-
-
Notifications
You must be signed in to change notification settings - Fork 39
New issue
Have a question about this project? Sign up for a free GitHub account to open an issue and contact its maintainers and the community.
By clicking “Sign up for GitHub”, you agree to our terms of service and privacy statement. We’ll occasionally send you account related emails.
Already on GitHub? Sign in to your account
Feature request/bug fix: Perform scaling and other operations in linear light #59
Comments
Hello! Thanks for doing the tests and experimenting. I am aware of the linear space operations on images, the correct functions are used in iNNfer: Where a "linear_resize" is also implemented: However, the conversions add considerable latency in the training process (every image in every batch has to be converted back and forth between SRGB and linear) and not all operations on images require them to be applied on the linear space. Additionally, the logic may be better implemented as a wrapper in https://github.com/victorca25/augmennt, but I haven't had time to evaluate the different implementation options and compare results between the current case and doing the linear conversions, but considering no current SOTA project does SRGB to linear conversions before doing the images operations and results are not impacted, the priority to implement it is relatively low, in comparison to other WIP elements. However, if during your testing you find results improve with the conversions, the priority can change. |
Looking at linear2srgb that appears to be missing a rounding step before conversion to avoid truncation ( I did a little experiment, it's not much but in the interest of time I ran 500 iterations on DIV2K (using the pre-trained 4xPSNR as a starting point) with both srgb and linear rgb downscaling (modifying MLResize augmentations.py). With the default sRGB downscaling my first validation was Using these two models I took this this original image: With no modifications to trainner (srgb downscaling): With augment.py switched to use linear RGB downscaling: While this was a very small test I do think it demonstrates the impact of colour spaces on downscaling on training. Neither one of them was perfect inside of 500 iterations but the models trained on linear RGB downscaling were much closer in colour. The point isn't that either of these two models is actually any good, but that downscaling colour space can make a difference. This should be repeatable. Even compared to one of the more well-regarded models (yandere neo xl) the results are roughly on par (I'd argue subjectively better, but marginally worse by PSNR/MAE) in terms of colour accuracy despite only 500 iterations. yandere_neo_xl
|
I was looking to try this out to train an upscaling model but thought to try one of my test images first, and found that downscaling was being done in srgb gamma. Most images are encoded as srgb (~188 is half as bright as 255) but downscaling algorithms, where it's especially relevant, assume they're taking linear rgb as input (~127 is half as bright as 255).
I used this image as my input (this isn't a good image for training an upscaler but it does demonstrate the problem) and manually ran it through
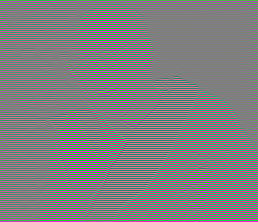
resize
fromimresize.py
, the same way it is done ingenerate_mod_LR_bic.py
. It's best to open this image in a program that does not perform any scaling, since your browser might be doing some.What I got out of it at 1/4 scale was a uniform grey square.
But I can fix this by converting it to and from linear RGB using the methods you already have in
colors.py
(the functions are named incorrectly,rgb2srgb
should besrgb2rgb
and vice versa):This code snippet gives me the expected result:
While this is an artificial example that exaggerates the effect, the colour distortion is going to happen to a varying degree on any images that are transformed in non-linear gamma. I believe this is decreasing the accuracy of the trained models, since they'll be learning to attempt to reverse this colour distortion which can cause noticeable colour shift when upscaling images that were not produced from srgb downscaling.
The text was updated successfully, but these errors were encountered: