New issue
Have a question about this project? Sign up for a free GitHub account to open an issue and contact its maintainers and the community.
By clicking “Sign up for GitHub”, you agree to our terms of service and privacy statement. We’ll occasionally send you account related emails.
Already on GitHub? Sign in to your account
AssertionError: Assigned values have not the same length than fitness weights #35
Comments
Hi @RNarayan73, sorry for the late response. Could you kindly provide a minimal example of code and data that reproduces the problem? |
@manuel-calzolari , here you go!
Sometimes, the grid_search runs through to completion. But if you repeat it, it will throw up the error! If you try replacing GeneticSelectionCV in the 'dim' step of the pipeline with any other, there is no error. Hope this helps. Regards |
Hi @manuel-calzolari |
Sorry, I haven't had time to look at it yet |
Hi @RNarayan73, I cannot reproduce the issue at the moment. I've modified your code in this way so that I can test it more quickly:
But even running it several times I do not get any error. |
I shall try to reproduce, but since it has been a while, things have changed with my environment resulting in issue #42 |
@manuel-calzolari looks like this is resolved! |
Hi All,
Has anyone come across the issue described below? I'd appreciate any direction to help resolve this.
System information
OS Platform and Distribution: Windows 11 Home
Sklearn-genetic version: 0.5.1
deap version: 1.3.3
Scikit-learn version: 1.1.2
Python version: 3.8.13
Describe the bug
When running my pipeline to tune hyperparameters, this error occurs intermittently.
I'm running TuneSearchCV (package tune-sklearn) to tune various hyperparameters of my pipeline below in this example, but have also encountered the error frequently when using GASearchCV (package sklearn-genetic-opt, also based on deap) :
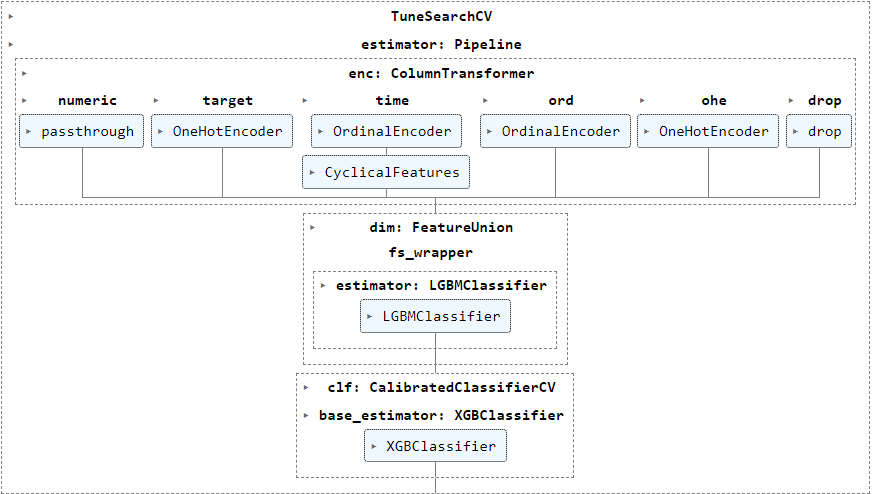
The following param_grid (generated using BayesSearchCV to show real information instead of the objects) show categorical values for various transformers steps enc__numeric, enc__target, enc__time__cyclicity and dim__fs_wrapper besides numerical parameter ranges for clf__base_estimator.
To Reproduce
Steps to reproduce the behavior:
<<< Please let me know if you would like more information to reproduce the error >>>
Expected behavior
On occasions when it ran successfully, I got the following results for best_params_ as expected:
However, when I exclude dim__fs_wrapper from the pipeline, the error does not occur at all. The purpose of this transformer is to select a feature selection method from amongst 'passthrough' and estimators wrapped in SelectFromModel, RFECV and GeneticSelectionCV and the error seems to originate when GeneticSelectionCV is used for feature selection.
Additional context
The text was updated successfully, but these errors were encountered: