-
Notifications
You must be signed in to change notification settings - Fork 115
New issue
Have a question about this project? Sign up for a free GitHub account to open an issue and contact its maintainers and the community.
By clicking “Sign up for GitHub”, you agree to our terms of service and privacy statement. We’ll occasionally send you account related emails.
Already on GitHub? Sign in to your account
paper #4
Comments
Sorry, I see what you mean. |
Thanks for your interest. Yes. Uniform Matching has a big effect on objects across various scales when we apply it to the baseline (the first line). It makes the model be fully trained as all ground truths participate in the training phase. However, its effect on large objects diminishes when we add Dilated Encoder. Thus, we may think that Uniform Matching improves the performance of small and medium objects largely while Dilated Encoder dominates large objects' performance. |
thanks |
hello, the effect of large objects is a 9.3% difference, which is not small compared to others
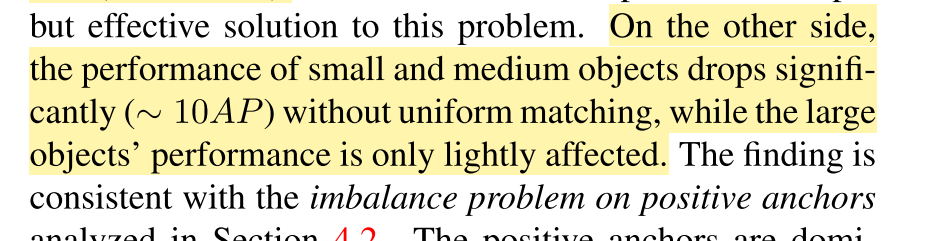
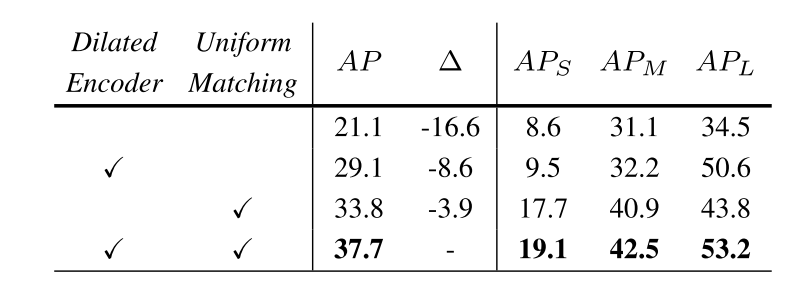
The text was updated successfully, but these errors were encountered: