New issue
Have a question about this project? Sign up for a free GitHub account to open an issue and contact its maintainers and the community.
By clicking “Sign up for GitHub”, you agree to our terms of service and privacy statement. We’ll occasionally send you account related emails.
Already on GitHub? Sign in to your account
About the ablation study #21
Comments
Thanks for your interest in our work. We think L2 Loss is simply to make the absolute distance between two positive features small, and does not consider the negative samples. So L2 Loss can be a very weak constraint compared to the contrastive loss. |
Yes, that makes sense in explaining why CL loss is good, but it still does not answer my question that why Exp.I is lower than SupOnly or ST. Does ST also use L2 loss? |
It is notable that compared to SupOnly, Exp.1 only adds an L2 Loss for unlabeled images. And the result is slightly lower than SupOnly. We think the reason may be that L2 Loss produces negative effects during training, because it may not be compatible with the CE Loss on the labeled data. ST is only based on SupOnly in the first phase. Then we produce pseudo labels for the unlabeled images based on the predictions of the SupOnly model. After that, we use the original labeled data as well as the unlabled images and their pseudo labels to re-train a model. |
Thank you for your amazing job.
I have one question about your ablation results. As in Table 3,
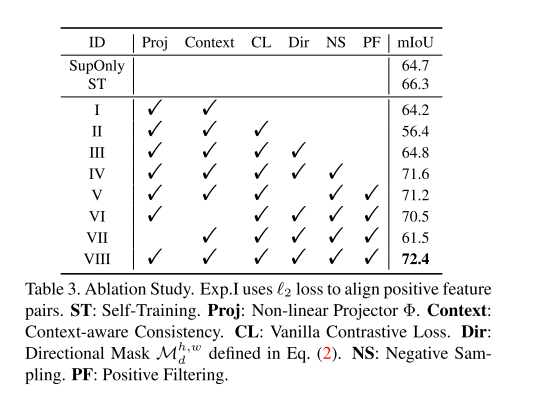
you applied Proj+Context+L2 regularization in Exp.1. However, the performance is lower than SupOnly.
Could you please give me some more explanation about this phenomenon?
The text was updated successfully, but these errors were encountered: