-
Notifications
You must be signed in to change notification settings - Fork 9
New issue
Have a question about this project? Sign up for a free GitHub account to open an issue and contact its maintainers and the community.
By clicking “Sign up for GitHub”, you agree to our terms of service and privacy statement. We’ll occasionally send you account related emails.
Already on GitHub? Sign in to your account
cannot reproduce the reported results #3
Comments
Hi, thanks for your reply, resetting the lr of finetuning works, the best mean Dice of 5 folds of mine is 0.8421(0.025) , I think it's a normal bias, thanks again for your wonderful work. |
But, with only labeled dataset(100 patients), the best mean Dice(sample_k=6) is 0.7858, which has no improvement against the baseline(0.7883). |
@CaiziLee For your questions:
|
a very large gap between the reported results and mines, did I miss any important tricks?
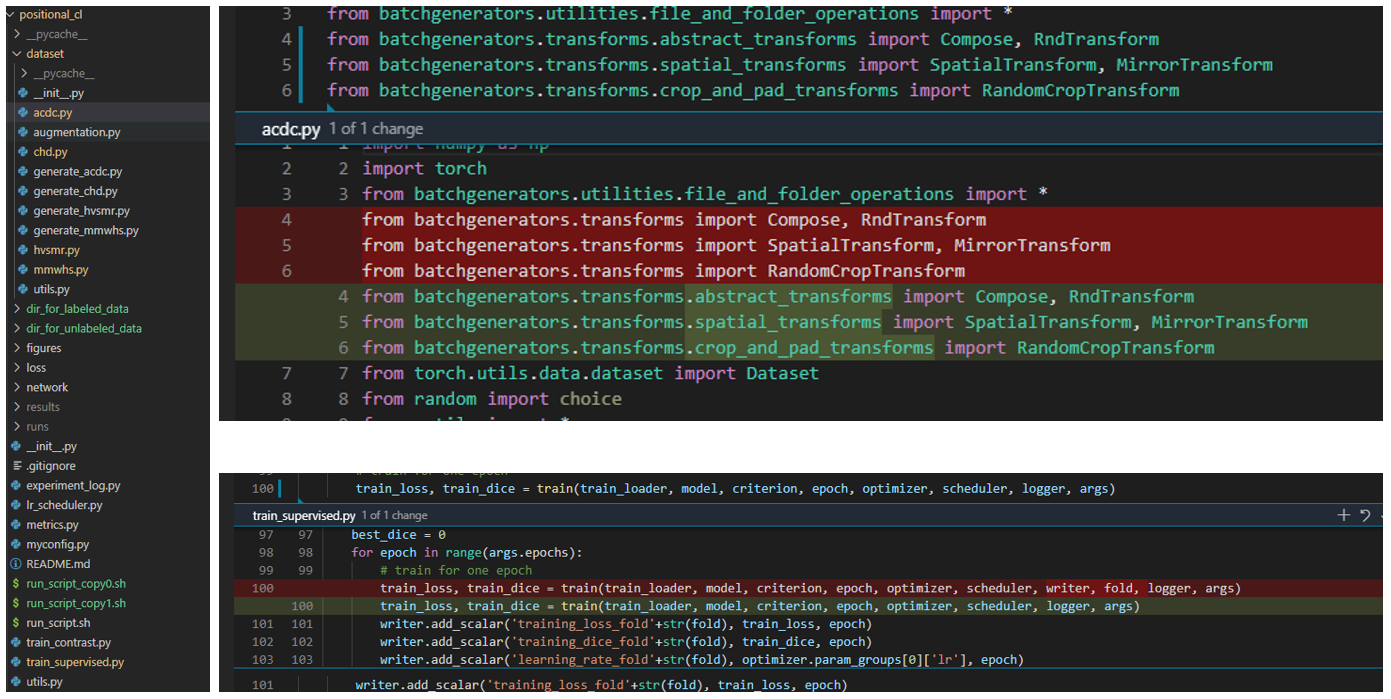
The details of my experiments are presented as follows,
1, fix two bugs:
2, prepare acdc dataset via generate_acdc.py
3, prepare running scripts:
(1) from scratch:
python train_supervised.py --device cuda:0 --batch_size 10 --epochs 200 --data_dir ./dir_for_labeled_data --lr 5e-4 --min_lr 5e-6 --dataset acdc --patch_size 352 352 --experiment_name supervised_acdc_random_sample_6_ --initial_filter_size 48 --classes 4 --enable_few_data --sampling_k 6;
(2) contrastive learning:
python train_contrast.py --device cuda:0 --batch_size 32 --epochs 300 --data_dir ./dir_for_unlabeled_data --lr 0.01 --do_contrast --dataset acdc --patch_size 352 352 --experiment_name contrast_acdc_pcl_temp01_thresh035_ --slice_threshold 0.35 --temp 0.1 --initial_filter_size 48 --classes 512 --contrastive_method pcl;
python train_contrast.py --device cuda:0 --batch_size 32 --epochs 300 --data_dir ./dir_for_unlabeled_data --lr 0.01 --do_contrast --dataset acdc --patch_size 352 352 --experiment_name contrast_acdc_gcl_temp01_thresh035_ --slice_threshold 0.35 --temp 0.1 --initial_filter_size 48 --classes 512 --contrastive_method gcl;
python train_contrast.py --device cuda:0 --batch_size 32 --epochs 300 --data_dir ./dir_for_unlabeled_data --lr 0.01 --do_contrast --dataset acdc --patch_size 352 352 --experiment_name contrast_acdc_simclr_temp01_thresh035_ --slice_threshold 0.35 --temp 0.1 --initial_filter_size 48 --classes 512 --contrastive_method simclr;
(3) finetuning:
python train_supervised.py --device cuda:0 --batch_size 10 --epochs 100 --data_dir ./dir_for_labeled_data --lr 5e-5 --min_lr 5e-6 --dataset acdc --patch_size 352 352 --experiment_name supervised_acdc_simclr_sample_6_ --initial_filter_size 48 --classes 4 --enable_few_data --sampling_k 6 --restart --pretrained_model_path ./results/contrast_acdc_simclr_temp01_thresh035_2021-12-05_09-43-38/model/latest.pth;
python train_supervised.py --device cuda:1 --batch_size 10 --epochs 100 --data_dir ./dir_for_labeled_data --lr 5e-5 --min_lr 5e-6 --dataset acdc --patch_size 352 352 --experiment_name supervised_acdc_gcl_sample_6_ --initial_filter_size 48 --classes 4 --enable_few_data --sampling_k 6 --restart --pretrained_model_path ./results/contrast_acdc_gcl_temp01_thresh035_2021-12-04_03-46-35/model/latest.pth;
python train_supervised.py --device cuda:1 --batch_size 10 --epochs 100 --data_dir ./dir_for_labeled_data --lr 5e-5 --min_lr 5e-6 --dataset acdc --patch_size 352 352 --experiment_name supervised_acdc_pcl_sample_6_ --initial_filter_size 48 --classes 4 --enable_few_data --sampling_k 6 --restart --pretrained_model_path ./results/contrast_acdc_pcl_temp01_thresh035_2021-12-02_21-48-13/model/latest.pth;
4, experimental results(ubuntu16.04, pytorch1.9, NVIDIA 2080Ti * 2, Dice metric, take sample_k=6 as an example):
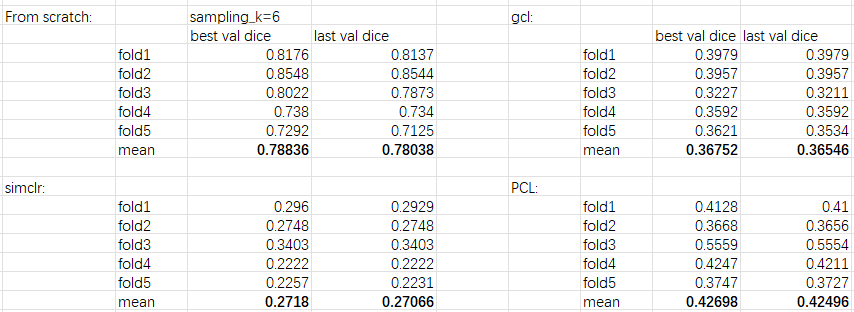
The text was updated successfully, but these errors were encountered: