You signed in with another tab or window. Reload to refresh your session.You signed out in another tab or window. Reload to refresh your session.You switched accounts on another tab or window. Reload to refresh your session.Dismiss alert
I am not sure if this is a bug, but just my lack of understanding. However, I have fine-tuned a model (EleutherAI/pythia-1b) to generate Cypher statement. In the studio chat, it all looks fantastic:
I have uploaded the weight to HF, and tried to replicate the text generation with transformers:
from transformers import AutoTokenizer, AutoModelForCausalLM
from transformers import GPTNeoXForCausalLM
device = "cuda:0"
tokenizer = AutoTokenizer.from_pretrained("tomasonjo/movie-generator-small")
model = GPTNeoXForCausalLM.from_pretrained("tomasonjo/movie-generator-small").to(device)
inputs = tokenizer("Create a Cypher statement to answer the following question:What movies did Tom Hanks star in?", return_tensors="pt").to(device)
tokens = model.generate(**inputs, max_new_tokens=256).to(device)
tokenizer.decode(tokens[0])
I get the following result:
Create a Cypher statement to answer the following question:What movies did Tom Hanks star in?Create a movieCreate a movieCreate a movieSend this message to:Create a movieCreate a movieSend this message to:Create a movieCreate a movieCreate a movieCreate a movieCreate a movieCreate a movieCreate a movieCreate a movieCreate a movieCreate a movieCreate a movieCreate a movieCreate a movieCreate a movieCreate a movieCreate a movieCreate a movieCreate a movieCreate a movieCreate a movieCreate a movieCreate a movieCreate a movieCreate a movieCreate a movieCreate a movieCreate a movieCreate a movieCreate a movieCreate a movieCreate a movieCreate a movieCreate a movieCreate a movieCreate a movieCreate a movieCreate a movieCreate a movieCreate a movieCreate a movieCreate a movieCreate a movieCreate a movieCreate a movieCreate a movieCreate a movieCreate a movieCreate a movieCreate a movieCreate a movieCreate a movieCreate a movieCreate a movieCreate a movieCreate
What am I missing?
To Reproduce
Fine-tune a model: EleutherAI/pythia-1b
Push the model to HF
Run model in transformers
I've tried both AutoModelForCausalLM and GPTNeoXForCausalLM
I can share the training data if needed, and have also made the HF model public
The text was updated successfully, but these errors were encountered:
The reason is that you need to align the prompting.
With default setting, we are adding an EOS token at the end of the prompt. So you would need to call it like that:
from transformers import AutoModelForCausalLM, AutoTokenizer
tokenizer = AutoTokenizer.from_pretrained("tomasonjo/movie-generator-small")
model = AutoModelForCausalLM.from_pretrained("tomasonjo/movie-generator-small")
model.half().cuda()
# need to match the input prompt how you are doing it in the LLM Studio Prompt
inputs = tokenizer("\nCreate a Cypher statement to answer the following question:What movies did Tom Hanks star in?<|endoftext|>", return_tensors="pt", add_special_tokens=False).to("cuda")
tokens = model.generate(
**inputs,
max_new_tokens=256,
temperature=0.3,
repetition_penalty=1.2,
num_beams=4
)[0]
tokens = tokens[inputs["input_ids"].shape[1]:]
print(tokenizer.decode(tokens, skip_special_tokens=True))
Which outputs MATCH (d:Person {name: 'Tom Hanks'})-[:ACTED_IN]->(m:Movie) RETURN {movie: m.title} AS result
An additional newline at the start also usually works well and I added it above, you can play with the prompt and the inference settings a bit.
We have an open issue to generate an automatic model card on HF to exactly describe how a prompt needs to look like based on the settings of the experiment: #5
馃悰 Bug
I am not sure if this is a bug, but just my lack of understanding. However, I have fine-tuned a model (EleutherAI/pythia-1b) to generate Cypher statement. In the studio chat, it all looks fantastic:
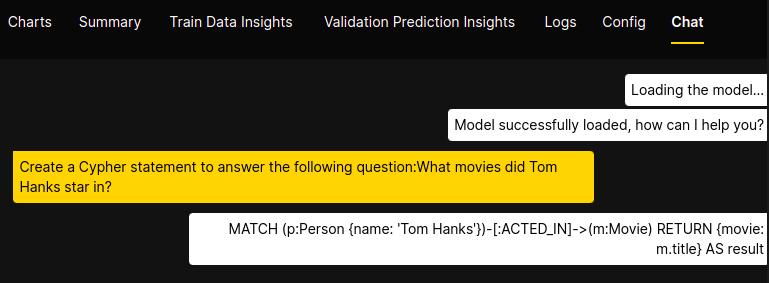
I have uploaded the weight to HF, and tried to replicate the text generation with transformers:
I get the following result:
Create a Cypher statement to answer the following question:What movies did Tom Hanks star in?Create a movieCreate a movieCreate a movieSend this message to:Create a movieCreate a movieSend this message to:Create a movieCreate a movieCreate a movieCreate a movieCreate a movieCreate a movieCreate a movieCreate a movieCreate a movieCreate a movieCreate a movieCreate a movieCreate a movieCreate a movieCreate a movieCreate a movieCreate a movieCreate a movieCreate a movieCreate a movieCreate a movieCreate a movieCreate a movieCreate a movieCreate a movieCreate a movieCreate a movieCreate a movieCreate a movieCreate a movieCreate a movieCreate a movieCreate a movieCreate a movieCreate a movieCreate a movieCreate a movieCreate a movieCreate a movieCreate a movieCreate a movieCreate a movieCreate a movieCreate a movieCreate a movieCreate a movieCreate a movieCreate a movieCreate a movieCreate a movieCreate a movieCreate a movieCreate a movieCreate a movieCreate
What am I missing?
To Reproduce
I've tried both
AutoModelForCausalLM
andGPTNeoXForCausalLM
I can share the training data if needed, and have also made the HF model public
The text was updated successfully, but these errors were encountered: