You signed in with another tab or window. Reload to refresh your session.You signed out in another tab or window. Reload to refresh your session.You switched accounts on another tab or window. Reload to refresh your session.Dismiss alert
I am attempting to train the ssd_resnet_50_fpn_coco object detector on a simplified coco dataset, however the same issue persists even if I use the all coco classes. The metrics reported by tensorboard look off:
The number of AvgNumGroundtruthBoxesPerImage is always 100, the maximum number of output boxes from the model.
Indeed in the tensorboard display, there are some images with no GT bboxes:
While the loss in general decreases, the performance of the detector is very poor. After 15000 steps with a batchsize of 8:
Average Precision (AP) @[ IoU=0.50:0.95 | area= all | maxDets=100 ] = 0.015
Average Precision (AP) @[ IoU=0.50 | area= all | maxDets=100 ] = 0.031
Average Precision (AP) @[ IoU=0.75 | area= all | maxDets=100 ] = 0.015
Average Precision (AP) @[ IoU=0.50:0.95 | area= small | maxDets=100 ] = 0.000
Average Precision (AP) @[ IoU=0.50:0.95 | area=medium | maxDets=100 ] = 0.004
Average Precision (AP) @[ IoU=0.50:0.95 | area= large | maxDets=100 ] = 0.031
Average Recall (AR) @[ IoU=0.50:0.95 | area= all | maxDets= 1 ] = 0.018
Average Recall (AR) @[ IoU=0.50:0.95 | area= all | maxDets= 10 ] = 0.025
Average Recall (AR) @[ IoU=0.50:0.95 | area= all | maxDets=100 ] = 0.026
Average Recall (AR) @[ IoU=0.50:0.95 | area= small | maxDets=100 ] = 0.000
Average Recall (AR) @[ IoU=0.50:0.95 | area=medium | maxDets=100 ] = 0.006
Average Recall (AR) @[ IoU=0.50:0.95 | area= large | maxDets=100 ] = 0.059
The text was updated successfully, but these errors were encountered:
Hi Zhangqian,
After tuning some hyperparameters, I got better performance (not super well, but enough for my needs). I think the problem is mainly due a reporting problem, and not an actual performance problem.
I think at this point, with YOLOv3 impressive performance, there is little reason to use a SSD architecture. YMMV
This is due to a parameter in the pipeline.config called unpad_groundtruth_tensors which I assume pads this list to whatever the maximum number of boxes is. Basically the stats in TargetAssignment give you information about how ground truth bounding boxes are being assigned to anchors in the model. These numbers don't affect training in any way, but may be useful to catch errors in your dataset and also for model debugging.
If you set this from false to true, you'll get the "right" number being reported. It's simply tracking how many ground truth boxes on average you have in your dataset.
I'm not sure what the purpose of padding this is - there are references to this in the codebase, e.g. here. As it doesn't adversely affect training, I'd leave it on just in case.
System information
Describe the problem
I am attempting to train the ssd_resnet_50_fpn_coco object detector on a simplified coco dataset, however the same issue persists even if I use the all coco classes. The metrics reported by tensorboard look off:
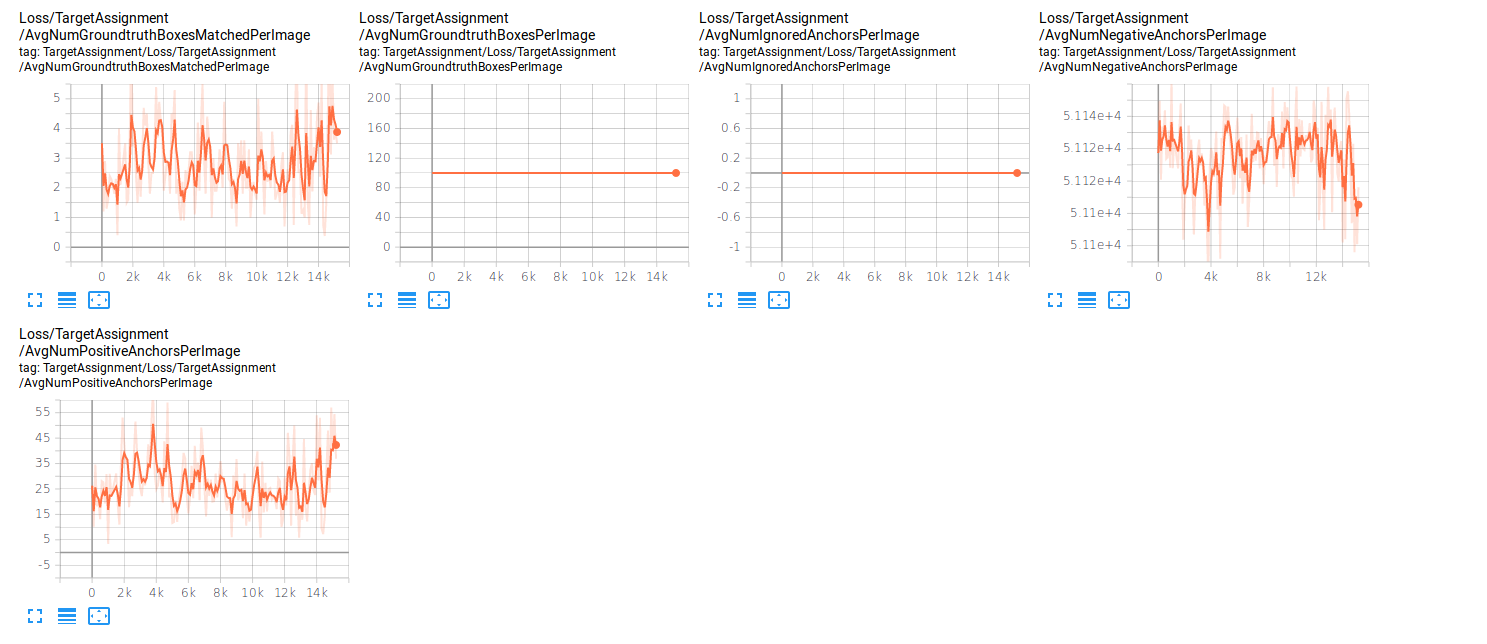
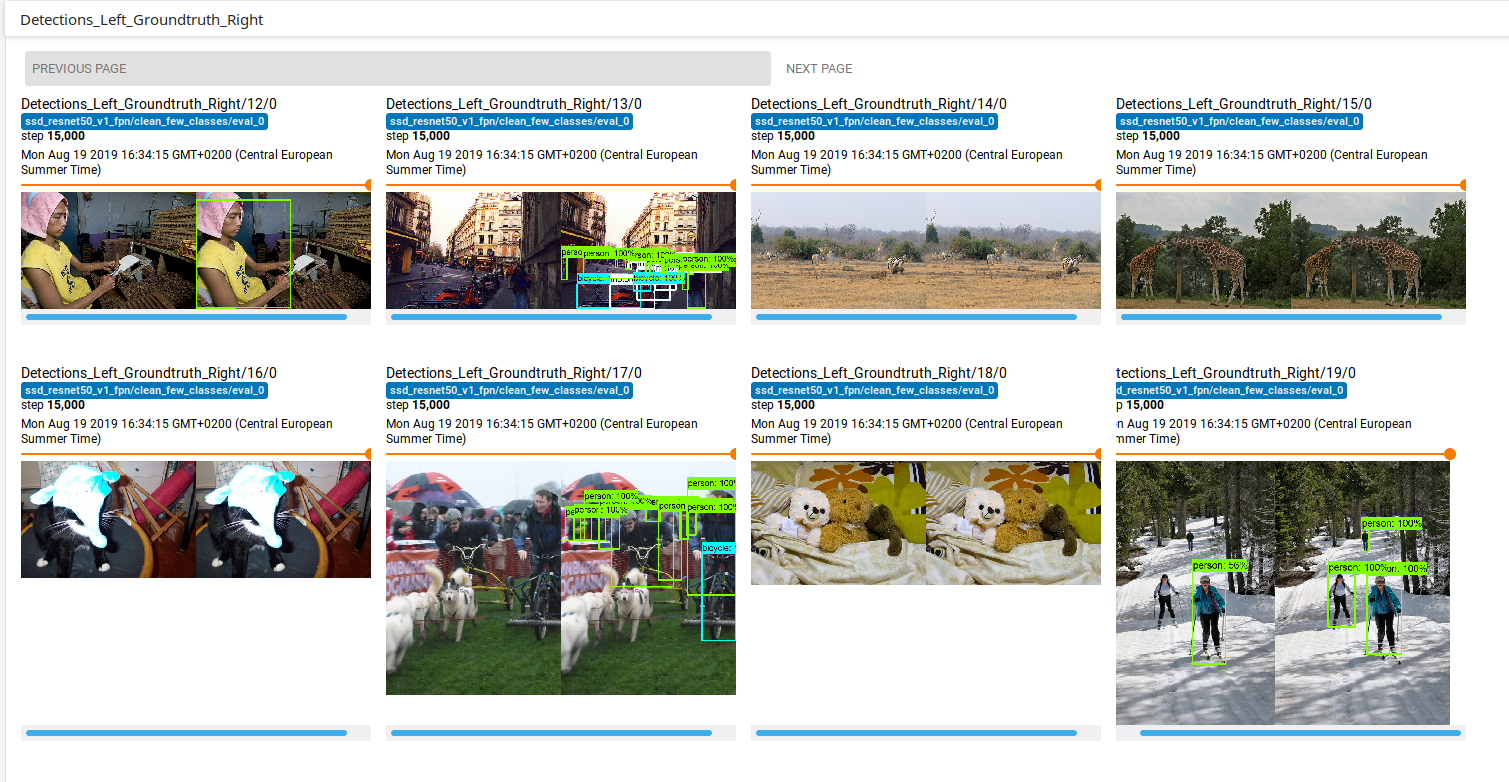
The number of AvgNumGroundtruthBoxesPerImage is always 100, the maximum number of output boxes from the model.
Indeed in the tensorboard display, there are some images with no GT bboxes:
While the loss in general decreases, the performance of the detector is very poor. After 15000 steps with a batchsize of 8:
The text was updated successfully, but these errors were encountered: