-
Notifications
You must be signed in to change notification settings - Fork 10
New issue
Have a question about this project? Sign up for a free GitHub account to open an issue and contact its maintainers and the community.
By clicking “Sign up for GitHub”, you agree to our terms of service and privacy statement. We鈥檒l occasionally send you account related emails.
Already on GitHub? Sign in to your account
Can we have shap value in the log-odds for binary target? #24
Comments
Actually, the SHAP values returned by XGBoost or LightGBM are on the logit link scale. library(shapviz)
library(xgboost)
X <- iris[, -1]
# Binary logistic regression with XGBoost
fit <- xgb.train(
params = list(objective = "binary:logistic"),
data = xgb.DMatrix(data.matrix(X), label = iris[, 1] >= 5.8),
nrounds = 30
)
# On logit scale
shp <- shapviz(fit, X_pred = data.matrix(X), X = X)
sv_waterfall(shp, row_id = 66) If you rather mean to switch to probabilities, I don't think it is possible without violating at least some of the Shapley fairness axioms (linearity). Still, I think we could add a utility function that would map the "shapviz" object approximately from logit to probability space, using the approach in the blog post you provided. |
The values in the plot above are log-odds and they are on the logit scale. So I think you are interested in transforming them (somehow) via logistic functio (= inverse logit) to propabilities without badly violating SHAP properties. I will look into that in the next time! |
I looked into the matter. According to the proposed transformation, a jump from 0.5 to 0.59 would be as large as a jump from 0.9 to 0.99 on the probability scale. I currently don't see a situation where this makes sense. Thus I wont add this transformation to "shapviz". If you still need it, simply do the transformation based on
|
Thank you for writing this specical transform funtion for me. However, it gives me a error "Error in g(b, S) : could not find function "g"". Can you help with this? |
I leave this as an exercise to you. Based on your link, it is just 2-3 lines of code. But as I said, I can't think of a situation where the proposed mapping makes much sense. On the contrary, I think it is misleading. |
Thank you! I ran the python codes to get an idea of what shap output array and baseline prediction looks like. I tried a few different codes and results turn out differntly. I will spend more time looking at it. Appreciate your help! |
@mayer79 I know the issue is closed, but I made a logit to binary linking function some time ago. I still understand that you don't want to add it in though :< |
As far as I remember, any non-linear transform will violate the fairness axioms of Shapley, so I'd like to keep it outside the package. Since the "shapviz" object contains the baseline b and all SHAP values S, you could write a blogpost or similar for those interested? |
I stumbled into this thread, searching for a way to display a shapviz waterfall with probabilities. @madprogramer ... it would be nice if you shared your solution. After having spent a good hour on my end, I'm sharing the completed function that was hinted last year, as it might help others in the future.
|
@actuarial-lonewolf : Thanks for this input! Comparing with Kernel SHAP (once on link scale and once on probability scale):
|
A lot of outcomes are binary. Is it poissble to have shap value in the log-odds in force plot or waterfall plot?
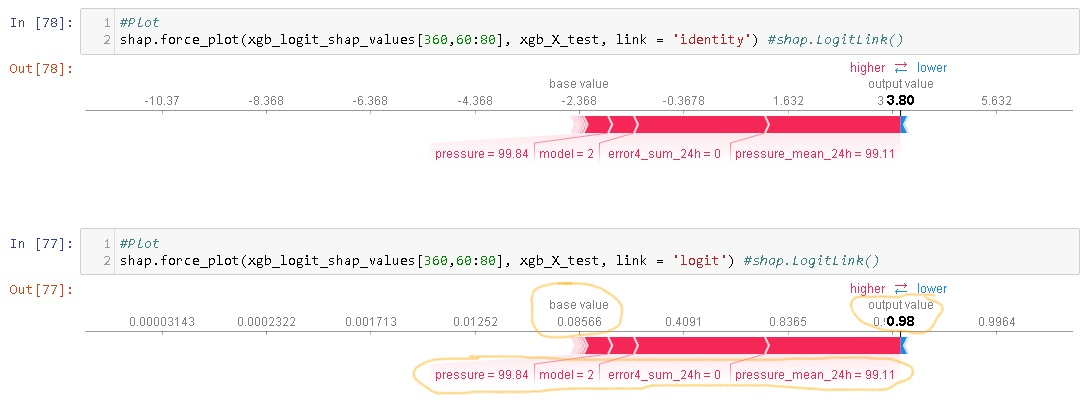
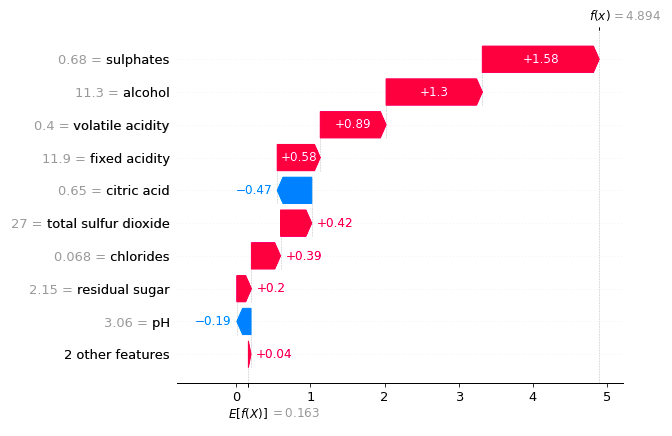
Example is :
discussion link 馃憤
shap/shap#29
(3) Binary Target section in below link:
https://medium.com/dataman-in-ai/the-shap-with-more-elegant-charts-bc3e73fa1c0c
The text was updated successfully, but these errors were encountered: