-
Notifications
You must be signed in to change notification settings - Fork 12
New issue
Have a question about this project? Sign up for a free GitHub account to open an issue and contact its maintainers and the community.
By clicking “Sign up for GitHub”, you agree to our terms of service and privacy statement. We’ll occasionally send you account related emails.
Already on GitHub? Sign in to your account
Problems on Running #4
Comments
@hkcao it seems that you enabled one attacker with the constant attack. Can you please try to set |
Thanks for your kindly reply. It seems like that the number of failing worker should be larger than 0. However, I tried the code again with 6 workers, and this time the loss is getting smaller suddenly after 230-th iteration (from more than 10000 to smaller than 10, and then get stuck at 2.3 for several hundreds of iterations). Is it the influence of SGD? Because I also tried some other values of workers and batch_size, and sometimes the loss can get smaller than 2 after some iterations and has the trends to converge. |
@hkcao I realized that you are using the cyclic coding scheme. We did observe that the cyclic coding scheme may suffer from numeric issues from settings to settings. Can you try the repetition coding scheme instead (by setting |
Thanks for your reply. I tried repetition scheme with the same parameters as before, and the loss is indeed getting smaller. According to my understanding , the numeric issues should only happens when the dimension of encoding matrix grows large. While the test I used has only 6 workers, which should not be an issue ? |
@hkcao you are right about the numerical issue. We also found when the dimension of the model becomes high, it also needs more precision for encoding+decoding. For the current version, we reduced the precision to attain better communication efficiency. You may want to try switching back to this commit for better precision (please also see what I changed there): 582616d. |
Thanks for your reply. It works ! :-) when I modified these changes back as you mention in that commit, the loss is indeed getting smaller, and seems can converge faster than previous version. I also tried some larger values like 40 workers, and it can also work now. It seems like the precision you mentioned is indeed important for the coding scheme. |
Glad to help @hkcao! Then we can probably close this issue for now and reopen it if you feel needed? |
Thanks again for the help! |
Hi, @hkcao can you double check if the model checkpoints are saved by the PS or workers? It seems master also tries to save checkpoints: https://github.com/hwang595/Draco/blob/master/src/master/rep_master.py#L136-L137. And we should not use the checkpoints from the master to evaluate as it does not contain any running statistics in the BN layers. |
Thanks for your reply. I was using the checkpoints saved by the master before. When the models saved by worker 1 are used, the loss and accuracy come to the same values as the result of Thanks again for the help! |
Hi, I was recently running your code. When I use 6 workers, and the batch size is set 180, i.e., each workers computes 30 points, the parameters are as following. However, the loss I get is somehow very large even after 5000 iterations (more than 10000). When uncoded scheme used, however, the loss is around 3. Is there any place that I did wrong?
the parameters are as follows:
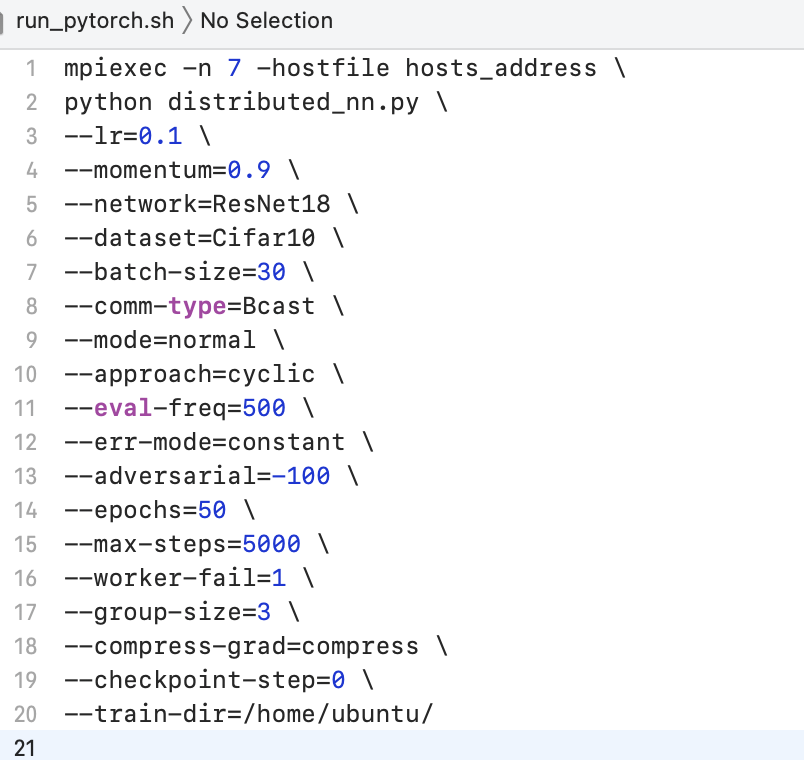
The text was updated successfully, but these errors were encountered: