You signed in with another tab or window. Reload to refresh your session.You signed out in another tab or window. Reload to refresh your session.You switched accounts on another tab or window. Reload to refresh your session.Dismiss alert
I have some time series forecasts of weekly data where forecasts are too smooth or not really pronounced (not sure how to describe it). Another time series however, for the same dates, same general value range, same model parameters, shows "correct" variability. Both datasets show the same warnings when run (generally about daily event timestamps due to weekly data and a warning that data is highly fragmented)
In another dataset (I have not currently access to), removing the last entry from the time-series fixed the variability. This does however not work for the dataset1 below.
Is this a bug or intended behaviour? If this is intended, which parameters would I have to tweak to mitigate this? Thx for your help
Dataset1: relatively smooth forcast (False)
Dataset2: correct variability forecast (True)
I am using the following settings for the model (Greykite v.0.4.0):
After some testing I assume this behaviour derives from the fitting algorithm. The default "ridge" method uses the following alphas by default np.logspace(-5, 5, 30):
So my guess is that some models/ts just do not fit very well, so that the ridge regression (probably during its internal cross validation) prefers a high alpha (~100 in the example case) that only fits the long term trend, bit not seasonality or other regressors.
By simply switching to 'linear' or 'ridge' with "fit_algorithm_params":{'alphas':np.logspace(-5, 1, 30) it "solves" the problem. I will check if these lower alphas work generally well for my use-cases or if I only use them once these smooth predictions arise.
So I guess this is not a bug, but also a bit hard to debug as it happens more or less at random for my time-series.
I have some time series forecasts of weekly data where forecasts are too smooth or not really pronounced (not sure how to describe it). Another time series however, for the same dates, same general value range, same model parameters, shows "correct" variability. Both datasets show the same warnings when run (generally about daily event timestamps due to weekly data and a warning that data is highly fragmented)
In another dataset (I have not currently access to), removing the last entry from the time-series fixed the variability. This does however not work for the dataset1 below.
Is this a bug or intended behaviour? If this is intended, which parameters would I have to tweak to mitigate this? Thx for your help
Dataset1: relatively smooth forcast (False)
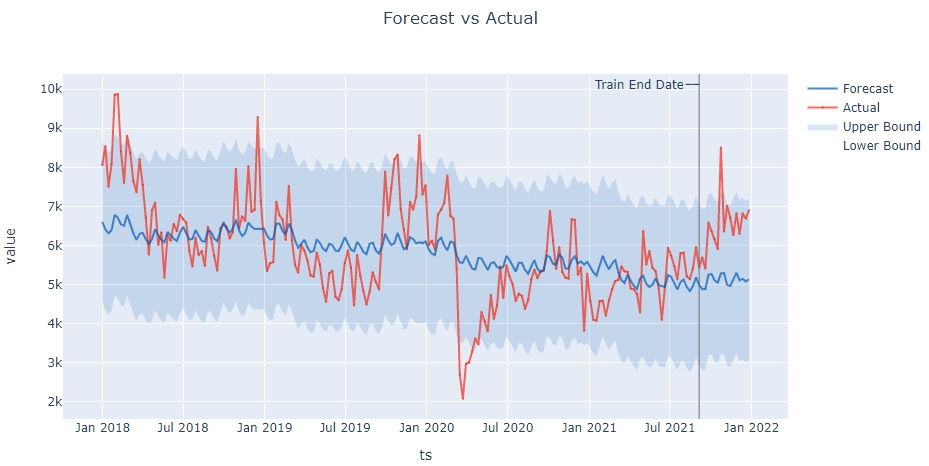
Dataset2: correct variability forecast (True)
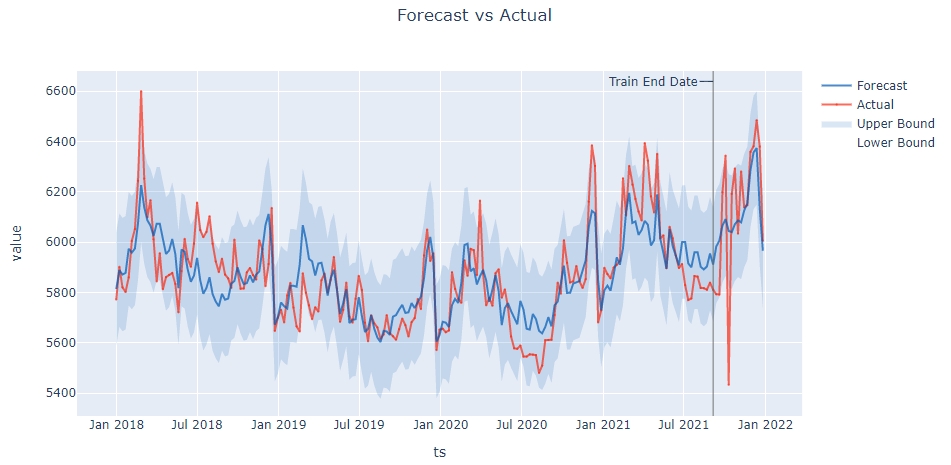
I am using the following settings for the model (Greykite v.0.4.0):
DataSet1 (False):
Dataset2 (True):
The text was updated successfully, but these errors were encountered: