-
Notifications
You must be signed in to change notification settings - Fork 231
New issue
Have a question about this project? Sign up for a free GitHub account to open an issue and contact its maintainers and the community.
By clicking “Sign up for GitHub”, you agree to our terms of service and privacy statement. We’ll occasionally send you account related emails.
Already on GitHub? Sign in to your account
Different transforms applied to CT and label #1071
Comments
note that in the code you apply other augmentation on scaled_subject but not on can_spac_subsject |
Thank you for the suggestion. I tried applying the transforms I found out that the issue comes with I then checked the spacing of the CT and the segmentation mask and I got the following: So could it be that the reason could be that they have different spacings? And if I try to give them both the same spacing using Any idea on how to solve this? Otherwise I will see what spatial transformation cause this issue and which don't and that's it. |
ok difficult to figure out, without an example, (if you can share on volume) it is weird to have the exact same matrix size but a very different voxel size .. : testing the superposition with different voxel size, may be handing, and results may depend of the used viewer in python I am not sure but it may display the matrix (without taking into acount the voxel size for instance) With mrview (viewer from mrtrix,) or Slicer you will get more correct comparison If the two are well alligned in Slicer (or mrview) then I would test to use but I suspect that the affine (and thus the voxel size) of the segmentation is not correct and it should be the same as the CT volume ... (was the segmentation not defined from the CT scan ??? if yes, then it should have the exact same voxel size (and affine) ) in this case you should use to |
Hi, @simonebonato. As @romainVala suggested, you can use copy_metadata = tio.CopyAffine('CT') However, I just tried that and the transform did nothing in my example! I'll debug this and come back to you. |
For visibility, this is somehow not working! import torch
import torchio as tio
ct = tio.ScalarImage('/tmp/upload_folder/case_01_IMG_CT.nrrd')
seg = tio.LabelMap('/tmp/upload_folder/case_01_segmentation.nrrd')
seg.affine = ct.affine
print(seg.affine)
|
Update: |
Ok, this happens because the affine is not being read correctly as the segmentation hasn't been loaded. That will be addressed. torchio/src/torchio/data/image.py Lines 255 to 260 in 0933def
Back to this issue. |
import torch
import torchio as tio
ct = tio.ScalarImage('/tmp/upload_folder/case_01_IMG_CT.nrrd')
seg = tio.LabelMap('/tmp/upload_folder/case_01_segmentation.nrrd')
seg.data; # just to temporarily overcome the bug we mentioned
subject = tio.Subject(ct=ct, seg=seg)
copy_metadata = tio.CopyAffine('ct')
fixed = copy_metadata(subject)
fixed.seg.save('/tmp/upload_folder/case_01_segmentation_fixed.nrrd') The image seems aligned after copying the affine. All looking good after applying transform = tio.RandomAffine()
torch.manual_seed(0)
transformed = transform(fixed)
transformed.ct.save('/tmp/upload_folder/case_01_IMG_CT_transformed.nrrd')
transformed.seg.save('/tmp/upload_folder/case_01_segmentation_fixed_transformed.nrrd') |
This has been fixed in |
oh I missed the link of the data I think this is a typical errors which is not easy to understand when one is not use to the 3D affine compare taking into account the affine (and thus the voxel size) is important |
Thank you both @fepegar and @romainVala for solving my problem. And thanks @romainVala I did what you told me and seen the difference.
Next time I will check directly the spacing first before making any operation. |
Is there an existing issue for this?
Problem summary
I have a CT scan and the corresponding label mask and I want to perform some augmentations on them.
Initially they have a different spacing but after using ToCanonical and Resample(1.0) they become the same; however when I perform some other augmentations and I make a plot where I overlay the label on the image (the same slice ofc), they are not overlapping and the label goes partially on the background.
Code for reproduction
Actual outcome
When I plot the label on the CT, this is the result:
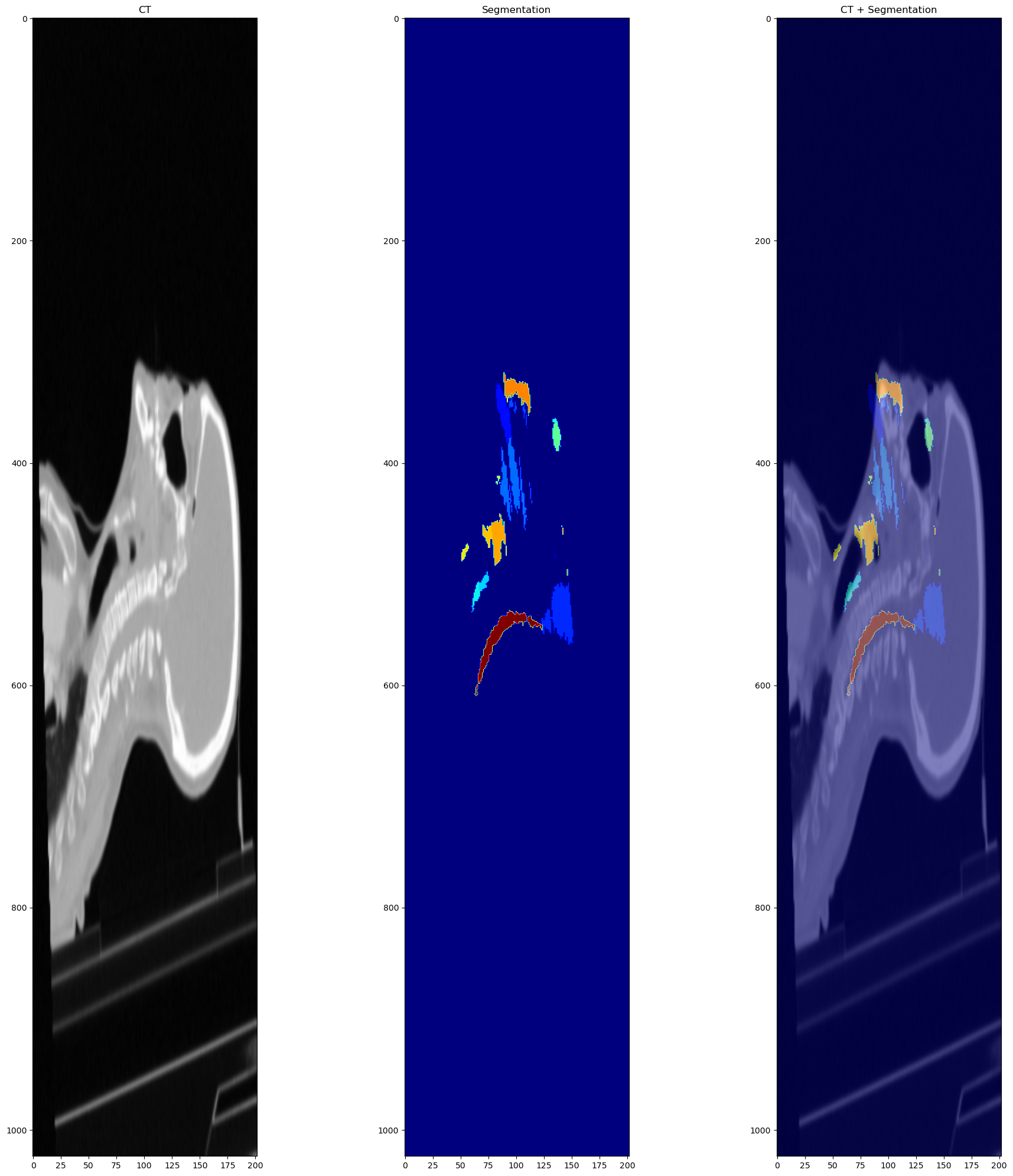
While before the transforms it looks like this:
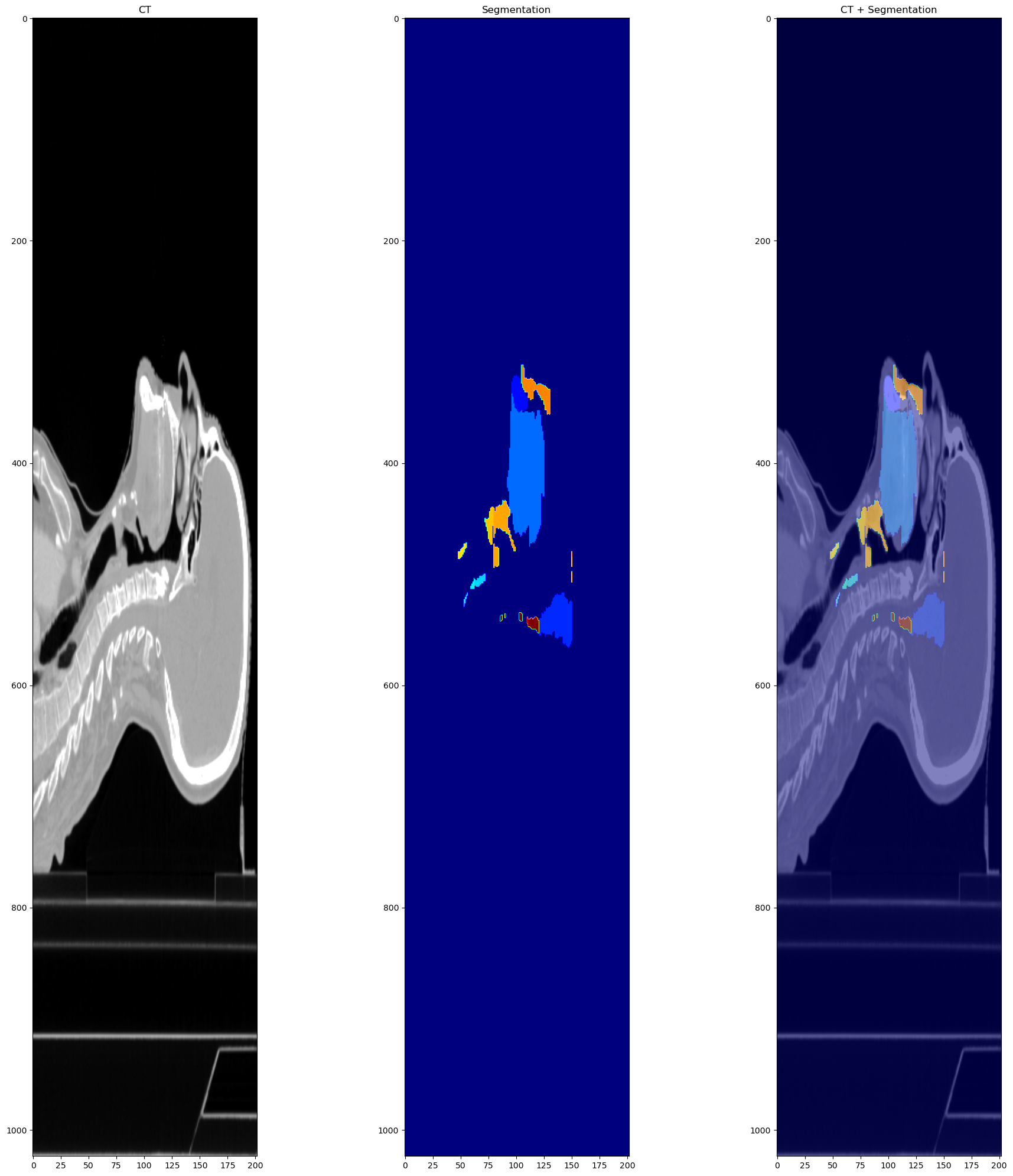
The image and the segmentation are the following.
And the slice I printed is CT[500, :, :], same for the label.
Error messages
No response
Expected outcome
What I would like to obtain is the label being on top of the CT, and not shifted.
System info
No response
The text was updated successfully, but these errors were encountered: