You signed in with another tab or window. Reload to refresh your session.You signed out in another tab or window. Reload to refresh your session.You switched accounts on another tab or window. Reload to refresh your session.Dismiss alert
I am trying to train ds2 on THCHS30 which is a mandarin dataset. A phenomenon is that we encounter error explosion easily when batch_size is big liking 64, 128 or 256. I try to clip the error to 1000 when inf appears. The clipping operation is very tricky, I catch inf and clip in paddle/v2/trainer.py as following:
We can train ds2 normally after adding the clipping operation. I have tried to train the model using batch_shuffle_clipped provider and instance_shuffle provider. The learning curves are as following:
As we can see, instance_shuffle converges badly and I abandon this configuration after training several iterations. The batch_shuffle_clipped configuration looks like converging very slowly when the training cost goes down to 170~. The key settings of above experiments are:
setting
value
batch_size
64
trainer_count
4
num_conv_layers
2
adam_learning_rate
0.0005
I also product another experiment on a tiny dataset which only contains 128 samples (first 128). Training data and validation data both use the tiny dataset. Training settings are same with above. Following are the learning curves:
From the figure above we can learn that the convergence is very unstable and slow. There exists a unreasonable gap between training curve and validation curve. Since the training data and validation data are same, the difference of two curves should be minor. Will figure out the reason of the anomalies.
The text was updated successfully, but these errors were encountered:
After some digging, a serious bug is found in Warp CTC Layer. This bug has been fixed by #3105. After Integrating this fixing, I conduct more experiments on THCHS30 and the model seems converging normally. First, I tried to train ds2 on a single example, the model converged to almost 0 in three experiments. Second, I tried to train on 10 examples and it converged to 5~ in 200 epochs. It seems that currently the model works reasonably. I'm trying to train the model on full THCHS30 dataset and following is the learning curve:
您好,此issue在近一个月内暂无更新,我们将于今天内关闭。若在关闭后您仍需跟进提问,可重新开启此问题,我们将在24小时内回复您。因关闭带来的不便我们深表歉意,请您谅解~感谢您对PaddlePaddle的支持!
Hello, this issue has not been updated in the past month. We will close it today for the sake of other user‘s experience. If you still need to follow up on this question after closing, please feel free to reopen it. In that case, we will get back to you within 24 hours. We apologize for the inconvenience caused by the closure and thank you so much for your support of PaddlePaddle Group!
I am trying to train ds2 on THCHS30 which is a mandarin dataset. A phenomenon is that we encounter error explosion easily when
batch_size
is big liking 64, 128 or 256. I try to clip the error to 1000 wheninf
appears. The clipping operation is very tricky, I catchinf
and clip inpaddle/v2/trainer.py
as following:We can train ds2 normally after adding the clipping operation. I have tried to train the model using
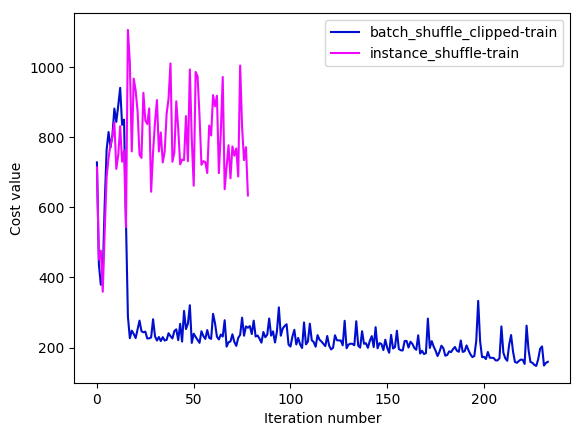
batch_shuffle_clipped
provider andinstance_shuffle
provider. The learning curves are as following:As we can see,
instance_shuffle
converges badly and I abandon this configuration after training several iterations. Thebatch_shuffle_clipped
configuration looks like converging very slowly when the training cost goes down to 170~. The key settings of above experiments are:I also product another experiment on a tiny dataset which only contains 128 samples (first 128). Training data and validation data both use the tiny dataset. Training settings are same with above. Following are the learning curves:
From the figure above we can learn that the convergence is very unstable and slow. There exists a unreasonable gap between training curve and validation curve. Since the training data and validation data are same, the difference of two curves should be minor. Will figure out the reason of the anomalies.
The text was updated successfully, but these errors were encountered: