.10003116726875305]
+ >
+ }
+ }
+}
diff --git a/using_loop_event_handlers.html b/using_loop_event_handlers.html
index 27d981e2..494d67a8 100644
--- a/using_loop_event_handlers.html
+++ b/using_loop_event_handlers.html
@@ -115,16 +115,16 @@
-Mix.install([
- {:axon, github: "elixir-nx/axon"},
- {:nx, "~> 0.3.0", github: "elixir-nx/nx", sparse: "nx", override: true}
-])
:ok
+Mix.install([
+ {:axon, github: "elixir-nx/axon"},
+ {:nx, "~> 0.3.0", github: "elixir-nx/nx", sparse: "nx", override: true}
+])
:ok
adding-event-handlers-to-training-loops
Adding event handlers to training loops
-
Often times you want more fine-grained control over things that happen during loop execution. For example, you might want to save loop state to a file every 500 iterations, or log some output to :stdout
at the end of every epoch. Axon loops allow more fine-grained control via events and event handlers.
Axon fires a number of events during loop execution which allow you to instrument various points in the loop execution cycle. You can attach event handlers to any of these events:
events = [
+Often times you want more fine-grained control over things that happen during loop execution. For example, you might want to save loop state to a file every 500 iterations, or log some output to :stdout
at the end of every epoch. Axon loops allow more fine-grained control via events and event handlers.
Axon fires a number of events during loop execution which allow you to instrument various points in the loop execution cycle. You can attach event handlers to any of these events:
events = [
:started, # After loop state initialization
:epoch_started, # On epoch start
:iteration_started, # On iteration start
@@ -133,103 +133,103 @@
:epoch_halted, # On epoch halt, if early halted
:halted, # On loop halt, if early halted
:completed # On loop completion
-]
Axon packages a number of common loop event handlers for you out of the box. These handlers should cover most of the common event handlers you would need to write in practice. Axon also allows for custom event handlers. See Writing custom event handlers for more information.
An event handler will take the current loop state at the time of the fired event, and alter or use it in someway before returning control back to the main loop execution. You can attach any of Axon's pre-packaged event handlers to a loop by using the function directly. For example, if you want to checkpoint loop state at the end of every epoch, you can use Axon.Loop.checkpoint/2
:
model =
- Axon.input("data")
- |> Axon.dense(8)
- |> Axon.relu()
- |> Axon.dense(4)
- |> Axon.relu()
- |> Axon.dense(1)
+]
Axon packages a number of common loop event handlers for you out of the box. These handlers should cover most of the common event handlers you would need to write in practice. Axon also allows for custom event handlers. See Writing custom event handlers for more information.
An event handler will take the current loop state at the time of the fired event, and alter or use it in someway before returning control back to the main loop execution. You can attach any of Axon's pre-packaged event handlers to a loop by using the function directly. For example, if you want to checkpoint loop state at the end of every epoch, you can use Axon.Loop.checkpoint/2
:
model =
+ Axon.input("data")
+ |> Axon.dense(8)
+ |> Axon.relu()
+ |> Axon.dense(4)
+ |> Axon.relu()
+ |> Axon.dense(1)
loop =
model
- |> Axon.Loop.trainer(:mean_squared_error, :sgd)
- |> Axon.Loop.checkpoint(event: :epoch_completed)
#Axon.Loop<
- handlers: %{
- completed: [],
- epoch_completed: [
- {#Function<14.20267452/1 in Axon.Loop.checkpoint/2>,
- #Function<5.20267452/1 in Axon.Loop.build_filter_fn/1>},
- {#Function<23.20267452/1 in Axon.Loop.log/5>,
- #Function<5.20267452/1 in Axon.Loop.build_filter_fn/1>}
- ],
- epoch_halted: [],
- epoch_started: [],
- halted: [],
- iteration_completed: [
- {#Function<23.20267452/1 in Axon.Loop.log/5>,
- #Function<3.20267452/1 in Axon.Loop.build_filter_fn/1>}
- ],
- iteration_started: [],
- started: []
- },
- metrics: %{
- "loss" => {#Function<12.6031754/3 in Axon.Metrics.running_average/1>,
- #Function<6.20267452/2 in Axon.Loop.build_loss_fn/1>}
- },
+ |> Axon.Loop.trainer(:mean_squared_error, :sgd)
+ |> Axon.Loop.checkpoint(event: :epoch_completed)
#Axon.Loop<
+ handlers: %{
+ completed: [],
+ epoch_completed: [
+ {#Function<14.20267452/1 in Axon.Loop.checkpoint/2>,
+ #Function<5.20267452/1 in Axon.Loop.build_filter_fn/1>},
+ {#Function<23.20267452/1 in Axon.Loop.log/5>,
+ #Function<5.20267452/1 in Axon.Loop.build_filter_fn/1>}
+ ],
+ epoch_halted: [],
+ epoch_started: [],
+ halted: [],
+ iteration_completed: [
+ {#Function<23.20267452/1 in Axon.Loop.log/5>,
+ #Function<3.20267452/1 in Axon.Loop.build_filter_fn/1>}
+ ],
+ iteration_started: [],
+ started: []
+ },
+ metrics: %{
+ "loss" => {#Function<12.6031754/3 in Axon.Metrics.running_average/1>,
+ #Function<6.20267452/2 in Axon.Loop.build_loss_fn/1>}
+ },
...
->
Now when you execute your loop, it will save a checkpoint at the end of every epoch:
train_data =
- Stream.repeatedly(fn ->
- xs = Nx.random_normal({8, 1})
- ys = Nx.sin(xs)
- {xs, ys}
- end)
-
-Axon.Loop.run(loop, train_data, %{}, epochs: 5, iterations: 100)
Epoch: 0, Batch: 100, loss: 0.2462310
+>
Now when you execute your loop, it will save a checkpoint at the end of every epoch:
train_data =
+ Stream.repeatedly(fn ->
+ xs = Nx.random_normal({8, 1})
+ ys = Nx.sin(xs)
+ {xs, ys}
+ end)
+
+Axon.Loop.run(loop, train_data, %{}, epochs: 5, iterations: 100)
Epoch: 0, Batch: 100, loss: 0.2462310
Epoch: 1, Batch: 100, loss: 0.1804814
Epoch: 2, Batch: 100, loss: 0.1452925
Epoch: 3, Batch: 100, loss: 0.1177117
-Epoch: 4, Batch: 100, loss: 0.1008184
%{
- "dense_0" => %{
- "bias" => #Nx.Tensor<
- f32[8]
- [0.36853691935539246, 0.24528849124908447, 0.13193830847740173, 0.03188902884721756, -0.06358373910188675, 0.044517479836940765, -0.1203451156616211, -6.352089694701135e-4]
- >,
- "kernel" => #Nx.Tensor<
- f32[1][8]
- [
- [0.49448737502098083, 0.5250089764595032, 0.7132464051246643, 0.47473379969596863, -0.043285828083753586, -0.14137212932109833, -0.07576408237218857, -0.48898136615753174]
- ]
- >
- },
- "dense_1" => %{
- "bias" => #Nx.Tensor<
- f32[4]
- [0.30324652791023254, 0.0385407879948616, -0.16782516241073608, 0.1984063982963562]
- >,
- "kernel" => #Nx.Tensor<
- f32[8][4]
- [
- [0.2536502778530121, 0.375381737947464, 0.7119463086128235, -0.14521682262420654],
- [0.20504063367843628, -0.11605211347341537, 0.49423739314079285, -0.03246872499585152],
- [-0.13834621012210846, -0.2579476833343506, 0.34836748242378235, -0.4670639634132385],
- [-0.11925031989812851, -0.6655324697494507, 0.5057039856910706, 0.496115118265152],
- [0.15856991708278656, -0.2239169478416443, 0.5550385117530823, -0.3774339258670807],
- [-0.326529860496521, -0.10192928463220596, 0.2961374819278717, 0.580808699131012],
- [0.46179524064064026, -0.4794206917285919, 0.47078272700309753, -0.5654175877571106],
- [-0.501025915145874, -0.38049301505088806, 0.3792027235031128, 0.685397207736969]
- ]
- >
- },
- "dense_2" => %{
- "bias" => #Nx.Tensor<
- f32[1]
- [-0.4034360647201538]
- >,
- "kernel" => #Nx.Tensor<
- f32[4][1]
- [
- [0.8062413334846497],
- [0.6867087483406067],
- [0.5137255787849426],
- [-0.5783006548881531]
- ]
- >
- }
-}
You can also use event handlers for things as simple as implementing custom logging with the pre-packaged Axon.Loop.log/4
event handler:
model
-|> Axon.Loop.trainer(:mean_squared_error, :sgd)
-|> Axon.Loop.log(:epoch_completed, fn _state -> "epoch is over\n" end, :stdio)
-|> Axon.Loop.run(train_data, %{}, epochs: 5, iterations: 100)
Epoch: 0, Batch: 100, loss: 0.2134880
+Epoch: 4, Batch: 100, loss: 0.1008184
%{
+ "dense_0" => %{
+ "bias" => #Nx.Tensor<
+ f32[8]
+ [0.36853691935539246, 0.24528849124908447, 0.13193830847740173, 0.03188902884721756, -0.06358373910188675, 0.044517479836940765, -0.1203451156616211, -6.352089694701135e-4]
+ >,
+ "kernel" => #Nx.Tensor<
+ f32[1][8]
+ [
+ [0.49448737502098083, 0.5250089764595032, 0.7132464051246643, 0.47473379969596863, -0.043285828083753586, -0.14137212932109833, -0.07576408237218857, -0.48898136615753174]
+ ]
+ >
+ },
+ "dense_1" => %{
+ "bias" => #Nx.Tensor<
+ f32[4]
+ [0.30324652791023254, 0.0385407879948616, -0.16782516241073608, 0.1984063982963562]
+ >,
+ "kernel" => #Nx.Tensor<
+ f32[8][4]
+ [
+ [0.2536502778530121, 0.375381737947464, 0.7119463086128235, -0.14521682262420654],
+ [0.20504063367843628, -0.11605211347341537, 0.49423739314079285, -0.03246872499585152],
+ [-0.13834621012210846, -0.2579476833343506, 0.34836748242378235, -0.4670639634132385],
+ [-0.11925031989812851, -0.6655324697494507, 0.5057039856910706, 0.496115118265152],
+ [0.15856991708278656, -0.2239169478416443, 0.5550385117530823, -0.3774339258670807],
+ [-0.326529860496521, -0.10192928463220596, 0.2961374819278717, 0.580808699131012],
+ [0.46179524064064026, -0.4794206917285919, 0.47078272700309753, -0.5654175877571106],
+ [-0.501025915145874, -0.38049301505088806, 0.3792027235031128, 0.685397207736969]
+ ]
+ >
+ },
+ "dense_2" => %{
+ "bias" => #Nx.Tensor<
+ f32[1]
+ [-0.4034360647201538]
+ >,
+ "kernel" => #Nx.Tensor<
+ f32[4][1]
+ [
+ [0.8062413334846497],
+ [0.6867087483406067],
+ [0.5137255787849426],
+ [-0.5783006548881531]
+ ]
+ >
+ }
+}
You can also use event handlers for things as simple as implementing custom logging with the pre-packaged Axon.Loop.log/4
event handler:
model
+|> Axon.Loop.trainer(:mean_squared_error, :sgd)
+|> Axon.Loop.log(:epoch_completed, fn _state -> "epoch is over\n" end, :stdio)
+|> Axon.Loop.run(train_data, %{}, epochs: 5, iterations: 100)
Epoch: 0, Batch: 100, loss: 0.2134880
epoch is over
Epoch: 1, Batch: 100, loss: 0.1604774
epoch is over
@@ -238,108 +238,108 @@
Epoch: 3, Batch: 100, loss: 0.1087099
epoch is over
Epoch: 4, Batch: 100, loss: 0.0940388
-epoch is over
%{
- "dense_0" => %{
- "bias" => #Nx.Tensor<
- f32[8]
- [0.1741544008255005, -0.013307991437613964, 0.0873112753033638, -0.04722493514418602, -0.12966567277908325, 0.04596322402358055, 0.3969370722770691, -0.04508184269070625]
- >,
- "kernel" => #Nx.Tensor<
- f32[1][8]
- [
- [0.31960299611091614, -0.5328841805458069, -0.24278149008750916, -0.47772416472435, 0.21538947522640228, -0.2799384295940399, 0.5947694778442383, 0.0497460775077343]
- ]
- >
- },
- "dense_1" => %{
- "bias" => #Nx.Tensor<
- f32[4]
- [0.25857725739479065, -0.07283111661672592, -0.10656370222568512, -0.08234459906816483]
- >,
- "kernel" => #Nx.Tensor<
- f32[8][4]
- [
- [0.3983175754547119, -0.5524351596832275, 0.36650899052619934, -0.23933114111423492],
- [0.06517457216978073, 0.2564122974872589, 0.6227137446403503, -0.5661884546279907],
- [-0.7012182474136353, 0.054501600563526154, -0.6726318597793579, 0.4774037301540375],
- [-0.11393500864505768, 0.1726256012916565, -0.6723376512527466, 0.6044175028800964],
- [-0.30502673983573914, 0.7011693120002747, 0.40034061670303345, -0.5748327374458313],
- [-0.07724377512931824, -0.251364529132843, -0.6626797914505005, -0.20940908789634705],
- [0.7290927767753601, 0.08563250303268433, -0.047927819192409515, -0.04336162284016609],
- [-0.34993213415145874, 0.281339168548584, -0.49343380331993103, -0.2481663078069687]
- ]
- >
- },
- "dense_2" => %{
- "bias" => #Nx.Tensor<
- f32[1]
- [-0.6856028437614441]
- >,
- "kernel" => #Nx.Tensor<
- f32[4][1]
- [
- [1.1966136693954468],
- [-0.00546963419765234],
- [-0.9349364042282104],
- [0.9214714765548706]
- ]
- >
- }
-}
For even more fine-grained control over when event handlers fire, you can add filters. For example, if you only want to checkpoint loop state every 2 epochs, you can use a filter:
model
-|> Axon.Loop.trainer(:mean_squared_error, :sgd)
-|> Axon.Loop.checkpoint(event: :epoch_completed, filter: [every: 2])
-|> Axon.Loop.run(train_data, %{}, epochs: 5, iterations: 100)
Epoch: 0, Batch: 100, loss: 0.1791917
+epoch is over
%{
+ "dense_0" => %{
+ "bias" => #Nx.Tensor<
+ f32[8]
+ [0.1741544008255005, -0.013307991437613964, 0.0873112753033638, -0.04722493514418602, -0.12966567277908325, 0.04596322402358055, 0.3969370722770691, -0.04508184269070625]
+ >,
+ "kernel" => #Nx.Tensor<
+ f32[1][8]
+ [
+ [0.31960299611091614, -0.5328841805458069, -0.24278149008750916, -0.47772416472435, 0.21538947522640228, -0.2799384295940399, 0.5947694778442383, 0.0497460775077343]
+ ]
+ >
+ },
+ "dense_1" => %{
+ "bias" => #Nx.Tensor<
+ f32[4]
+ [0.25857725739479065, -0.07283111661672592, -0.10656370222568512, -0.08234459906816483]
+ >,
+ "kernel" => #Nx.Tensor<
+ f32[8][4]
+ [
+ [0.3983175754547119, -0.5524351596832275, 0.36650899052619934, -0.23933114111423492],
+ [0.06517457216978073, 0.2564122974872589, 0.6227137446403503, -0.5661884546279907],
+ [-0.7012182474136353, 0.054501600563526154, -0.6726318597793579, 0.4774037301540375],
+ [-0.11393500864505768, 0.1726256012916565, -0.6723376512527466, 0.6044175028800964],
+ [-0.30502673983573914, 0.7011693120002747, 0.40034061670303345, -0.5748327374458313],
+ [-0.07724377512931824, -0.251364529132843, -0.6626797914505005, -0.20940908789634705],
+ [0.7290927767753601, 0.08563250303268433, -0.047927819192409515, -0.04336162284016609],
+ [-0.34993213415145874, 0.281339168548584, -0.49343380331993103, -0.2481663078069687]
+ ]
+ >
+ },
+ "dense_2" => %{
+ "bias" => #Nx.Tensor<
+ f32[1]
+ [-0.6856028437614441]
+ >,
+ "kernel" => #Nx.Tensor<
+ f32[4][1]
+ [
+ [1.1966136693954468],
+ [-0.00546963419765234],
+ [-0.9349364042282104],
+ [0.9214714765548706]
+ ]
+ >
+ }
+}
For even more fine-grained control over when event handlers fire, you can add filters. For example, if you only want to checkpoint loop state every 2 epochs, you can use a filter:
model
+|> Axon.Loop.trainer(:mean_squared_error, :sgd)
+|> Axon.Loop.checkpoint(event: :epoch_completed, filter: [every: 2])
+|> Axon.Loop.run(train_data, %{}, epochs: 5, iterations: 100)
Epoch: 0, Batch: 100, loss: 0.1791917
Epoch: 1, Batch: 100, loss: 0.1373887
Epoch: 2, Batch: 100, loss: 0.1156979
Epoch: 3, Batch: 100, loss: 0.0965481
-Epoch: 4, Batch: 100, loss: 0.0865761
%{
- "dense_0" => %{
- "bias" => #Nx.Tensor<
- f32[8]
- [0.00938357226550579, 0.16315333545207977, 0.2767408788204193, -0.22733710706233978, 0.2830233573913574, -0.10280115902423859, -0.07500249892473221, 0.2947545647621155]
- >,
- "kernel" => #Nx.Tensor<
- f32[1][8]
- [
- [0.522411048412323, 0.15686289966106415, 0.30727216601371765, 0.3295647203922272, 0.38795727491378784, 0.17159366607666016, 0.7608513236045837, 0.4526905119419098]
- ]
- >
- },
- "dense_1" => %{
- "bias" => #Nx.Tensor<
- f32[4]
- [-0.024011338129639626, 0.0, -0.00135718728415668, -0.0015321056125685573]
- >,
- "kernel" => #Nx.Tensor<
- f32[8][4]
- [
- [0.606391966342926, -0.08385708928108215, 0.06838012486696243, -0.08704598248004913],
- [0.5944894552230835, -0.17639528214931488, 0.26653605699539185, 0.35148826241493225],
- [-0.06138936057686806, -0.024123376235365868, 0.29706713557243347, 0.5498997569084167],
- [0.26888611912727356, 0.024979088455438614, -0.653775155544281, -0.4111217260360718],
- [-0.5042538046836853, -0.6867390871047974, 0.13647332787513733, 0.7193269729614258],
- [-0.052732646465301514, 0.099549300968647, -0.6970457434654236, 0.3078557252883911],
- [-0.261769562959671, 0.17121906578540802, -0.08267408609390259, -0.2213396430015564],
- [-0.09766292572021484, -0.5843542218208313, 0.369784414768219, 0.48434120416641235]
- ]
- >
- },
- "dense_2" => %{
- "bias" => #Nx.Tensor<
- f32[1]
- [-0.6914201378822327]
- >,
- "kernel" => #Nx.Tensor<
- f32[4][1]
- [
- [0.96906977891922],
- [-0.5032458901405334],
- [0.9275273680686951],
- [0.8574270606040955]
- ]
- >
- }
-}
Axon event handlers support both keyword and function filters. Keyword filters include keywords such as :every
, :once
, and :always
. Function filters are arity-1 functions which accept the current loop state and return a boolean.
+Epoch: 4, Batch: 100, loss: 0.0865761
%{
+ "dense_0" => %{
+ "bias" => #Nx.Tensor<
+ f32[8]
+ [0.00938357226550579, 0.16315333545207977, 0.2767408788204193, -0.22733710706233978, 0.2830233573913574, -0.10280115902423859, -0.07500249892473221, 0.2947545647621155]
+ >,
+ "kernel" => #Nx.Tensor<
+ f32[1][8]
+ [
+ [0.522411048412323, 0.15686289966106415, 0.30727216601371765, 0.3295647203922272, 0.38795727491378784, 0.17159366607666016, 0.7608513236045837, 0.4526905119419098]
+ ]
+ >
+ },
+ "dense_1" => %{
+ "bias" => #Nx.Tensor<
+ f32[4]
+ [-0.024011338129639626, 0.0, -0.00135718728415668, -0.0015321056125685573]
+ >,
+ "kernel" => #Nx.Tensor<
+ f32[8][4]
+ [
+ [0.606391966342926, -0.08385708928108215, 0.06838012486696243, -0.08704598248004913],
+ [0.5944894552230835, -0.17639528214931488, 0.26653605699539185, 0.35148826241493225],
+ [-0.06138936057686806, -0.024123376235365868, 0.29706713557243347, 0.5498997569084167],
+ [0.26888611912727356, 0.024979088455438614, -0.653775155544281, -0.4111217260360718],
+ [-0.5042538046836853, -0.6867390871047974, 0.13647332787513733, 0.7193269729614258],
+ [-0.052732646465301514, 0.099549300968647, -0.6970457434654236, 0.3078557252883911],
+ [-0.261769562959671, 0.17121906578540802, -0.08267408609390259, -0.2213396430015564],
+ [-0.09766292572021484, -0.5843542218208313, 0.369784414768219, 0.48434120416641235]
+ ]
+ >
+ },
+ "dense_2" => %{
+ "bias" => #Nx.Tensor<
+ f32[1]
+ [-0.6914201378822327]
+ >,
+ "kernel" => #Nx.Tensor<
+ f32[4][1]
+ [
+ [0.96906977891922],
+ [-0.5032458901405334],
+ [0.9275273680686951],
+ [0.8574270606040955]
+ ]
+ >
+ }
+}
Axon event handlers support both keyword and function filters. Keyword filters include keywords such as :every
, :once
, and :always
. Function filters are arity-1 functions which accept the current loop state and return a boolean.
diff --git a/writing_custom_event_handlers.html b/writing_custom_event_handlers.html
index ec7a0548..677dd9ac 100644
--- a/writing_custom_event_handlers.html
+++ b/writing_custom_event_handlers.html
@@ -115,65 +115,65 @@
-Mix.install([
- {:axon, github: "elixir-nx/axon"},
- {:nx, "~> 0.3.0", github: "elixir-nx/nx", sparse: "nx", override: true}
-])
:ok
+Mix.install([
+ {:axon, github: "elixir-nx/axon"},
+ {:nx, "~> 0.3.0", github: "elixir-nx/nx", sparse: "nx", override: true}
+])
:ok
writing-custom-event-handlers
Writing custom event handlers
-
If you require functionality not offered by any of Axon's built-in event handlers, then you'll need to write a custom event handler. Custom event handlers are functions which accept loop state, perform some action, and then defer execution back to the main loop. For example, you can write custom loop handlers which visualize model outputs, communicate with an external Kino process, or simply halt the loop based on some criteria.
All event handlers must accept an %Axon.Loop.State{}
struct and return a tuple of {control_term, state}
where control_term
is one of :continue
, :halt_epoch
, or :halt_loop
and state
is the updated loop state:
defmodule CustomEventHandler do
+If you require functionality not offered by any of Axon's built-in event handlers, then you'll need to write a custom event handler. Custom event handlers are functions which accept loop state, perform some action, and then defer execution back to the main loop. For example, you can write custom loop handlers which visualize model outputs, communicate with an external Kino process, or simply halt the loop based on some criteria.
All event handlers must accept an %Axon.Loop.State{}
struct and return a tuple of {control_term, state}
where control_term
is one of :continue
, :halt_epoch
, or :halt_loop
and state
is the updated loop state:
defmodule CustomEventHandler do
alias Axon.Loop.State
- def my_weird_handler(%State{} = state) do
- IO.puts("My weird handler: fired")
- {:continue, state}
- end
-end
{:module, CustomEventHandler, <<70, 79, 82, 49, 0, 0, 6, ...>>, {:my_weird_handler, 1}}
To register event handlers, you use Axon.Loop.handle/4
:
model =
- Axon.input("data")
- |> Axon.dense(8)
- |> Axon.relu()
- |> Axon.dense(4)
- |> Axon.relu()
- |> Axon.dense(1)
+ def my_weird_handler(%State{} = state) do
+ IO.puts("My weird handler: fired")
+ {:continue, state}
+ end
+end
{:module, CustomEventHandler, <<70, 79, 82, 49, 0, 0, 6, ...>>, {:my_weird_handler, 1}}
To register event handlers, you use Axon.Loop.handle/4
:
model =
+ Axon.input("data")
+ |> Axon.dense(8)
+ |> Axon.relu()
+ |> Axon.dense(4)
+ |> Axon.relu()
+ |> Axon.dense(1)
loop =
model
- |> Axon.Loop.trainer(:mean_squared_error, :sgd)
- |> Axon.Loop.handle_event(:epoch_completed, &CustomEventHandler.my_weird_handler/1)
#Axon.Loop<
- handlers: %{
- completed: [],
- epoch_completed: [
- {&CustomEventHandler.my_weird_handler/1,
- #Function<5.33119226/1 in Axon.Loop.build_filter_fn/1>},
- {#Function<23.33119226/1 in Axon.Loop.log/5>,
- #Function<5.33119226/1 in Axon.Loop.build_filter_fn/1>}
- ],
- epoch_halted: [],
- epoch_started: [],
- halted: [],
- iteration_completed: [
- {#Function<23.33119226/1 in Axon.Loop.log/5>,
- #Function<3.33119226/1 in Axon.Loop.build_filter_fn/1>}
- ],
- iteration_started: [],
- started: []
- },
- metrics: %{
- "loss" => {#Function<12.46375131/3 in Axon.Metrics.running_average/1>,
- #Function<6.33119226/2 in Axon.Loop.build_loss_fn/1>}
- },
+ |> Axon.Loop.trainer(:mean_squared_error, :sgd)
+ |> Axon.Loop.handle_event(:epoch_completed, &CustomEventHandler.my_weird_handler/1)
#Axon.Loop<
+ handlers: %{
+ completed: [],
+ epoch_completed: [
+ {&CustomEventHandler.my_weird_handler/1,
+ #Function<5.33119226/1 in Axon.Loop.build_filter_fn/1>},
+ {#Function<23.33119226/1 in Axon.Loop.log/5>,
+ #Function<5.33119226/1 in Axon.Loop.build_filter_fn/1>}
+ ],
+ epoch_halted: [],
+ epoch_started: [],
+ halted: [],
+ iteration_completed: [
+ {#Function<23.33119226/1 in Axon.Loop.log/5>,
+ #Function<3.33119226/1 in Axon.Loop.build_filter_fn/1>}
+ ],
+ iteration_started: [],
+ started: []
+ },
+ metrics: %{
+ "loss" => {#Function<12.46375131/3 in Axon.Metrics.running_average/1>,
+ #Function<6.33119226/2 in Axon.Loop.build_loss_fn/1>}
+ },
...
->
Axon will trigger your custom handler to run on the attached event:
train_data =
- Stream.repeatedly(fn ->
- xs = Nx.random_normal({8, 1})
- ys = Nx.sin(xs)
- {xs, ys}
- end)
-
-Axon.Loop.run(loop, train_data, %{}, epochs: 5, iterations: 100)
Epoch: 0, Batch: 100, loss: 0.1905403
+>
Axon will trigger your custom handler to run on the attached event:
train_data =
+ Stream.repeatedly(fn ->
+ xs = Nx.random_normal({8, 1})
+ ys = Nx.sin(xs)
+ {xs, ys}
+ end)
+
+Axon.Loop.run(loop, train_data, %{}, epochs: 5, iterations: 100)
Epoch: 0, Batch: 100, loss: 0.1905403
My weird handler: fired
Epoch: 1, Batch: 100, loss: 0.1478554
My weird handler: fired
@@ -182,128 +182,128 @@
Epoch: 3, Batch: 100, loss: 0.0983292
My weird handler: fired
Epoch: 4, Batch: 100, loss: 0.0845697
-My weird handler: fired
%{
- "dense_0" => %{
- "bias" => #Nx.Tensor<
- f32[8]
- [0.014659373089671135, 0.08941870182752609, -0.09661660343408585, 0.2650177478790283, -0.06400775164365768, -0.07953602075576782, 0.22094617784023285, -0.014790073968470097]
- >,
- "kernel" => #Nx.Tensor<
- f32[1][8]
- [
- [0.3581556975841522, 0.38828182220458984, -0.3311854302883148, -0.4059808552265167, 0.6334917545318604, 0.17008493840694427, -0.5630434155464172, 0.3790667653083801]
- ]
- >
- },
- "dense_1" => %{
- "bias" => #Nx.Tensor<
- f32[4]
- [0.3047839403152466, -0.025677276775240898, 0.18113580346107483, 0.19019420444965363]
- >,
- "kernel" => #Nx.Tensor<
- f32[8][4]
- [
- [-0.25477269291877747, 0.28833284974098206, -0.25498083233833313, 0.40912926197052],
- [-0.387851357460022, 0.009837300516664982, -0.48930269479751587, -0.6119663715362549],
- [0.49769237637519836, -0.45746952295303345, -0.3886529505252838, -0.49895355105400085],
- [0.6451961994171143, 0.16054697334766388, 0.27802371978759766, -0.15226426720619202],
- [0.17125651240348816, -0.048851024359464645, 0.19429178535938263, 0.24933232367038727],
- [0.5465306043624878, -0.15836869180202484, 0.39782997965812683, -0.3635501563549042],
- [-0.36660289764404297, -0.011948992498219013, 0.48680511116981506, 0.5263928174972534],
- [-0.6284276843070984, -0.5880372524261475, 0.004470183979719877, -0.4550755023956299]
- ]
- >
- },
- "dense_2" => %{
- "bias" => #Nx.Tensor<
- f32[1]
- [0.7117368578910828]
- >,
- "kernel" => #Nx.Tensor<
- f32[4][1]
- [
- [-0.7743457555770874],
- [0.3977936804294586],
- [-1.0638943910598755],
- [-0.6494196653366089]
- ]
- >
- }
-}
You can use event handlers to early-stop a loop or loop epoch by returning a :halt_*
control term. Halt control terms can be one of :halt_epoch
or :halt_loop
. :halt_epoch
halts the current epoch and continues to the next. :halt_loop
halts the loop altogether.
defmodule CustomEventHandler do
+My weird handler: fired
%{
+ "dense_0" => %{
+ "bias" => #Nx.Tensor<
+ f32[8]
+ [0.014659373089671135, 0.08941870182752609, -0.09661660343408585, 0.2650177478790283, -0.06400775164365768, -0.07953602075576782, 0.22094617784023285, -0.014790073968470097]
+ >,
+ "kernel" => #Nx.Tensor<
+ f32[1][8]
+ [
+ [0.3581556975841522, 0.38828182220458984, -0.3311854302883148, -0.4059808552265167, 0.6334917545318604, 0.17008493840694427, -0.5630434155464172, 0.3790667653083801]
+ ]
+ >
+ },
+ "dense_1" => %{
+ "bias" => #Nx.Tensor<
+ f32[4]
+ [0.3047839403152466, -0.025677276775240898, 0.18113580346107483, 0.19019420444965363]
+ >,
+ "kernel" => #Nx.Tensor<
+ f32[8][4]
+ [
+ [-0.25477269291877747, 0.28833284974098206, -0.25498083233833313, 0.40912926197052],
+ [-0.387851357460022, 0.009837300516664982, -0.48930269479751587, -0.6119663715362549],
+ [0.49769237637519836, -0.45746952295303345, -0.3886529505252838, -0.49895355105400085],
+ [0.6451961994171143, 0.16054697334766388, 0.27802371978759766, -0.15226426720619202],
+ [0.17125651240348816, -0.048851024359464645, 0.19429178535938263, 0.24933232367038727],
+ [0.5465306043624878, -0.15836869180202484, 0.39782997965812683, -0.3635501563549042],
+ [-0.36660289764404297, -0.011948992498219013, 0.48680511116981506, 0.5263928174972534],
+ [-0.6284276843070984, -0.5880372524261475, 0.004470183979719877, -0.4550755023956299]
+ ]
+ >
+ },
+ "dense_2" => %{
+ "bias" => #Nx.Tensor<
+ f32[1]
+ [0.7117368578910828]
+ >,
+ "kernel" => #Nx.Tensor<
+ f32[4][1]
+ [
+ [-0.7743457555770874],
+ [0.3977936804294586],
+ [-1.0638943910598755],
+ [-0.6494196653366089]
+ ]
+ >
+ }
+}
You can use event handlers to early-stop a loop or loop epoch by returning a :halt_*
control term. Halt control terms can be one of :halt_epoch
or :halt_loop
. :halt_epoch
halts the current epoch and continues to the next. :halt_loop
halts the loop altogether.
defmodule CustomEventHandler do
alias Axon.Loop.State
- def always_halts(%State{} = state) do
- IO.puts("stopping loop")
- {:halt_loop, state}
- end
-end
{:module, CustomEventHandler, <<70, 79, 82, 49, 0, 0, 6, ...>>, {:always_halts, 1}}
The loop will immediately stop executing and return the current state at the time it was halted:
model
-|> Axon.Loop.trainer(:mean_squared_error, :sgd)
-|> Axon.Loop.handle_event(:epoch_completed, &CustomEventHandler.always_halts/1)
-|> Axon.Loop.run(train_data, %{}, epochs: 5, iterations: 100)
Epoch: 0, Batch: 100, loss: 0.1967763
-stopping loop
%{
- "dense_0" => %{
- "bias" => #Nx.Tensor<
- f32[8]
- [-0.05958094820380211, 0.08930676430463791, -0.006259916350245476, 0.05067025125026703, 0.10981185734272003, -0.011248357594013214, -0.007601946126669645, 0.036958880722522736]
- >,
- "kernel" => #Nx.Tensor<
- f32[1][8]
- [
- [0.050393108278512955, -0.5486620664596558, 0.6901980042457581, 0.42280837893486023, 0.6446300745010376, 0.25207778811454773, -0.13566234707832336, 0.26625606417655945]
- ]
- >
- },
- "dense_1" => %{
- "bias" => #Nx.Tensor<
- f32[4]
- [-0.06729397922754288, 0.14259757101535797, -0.0020351663697510958, 0.16679106652736664]
- >,
- "kernel" => #Nx.Tensor<
- f32[8][4]
- [
- [-0.5964004397392273, -0.5631846785545349, 0.15613533556461334, 0.1943722516298294],
- [0.19513694941997528, -0.24765732884407043, -0.06751974672079086, 0.6707308292388916],
- [-0.6826592087745667, -0.006577506195753813, -0.6097249984741211, -0.5801466703414917],
- [-0.30076032876968384, 0.34819719195365906, -0.5906499028205872, -0.37741175293922424],
- [0.16266342997550964, 0.7666646838188171, 0.6456886529922485, -0.4589986801147461],
- [-0.2686948776245117, -0.06113003194332123, 0.22663049399852753, -0.12092678993940353],
- [-0.5785921216011047, -0.641874372959137, -0.24317769706249237, -0.2897084951400757],
- [0.14917287230491638, 0.24462535977363586, -0.64858478307724, -0.5138146877288818]
- ]
- >
- },
- "dense_2" => %{
- "bias" => #Nx.Tensor<
- f32[1]
- [-0.11649220436811447]
- >,
- "kernel" => #Nx.Tensor<
- f32[4][1]
- [
- [0.7849427461624146],
- [0.5966104865074158],
- [-0.5520159602165222],
- [-0.4974740147590637]
- ]
- >
- }
-}
Note that halting an epoch will fire a different event than completing an epoch. So if you implement a custom handler to halt the loop when an epoch completes, it will never fire if the epoch always halts prematurely:
defmodule CustomEventHandler do
+ def always_halts(%State{} = state) do
+ IO.puts("stopping loop")
+ {:halt_loop, state}
+ end
+end
{:module, CustomEventHandler, <<70, 79, 82, 49, 0, 0, 6, ...>>, {:always_halts, 1}}
The loop will immediately stop executing and return the current state at the time it was halted:
model
+|> Axon.Loop.trainer(:mean_squared_error, :sgd)
+|> Axon.Loop.handle_event(:epoch_completed, &CustomEventHandler.always_halts/1)
+|> Axon.Loop.run(train_data, %{}, epochs: 5, iterations: 100)
Epoch: 0, Batch: 100, loss: 0.1967763
+stopping loop
%{
+ "dense_0" => %{
+ "bias" => #Nx.Tensor<
+ f32[8]
+ [-0.05958094820380211, 0.08930676430463791, -0.006259916350245476, 0.05067025125026703, 0.10981185734272003, -0.011248357594013214, -0.007601946126669645, 0.036958880722522736]
+ >,
+ "kernel" => #Nx.Tensor<
+ f32[1][8]
+ [
+ [0.050393108278512955, -0.5486620664596558, 0.6901980042457581, 0.42280837893486023, 0.6446300745010376, 0.25207778811454773, -0.13566234707832336, 0.26625606417655945]
+ ]
+ >
+ },
+ "dense_1" => %{
+ "bias" => #Nx.Tensor<
+ f32[4]
+ [-0.06729397922754288, 0.14259757101535797, -0.0020351663697510958, 0.16679106652736664]
+ >,
+ "kernel" => #Nx.Tensor<
+ f32[8][4]
+ [
+ [-0.5964004397392273, -0.5631846785545349, 0.15613533556461334, 0.1943722516298294],
+ [0.19513694941997528, -0.24765732884407043, -0.06751974672079086, 0.6707308292388916],
+ [-0.6826592087745667, -0.006577506195753813, -0.6097249984741211, -0.5801466703414917],
+ [-0.30076032876968384, 0.34819719195365906, -0.5906499028205872, -0.37741175293922424],
+ [0.16266342997550964, 0.7666646838188171, 0.6456886529922485, -0.4589986801147461],
+ [-0.2686948776245117, -0.06113003194332123, 0.22663049399852753, -0.12092678993940353],
+ [-0.5785921216011047, -0.641874372959137, -0.24317769706249237, -0.2897084951400757],
+ [0.14917287230491638, 0.24462535977363586, -0.64858478307724, -0.5138146877288818]
+ ]
+ >
+ },
+ "dense_2" => %{
+ "bias" => #Nx.Tensor<
+ f32[1]
+ [-0.11649220436811447]
+ >,
+ "kernel" => #Nx.Tensor<
+ f32[4][1]
+ [
+ [0.7849427461624146],
+ [0.5966104865074158],
+ [-0.5520159602165222],
+ [-0.4974740147590637]
+ ]
+ >
+ }
+}
Note that halting an epoch will fire a different event than completing an epoch. So if you implement a custom handler to halt the loop when an epoch completes, it will never fire if the epoch always halts prematurely:
defmodule CustomEventHandler do
alias Axon.Loop.State
- def always_halts_epoch(%State{} = state) do
- IO.puts("\nstopping epoch")
- {:halt_epoch, state}
- end
-
- def always_halts_loop(%State{} = state) do
- IO.puts("stopping loop\n")
- {:halt_loop, state}
- end
-end
{:module, CustomEventHandler, <<70, 79, 82, 49, 0, 0, 7, ...>>, {:always_halts_loop, 1}}
If you run these handlers in conjunction, the loop will not terminate prematurely:
model
-|> Axon.Loop.trainer(:mean_squared_error, :sgd)
-|> Axon.Loop.handle_event(:iteration_completed, &CustomEventHandler.always_halts_epoch/1)
-|> Axon.Loop.handle_event(:epoch_completed, &CustomEventHandler.always_halts_loop/1)
-|> Axon.Loop.run(train_data, %{}, epochs: 5, iterations: 100)
Epoch: 0, Batch: 0, loss: 0.0000000
+ def always_halts_epoch(%State{} = state) do
+ IO.puts("\nstopping epoch")
+ {:halt_epoch, state}
+ end
+
+ def always_halts_loop(%State{} = state) do
+ IO.puts("stopping loop\n")
+ {:halt_loop, state}
+ end
+end
{:module, CustomEventHandler, <<70, 79, 82, 49, 0, 0, 7, ...>>, {:always_halts_loop, 1}}
If you run these handlers in conjunction, the loop will not terminate prematurely:
model
+|> Axon.Loop.trainer(:mean_squared_error, :sgd)
+|> Axon.Loop.handle_event(:iteration_completed, &CustomEventHandler.always_halts_epoch/1)
+|> Axon.Loop.handle_event(:epoch_completed, &CustomEventHandler.always_halts_loop/1)
+|> Axon.Loop.run(train_data, %{}, epochs: 5, iterations: 100)
Epoch: 0, Batch: 0, loss: 0.0000000
stopping epoch
Epoch: 0, Batch: 0, loss: 0.7256396
stopping epoch
@@ -312,54 +312,54 @@
Epoch: 0, Batch: 0, loss: 0.4981923
stopping epoch
Epoch: 0, Batch: 0, loss: 0.4377063
-stopping epoch
%{
- "dense_0" => %{
- "bias" => #Nx.Tensor<
- f32[8]
- [9.248655405826867e-4, -0.0038722341414541006, -0.0015197680331766605, -0.001993122510612011, -0.0015419051051139832, -0.004070846363902092, 0.001461982261389494, 0.0043989671394228935]
- >,
- "kernel" => #Nx.Tensor<
- f32[1][8]
- [
- [-0.6537156701087952, 0.2857331335544586, -0.339731365442276, 0.46841081976890564, -0.5864744782447815, -0.364472359418869, -0.5385616421699524, -0.694677472114563]
- ]
- >
- },
- "dense_1" => %{
- "bias" => #Nx.Tensor<
- f32[4]
- [0.0, -0.017093738541007042, 0.00152371556032449, -0.0019599769730120897]
- >,
- "kernel" => #Nx.Tensor<
- f32[8][4]
- [
- [-0.21336764097213745, -0.6211493611335754, 0.676548957824707, 0.3768426477909088],
- [-0.24921125173568726, 0.217195525765419, 0.23704318702220917, 0.1597728431224823],
- [-0.12178827077150345, -0.4966273307800293, -0.283501535654068, 0.00888047181069851],
- [-0.19504092633724213, 0.18697738647460938, 0.14705461263656616, 0.39286476373672485],
- [-0.5945789813995361, -0.5958647727966309, -0.3320448100566864, -0.02747068926692009],
- [-0.2157520055770874, -0.2990635335445404, -0.16008871793746948, 0.4921063184738159],
- [-0.529068648815155, -0.383655846118927, -0.07292155921459198, -0.2834954559803009],
- [-0.3056498169898987, -0.28507867455482483, 0.554026186466217, -0.24665579199790955]
- ]
- >
- },
- "dense_2" => %{
- "bias" => #Nx.Tensor<
- f32[1]
- [-0.010511377826333046]
- >,
- "kernel" => #Nx.Tensor<
- f32[4][1]
- [
- [0.9865502119064331],
- [-0.686279296875],
- [-0.15436960756778717],
- [0.18355509638786316]
- ]
- >
- }
-}
You may access and update any portion of the loop state. Keep in mind that event handlers are not JIT-compiled, so you should be certain to manually JIT-compile any long-running or expensive operations.
+stopping epoch
%{
+ "dense_0" => %{
+ "bias" => #Nx.Tensor<
+ f32[8]
+ [9.248655405826867e-4, -0.0038722341414541006, -0.0015197680331766605, -0.001993122510612011, -0.0015419051051139832, -0.004070846363902092, 0.001461982261389494, 0.0043989671394228935]
+ >,
+ "kernel" => #Nx.Tensor<
+ f32[1][8]
+ [
+ [-0.6537156701087952, 0.2857331335544586, -0.339731365442276, 0.46841081976890564, -0.5864744782447815, -0.364472359418869, -0.5385616421699524, -0.694677472114563]
+ ]
+ >
+ },
+ "dense_1" => %{
+ "bias" => #Nx.Tensor<
+ f32[4]
+ [0.0, -0.017093738541007042, 0.00152371556032449, -0.0019599769730120897]
+ >,
+ "kernel" => #Nx.Tensor<
+ f32[8][4]
+ [
+ [-0.21336764097213745, -0.6211493611335754, 0.676548957824707, 0.3768426477909088],
+ [-0.24921125173568726, 0.217195525765419, 0.23704318702220917, 0.1597728431224823],
+ [-0.12178827077150345, -0.4966273307800293, -0.283501535654068, 0.00888047181069851],
+ [-0.19504092633724213, 0.18697738647460938, 0.14705461263656616, 0.39286476373672485],
+ [-0.5945789813995361, -0.5958647727966309, -0.3320448100566864, -0.02747068926692009],
+ [-0.2157520055770874, -0.2990635335445404, -0.16008871793746948, 0.4921063184738159],
+ [-0.529068648815155, -0.383655846118927, -0.07292155921459198, -0.2834954559803009],
+ [-0.3056498169898987, -0.28507867455482483, 0.554026186466217, -0.24665579199790955]
+ ]
+ >
+ },
+ "dense_2" => %{
+ "bias" => #Nx.Tensor<
+ f32[1]
+ [-0.010511377826333046]
+ >,
+ "kernel" => #Nx.Tensor<
+ f32[4][1]
+ [
+ [0.9865502119064331],
+ [-0.686279296875],
+ [-0.15436960756778717],
+ [0.18355509638786316]
+ ]
+ >
+ }
+}
You may access and update any portion of the loop state. Keep in mind that event handlers are not JIT-compiled, so you should be certain to manually JIT-compile any long-running or expensive operations.
diff --git a/writing_custom_metrics.html b/writing_custom_metrics.html
index 34d2ec6d..90439e61 100644
--- a/writing_custom_metrics.html
+++ b/writing_custom_metrics.html
@@ -115,312 +115,312 @@
-Mix.install([
- {:axon, github: "elixir-nx/axon"},
- {:nx, "~> 0.3.0", github: "elixir-nx/nx", sparse: "nx", override: true}
-])
:ok
+Mix.install([
+ {:axon, github: "elixir-nx/axon"},
+ {:nx, "~> 0.3.0", github: "elixir-nx/nx", sparse: "nx", override: true}
+])
:ok
writing-custom-metrics
Writing custom metrics
-
When passing an atom to Axon.Loop.metric/5
, Axon dispatches the function to a built-in function in Axon.Metrics
. If you find you'd like to use a metric that does not exist in Axon.Metrics
, you can define a custom function:
defmodule CustomMetric do
+When passing an atom to Axon.Loop.metric/5
, Axon dispatches the function to a built-in function in Axon.Metrics
. If you find you'd like to use a metric that does not exist in Axon.Metrics
, you can define a custom function:
defmodule CustomMetric do
import Nx.Defn
- defn my_weird_metric(y_true, y_pred) do
- Nx.atan2(y_true, y_pred) |> Nx.sum()
- end
-end
{:module, CustomMetric, <<70, 79, 82, 49, 0, 0, 8, ...>>, {:my_weird_metric, 2}}
Then you can pass that directly to Axon.Loop.metric/5
. You must provide a name for your custom metric:
model =
- Axon.input("data")
- |> Axon.dense(8)
- |> Axon.relu()
- |> Axon.dense(4)
- |> Axon.relu()
- |> Axon.dense(1)
+ defn my_weird_metric(y_true, y_pred) do
+ Nx.atan2(y_true, y_pred) |> Nx.sum()
+ end
+end
{:module, CustomMetric, <<70, 79, 82, 49, 0, 0, 8, ...>>, {:my_weird_metric, 2}}
Then you can pass that directly to Axon.Loop.metric/5
. You must provide a name for your custom metric:
model =
+ Axon.input("data")
+ |> Axon.dense(8)
+ |> Axon.relu()
+ |> Axon.dense(4)
+ |> Axon.relu()
+ |> Axon.dense(1)
loop =
model
- |> Axon.Loop.trainer(:mean_squared_error, :sgd)
- |> Axon.Loop.metric(&CustomMetric.my_weird_metric/2, "my weird metric")
#Axon.Loop<
- handlers: %{
- completed: [],
- epoch_completed: [
- {#Function<23.77614421/1 in Axon.Loop.log/5>,
- #Function<5.77614421/1 in Axon.Loop.build_filter_fn/1>}
- ],
- epoch_halted: [],
- epoch_started: [],
- halted: [],
- iteration_completed: [
- {#Function<23.77614421/1 in Axon.Loop.log/5>,
- #Function<3.77614421/1 in Axon.Loop.build_filter_fn/1>}
- ],
- iteration_started: [],
- started: []
- },
- metrics: %{
- "loss" => {#Function<12.46375131/3 in Axon.Metrics.running_average/1>,
- #Function<6.77614421/2 in Axon.Loop.build_loss_fn/1>},
- "my weird metric" => {#Function<12.46375131/3 in Axon.Metrics.running_average/1>,
- &CustomMetric.my_weird_metric/2}
- },
+ |> Axon.Loop.trainer(:mean_squared_error, :sgd)
+ |> Axon.Loop.metric(&CustomMetric.my_weird_metric/2, "my weird metric")
#Axon.Loop<
+ handlers: %{
+ completed: [],
+ epoch_completed: [
+ {#Function<23.77614421/1 in Axon.Loop.log/5>,
+ #Function<5.77614421/1 in Axon.Loop.build_filter_fn/1>}
+ ],
+ epoch_halted: [],
+ epoch_started: [],
+ halted: [],
+ iteration_completed: [
+ {#Function<23.77614421/1 in Axon.Loop.log/5>,
+ #Function<3.77614421/1 in Axon.Loop.build_filter_fn/1>}
+ ],
+ iteration_started: [],
+ started: []
+ },
+ metrics: %{
+ "loss" => {#Function<12.46375131/3 in Axon.Metrics.running_average/1>,
+ #Function<6.77614421/2 in Axon.Loop.build_loss_fn/1>},
+ "my weird metric" => {#Function<12.46375131/3 in Axon.Metrics.running_average/1>,
+ &CustomMetric.my_weird_metric/2}
+ },
...
->
Then when running, Axon will invoke your custom metric function and accumulate it with the given aggregator:
train_data =
- Stream.repeatedly(fn ->
- xs = Nx.random_normal({8, 1})
- ys = Nx.sin(xs)
- {xs, ys}
- end)
-
-Axon.Loop.run(loop, train_data, %{}, iterations: 1000)
Epoch: 0, Batch: 1000, loss: 0.0468431 my weird metric: -5.7462921
%{
- "dense_0" => %{
- "bias" => #Nx.Tensor<
- f32[8]
- [0.011475208215415478, 0.23035769164562225, 0.01538881566375494, 0.08167446404695511, 0.23642019927501678, 0.10298296064138412, 0.20279639959335327, -0.18916435539722443]
- >,
- "kernel" => #Nx.Tensor<
- f32[1][8]
- [
- [0.7426201105117798, 0.734136700630188, -0.5648708343505859, -0.5230435132980347, 0.3056533932685852, 0.3383721709251404, -0.3518844544887543, -0.19460521638393402]
- ]
- >
- },
- "dense_1" => %{
- "bias" => #Nx.Tensor<
- f32[4]
- [0.2185358852148056, 0.23043134808540344, 0.0, 0.2650437355041504]
- >,
- "kernel" => #Nx.Tensor<
- f32[8][4]
- [
- [0.19164204597473145, -0.26440876722335815, 0.060297321528196335, 0.004777891095727682],
- [0.019263261929154396, -0.6267783045768738, -0.33454063534736633, 0.33268266916275024],
- [-0.18489953875541687, 0.4653063714504242, -0.6056118607521057, -0.046012550592422485],
- [0.5975558161735535, -0.237883061170578, -0.6522921919822693, 0.019332828000187874],
- [-0.7424253225326538, 0.593705952167511, 0.2551117241382599, 0.26270362734794617],
- [0.018434584140777588, 0.15290242433547974, 0.08793036639690399, 0.1839984804391861],
- [0.6048195958137512, -0.20294713973999023, -0.694927990436554, -0.45577046275138855],
- [-0.628790020942688, 0.21741150319576263, -0.08936657756567001, 0.6170362234115601]
- ]
- >
- },
- "dense_2" => %{
- "bias" => #Nx.Tensor<
- f32[1]
- [-0.03722470998764038]
- >,
- "kernel" => #Nx.Tensor<
- f32[4][1]
- [
- [-0.7919473648071289],
- [-0.4341854751110077],
- [-0.39114490151405334],
- [0.9605273008346558]
- ]
- >
- }
-}
While the metric defaults are designed with supervised training loops in mind, they can be used for much more flexible purposes. By default, metrics look for the fields :y_true
and :y_pred
in the given loop's step state. They then apply the given metric function on those inputs. You can also define metrics which work on other fields. For example you can track the running average of a given parameter with a metric just by defining a custom output transform:
model =
- Axon.input("data")
- |> Axon.dense(8)
- |> Axon.relu()
- |> Axon.dense(4)
- |> Axon.relu()
- |> Axon.dense(1)
-
-output_transform = fn %{model_state: model_state} ->
- [model_state["dense_0"]["kernel"]]
-end
+>
Then when running, Axon will invoke your custom metric function and accumulate it with the given aggregator:
train_data =
+ Stream.repeatedly(fn ->
+ xs = Nx.random_normal({8, 1})
+ ys = Nx.sin(xs)
+ {xs, ys}
+ end)
+
+Axon.Loop.run(loop, train_data, %{}, iterations: 1000)
Epoch: 0, Batch: 1000, loss: 0.0468431 my weird metric: -5.7462921
%{
+ "dense_0" => %{
+ "bias" => #Nx.Tensor<
+ f32[8]
+ [0.011475208215415478, 0.23035769164562225, 0.01538881566375494, 0.08167446404695511, 0.23642019927501678, 0.10298296064138412, 0.20279639959335327, -0.18916435539722443]
+ >,
+ "kernel" => #Nx.Tensor<
+ f32[1][8]
+ [
+ [0.7426201105117798, 0.734136700630188, -0.5648708343505859, -0.5230435132980347, 0.3056533932685852, 0.3383721709251404, -0.3518844544887543, -0.19460521638393402]
+ ]
+ >
+ },
+ "dense_1" => %{
+ "bias" => #Nx.Tensor<
+ f32[4]
+ [0.2185358852148056, 0.23043134808540344, 0.0, 0.2650437355041504]
+ >,
+ "kernel" => #Nx.Tensor<
+ f32[8][4]
+ [
+ [0.19164204597473145, -0.26440876722335815, 0.060297321528196335, 0.004777891095727682],
+ [0.019263261929154396, -0.6267783045768738, -0.33454063534736633, 0.33268266916275024],
+ [-0.18489953875541687, 0.4653063714504242, -0.6056118607521057, -0.046012550592422485],
+ [0.5975558161735535, -0.237883061170578, -0.6522921919822693, 0.019332828000187874],
+ [-0.7424253225326538, 0.593705952167511, 0.2551117241382599, 0.26270362734794617],
+ [0.018434584140777588, 0.15290242433547974, 0.08793036639690399, 0.1839984804391861],
+ [0.6048195958137512, -0.20294713973999023, -0.694927990436554, -0.45577046275138855],
+ [-0.628790020942688, 0.21741150319576263, -0.08936657756567001, 0.6170362234115601]
+ ]
+ >
+ },
+ "dense_2" => %{
+ "bias" => #Nx.Tensor<
+ f32[1]
+ [-0.03722470998764038]
+ >,
+ "kernel" => #Nx.Tensor<
+ f32[4][1]
+ [
+ [-0.7919473648071289],
+ [-0.4341854751110077],
+ [-0.39114490151405334],
+ [0.9605273008346558]
+ ]
+ >
+ }
+}
While the metric defaults are designed with supervised training loops in mind, they can be used for much more flexible purposes. By default, metrics look for the fields :y_true
and :y_pred
in the given loop's step state. They then apply the given metric function on those inputs. You can also define metrics which work on other fields. For example you can track the running average of a given parameter with a metric just by defining a custom output transform:
model =
+ Axon.input("data")
+ |> Axon.dense(8)
+ |> Axon.relu()
+ |> Axon.dense(4)
+ |> Axon.relu()
+ |> Axon.dense(1)
+
+output_transform = fn %{model_state: model_state} ->
+ [model_state["dense_0"]["kernel"]]
+end
loop =
model
- |> Axon.Loop.trainer(:mean_squared_error, :sgd)
- |> Axon.Loop.metric(&Nx.mean/1, "dense_0_kernel_mean", :running_average, output_transform)
- |> Axon.Loop.metric(&Nx.variance/1, "dense_0_kernel_var", :running_average, output_transform)
#Axon.Loop<
- handlers: %{
- completed: [],
- epoch_completed: [
- {#Function<23.77614421/1 in Axon.Loop.log/5>,
- #Function<5.77614421/1 in Axon.Loop.build_filter_fn/1>}
- ],
- epoch_halted: [],
- epoch_started: [],
- halted: [],
- iteration_completed: [
- {#Function<23.77614421/1 in Axon.Loop.log/5>,
- #Function<3.77614421/1 in Axon.Loop.build_filter_fn/1>}
- ],
- iteration_started: [],
- started: []
- },
- metrics: %{
- "dense_0_kernel_mean" => {#Function<12.46375131/3 in Axon.Metrics.running_average/1>,
- &Nx.mean/1},
- "dense_0_kernel_var" => {#Function<12.46375131/3 in Axon.Metrics.running_average/1>,
- &Nx.variance/1},
- "loss" => {#Function<12.46375131/3 in Axon.Metrics.running_average/1>,
- #Function<6.77614421/2 in Axon.Loop.build_loss_fn/1>}
- },
+ |> Axon.Loop.trainer(:mean_squared_error, :sgd)
+ |> Axon.Loop.metric(&Nx.mean/1, "dense_0_kernel_mean", :running_average, output_transform)
+ |> Axon.Loop.metric(&Nx.variance/1, "dense_0_kernel_var", :running_average, output_transform)
#Axon.Loop<
+ handlers: %{
+ completed: [],
+ epoch_completed: [
+ {#Function<23.77614421/1 in Axon.Loop.log/5>,
+ #Function<5.77614421/1 in Axon.Loop.build_filter_fn/1>}
+ ],
+ epoch_halted: [],
+ epoch_started: [],
+ halted: [],
+ iteration_completed: [
+ {#Function<23.77614421/1 in Axon.Loop.log/5>,
+ #Function<3.77614421/1 in Axon.Loop.build_filter_fn/1>}
+ ],
+ iteration_started: [],
+ started: []
+ },
+ metrics: %{
+ "dense_0_kernel_mean" => {#Function<12.46375131/3 in Axon.Metrics.running_average/1>,
+ &Nx.mean/1},
+ "dense_0_kernel_var" => {#Function<12.46375131/3 in Axon.Metrics.running_average/1>,
+ &Nx.variance/1},
+ "loss" => {#Function<12.46375131/3 in Axon.Metrics.running_average/1>,
+ #Function<6.77614421/2 in Axon.Loop.build_loss_fn/1>}
+ },
...
->
Axon will apply your custom output transform to the loop's step state and forward the result to your custom metric function:
train_data =
- Stream.repeatedly(fn ->
- xs = Nx.random_normal({8, 1})
- ys = Nx.sin(xs)
- {xs, ys}
- end)
-
-Axon.Loop.run(loop, train_data, %{}, iterations: 1000)
Epoch: 0, Batch: 1000, dense_0_kernel_mean: 0.0807205 dense_0_kernel_var: 0.1448047 loss: 0.0626600
%{
- "dense_0" => %{
- "bias" => #Nx.Tensor<
- f32[8]
- [-0.14429236948490143, 0.3176318109035492, 0.0036036474630236626, 0.01434470433741808, 0.21225003898143768, -0.1406097412109375, 0.32469284534454346, -0.18893203139305115]
- >,
- "kernel" => #Nx.Tensor<
- f32[1][8]
- [
- [0.2918722331523895, -0.44978663325309753, -0.28219935297966003, -0.10681337863206863, 0.5192054510116577, 0.312747985124588, -0.15127503871917725, 0.5638187527656555]
- ]
- >
- },
- "dense_1" => %{
- "bias" => #Nx.Tensor<
- f32[4]
- [0.0, -0.003864143043756485, 0.5194356441497803, 0.028363214805722237]
- >,
- "kernel" => #Nx.Tensor<
- f32[8][4]
- [
- [-0.6123268008232117, 0.22753892838954926, 0.12077417969703674, 0.4875330626964569],
- [-0.5840837359428406, 0.2259720116853714, 0.4917944371700287, 0.22638437151908875],
- [-0.22699439525604248, -0.6744257807731628, -0.2907045781612396, 0.35300591588020325],
- [-0.16367988288402557, -0.5971682071685791, -0.39346548914909363, 0.5823913812637329],
- [-0.5512545704841614, -0.6812713742256165, -0.5777145624160767, -0.653957188129425],
- [-0.23620283603668213, -0.47966212034225464, -0.273225873708725, 0.3827615976333618],
- [-0.5591338276863098, -0.1730434000492096, 0.25726518034935, 0.7179149389266968],
- [0.3902169167995453, 0.6351881623268127, -0.602277398109436, 0.40137141942977905]
- ]
- >
- },
- "dense_2" => %{
- "bias" => #Nx.Tensor<
- f32[1]
- [0.824558675289154]
- >,
- "kernel" => #Nx.Tensor<
- f32[4][1]
- [
- [0.9618374109268188],
- [-0.028266794979572296],
- [-1.1059081554412842],
- [-0.7398673892021179]
- ]
- >
- }
-}
You can also define custom accumulation functions. Axon has definitions for computing running averages and running sums; however, you might find you need something like an exponential moving average:
defmodule CustomAccumulator do
+>
Axon will apply your custom output transform to the loop's step state and forward the result to your custom metric function:
train_data =
+ Stream.repeatedly(fn ->
+ xs = Nx.random_normal({8, 1})
+ ys = Nx.sin(xs)
+ {xs, ys}
+ end)
+
+Axon.Loop.run(loop, train_data, %{}, iterations: 1000)
Epoch: 0, Batch: 1000, dense_0_kernel_mean: 0.0807205 dense_0_kernel_var: 0.1448047 loss: 0.0626600
%{
+ "dense_0" => %{
+ "bias" => #Nx.Tensor<
+ f32[8]
+ [-0.14429236948490143, 0.3176318109035492, 0.0036036474630236626, 0.01434470433741808, 0.21225003898143768, -0.1406097412109375, 0.32469284534454346, -0.18893203139305115]
+ >,
+ "kernel" => #Nx.Tensor<
+ f32[1][8]
+ [
+ [0.2918722331523895, -0.44978663325309753, -0.28219935297966003, -0.10681337863206863, 0.5192054510116577, 0.312747985124588, -0.15127503871917725, 0.5638187527656555]
+ ]
+ >
+ },
+ "dense_1" => %{
+ "bias" => #Nx.Tensor<
+ f32[4]
+ [0.0, -0.003864143043756485, 0.5194356441497803, 0.028363214805722237]
+ >,
+ "kernel" => #Nx.Tensor<
+ f32[8][4]
+ [
+ [-0.6123268008232117, 0.22753892838954926, 0.12077417969703674, 0.4875330626964569],
+ [-0.5840837359428406, 0.2259720116853714, 0.4917944371700287, 0.22638437151908875],
+ [-0.22699439525604248, -0.6744257807731628, -0.2907045781612396, 0.35300591588020325],
+ [-0.16367988288402557, -0.5971682071685791, -0.39346548914909363, 0.5823913812637329],
+ [-0.5512545704841614, -0.6812713742256165, -0.5777145624160767, -0.653957188129425],
+ [-0.23620283603668213, -0.47966212034225464, -0.273225873708725, 0.3827615976333618],
+ [-0.5591338276863098, -0.1730434000492096, 0.25726518034935, 0.7179149389266968],
+ [0.3902169167995453, 0.6351881623268127, -0.602277398109436, 0.40137141942977905]
+ ]
+ >
+ },
+ "dense_2" => %{
+ "bias" => #Nx.Tensor<
+ f32[1]
+ [0.824558675289154]
+ >,
+ "kernel" => #Nx.Tensor<
+ f32[4][1]
+ [
+ [0.9618374109268188],
+ [-0.028266794979572296],
+ [-1.1059081554412842],
+ [-0.7398673892021179]
+ ]
+ >
+ }
+}
You can also define custom accumulation functions. Axon has definitions for computing running averages and running sums; however, you might find you need something like an exponential moving average:
defmodule CustomAccumulator do
import Nx.Defn
- defn running_ema(acc, obs, _i, opts \\ []) do
- opts = keyword!(opts, alpha: 0.9)
- obs * opts[:alpha] + acc * (1 - opts[:alpha])
- end
-end
{:module, CustomAccumulator, <<70, 79, 82, 49, 0, 0, 11, ...>>, {:running_ema, 4}}
Your accumulator must be an arity-3 function which accepts the current accumulated value, the current observation, and the current iteration and returns the aggregated metric. You can pass a function direct as an accumulator in your metric:
model =
- Axon.input("data")
- |> Axon.dense(8)
- |> Axon.relu()
- |> Axon.dense(4)
- |> Axon.relu()
- |> Axon.dense(1)
-
-output_transform = fn %{model_state: model_state} ->
- [model_state["dense_0"]["kernel"]]
-end
+ defn running_ema(acc, obs, _i, opts \\ []) do
+ opts = keyword!(opts, alpha: 0.9)
+ obs * opts[:alpha] + acc * (1 - opts[:alpha])
+ end
+end
{:module, CustomAccumulator, <<70, 79, 82, 49, 0, 0, 11, ...>>, {:running_ema, 4}}
Your accumulator must be an arity-3 function which accepts the current accumulated value, the current observation, and the current iteration and returns the aggregated metric. You can pass a function direct as an accumulator in your metric:
model =
+ Axon.input("data")
+ |> Axon.dense(8)
+ |> Axon.relu()
+ |> Axon.dense(4)
+ |> Axon.relu()
+ |> Axon.dense(1)
+
+output_transform = fn %{model_state: model_state} ->
+ [model_state["dense_0"]["kernel"]]
+end
loop =
model
- |> Axon.Loop.trainer(:mean_squared_error, :sgd)
- |> Axon.Loop.metric(
+ |> Axon.Loop.trainer(:mean_squared_error, :sgd)
+ |> Axon.Loop.metric(
&Nx.mean/1,
"dense_0_kernel_ema_mean",
&CustomAccumulator.running_ema/3,
output_transform
- )
#Axon.Loop<
- handlers: %{
- completed: [],
- epoch_completed: [
- {#Function<23.77614421/1 in Axon.Loop.log/5>,
- #Function<5.77614421/1 in Axon.Loop.build_filter_fn/1>}
- ],
- epoch_halted: [],
- epoch_started: [],
- halted: [],
- iteration_completed: [
- {#Function<23.77614421/1 in Axon.Loop.log/5>,
- #Function<3.77614421/1 in Axon.Loop.build_filter_fn/1>}
- ],
- iteration_started: [],
- started: []
- },
- metrics: %{
- "dense_0_kernel_ema_mean" => {#Function<12.77614421/3 in Axon.Loop.build_metric_fn/3>,
- &Nx.mean/1},
- "loss" => {#Function<12.46375131/3 in Axon.Metrics.running_average/1>,
- #Function<6.77614421/2 in Axon.Loop.build_loss_fn/1>}
- },
+ )
#Axon.Loop<
+ handlers: %{
+ completed: [],
+ epoch_completed: [
+ {#Function<23.77614421/1 in Axon.Loop.log/5>,
+ #Function<5.77614421/1 in Axon.Loop.build_filter_fn/1>}
+ ],
+ epoch_halted: [],
+ epoch_started: [],
+ halted: [],
+ iteration_completed: [
+ {#Function<23.77614421/1 in Axon.Loop.log/5>,
+ #Function<3.77614421/1 in Axon.Loop.build_filter_fn/1>}
+ ],
+ iteration_started: [],
+ started: []
+ },
+ metrics: %{
+ "dense_0_kernel_ema_mean" => {#Function<12.77614421/3 in Axon.Loop.build_metric_fn/3>,
+ &Nx.mean/1},
+ "loss" => {#Function<12.46375131/3 in Axon.Metrics.running_average/1>,
+ #Function<6.77614421/2 in Axon.Loop.build_loss_fn/1>}
+ },
...
->
Then when you run the loop, Axon will use your custom accumulator:
train_data =
- Stream.repeatedly(fn ->
- xs = Nx.random_normal({8, 1})
- ys = Nx.sin(xs)
- {xs, ys}
- end)
-
-Axon.Loop.run(loop, train_data, %{}, iterations: 1000)
Epoch: 0, Batch: 1000, dense_0_kernel_ema_mean: 0.2137861 loss: 0.0709054
%{
- "dense_0" => %{
- "bias" => #Nx.Tensor<
- f32[8]
- [0.08160790055990219, -0.21322371065616608, -0.1431925743818283, 0.2848915755748749, -0.007875560782849789, 0.3923396170139313, -0.04444991424679756, 0.23083189129829407]
- >,
- "kernel" => #Nx.Tensor<
- f32[1][8]
- [
- [-0.6269387006759644, 0.3289071023464203, 0.19450749456882477, 0.7400281429290771, 0.23878233134746552, 0.36140456795692444, 0.10503113269805908, 0.3685782253742218]
- ]
- >
- },
- "dense_1" => %{
- "bias" => #Nx.Tensor<
- f32[4]
- [0.2350393682718277, 0.06712433695793152, -0.03675961494445801, -0.06366443634033203]
- >,
- "kernel" => #Nx.Tensor<
- f32[8][4]
- [
- [-0.35826751589775085, -0.10699580609798431, -0.3681609034538269, 0.08517063409090042],
- [-0.7694831490516663, 0.13644370436668396, -0.2390032261610031, 0.6069303154945374],
- [-0.6424086689949036, 0.13374455273151398, -0.35404452681541443, 0.6343701481819153],
- [-0.09528166800737381, 0.7048070430755615, 0.13699916005134583, 0.6482889652252197],
- [-0.08044164627790451, 0.010588583536446095, 0.11140558868646622, 0.33911004662513733],
- [0.7361723780632019, 0.757600724697113, -0.0011848200811073184, 0.2799053192138672],
- [0.3472788631916046, -0.5225644111633301, 0.04859891161322594, -0.4931156039237976],
- [0.09371320903301239, 0.5478940606117249, 0.5831385254859924, -0.21019525825977325]
- ]
- >
- },
- "dense_2" => %{
- "bias" => #Nx.Tensor<
- f32[1]
- [-0.835706889629364]
- >,
- "kernel" => #Nx.Tensor<
- f32[4][1]
- [
- [1.0109968185424805],
- [0.574639618396759],
- [-0.01302765030413866],
- [-0.008134203962981701]
- ]
- >
- }
-}
+>
Then when you run the loop, Axon will use your custom accumulator:
train_data =
+ Stream.repeatedly(fn ->
+ xs = Nx.random_normal({8, 1})
+ ys = Nx.sin(xs)
+ {xs, ys}
+ end)
+
+Axon.Loop.run(loop, train_data, %{}, iterations: 1000)
Epoch: 0, Batch: 1000, dense_0_kernel_ema_mean: 0.2137861 loss: 0.0709054
%{
+ "dense_0" => %{
+ "bias" => #Nx.Tensor<
+ f32[8]
+ [0.08160790055990219, -0.21322371065616608, -0.1431925743818283, 0.2848915755748749, -0.007875560782849789, 0.3923396170139313, -0.04444991424679756, 0.23083189129829407]
+ >,
+ "kernel" => #Nx.Tensor<
+ f32[1][8]
+ [
+ [-0.6269387006759644, 0.3289071023464203, 0.19450749456882477, 0.7400281429290771, 0.23878233134746552, 0.36140456795692444, 0.10503113269805908, 0.3685782253742218]
+ ]
+ >
+ },
+ "dense_1" => %{
+ "bias" => #Nx.Tensor<
+ f32[4]
+ [0.2350393682718277, 0.06712433695793152, -0.03675961494445801, -0.06366443634033203]
+ >,
+ "kernel" => #Nx.Tensor<
+ f32[8][4]
+ [
+ [-0.35826751589775085, -0.10699580609798431, -0.3681609034538269, 0.08517063409090042],
+ [-0.7694831490516663, 0.13644370436668396, -0.2390032261610031, 0.6069303154945374],
+ [-0.6424086689949036, 0.13374455273151398, -0.35404452681541443, 0.6343701481819153],
+ [-0.09528166800737381, 0.7048070430755615, 0.13699916005134583, 0.6482889652252197],
+ [-0.08044164627790451, 0.010588583536446095, 0.11140558868646622, 0.33911004662513733],
+ [0.7361723780632019, 0.757600724697113, -0.0011848200811073184, 0.2799053192138672],
+ [0.3472788631916046, -0.5225644111633301, 0.04859891161322594, -0.4931156039237976],
+ [0.09371320903301239, 0.5478940606117249, 0.5831385254859924, -0.21019525825977325]
+ ]
+ >
+ },
+ "dense_2" => %{
+ "bias" => #Nx.Tensor<
+ f32[1]
+ [-0.835706889629364]
+ >,
+ "kernel" => #Nx.Tensor<
+ f32[4][1]
+ [
+ [1.0109968185424805],
+ [0.574639618396759],
+ [-0.01302765030413866],
+ [-0.008134203962981701]
+ ]
+ >
+ }
+}
diff --git a/xor.html b/xor.html
index 84ca2d5e..1df7ab92 100644
--- a/xor.html
+++ b/xor.html
@@ -115,14 +115,14 @@
-Mix.install([
- {:axon, "~> 0.3.0"},
- {:nx, "~> 0.4.0", override: true},
- {:exla, "~> 0.4.0"},
- {:kino_vega_lite, "~> 0.1.6"}
-])
+Mix.install([
+ {:axon, "~> 0.3.0"},
+ {:nx, "~> 0.4.0", override: true},
+ {:exla, "~> 0.4.0"},
+ {:kino_vega_lite, "~> 0.1.6"}
+])
-Nx.Defn.default_options(compiler: EXLA)
+Nx.Defn.default_options(compiler: EXLA)
alias VegaLite, as: Vl
@@ -136,14 +136,14 @@
The model
-Let's start with the model. We need two inputs, since XOR has two operands. We then concatenate them into a single input vector with Axon.concatenate/3
. Then we have one hidden layer and one output layer, both of them dense.
Note: the model is a sequential neural network. In Axon, we can conveniently create such a model by using the pipe operator (|>
) to add layers one by one.
x1_input = Axon.input("x1", shape: {nil, 1})
-x2_input = Axon.input("x2", shape: {nil, 1})
+Let's start with the model. We need two inputs, since XOR has two operands. We then concatenate them into a single input vector with Axon.concatenate/3
. Then we have one hidden layer and one output layer, both of them dense.
Note: the model is a sequential neural network. In Axon, we can conveniently create such a model by using the pipe operator (|>
) to add layers one by one.
x1_input = Axon.input("x1", shape: {nil, 1})
+x2_input = Axon.input("x2", shape: {nil, 1})
model =
x1_input
- |> Axon.concatenate(x2_input)
- |> Axon.dense(8, activation: :tanh)
- |> Axon.dense(1, activation: :sigmoid)
+
|> Axon.concatenate(x2_input)
+ |> Axon.dense(8, activation: :tanh)
+ |> Axon.dense(1, activation: :sigmoid)
training-data
@@ -152,13 +152,13 @@
The next step is to prepare training data. Since we are modeling a well-defined operation, we can just generate random operands and compute the expected XOR result for them.
The training works with batches of examples, so we repeatedly generate a whole batch of inputs and the expected result.
batch_size = 32
data =
- Stream.repeatedly(fn ->
- x1 = Nx.random_uniform({batch_size, 1}, 0, 2)
- x2 = Nx.random_uniform({batch_size, 1}, 0, 2)
- y = Nx.logical_xor(x1, x2)
+ Stream.repeatedly(fn ->
+ x1 = Nx.random_uniform({batch_size, 1}, 0, 2)
+ x2 = Nx.random_uniform({batch_size, 1}, 0, 2)
+ y = Nx.logical_xor(x1, x2)
- {%{"x1" => x1, "x2" => x2}, y}
- end)
Here's how a sample batch looks:
Enum.at(data, 0)
+
{%{"x1" => x1, "x2" => x2}, y}
+ end)
Here's how a sample batch looks:
Enum.at(data, 0)
training
@@ -168,17 +168,17 @@
params =
model
- |> Axon.Loop.trainer(:binary_cross_entropy, :sgd)
- |> Axon.Loop.run(data, %{}, epochs: epochs, iterations: 1000)
+ |> Axon.Loop.trainer(:binary_cross_entropy, :sgd)
+ |> Axon.Loop.run(data, %{}, epochs: epochs, iterations: 1000)
trying-the-model
Trying the model
-
Finally, we can test our model on sample data.
Axon.predict(model, params, %{
- "x1" => Nx.tensor([[0]]),
- "x2" => Nx.tensor([[1]])
-})
Try other combinations of $x_1$ and $x_2$ and see what the output is. To improve the model performance, you can increase the number of training epochs.
+
Finally, we can test our model on sample data.
Axon.predict(model, params, %{
+ "x1" => Nx.tensor([[0]]),
+ "x2" => Nx.tensor([[1]])
+})
Try other combinations of $x_1$ and $x_2$ and see what the output is. To improve the model performance, you can increase the number of training epochs.
visualizing-the-model-predictions
@@ -188,22 +188,22 @@
n = 50
# We generate coordinates of in the (n x n) grid
-x1 = Nx.iota({n, n}, axis: 0) |> Nx.divide(n) |> Nx.reshape({:auto, 1})
-x2 = Nx.iota({n, n}, axis: 1) |> Nx.divide(n) |> Nx.reshape({:auto, 1})
+x1 = Nx.iota({n, n}, axis: 0) |> Nx.divide(n) |> Nx.reshape({:auto, 1})
+x2 = Nx.iota({n, n}, axis: 1) |> Nx.divide(n) |> Nx.reshape({:auto, 1})
# The output is also a real number, but we round it into one of the two classes
-y = Axon.predict(model, params, %{"x1" => x1, "x2" => x2}) |> Nx.round()
-
-Vl.new(width: 300, height: 300)
-|> Vl.data_from_values(
- x1: Nx.to_flat_list(x1),
- x2: Nx.to_flat_list(x2),
- y: Nx.to_flat_list(y)
-)
-|> Vl.mark(:circle)
-|> Vl.encode_field(:x, "x1", type: :quantitative)
-|> Vl.encode_field(:y, "x2", type: :quantitative)
-|> Vl.encode_field(:color, "y", type: :nominal)
From the plot we can clearly see that during training our model learnt two clean boundaries to separate $(0,0)$, $(1,1)$ from $(0,1)$, $(1,0)$.
+y = Axon.predict(model, params, %{"x1" => x1, "x2" => x2}) |> Nx.round()
+
+Vl.new(width: 300, height: 300)
+|> Vl.data_from_values(
+ x1: Nx.to_flat_list(x1),
+ x2: Nx.to_flat_list(x2),
+ y: Nx.to_flat_list(y)
+)
+|> Vl.mark(:circle)
+|> Vl.encode_field(:x, "x1", type: :quantitative)
+|> Vl.encode_field(:y, "x2", type: :quantitative)
+|> Vl.encode_field(:color, "y", type: :nominal)From the plot we can clearly see that during training our model learnt two clean boundaries to separate $(0,0)$, $(1,1)$ from $(0,1)$, $(1,0)$.
diff --git a/your_first_axon_model.html b/your_first_axon_model.html
index a707fd02..21c577c4 100644
--- a/your_first_axon_model.html
+++ b/your_first_axon_model.html
@@ -115,30 +115,30 @@
-Mix.install([
- {:axon, github: "elixir-nx/axon"},
- {:nx, github: "elixir-nx/nx", sparse: "nx", override: true},
- {:kino, "~> 0.7.0"}
-])
:ok
+Mix.install([
+ {:axon, github: "elixir-nx/axon"},
+ {:nx, github: "elixir-nx/nx", sparse: "nx", override: true},
+ {:kino, "~> 0.7.0"}
+])
:ok
your-first-model
Your first model
-
Axon is a library for creating and training neural networks in Elixir. Everything in Axon centers around the %Axon{}
struct which represents an instance of an Axon model.
Models are just graphs which represent the transformation and flow of input data to a desired output. Really, you can think of models as representing a single computation or function. An Axon model, when executed, takes data as input and returns transformed data as output.
All Axon models start with a declaration of input nodes. These are the root nodes of your computation graph, and correspond to the actual input data you want to send to Axon:
input = Axon.input("data")
#Axon<
- inputs: %{"data" => nil}
+Axon is a library for creating and training neural networks in Elixir. Everything in Axon centers around the %Axon{}
struct which represents an instance of an Axon model.
Models are just graphs which represent the transformation and flow of input data to a desired output. Really, you can think of models as representing a single computation or function. An Axon model, when executed, takes data as input and returns transformed data as output.
All Axon models start with a declaration of input nodes. These are the root nodes of your computation graph, and correspond to the actual input data you want to send to Axon:
input = Axon.input("data")
#Axon<
+ inputs: %{"data" => nil}
outputs: "data"
nodes: 1
->
Technically speaking, input
is now a valid Axon model which you can inspect, execute, and initialize. You can visualize how data flows through the graph using Axon.Display.as_graph/2
:
template = Nx.template({2, 8}, :f32)
-Axon.Display.as_graph(input, template)
graph TD;
+
>
Technically speaking, input
is now a valid Axon model which you can inspect, execute, and initialize. You can visualize how data flows through the graph using Axon.Display.as_graph/2
:
template = Nx.template({2, 8}, :f32)
+Axon.Display.as_graph(input, template)
graph TD;
3[/"data (:input) {2, 8}"/];
-;
Notice the execution flow is just a single node, because your graph only consists of an input node! You pass data in and the model spits the same data back out, without any intermediate transformations.
You can see this in action by actually executing your model. You can build the %Axon{}
struct into it's initialization
and forward
functions by calling Axon.build/2
. This pattern of "lowering" or transforming the %Axon{}
data structure into other functions or representations is very common in Axon. By simply traversing the data structure, you can create useful functions, execution visualizations, and more!
{init_fn, predict_fn} = Axon.build(input)
{#Function<137.55749718/2 in Nx.Defn.wrap_arity/2>,
- #Function<137.55749718/2 in Nx.Defn.wrap_arity/2>}
Notice that Axon.build/2
returns a tuple of {init_fn, predict_fn}
. init_fn
has the signature:
init_fn.(template :: map(tensor) | tensor, initial_params :: map) :: map(tensor)
while predict_fn
has the signature:
predict_fn.(params :: map(tensor), input :: map(tensor) | tensor)
init_fn
returns all of your model's trainable parameters and state. You need to pass a template of the expected inputs because the shape of certain model parameters often depend on the shape of model inputs. You also need to pass any initial parameters you want your model to start with. This is useful for things like transfer learning, which you can read about in another guide.
predict_fn
returns transformed inputs from your model's trainable parameters and the given inputs.
params = init_fn.(Nx.template({1, 8}, :f32), %{})
%{}
In this example, you use Nx.template/2
to create a template tensor, which is a placeholder that does not actually consume any memory. Templates are useful for initialization because you don't actually need to know anything about your inputs other than their shape and type.
Notice init_fn
returned an empty map because your model does not have any trainable parameters. This should make sense because it's just an input layer.
Now you can pass these trainable parameters to predict_fn
along with some input to actually execute your model:
predict_fn.(params, Nx.iota({1, 8}, type: :f32))
#Nx.Tensor<
- f32[1][8]
- [
- [0.0, 1.0, 2.0, 3.0, 4.0, 5.0, 6.0, 7.0]
- ]
->
And your model just returned the given input, as expected!
+;Notice the execution flow is just a single node, because your graph only consists of an input node! You pass data in and the model spits the same data back out, without any intermediate transformations.
You can see this in action by actually executing your model. You can build the %Axon{}
struct into it's initialization
and forward
functions by calling Axon.build/2
. This pattern of "lowering" or transforming the %Axon{}
data structure into other functions or representations is very common in Axon. By simply traversing the data structure, you can create useful functions, execution visualizations, and more!
{init_fn, predict_fn} = Axon.build(input)
{#Function<137.55749718/2 in Nx.Defn.wrap_arity/2>,
+ #Function<137.55749718/2 in Nx.Defn.wrap_arity/2>}
Notice that Axon.build/2
returns a tuple of {init_fn, predict_fn}
. init_fn
has the signature:
init_fn.(template :: map(tensor) | tensor, initial_params :: map) :: map(tensor)
while predict_fn
has the signature:
predict_fn.(params :: map(tensor), input :: map(tensor) | tensor)
init_fn
returns all of your model's trainable parameters and state. You need to pass a template of the expected inputs because the shape of certain model parameters often depend on the shape of model inputs. You also need to pass any initial parameters you want your model to start with. This is useful for things like transfer learning, which you can read about in another guide.
predict_fn
returns transformed inputs from your model's trainable parameters and the given inputs.
params = init_fn.(Nx.template({1, 8}, :f32), %{})
%{}
In this example, you use Nx.template/2
to create a template tensor, which is a placeholder that does not actually consume any memory. Templates are useful for initialization because you don't actually need to know anything about your inputs other than their shape and type.
Notice init_fn
returned an empty map because your model does not have any trainable parameters. This should make sense because it's just an input layer.
Now you can pass these trainable parameters to predict_fn
along with some input to actually execute your model:
predict_fn.(params, Nx.iota({1, 8}, type: :f32))
#Nx.Tensor<
+ f32[1][8]
+ [
+ [0.0, 1.0, 2.0, 3.0, 4.0, 5.0, 6.0, 7.0]
+ ]
+>
And your model just returned the given input, as expected!
diff --git a/your_first_evaluation_loop.html b/your_first_evaluation_loop.html
index fcd6b7cf..3104bb81 100644
--- a/your_first_evaluation_loop.html
+++ b/your_first_evaluation_loop.html
@@ -115,122 +115,122 @@
-Mix.install([
- {:axon, github: "elixir-nx/axon"},
- {:nx, "~> 0.3.0", github: "elixir-nx/nx", sparse: "nx", override: true}
-])
:ok
+Mix.install([
+ {:axon, github: "elixir-nx/axon"},
+ {:nx, "~> 0.3.0", github: "elixir-nx/nx", sparse: "nx", override: true}
+])
:ok
creating-an-axon-evaluation-loop
Creating an Axon evaluation loop
Once you have a trained model, it's necessary to test the trained model on some test data. Axon's loop abstraction is general enough to work for both training and evaluating models. Just as Axon implements a canned Axon.Loop.trainer/3
factory, it also implements a canned Axon.Loop.evaluator/1
factory.
Axon.Loop.evaluator/1
creates an evaluation loop which you can instrument with metrics to measure the performance of a trained model on test data. First, you need a trained model:
model =
- Axon.input("data")
- |> Axon.dense(8)
- |> Axon.relu()
- |> Axon.dense(4)
- |> Axon.relu()
- |> Axon.dense(1)
+ Axon.input("data")
+ |> Axon.dense(8)
+ |> Axon.relu()
+ |> Axon.dense(4)
+ |> Axon.relu()
+ |> Axon.dense(1)
-train_loop = Axon.Loop.trainer(model, :mean_squared_error, :sgd)
+train_loop = Axon.Loop.trainer(model, :mean_squared_error, :sgd)
data =
- Stream.repeatedly(fn ->
- xs = Nx.random_normal({8, 1})
- ys = Nx.sin(xs)
- {xs, ys}
- end)
-
-trained_model_state = Axon.Loop.run(train_loop, data, %{}, iterations: 1000)
Epoch: 0, Batch: 1000, loss: 0.0348526
%{
- "dense_0" => %{
- "bias" => #Nx.Tensor<
- f32[8]
- [0.12334823608398438, 0.23830991983413696, 0.07463178038597107, -0.18479900062084198, -0.2544017434120178, -0.1100262850522995, 0.04137010499835014, 0.22781872749328613]
- >,
- "kernel" => #Nx.Tensor<
- f32[1][8]
- [
- [-0.7397015690803528, 0.8709579110145569, -0.33129510283470154, -0.4521639347076416, -0.5752679109573364, 0.5516160726547241, -0.1265108585357666, -0.5665484666824341]
- ]
- >
- },
- "dense_1" => %{
- "bias" => #Nx.Tensor<
- f32[4]
- [7.311657827813178e-5, -0.027584673836827278, 0.20344746112823486, 0.1330498605966568]
- >,
- "kernel" => #Nx.Tensor<
- f32[8][4]
- [
- [-0.19199007749557495, 0.15660767257213593, 0.5446576476097107, 0.07457015663385391],
- [0.034533075988292694, -0.10262273252010345, 0.05103863775730133, 0.5708968639373779],
- [-0.4212855398654938, -0.47742989659309387, 0.18940746784210205, -0.40659299492836],
- [0.2127801775932312, -0.07477620989084244, -0.11274989694356918, 0.4552466869354248],
- [-0.13839538395404816, 0.09832656383514404, -0.16157560050487518, 0.7074514627456665],
- [-0.6366024017333984, 0.3754875361919403, -0.6808919906616211, -0.209626242518425],
- [0.595952033996582, 0.6973875164985657, 0.4453340172767639, 0.6247327327728271],
- [-0.6312451958656311, 0.33275362849235535, 0.5079866051673889, -0.2508215010166168]
- ]
- >
- },
- "dense_2" => %{
- "bias" => #Nx.Tensor<
- f32[1]
- [0.17476916313171387]
- >,
- "kernel" => #Nx.Tensor<
- f32[4][1]
- [
- [0.8893225193023682],
- [-0.4548797905445099],
- [-0.8288624286651611],
- [0.8321414589881897]
- ]
- >
- }
-}
Running loops with Axon.Loop.trainer/3
returns a trained model state which you can use to evaluate your model. To construct an evaluation loop, you just call Axon.Loop.evaluator/1
with your pre-trained model:
test_loop = Axon.Loop.evaluator(model)
#Axon.Loop<
- handlers: %{
- completed: [],
- epoch_completed: [],
- epoch_halted: [],
- epoch_started: [],
- halted: [],
- iteration_completed: [
- {#Function<23.20267452/1 in Axon.Loop.log/5>,
- #Function<5.20267452/1 in Axon.Loop.build_filter_fn/1>}
- ],
- iteration_started: [],
- started: []
- },
- metrics: %{},
+ Stream.repeatedly(fn ->
+ xs = Nx.random_normal({8, 1})
+ ys = Nx.sin(xs)
+ {xs, ys}
+ end)
+
+trained_model_state = Axon.Loop.run(train_loop, data, %{}, iterations: 1000)
Epoch: 0, Batch: 1000, loss: 0.0348526
%{
+ "dense_0" => %{
+ "bias" => #Nx.Tensor<
+ f32[8]
+ [0.12334823608398438, 0.23830991983413696, 0.07463178038597107, -0.18479900062084198, -0.2544017434120178, -0.1100262850522995, 0.04137010499835014, 0.22781872749328613]
+ >,
+ "kernel" => #Nx.Tensor<
+ f32[1][8]
+ [
+ [-0.7397015690803528, 0.8709579110145569, -0.33129510283470154, -0.4521639347076416, -0.5752679109573364, 0.5516160726547241, -0.1265108585357666, -0.5665484666824341]
+ ]
+ >
+ },
+ "dense_1" => %{
+ "bias" => #Nx.Tensor<
+ f32[4]
+ [7.311657827813178e-5, -0.027584673836827278, 0.20344746112823486, 0.1330498605966568]
+ >,
+ "kernel" => #Nx.Tensor<
+ f32[8][4]
+ [
+ [-0.19199007749557495, 0.15660767257213593, 0.5446576476097107, 0.07457015663385391],
+ [0.034533075988292694, -0.10262273252010345, 0.05103863775730133, 0.5708968639373779],
+ [-0.4212855398654938, -0.47742989659309387, 0.18940746784210205, -0.40659299492836],
+ [0.2127801775932312, -0.07477620989084244, -0.11274989694356918, 0.4552466869354248],
+ [-0.13839538395404816, 0.09832656383514404, -0.16157560050487518, 0.7074514627456665],
+ [-0.6366024017333984, 0.3754875361919403, -0.6808919906616211, -0.209626242518425],
+ [0.595952033996582, 0.6973875164985657, 0.4453340172767639, 0.6247327327728271],
+ [-0.6312451958656311, 0.33275362849235535, 0.5079866051673889, -0.2508215010166168]
+ ]
+ >
+ },
+ "dense_2" => %{
+ "bias" => #Nx.Tensor<
+ f32[1]
+ [0.17476916313171387]
+ >,
+ "kernel" => #Nx.Tensor<
+ f32[4][1]
+ [
+ [0.8893225193023682],
+ [-0.4548797905445099],
+ [-0.8288624286651611],
+ [0.8321414589881897]
+ ]
+ >
+ }
+}
Running loops with Axon.Loop.trainer/3
returns a trained model state which you can use to evaluate your model. To construct an evaluation loop, you just call Axon.Loop.evaluator/1
with your pre-trained model:
test_loop = Axon.Loop.evaluator(model)
#Axon.Loop<
+ handlers: %{
+ completed: [],
+ epoch_completed: [],
+ epoch_halted: [],
+ epoch_started: [],
+ halted: [],
+ iteration_completed: [
+ {#Function<23.20267452/1 in Axon.Loop.log/5>,
+ #Function<5.20267452/1 in Axon.Loop.build_filter_fn/1>}
+ ],
+ iteration_started: [],
+ started: []
+ },
+ metrics: %{},
...
->
Next, you'll need to instrument your test loop with the metrics you'd like to aggregate:
test_loop = test_loop |> Axon.Loop.metric(:mean_absolute_error)
#Axon.Loop<
- handlers: %{
- completed: [],
- epoch_completed: [],
- epoch_halted: [],
- epoch_started: [],
- halted: [],
- iteration_completed: [
- {#Function<23.20267452/1 in Axon.Loop.log/5>,
- #Function<5.20267452/1 in Axon.Loop.build_filter_fn/1>}
- ],
- iteration_started: [],
- started: []
- },
- metrics: %{
- "mean_absolute_error" => {#Function<12.6031754/3 in Axon.Metrics.running_average/1>,
- :mean_absolute_error}
- },
+>
Next, you'll need to instrument your test loop with the metrics you'd like to aggregate:
test_loop = test_loop |> Axon.Loop.metric(:mean_absolute_error)
#Axon.Loop<
+ handlers: %{
+ completed: [],
+ epoch_completed: [],
+ epoch_halted: [],
+ epoch_started: [],
+ halted: [],
+ iteration_completed: [
+ {#Function<23.20267452/1 in Axon.Loop.log/5>,
+ #Function<5.20267452/1 in Axon.Loop.build_filter_fn/1>}
+ ],
+ iteration_started: [],
+ started: []
+ },
+ metrics: %{
+ "mean_absolute_error" => {#Function<12.6031754/3 in Axon.Metrics.running_average/1>,
+ :mean_absolute_error}
+ },
...
->
Finally, you can run your loop on test data. Because you want to test your trained model, you need to provide your model's initial state to the test loop:
Axon.Loop.run(test_loop, data, trained_model_state, iterations: 1000)
Batch: 1000, mean_absolute_error: 0.0955574
%{
- 0 => %{
- "mean_absolute_error" => #Nx.Tensor<
+>
Finally, you can run your loop on test data. Because you want to test your trained model, you need to provide your model's initial state to the test loop:
Axon.Loop.run(test_loop, data, trained_model_state, iterations: 1000)
Batch: 1000, mean_absolute_error: 0.0955574
%{
+ 0 => %{
+ "mean_absolute_error" => #Nx.Tensor<
f32
0.09555738419294357
- >
- }
-}
+ >
+ }
+}
diff --git a/your_first_training_loop.html b/your_first_training_loop.html
index 26f9dea5..e3810554 100644
--- a/your_first_training_loop.html
+++ b/your_first_training_loop.html
@@ -115,198 +115,198 @@
-Mix.install([
- {:axon, github: "elixir-nx/axon"},
- {:nx, "~> 0.3.0", github: "elixir-nx/nx", sparse: "nx", override: true}
-])
:ok
+Mix.install([
+ {:axon, github: "elixir-nx/axon"},
+ {:nx, "~> 0.3.0", github: "elixir-nx/nx", sparse: "nx", override: true}
+])
:ok
creating-an-axon-training-loop
Creating an Axon training loop
Axon generalizes the concept of training, evaluation, hyperparameter optimization, and more into the Axon.Loop
API. Axon loops are a instrumented reductions over Elixir Streams - that basically means you can accumulate some state over an Elixir Stream
and control different points in the loop execution.
With Axon, you'll most commonly implement and work with supervised training loops. Because supervised training loops are so common in deep learning, Axon has a loop factory function which takes care of most of the boilerplate of creating a supervised training loop for you. In the beginning of your deep learning journey, you'll almost exclusively use Axon's loop factories to create and run loops.
Axon's supervised training loop assumes you have an input stream of data with entries that look like:
{batch_inputs, batch_labels}
Each entry is a batch of input data with a corresponding batch of labels. You can simulate some real training data by constructing an Elixir stream:
train_data =
- Stream.repeatedly(fn ->
- xs = Nx.random_normal({8, 1})
- ys = Nx.sin(xs)
- {xs, ys}
- end)
#Function<50.127921642/2 in Stream.repeatedly/1>
The most basic supervised training loop in Axon requires 3 things:
- An Axon model
- A loss function
- An optimizer
You can construct an Axon model using the knowledge you've gained from going through the model creation guides:
model =
- Axon.input("data")
- |> Axon.dense(8)
- |> Axon.relu()
- |> Axon.dense(4)
- |> Axon.relu()
- |> Axon.dense(1)
#Axon<
- inputs: %{"data" => nil}
+ Stream.repeatedly(fn ->
+ xs = Nx.random_normal({8, 1})
+ ys = Nx.sin(xs)
+ {xs, ys}
+ end)
#Function<50.127921642/2 in Stream.repeatedly/1>
The most basic supervised training loop in Axon requires 3 things:
- An Axon model
- A loss function
- An optimizer
You can construct an Axon model using the knowledge you've gained from going through the model creation guides:
model =
+ Axon.input("data")
+ |> Axon.dense(8)
+ |> Axon.relu()
+ |> Axon.dense(4)
+ |> Axon.relu()
+ |> Axon.dense(1)
#Axon<
+ inputs: %{"data" => nil}
outputs: "dense_2"
nodes: 6
->
Axon comes with built-in loss functions and optimizers which you can use directly when constructing your training loop. To construct your training loop, you use Axon.Loop.trainer/3
:
loop = Axon.Loop.trainer(model, :mean_squared_error, :sgd)
#Axon.Loop<
- handlers: %{
- completed: [],
- epoch_completed: [
- {#Function<23.20267452/1 in Axon.Loop.log/5>,
- #Function<5.20267452/1 in Axon.Loop.build_filter_fn/1>}
- ],
- epoch_halted: [],
- epoch_started: [],
- halted: [],
- iteration_completed: [
- {#Function<23.20267452/1 in Axon.Loop.log/5>,
- #Function<3.20267452/1 in Axon.Loop.build_filter_fn/1>}
- ],
- iteration_started: [],
- started: []
- },
- metrics: %{
- "loss" => {#Function<12.17233431/3 in Axon.Metrics.running_average/1>,
- #Function<6.20267452/2 in Axon.Loop.build_loss_fn/1>}
- },
+>
Axon comes with built-in loss functions and optimizers which you can use directly when constructing your training loop. To construct your training loop, you use Axon.Loop.trainer/3
:
loop = Axon.Loop.trainer(model, :mean_squared_error, :sgd)
#Axon.Loop<
+ handlers: %{
+ completed: [],
+ epoch_completed: [
+ {#Function<23.20267452/1 in Axon.Loop.log/5>,
+ #Function<5.20267452/1 in Axon.Loop.build_filter_fn/1>}
+ ],
+ epoch_halted: [],
+ epoch_started: [],
+ halted: [],
+ iteration_completed: [
+ {#Function<23.20267452/1 in Axon.Loop.log/5>,
+ #Function<3.20267452/1 in Axon.Loop.build_filter_fn/1>}
+ ],
+ iteration_started: [],
+ started: []
+ },
+ metrics: %{
+ "loss" => {#Function<12.17233431/3 in Axon.Metrics.running_average/1>,
+ #Function<6.20267452/2 in Axon.Loop.build_loss_fn/1>}
+ },
...
->
You'll notice that Axon.Loop.trainer/3
returns an %Axon.Loop{}
data structure. This data structure contains information which Axon uses to control the execution of the loop. In order to run the loop, you need to explicitly pass it to Axon.Loop.run/4
:
Axon.Loop.run(loop, train_data, %{}, iterations: 1000)
Epoch: 0, Batch: 1000, loss: 0.0421094
%{
- "dense_0" => %{
- "bias" => #Nx.Tensor<
- f32[8]
- [0.18567155301570892, -0.24138866364955902, 0.13732704520225525, 0.2081741988658905, 0.013805730268359184, 0.18336650729179382, 0.07754829525947571, -0.12579604983329773]
- >,
- "kernel" => #Nx.Tensor<
- f32[1][8]
- [
- [0.06517036259174347, -0.7166120409965515, 0.649202823638916, -0.3636767566204071, 0.33472830057144165, -0.6622008681297302, -0.6205887198448181, -0.1951046586036682]
- ]
- >
- },
- "dense_1" => %{
- "bias" => #Nx.Tensor<
- f32[4]
- [0.2652607262134552, 0.1563350260257721, -0.12963515520095825, -0.15289783477783203]
- >,
- "kernel" => #Nx.Tensor<
- f32[8][4]
- [
- [0.5483533143997192, 0.16270962357521057, -0.29001912474632263, 0.16584330797195435],
- [-0.3257339596748352, 0.6900827884674072, 0.17480286955833435, -0.5176011323928833],
- [-0.5791758298873901, 0.7136418223381042, 0.2863248288631439, 0.2406335324048996],
- [0.5999854803085327, -0.09972921013832092, 0.16846133768558502, 0.21690420806407928],
- [0.10213596373796463, 0.01878557913005352, 0.03252492845058441, -0.25937923789024353],
- [0.4094444811344147, -0.48399242758750916, 0.18455447256565094, 0.40939682722091675],
- [0.2809498906135559, 0.7121831178665161, 0.42944926023483276, -0.4959437847137451],
- [-0.21076196432113647, -0.3021833896636963, -0.46126121282577515, -0.5571116805076599]
- ]
- >
- },
- "dense_2" => %{
- "bias" => #Nx.Tensor<
- f32[1]
- [0.3293934762477875]
- >,
- "kernel" => #Nx.Tensor<
- f32[4][1]
- [
- [-1.041453242301941],
- [0.6521084308624268],
- [-0.5688052773475647],
- [-0.5789349675178528]
- ]
- >
- }
-}
Axon.Loop.run/4
expects a loop to execute, some data to loop over, and any initial state you explicitly want your loop to start with. Axon.Loop.run/4
will then iterate over your data, executing a step function on each batch, and accumulating some generic loop state. In the case of a supervised training loop, this generic loop state actually represents training state including your model's trained parameters.
Axon.Loop.run/4
also accepts options which control the loops execution. This includes :iterations
which controls the number of iterations per epoch a loop should execute for, and :epochs
which controls the number of epochs a loop should execute for:
Axon.Loop.run(loop, train_data, %{}, epochs: 3, iterations: 500)
Epoch: 0, Batch: 500, loss: 0.0376754
+>
You'll notice that Axon.Loop.trainer/3
returns an %Axon.Loop{}
data structure. This data structure contains information which Axon uses to control the execution of the loop. In order to run the loop, you need to explicitly pass it to Axon.Loop.run/4
:
Axon.Loop.run(loop, train_data, %{}, iterations: 1000)
Epoch: 0, Batch: 1000, loss: 0.0421094
%{
+ "dense_0" => %{
+ "bias" => #Nx.Tensor<
+ f32[8]
+ [0.18567155301570892, -0.24138866364955902, 0.13732704520225525, 0.2081741988658905, 0.013805730268359184, 0.18336650729179382, 0.07754829525947571, -0.12579604983329773]
+ >,
+ "kernel" => #Nx.Tensor<
+ f32[1][8]
+ [
+ [0.06517036259174347, -0.7166120409965515, 0.649202823638916, -0.3636767566204071, 0.33472830057144165, -0.6622008681297302, -0.6205887198448181, -0.1951046586036682]
+ ]
+ >
+ },
+ "dense_1" => %{
+ "bias" => #Nx.Tensor<
+ f32[4]
+ [0.2652607262134552, 0.1563350260257721, -0.12963515520095825, -0.15289783477783203]
+ >,
+ "kernel" => #Nx.Tensor<
+ f32[8][4]
+ [
+ [0.5483533143997192, 0.16270962357521057, -0.29001912474632263, 0.16584330797195435],
+ [-0.3257339596748352, 0.6900827884674072, 0.17480286955833435, -0.5176011323928833],
+ [-0.5791758298873901, 0.7136418223381042, 0.2863248288631439, 0.2406335324048996],
+ [0.5999854803085327, -0.09972921013832092, 0.16846133768558502, 0.21690420806407928],
+ [0.10213596373796463, 0.01878557913005352, 0.03252492845058441, -0.25937923789024353],
+ [0.4094444811344147, -0.48399242758750916, 0.18455447256565094, 0.40939682722091675],
+ [0.2809498906135559, 0.7121831178665161, 0.42944926023483276, -0.4959437847137451],
+ [-0.21076196432113647, -0.3021833896636963, -0.46126121282577515, -0.5571116805076599]
+ ]
+ >
+ },
+ "dense_2" => %{
+ "bias" => #Nx.Tensor<
+ f32[1]
+ [0.3293934762477875]
+ >,
+ "kernel" => #Nx.Tensor<
+ f32[4][1]
+ [
+ [-1.041453242301941],
+ [0.6521084308624268],
+ [-0.5688052773475647],
+ [-0.5789349675178528]
+ ]
+ >
+ }
+}
Axon.Loop.run/4
expects a loop to execute, some data to loop over, and any initial state you explicitly want your loop to start with. Axon.Loop.run/4
will then iterate over your data, executing a step function on each batch, and accumulating some generic loop state. In the case of a supervised training loop, this generic loop state actually represents training state including your model's trained parameters.
Axon.Loop.run/4
also accepts options which control the loops execution. This includes :iterations
which controls the number of iterations per epoch a loop should execute for, and :epochs
which controls the number of epochs a loop should execute for:
Axon.Loop.run(loop, train_data, %{}, epochs: 3, iterations: 500)
Epoch: 0, Batch: 500, loss: 0.0376754
Epoch: 1, Batch: 500, loss: 0.0300909
-Epoch: 2, Batch: 500, loss: 0.0260511
%{
- "dense_0" => %{
- "bias" => #Nx.Tensor<
- f32[8]
- [-0.09743800014257431, 0.36350908875465393, 0.23338767886161804, 0.21299506723880768, -0.04753172770142555, -0.03144805133342743, 0.0230794008821249, -0.17029045522212982]
- >,
- "kernel" => #Nx.Tensor<
- f32[1][8]
- [
- [-0.14422392845153809, -0.3840259611606598, 0.7611677050590515, 0.1216919794678688, -0.4270862638950348, 0.43146076798439026, -0.3569082021713257, 0.4051334857940674]
- ]
- >
- },
- "dense_1" => %{
- "bias" => #Nx.Tensor<
- f32[4]
- [0.21392156183719635, 0.02405611053109169, 0.2970339059829712, 0.02390623465180397]
- >,
- "kernel" => #Nx.Tensor<
- f32[8][4]
- [
- [-0.12441369146108627, 0.44625332951545715, -0.2095455527305603, -0.28127536177635193],
- [0.6052687764167786, 0.1358352154493332, -0.24579593539237976, 0.6278529167175293],
- [-0.5855410695075989, 0.014370989985764027, 0.4479483664035797, -0.07460466772317886],
- [0.5286814570426941, -0.6323351263999939, 0.4167028069496155, -0.4724753797054291],
- [-0.3705250918865204, 0.41602230072021484, -0.626926600933075, -0.03850430250167847],
- [0.22140666842460632, -0.6492624878883362, 0.09525017440319061, 0.3179352283477783],
- [-0.27787405252456665, 0.43634578585624695, 0.2430884689092636, 0.18133315443992615],
- [0.4248749911785126, -0.059922583401203156, -0.09462974965572357, 0.57406085729599]
- ]
- >
- },
- "dense_2" => %{
- "bias" => #Nx.Tensor<
- f32[1]
- [0.015223611146211624]
- >,
- "kernel" => #Nx.Tensor<
- f32[4][1]
- [
- [-0.6736029386520386],
- [-0.019722800701856613],
- [0.932664692401886],
- [-0.9208926558494568]
- ]
- >
- }
-}
You may have noticed that by default Axon.Loop.trainer/3
configures your loop to log information about training progress every 50 iterations. You can control this when constructing your supervised training loop with the :log
option:
model
-|> Axon.Loop.trainer(:mean_squared_error, :sgd, log: 100)
-|> Axon.Loop.run(train_data, %{}, iterations: 1000)
Epoch: 0, Batch: 1000, loss: 0.0700251
%{
- "dense_0" => %{
- "bias" => #Nx.Tensor<
- f32[8]
- [-0.10562735795974731, 0.3525764048099518, -0.0731351301074028, 0.3316117525100708, -0.08621923625469208, 0.15377338230609894, 0.02795499749481678, 0.19813594222068787]
- >,
- "kernel" => #Nx.Tensor<
- f32[1][8]
- [
- [0.46547073125839233, -0.3838779926300049, 0.06413891166448593, 0.6604263186454773, 0.09603694081306458, -0.3142688274383545, -0.0673874095082283, -0.1551232486963272]
- ]
- >
- },
- "dense_1" => %{
- "bias" => #Nx.Tensor<
- f32[4]
- [0.16770508885383606, -0.11785938590765, -0.08730955421924591, 0.18854482471942902]
- >,
- "kernel" => #Nx.Tensor<
- f32[8][4]
- [
- [-0.32443270087242126, 0.33927711844444275, 0.5110990405082703, -0.34353166818618774],
- [0.6843343377113342, -0.09189904481172562, 0.4550926983356476, -0.27025723457336426],
- [0.029612643644213676, 0.3680649697780609, 0.5105444192886353, -0.1120513379573822],
- [-0.12359219789505005, -0.2177252620458603, -0.2753210961818695, 0.7462171912193298],
- [0.2723115086555481, 0.39580288529396057, -0.41799622774124146, 0.003858723910525441],
- [0.21861012279987335, -0.37737029790878296, -0.5444738268852234, -0.12978340685367584],
- [0.12569139897823334, 0.09505560994148254, 0.13603702187538147, 0.20154744386672974],
- [0.4721740484237671, 0.27258655428886414, -0.6905713677406311, 0.09732398390769958]
- ]
- >
- },
- "dense_2" => %{
- "bias" => #Nx.Tensor<
- f32[1]
- [0.2536466121673584]
- >,
- "kernel" => #Nx.Tensor<
- f32[4][1]
- [
- [-0.9850672483444214],
- [-0.5319440960884094],
- [-0.8099393844604492],
- [0.6502916216850281]
- ]
- >
- }
-}
+Epoch: 2, Batch: 500, loss: 0.0260511%{
+ "dense_0" => %{
+ "bias" => #Nx.Tensor<
+ f32[8]
+ [-0.09743800014257431, 0.36350908875465393, 0.23338767886161804, 0.21299506723880768, -0.04753172770142555, -0.03144805133342743, 0.0230794008821249, -0.17029045522212982]
+ >,
+ "kernel" => #Nx.Tensor<
+ f32[1][8]
+ [
+ [-0.14422392845153809, -0.3840259611606598, 0.7611677050590515, 0.1216919794678688, -0.4270862638950348, 0.43146076798439026, -0.3569082021713257, 0.4051334857940674]
+ ]
+ >
+ },
+ "dense_1" => %{
+ "bias" => #Nx.Tensor<
+ f32[4]
+ [0.21392156183719635, 0.02405611053109169, 0.2970339059829712, 0.02390623465180397]
+ >,
+ "kernel" => #Nx.Tensor<
+ f32[8][4]
+ [
+ [-0.12441369146108627, 0.44625332951545715, -0.2095455527305603, -0.28127536177635193],
+ [0.6052687764167786, 0.1358352154493332, -0.24579593539237976, 0.6278529167175293],
+ [-0.5855410695075989, 0.014370989985764027, 0.4479483664035797, -0.07460466772317886],
+ [0.5286814570426941, -0.6323351263999939, 0.4167028069496155, -0.4724753797054291],
+ [-0.3705250918865204, 0.41602230072021484, -0.626926600933075, -0.03850430250167847],
+ [0.22140666842460632, -0.6492624878883362, 0.09525017440319061, 0.3179352283477783],
+ [-0.27787405252456665, 0.43634578585624695, 0.2430884689092636, 0.18133315443992615],
+ [0.4248749911785126, -0.059922583401203156, -0.09462974965572357, 0.57406085729599]
+ ]
+ >
+ },
+ "dense_2" => %{
+ "bias" => #Nx.Tensor<
+ f32[1]
+ [0.015223611146211624]
+ >,
+ "kernel" => #Nx.Tensor<
+ f32[4][1]
+ [
+ [-0.6736029386520386],
+ [-0.019722800701856613],
+ [0.932664692401886],
+ [-0.9208926558494568]
+ ]
+ >
+ }
+}
You may have noticed that by default Axon.Loop.trainer/3
configures your loop to log information about training progress every 50 iterations. You can control this when constructing your supervised training loop with the :log
option:
model
+|> Axon.Loop.trainer(:mean_squared_error, :sgd, log: 100)
+|> Axon.Loop.run(train_data, %{}, iterations: 1000)
Epoch: 0, Batch: 1000, loss: 0.0700251
%{
+ "dense_0" => %{
+ "bias" => #Nx.Tensor<
+ f32[8]
+ [-0.10562735795974731, 0.3525764048099518, -0.0731351301074028, 0.3316117525100708, -0.08621923625469208, 0.15377338230609894, 0.02795499749481678, 0.19813594222068787]
+ >,
+ "kernel" => #Nx.Tensor<
+ f32[1][8]
+ [
+ [0.46547073125839233, -0.3838779926300049, 0.06413891166448593, 0.6604263186454773, 0.09603694081306458, -0.3142688274383545, -0.0673874095082283, -0.1551232486963272]
+ ]
+ >
+ },
+ "dense_1" => %{
+ "bias" => #Nx.Tensor<
+ f32[4]
+ [0.16770508885383606, -0.11785938590765, -0.08730955421924591, 0.18854482471942902]
+ >,
+ "kernel" => #Nx.Tensor<
+ f32[8][4]
+ [
+ [-0.32443270087242126, 0.33927711844444275, 0.5110990405082703, -0.34353166818618774],
+ [0.6843343377113342, -0.09189904481172562, 0.4550926983356476, -0.27025723457336426],
+ [0.029612643644213676, 0.3680649697780609, 0.5105444192886353, -0.1120513379573822],
+ [-0.12359219789505005, -0.2177252620458603, -0.2753210961818695, 0.7462171912193298],
+ [0.2723115086555481, 0.39580288529396057, -0.41799622774124146, 0.003858723910525441],
+ [0.21861012279987335, -0.37737029790878296, -0.5444738268852234, -0.12978340685367584],
+ [0.12569139897823334, 0.09505560994148254, 0.13603702187538147, 0.20154744386672974],
+ [0.4721740484237671, 0.27258655428886414, -0.6905713677406311, 0.09732398390769958]
+ ]
+ >
+ },
+ "dense_2" => %{
+ "bias" => #Nx.Tensor<
+ f32[1]
+ [0.2536466121673584]
+ >,
+ "kernel" => #Nx.Tensor<
+ f32[4][1]
+ [
+ [-0.9850672483444214],
+ [-0.5319440960884094],
+ [-0.8099393844604492],
+ [0.6502916216850281]
+ ]
+ >
+ }
+}