You signed in with another tab or window. Reload to refresh your session.You signed out in another tab or window. Reload to refresh your session.You switched accounts on another tab or window. Reload to refresh your session.Dismiss alert
Hello Yukangchen, thank you for your excellent work in the field of 3D detection and tracking. I carefully studied this repo project as soon as it was released, and I am very interested in the new backbone network VoxelNext. The network structure is concise and similar to VoxelRCNN. I tried to use VoxelNext to train my own dataset and see how it performs. My dataset is in a format similar to KITTI, with four dimensions for each point cloud frame: x, y, z, intensity, and annotation files divided into 11 classes. I have completed the related modules for the custom dataloader, and I can load the data for training normally and generate the corresponding pkl files after preprocessing. However, I encountered the following problem at the beginning of training:
assert boxes_a.shape[0] == boxes_b.shape[0]
I have checked the progress of training my own data, and the error did not occur at the beginning but when running a certain frame, where boxes_a.shape[0] is 142 and boxes_b.shape[0] is 143. This caused an error and abnormal calculation of the IOU loss. Then I considered that this might be a data issue, but it only occurred in a very small number of data. I wonder if it is possible to ignore these data and set the loss to 0 when encountering such cases, without affecting the loss calculation of other normal data.
So I modified the code to the following form:
After I made the modifications, the training was able to proceed normally. I don't know if these changes could have introduced any errors. After training a batch of data today, I obtained the trained model. However, when I tried to load the trained model for inference, I encountered a problem where the scores of the detection boxes were extremely low, less than 0.1. As a result, when I set the input score threshold for post-processing to be greater than 0.1, no detection results were outputted. I'm not sure what the reason for this is, so I was wondering if you could help me analyze it.
I will continue to follow up on your project and hope to have more discussions with you. Below are my data and model configuration files:
voxelnext_ioubranch.yaml:
`CLASS_NAMES: ['car', 'pedestrian', 'cyclist', 'tricyclist', 'bus', 'truck', 'special_vehicle', 'traffic_cone',
'small_obstacle', 'traffic_facilities', 'other']
Thanks for your interest in our work. After carefully reading your issue, I suggest that there are two choices to try.
1. Disable IoU branch
The IoU branch is just for the Waymo dataset. I think it might be useless for others.
Set IOU_BRANCH: Falsein the config file.
And remove 'iou': {'out_channels': 1, 'num_conv': 2},in the HEAD_DICT.
2. Try VoxelNeXt backbone with other head.
After reading your comment, I think what your really like is the VoxelResBackBone8xVoxelNeXt backbone network. You can use it with other detector head, like Voxel R-CNN head. What you additionally need to do is just to convert the sparse out of VoxelResBackBone8xVoxelNeXt to dense, following the common VoxelResBackBone8x.
In addition, I am very happy for more discussion via WeChat. I will contact you latter.
I have problem in training custom dataset. My dataset has only Lidar (x, y, z), and it works normally with Second, Pointpillar, PVRCNN config, but with VoxelNext It wont work. I tried and have the same errors as you. Would you guide me abit to fix it.
Hello Yukangchen, thank you for your excellent work in the field of 3D detection and tracking. I carefully studied this repo project as soon as it was released, and I am very interested in the new backbone network VoxelNext. The network structure is concise and similar to VoxelRCNN. I tried to use VoxelNext to train my own dataset and see how it performs. My dataset is in a format similar to KITTI, with four dimensions for each point cloud frame: x, y, z, intensity, and annotation files divided into 11 classes. I have completed the related modules for the custom dataloader, and I can load the data for training normally and generate the corresponding pkl files after preprocessing. However, I encountered the following problem at the beginning of training:
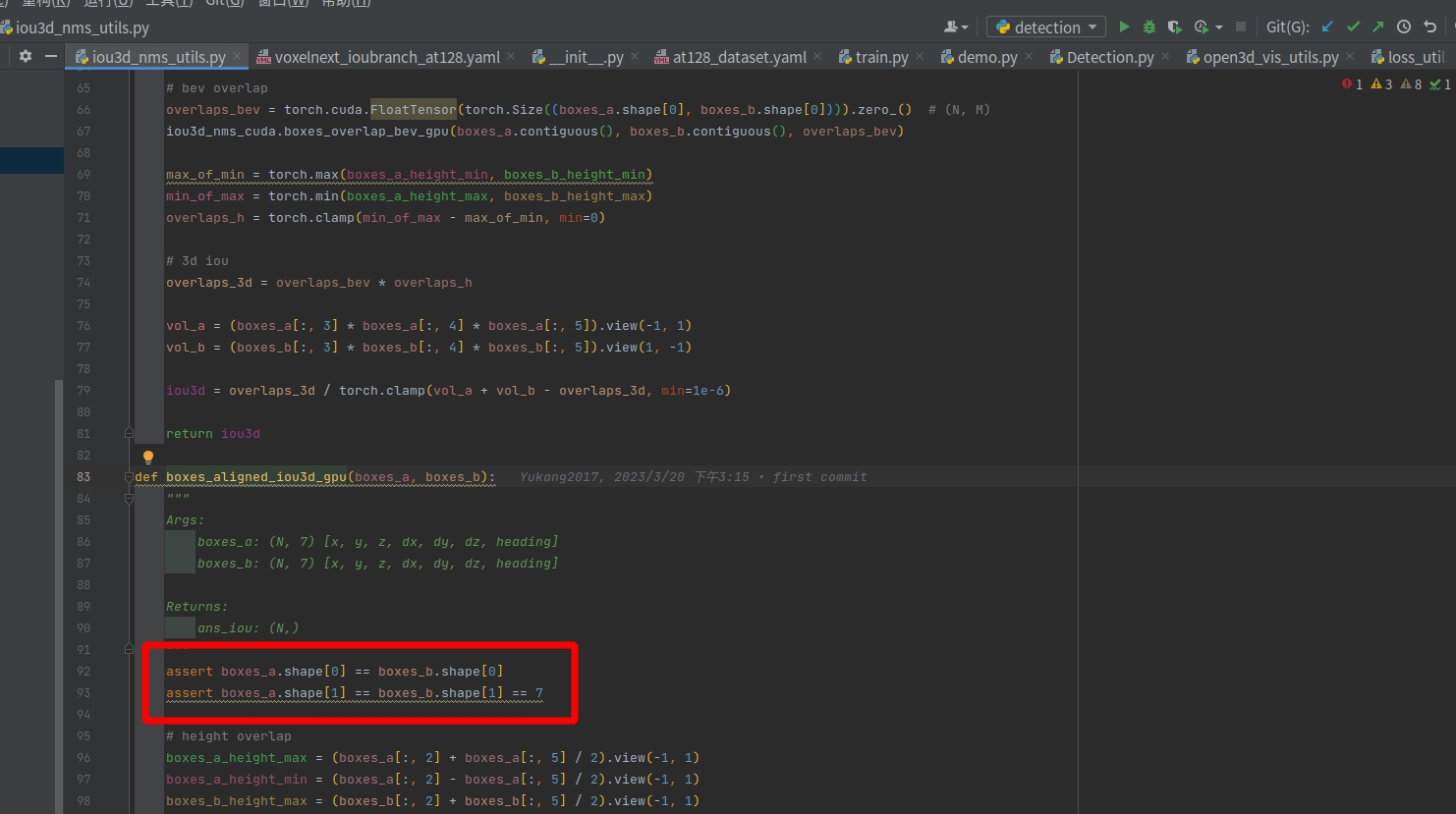
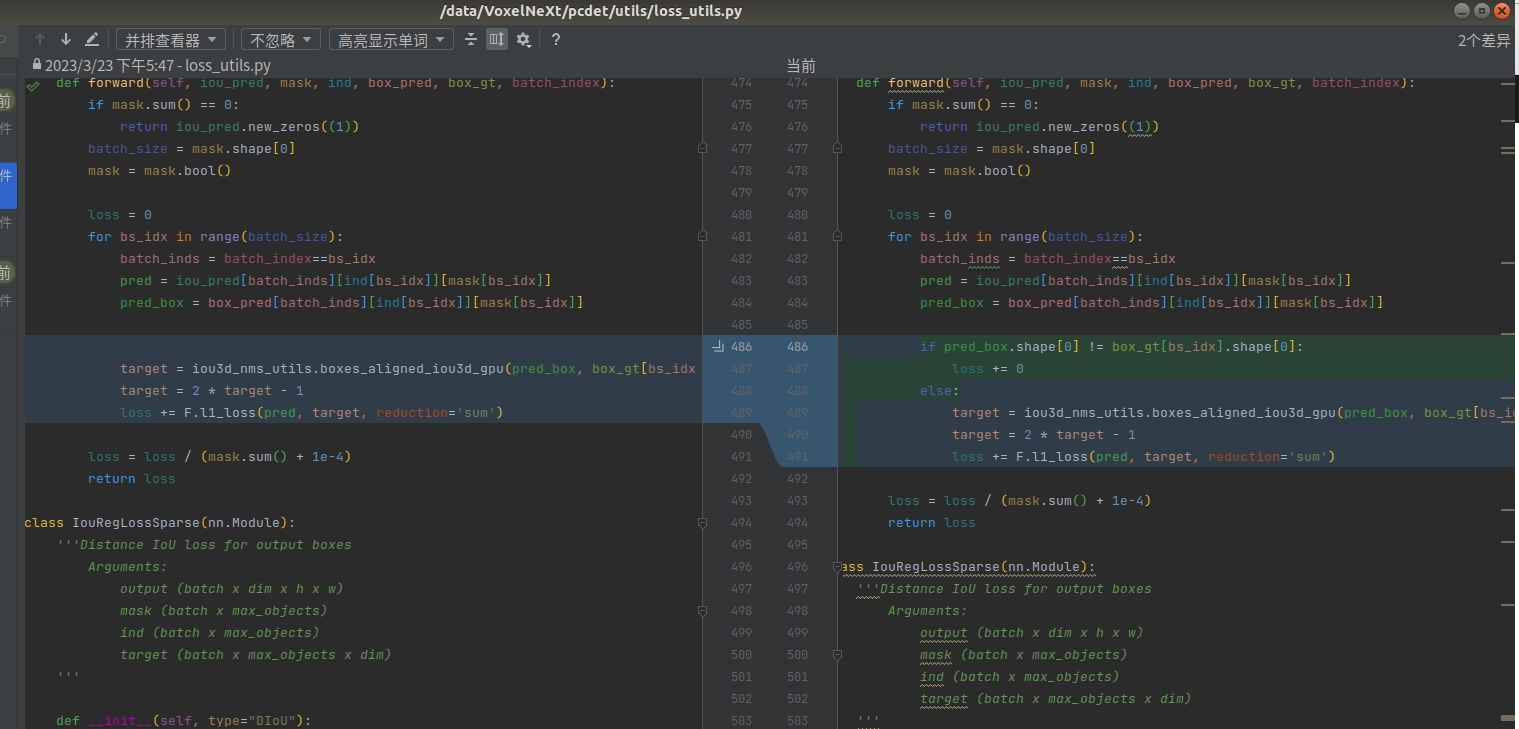
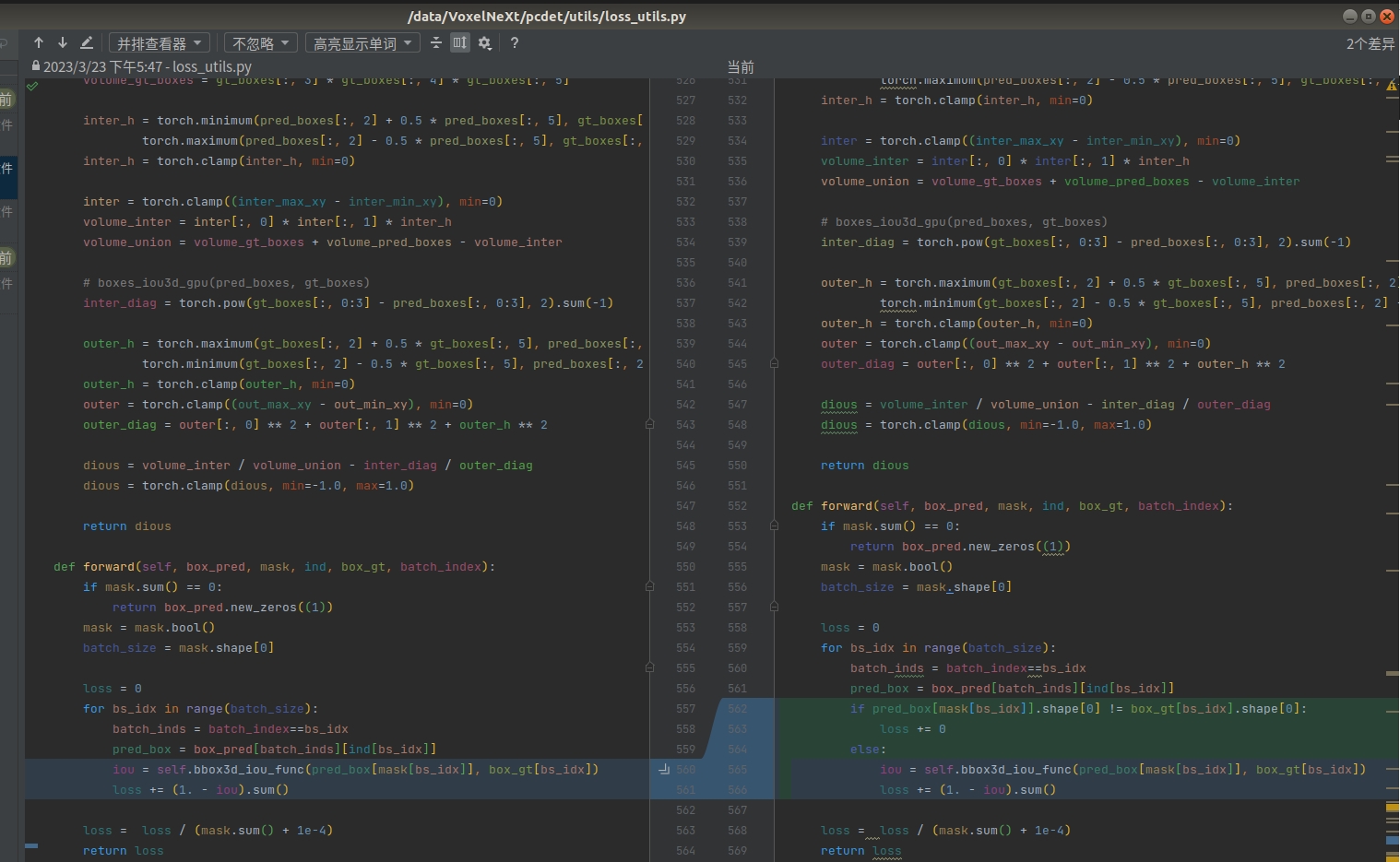
assert boxes_a.shape[0] == boxes_b.shape[0]
I have checked the progress of training my own data, and the error did not occur at the beginning but when running a certain frame, where boxes_a.shape[0] is 142 and boxes_b.shape[0] is 143. This caused an error and abnormal calculation of the IOU loss. Then I considered that this might be a data issue, but it only occurred in a very small number of data. I wonder if it is possible to ignore these data and set the loss to 0 when encountering such cases, without affecting the loss calculation of other normal data.
So I modified the code to the following form:
After I made the modifications, the training was able to proceed normally. I don't know if these changes could have introduced any errors. After training a batch of data today, I obtained the trained model. However, when I tried to load the trained model for inference, I encountered a problem where the scores of the detection boxes were extremely low, less than 0.1. As a result, when I set the input score threshold for post-processing to be greater than 0.1, no detection results were outputted. I'm not sure what the reason for this is, so I was wondering if you could help me analyze it.
I will continue to follow up on your project and hope to have more discussions with you. Below are my data and model configuration files:
voxelnext_ioubranch.yaml:
`CLASS_NAMES: ['car', 'pedestrian', 'cyclist', 'tricyclist', 'bus', 'truck', 'special_vehicle', 'traffic_cone',
'small_obstacle', 'traffic_facilities', 'other']
DATA_CONFIG:
BASE_CONFIG: cfgs/dataset_configs/at128_dataset.yaml
OUTPUT_PATH: '/lpai/output/models'
MODEL:
NAME: VoxelNeXt
NMS_THRESH: [0.8, 0.55, 0.55] #0.7
NMS_PRE_MAXSIZE: [2048, 1024, 1024] #[4096]
NMS_POST_MAXSIZE: [200, 150, 150] #500
OPTIMIZATION:
BATCH_SIZE_PER_GPU: 26
NUM_EPOCHS: 50
custom_dataset.yaml:
DATASET: 'At128Dataset'DATA_PATH: '/lpai/volumes/lpai-autopilot-root/autopilot-cloud/lidar_at128_dataset_1W/autopilot-cloud/lidar_at128_dataset_1W'
POINT_CLOUD_RANGE: [0.0, -40, -2, 150.4, 40, 4]
DATA_SPLIT: {
'train': train,
'test': val
}
INFO_PATH: {
'train': [at128_infos_train.pkl],
'test': [at128_infos_val.pkl],
}
BALANCED_RESAMPLING: True
GET_ITEM_LIST: ["points"]
DATA_AUGMENTOR:
DISABLE_AUG_LIST: ['placeholder']
AUG_CONFIG_LIST:
- NAME: gt_sampling
DB_INFO_PATH:
- at128_dbinfos_train.pkl
USE_SHARED_MEMORY: False
PREPARE: {
filter_by_min_points: ['car:12', 'pedestrian:8', 'cyclist:10', 'tricyclist:15', 'bus:40', 'truck:30', 'special_vehicle:35',
'traffic_cone:10', 'small_obstacle:7', 'traffic_facilities:11', 'other:16']
}
POINT_FEATURE_ENCODING: {
encoding_type: absolute_coordinates_encoding,
used_feature_list: ['x', 'y', 'z', 'intensity'],
src_feature_list: ['x', 'y', 'z', 'intensity'],
}
DATA_PROCESSOR:
- NAME: mask_points_and_boxes_outside_range
REMOVE_OUTSIDE_BOXES: True
If possible, feel free to add me on WeChat or contact me through email. Let's discuss together.
Wechat: lyb543918165
Email: lybluoxiao@163.com
The text was updated successfully, but these errors were encountered: