-
Notifications
You must be signed in to change notification settings - Fork 3.2k
New issue
Have a question about this project? Sign up for a free GitHub account to open an issue and contact its maintainers and the community.
By clicking “Sign up for GitHub”, you agree to our terms of service and privacy statement. We’ll occasionally send you account related emails.
Already on GitHub? Sign in to your account
DeepExplainer: Strange results using loaded model #945
Comments
Does your reloaded model have the exact same outputs as the previous model? It looks like the weights are scrambled. |
I am not a neural network expert. But the summary of both models (reloaded or not) look identical: Layer (type) Output Shape Param #conv2d_1 (Conv2D) (None, 26, 26, 32) 320 activation_1 (Activation) (None, 26, 26, 32) 0 conv2d_2 (Conv2D) (None, 24, 24, 32) 9248 activation_2 (Activation) (None, 24, 24, 32) 0 max_pooling2d_1 (MaxPooling2 (None, 12, 12, 32) 0 dropout_1 (Dropout) (None, 12, 12, 32) 0 conv2d_3 (Conv2D) (None, 10, 10, 64) 18496 activation_3 (Activation) (None, 10, 10, 64) 0 conv2d_4 (Conv2D) (None, 8, 8, 64) 36928 activation_4 (Activation) (None, 8, 8, 64) 0 max_pooling2d_2 (MaxPooling2 (None, 4, 4, 64) 0 dropout_2 (Dropout) (None, 4, 4, 64) 0 flatten_1 (Flatten) (None, 1024) 0 dense_1 (Dense) (None, 128) 131200 activation_5 (Activation) (None, 128) 0 dropout_3 (Dropout) (None, 128) 0 dense_2 (Dense) (None, 10) 1290 activation_6 (Activation) (None, 10) 0Total params: 197,482 For the determination of an example output I use a defined test-image. The output of the last layer of the "freshly" (=not reloaded) trained model is: Does this help to find the reason for the "strange" result? |
I have to excuse myself. I have found my "beginners" mistake. Here the wrong code in my model training script: . . . Putting the line "model.save(model_name)" after the line "cnn = model.fit ...." solved everything. |
I have reproduced the MNIST handwriting DeepExplainer results using my own model architecture. Producing a SHAP image_plot "directly" after the model training gave me the expected result ("result_shap_mnist_direct.png"). The model was saved into a file ("test_mnist.h5").
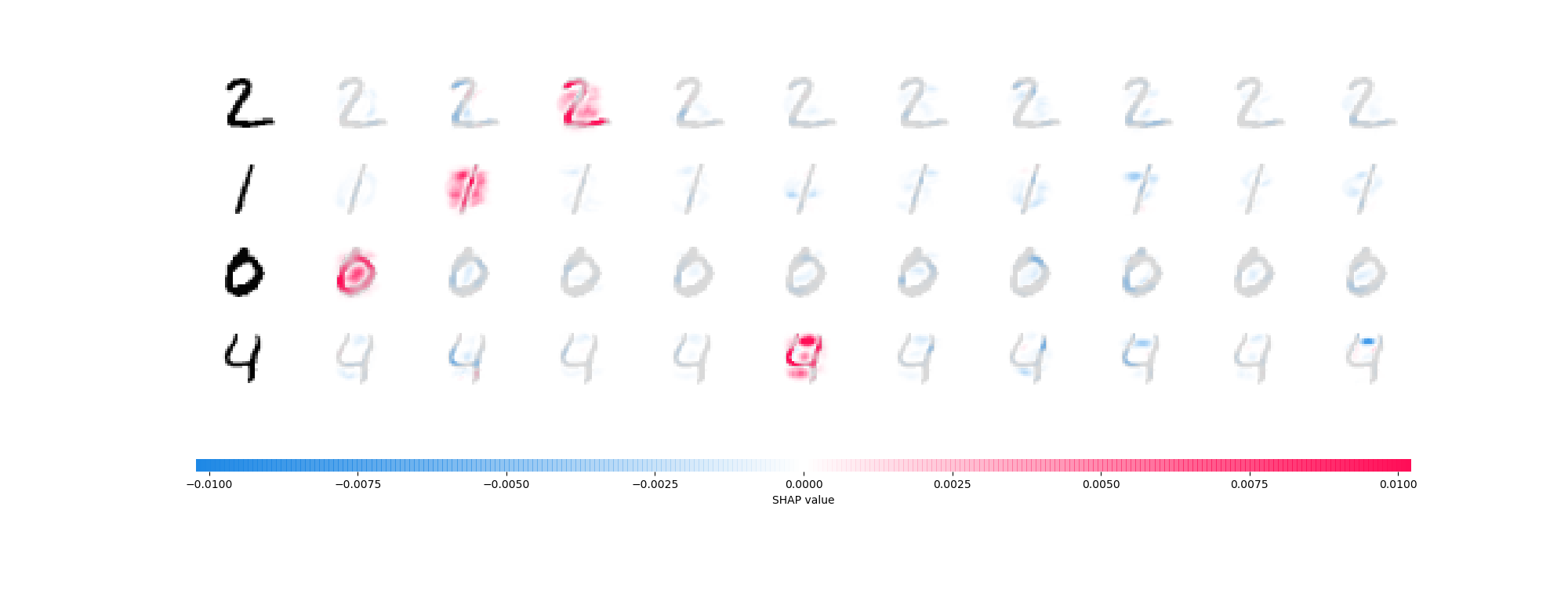
Resulting image_plot:
Using a extra script where I load this model (and the MNIST image data) I get a "strange" result in the SHAP image_plot.
Is the saved model missing something SHAP is needing?
Code:
from keras.models import load_model
import shap
model = load_model(model_name)
background = x_train[np.random.choice(x_train.shape[0], 100, replace=False)]
e = shap.DeepExplainer(model, background)
shap_values = e.shap_values(x_test[1:5])
shap.image_plot(shap_values, -x_test[1:5])
plt.savefig('result_shap_mnist_load_model.png')
Resulting image_plot:
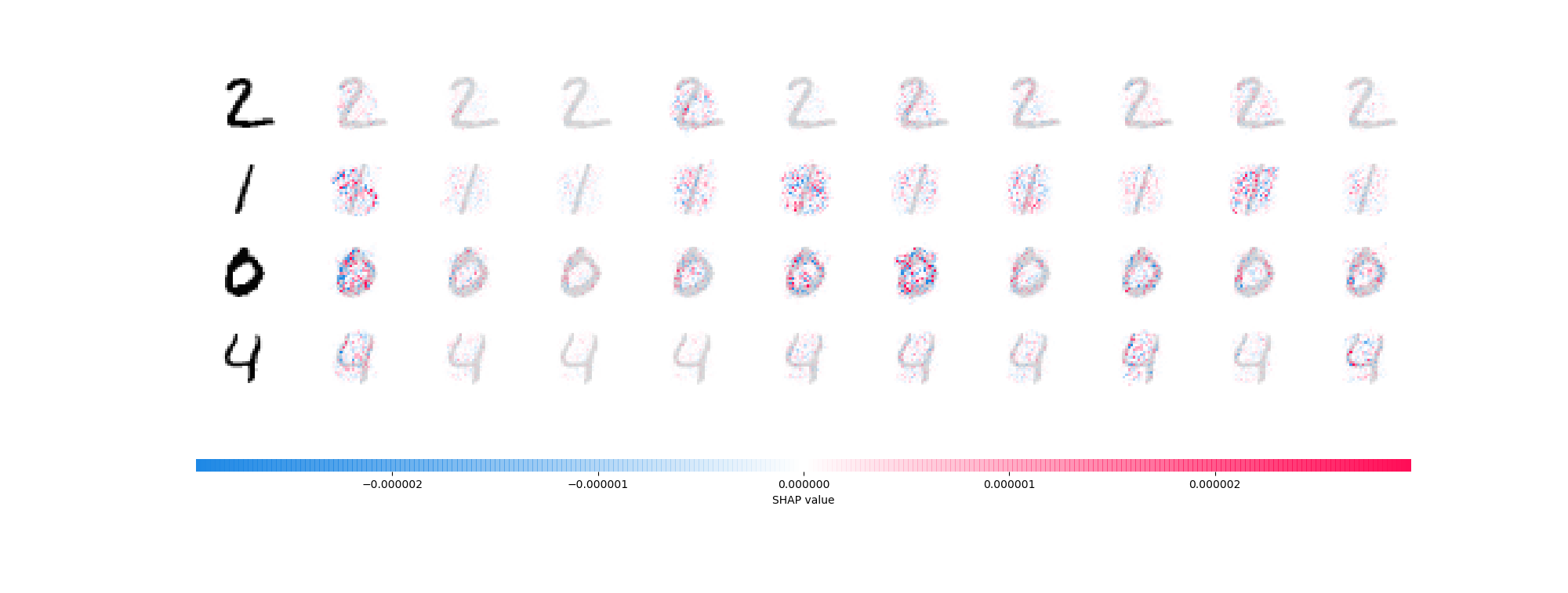
The text was updated successfully, but these errors were encountered: