-
Notifications
You must be signed in to change notification settings - Fork 94
New issue
Have a question about this project? Sign up for a free GitHub account to open an issue and contact its maintainers and the community.
By clicking “Sign up for GitHub”, you agree to our terms of service and privacy statement. We’ll occasionally send you account related emails.
Already on GitHub? Sign in to your account
[RobertaForSequenceClassification] RAM memory leaks during retrieving word attributions #78
Comments
Hi @cdpierse 👋 do you know what could be the workaround here? |
Hi @roma-glushko, Thanks for using the package and for such a detailed overview of the issue you are running into. I think I have a fix for it. It's not well documented yet (which I must do) but in one of the more recent releases, I added a new feature to control the internal batch size that is passed when calculating the attributions. By default, the example text and every n_steps used to approximate the attributions are all calculated at once. This is available for every explainer and future explainers. However, if you pass in # find a batch size number that works for you, smaller batch will result in slower calculation times
self.explainer(agent_notes, internal_batch_size=2)[1:-1] I actually ran into this same issue myself with a demo app on streamlit that had very limited RAM I did the internal batch size trick there too and it worked really well. You are totally correct btw as to why this is happening. Gradients are calculated for I hope this helps, do let me know how you get on. |
Hi @cdpierse Charles, thank you for the swift response 👍 The In case of serving an application via conventional web frameworks (like FastAPI, Flask, etc), they don't restart the whole application over and over again, so the leaked memory is not cleared up like it happened in the Streamlit case. As a result, the application is going to run out of memory no matter how many we are ready to give it. Could be so that we are missing |
Alright, I think it also makes sense to create an issue in Captum repository: pytorch/captum#866 |
Hi @roma-glushko, Thanks for looking into this further. I think you're right regarding the issue, I ran a long-running process locally and noticed a gradual memory increase regardless of batch size. I also tried experimenting with zeroing the gradients on the model but it doesn't seem to work. Most likely it's as you say that the I'll keep trying to debug a bit on my side to see if there is a way for me to force accumulation to be dropped. It might be on Captums' side too so I'll take a look at that too and see if I can up with a PR. Will keep you posted! |
Hi @cdpierse, Thank you for keeping investigation in this task 🙏
Yeah, can confirm it did not work for me neither. Please let me know if I can help somehow with this issue. I would love to but I have already ran out of ideas how to track this issue down. |
Hi @roma-glushko was just thinking about this issue again and was wondering in your tests of you had tried doing a loop of inferences on the model itself without the explainer, trying to isolate where the leak is. Iirc Pytorch models should be stateless with each new forward pass, so it must likely be with the explainer. |
Hi @cdpierse,
Yes, I have tried to comment out the explainer part and the pytorch inference itself seemed to be stable in terms of RAM usage. So yeah, I can confirm that results of my tests pointed to the explainer code. However, it was not clear what exactly the issue in the explainer iteself nor in captum lib. |
Hi folks,
I'm experiencing some memory leaks when using the Transformers Interpret (TI) library which triggers out of memory errors and kills the model service process.
Setup
Here is how the TI lib is being using (roughly):
The code is running as a FastAPI view with one server worker:
The whole service is running on CPU/RAM, no GPU/CUDA is available.
Problem
Now when I start to send sequential requests to the service, it allocates more and more memory after each of the request. Eventually this leads to OOM errors and the server gets killed by the system.
Memory allocation roughly looks like this (these statistics I have collected on my local docker environment where I have 6 GB RAM limit):
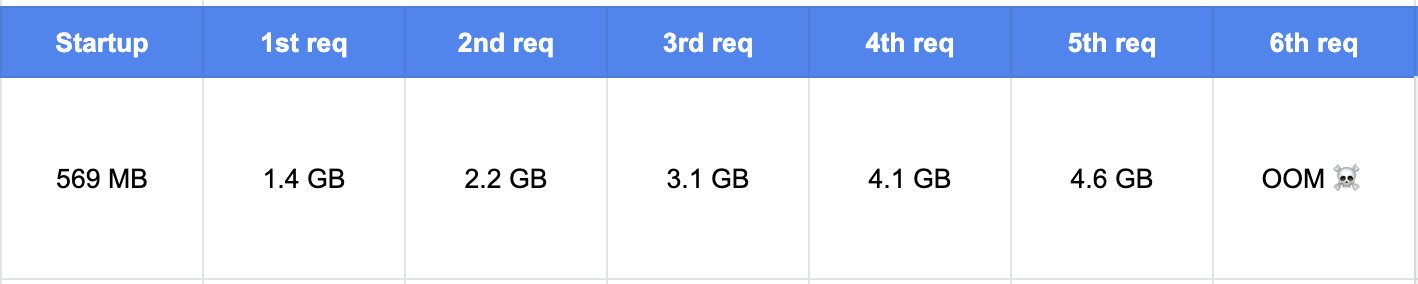
Using empirical experiments, I was able to define that problem lays in the following line:
When I disabled the line, the service used not more than 500-700MB and the memory consumption almost stayed the same.
Now from what I understand the TI library calculates gradients in order to identify word attributions. I suspect that this is the reason of the issue. However, using zero_grad() on the whole model did not help me to clean up RAM. I have tried more tricks like forcing GC collection, removing the
explainer
andmodel
instances but non of the things really helped.Do you have any ideas how could I clean up RAM that the service uses after running the Transformers Interpret library?
Appreciate your help 🙏
The text was updated successfully, but these errors were encountered: