You signed in with another tab or window. Reload to refresh your session.You signed out in another tab or window. Reload to refresh your session.You switched accounts on another tab or window. Reload to refresh your session.Dismiss alert
Hello, thanks for your work, I have tried to rewrite your network using PyTorch, but what I got from the network is a coarse image where I can only see the profile of my segmentation object, would you like to tell me where I was wrong, thanks!
Hello, thanks for your work, I have tried to rewrite your network using PyTorch, but what I got from the network is a coarse image where I can only see the profile of my segmentation object, would you like to tell me where I was wrong, thanks!
my model code is like this:
and my output is like:
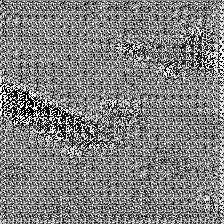
The text was updated successfully, but these errors were encountered: