New issue
Have a question about this project? Sign up for a free GitHub account to open an issue and contact its maintainers and the community.
By clicking “Sign up for GitHub”, you agree to our terms of service and privacy statement. We’ll occasionally send you account related emails.
Already on GitHub? Sign in to your account
Issues on FedScale and Oort: (1) widely promotes its so-called advantages that are not based new version of FedML (10 months outdated until today) (2) The evaluation of FedML old version (Oct, 2021) is not based on facts and overlapping with a published paper; (3) unrealistic overlap between system efficiency and data distribution (4) issues on numerical optimization (5) dual submission? #163
Comments
We are very happy to see your entire team trying out FedScale! This means we are on the right track, and will encourage us to work hard to improve FedScale as a good open-source tool for the community. We're happy to see FedML making good progress in the latest version. Meanwhile, we notice your concerns coincide with a thoughtful review here (especially Reviewer#3) that helped us clarify and update our work last year. Finally, we thank you for your support and appreciate your pull request to improve FedScale. |
@fanlai0990 Please do not close this issue before addressing the concern. I am waiting for your paper update to clarify our concern. FedML team, USC lab, and many collaborators take these issues seriously. I don't think my comments here coincide with the NeurIPS dataset track review. Thanks for reminding us to read your previous review. After reading the review you posted, I think the review writing is professional with many facts. The score there is also reasonable. |
Thank you for diligently helping us to improve FedScale! These similar concerns remind us of the thoughtful Reviewer#3@ NeurIPS'22. While Reviewer#3 gave us a strong reject -- the rest of reviewers@NeurIPS gave 2 strong accept, 2 accept and 2 weak accept, and this benchmarking part of FedScale is eventually accepted@ICML -- his/her feedback is valuable. We believe readers can have a better understanding/answer to your concerns after reading our rebuttal.
Note:
|
This is my final reply. I am happy to see more researchers working on federated/distributed ML. Your contribution definitely pushes forwards the progress in this domain. You will earn more respect from the FedML team based on a proper comparison and description. Summary of my final comment:
For details, please check the fact below.@fanlai0990 Hi Fan, again, we are talking about the issues I raised here, not about an outdated NeurIPS review. It's strange to me that why you keep mentioning the NuerIPS review to us. I only want to solve the issues I mentioned in this thread professionally. Let's focus on facts. For the disrespectful and wrong claims over FedML, it's obvious that only mentioning an ID in the appendix without date is not enough (https://arxiv.org/pdf/2105.11367v5.pdf, commit id in appendix without date), since most readers won't read the Appendix (e.g., I only know you mention the ID after you told me today). More seriously, your blogs (e.g. Zhihu post sent to FedML WeChat group) and many other media posts do not mention the version ID. This triggers even more misunderstandings in widespread, which invades FedML reputation widely. The commit ID you compared with is commit@2ee0517 on Oct 3, 2021 (https://arxiv.org/pdf/2105.11367v5.pdf), which is around 10 months before the ICML conference date (July 2022) (so outdated!!!). And even more seriously, the version date is 3.5+ months earlier than the ICML submission deadline (Jan 20, 2022 AOE)! (https://icml.cc/Conferences/2022/CallForPapers), and half an year before the Author Feedback Opens Apr 06 '22(Anywhere on Earth, https://icml.cc/Conferences/2022/Dates). At that time, FedML new version has been released. The version date does not match with Jiachen's claim on twitter and wechat. Please check your Zhihu post sent to FedML WeChat group here: https://zhuanlan.zhihu.com/p/520020117 and https://zhuanlan.zhihu.com/p/492795490. No software version is mentioned. Our team believes that you misunderstand FedML library a lot and only compares a small part of the code. See the following timeline, it's strange to our team that you even don't update your paper in the conference date and keep updating social media without telling readers any version tag even after we started our company and released new versions. Timeline: Date: June 2020 - NeurIPS 2022 FL workshop- FedML library won Best Paper Awards; the code you compared in your paper is not the big picture of our system. We believe you misunderstood us. Date: Oct 3, 2021: the version you used to run your experiments. You only compare with part of FedML code, which is ok to us since you appreciate our work and compare it for your ICML submission. Date: Jan 20, 2022 AOE: ICML Abstract submission deadline. Note: it's 3.5 months after the version you used for FedML comparison. Date: Feb, 2022 - FedML new version was released: this is much earlier than the date ICML was doing rebuttal and ICML camera ready version. Check ICML timeline here: https://icml.cc/Conferences/2022/Dates. Date: April 2022: your Zhihu post date(https://zhuanlan.zhihu.com/p/520020117 and https://zhuanlan.zhihu.com/p/492795490). At this version, you didn't mention any version ID. The version you used is half a year passed (such a long time for software iteration)! You even continue to claim your advantages, which brings some trouble for FedML reputation, but we didn't reply publicly. We believe people will see our progress. Date: July 17-23, 2022 - your ICML presentation: at this version, you didn't mention any version ID in the main text. In the appendix, the commit id is from Oct 3, 2021. 9 months passed, still no update in the paper!!! Date: August, 2022 (Early this week) - This triggers my reply here: we don't want to comment or discuss any improper behaviors from your team, but someone posted the zhihu message to promote your library in our FedML WeChat group to promote it, and your co-author (Jiachen) has some improper further comments in our group chat (claiming some advantages and misunderstanding of FedML, most importantly, "we compare an early version this year (2022)". This fully triggers wide concerns from FedML company and USC lab. Many collaborators and our CEO Professor Salman Avestimehr are also there. So we raised issues here to defend. For the issue item 3 I raised (training using local iteration), it is the evidence of your improper comparison and experimental results. If you keep the iteration number the same, it definitely runs faster than epoch-based FedML experiments since you reduce some running time related to data heterogeneity, i.e., the client with more samples needs to run more time for a single epoch, but runs the same time using iteration-based method as all other clients. FedML old version does not support iteration-based training. If comparing with FedML using iteration-based location training, how did you run such experiments? by changing FedML code? any comments in your paper? for the issue item 2, you haven't answered it yet. But it's ok, we don't care about it. BTW, thanks for you reminding us the open review from NeurIPS benchmark track. Why don't you dare to post the entire open review and only post a screenshot for a specific review? are other "strong acc"/"acc" reviews from professional reviewers who have experience in FL and understand FL library deeply? Do they write reviews based on facts? Please let people see the entire big picture and don't hide something. |
Dear FedScale team, we are open to discuss these potential issues. So far, we unfortunately haven’t received clear answers for these issues yet. |
Dear Authors of FedScale,
I didn't want to comment too much on FedScale because I thought all the experts in the field knew the truth. But you promote your outdated paper for a long time without based on facts and your co-author (e.g., Jiachen) always publicly claim the inaccurate advantages of FedScale over FedML on the Internet, which is a deep harm and disrespect to FedML's past academic efforts and current industrialization efforts. Therefore, it is necessary for me to state some facts here, and let people know the truth.
Summary
FedScale paper have adopted the method of evaluating the old version of FedML during the ICML submission (3 months expired at the time of submission), and still did not mention it during Camera Ready (6 months expired) and during the conference speech period (about 10 months expired). The issue of comparing with FedML in an old version, and in this case, widely publicizing its so-called advantages that are not based on facts and new version, has brought a lot of harm and loss to FedML. Aside from the harm caused by the publication and publicity of the old version, even the comparison of the old version is academically inaccurate and wrong: there are 4 core arguments in the paper, 3 are not in line with the facts, and the fourth has highly overlaps with existing papers. In addition, the ICML paper substantially overlaps with a proceeding-based published paper at another workshop. Based on these issues, we think this paper violates the dual submission policy and does not meet the criteria for publication. We also hope FedScale team can update paper (https://arxiv.org/pdf/2105.11367v5.pdf) and media articles (Zhihu, etc.) in a timely manner, clarifying the above issues, avoiding misunderstandings among users and peers in the Chinese and English communities, and terminating unnecessary reputation damage .
Issue 1: FedScale widely promotes its so-called advantages that are not based new version (10 months outdated until today)
https://arxiv.org/pdf/2105.11367v5.pdf - Table 1's comment on FedML is fully wrong and outdated.
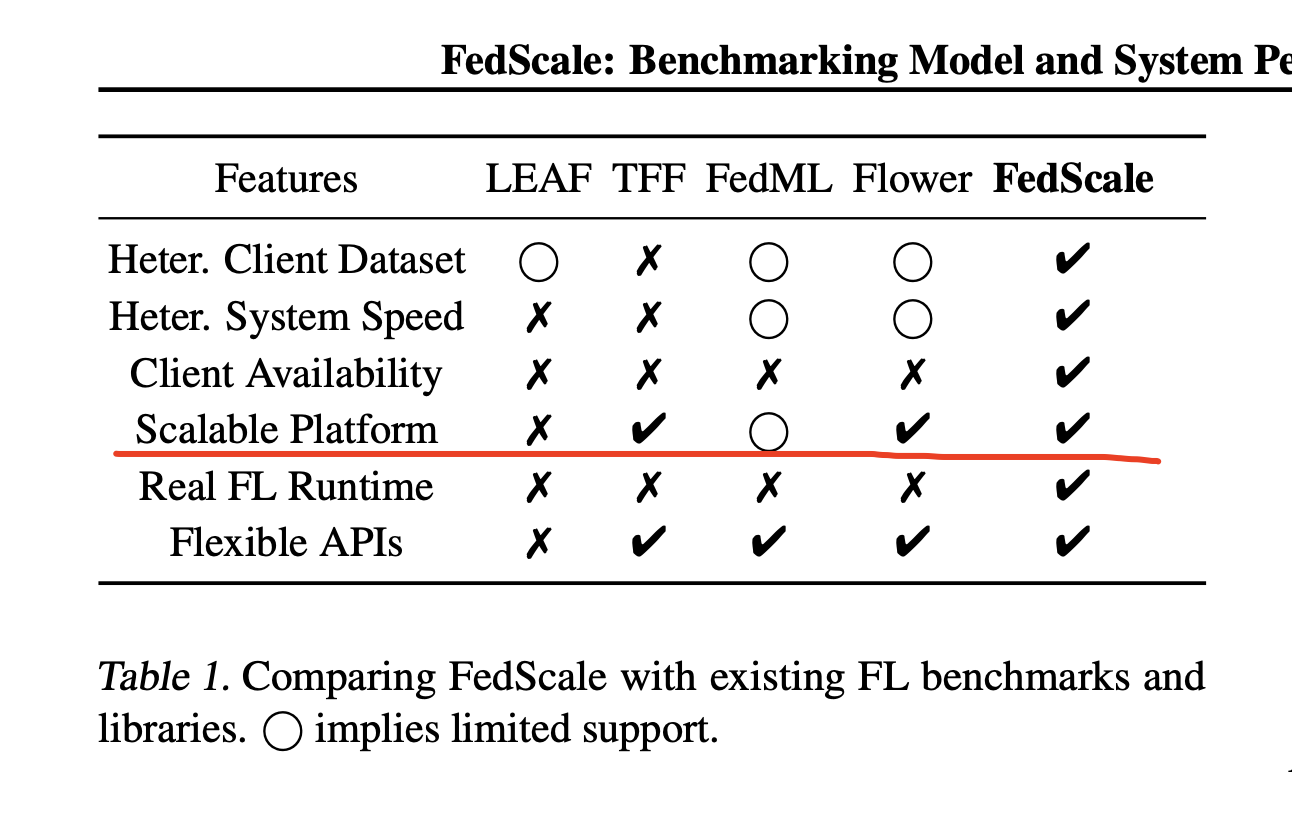
My comments: It's surprising to many engineers and researchers at USC and FedML that you overclaim that your platform has stronger "Scalable Platform". Please check our platform at https://fedml.ai.
FedML AI platform releases the world’s federated learning open platform on the public cloud with an in-depth introduction of products and technologies!
https://medium.com/@FedML/fedml-ai-platform-releases-the-worlds-federated-learning-open-platform-on-public-cloud-with-an-8024e68a70b6
Issues 2: The evaluation of FedML old version (Oct, 2021) is not based on facts. In addition, the core contribution of FedScale ICML paper (system and data heterogeneity) overlaps a published paper Oort. ICML reviewers should be aware of these issues.
Quote from https://arxiv.org/pdf/2105.11367v5.pdf: "First, they are limited in the versatility of data for various real-world FL applications. Indeed, even though they may have quite a few datasets and FL training tasks (e.g., LEAF (Caldas et al.,
2019)), their datasets often contain synthetically generated partitions derived from conventional datasets (e.g., CIFAR)
and do not represent realistic characteristics. This is because these benchmarks are mostly borrowed from traditional ML benchmarks (e.g., MLPerf (Mattson et al., 2020)) or designed for simulated FL environments like TensorFlow Federated (TFF) (tff) or PySyft (pys). Second, existing benchmarks often overlook system speed, connectivity, and availability of the clients (e.g., FedML (He et al., 2020) and Flower (Beutel et al., 2021)). This discourages FL efforts from considering system efficiency and leads to overly optimistic statistical performance (§2). Third, their datasets are primarily small-scale, because their experimental environments are unable to emulate large-scale FL deployments. While real FL often involves thousands of participants in each training round (Kairouz et al., 2021b; Yang et al., 2018), most existing benchmarking platforms can merely support the training of tens of participants per round. Finally, most of them lack user-friendly APIs for automated integration, resulting in great engineering efforts for benchmarking at scale"
These four core arguments are not based on facts:
FedGraphNN: FedGraphNN: A Federated Learning System and Benchmark for Graph Neural Networks. https://arxiv.org/abs/2104.07145 (Arxiv Time: 4 Apr 2021)
FedNLP: FedNLP: Benchmarking Federated Learning Methods for Natural Language Processing Tasks. https://arxiv.org/abs/2104.08815 (Arxiv Time: 8 Apr 2021)
FedCV: https://arxiv.org/abs/2111.11066 (Arxiv time: 22 Nov 2021)
FedIoT: https://arxiv.org/abs/2106.07976v1 (Arxiv time: 15 Jun 2021)
(1) https://arxiv.org/pdf/2105.11367v5.pdf - "FedML can only support 30 participants because of its suboptimal scalability, which under-reports the FL performance that the algorithm can indeed achieve"
My comments: This doesn't match the fact. From the oldest version of FedML, it always supports arbitrary number of clients training by using the single process (standalone in the old version) sequential training. In addition, our users can run parallel experiments (one GPU per job/run) with multiple GPUs to accelerate the hyperparameter tuning. This avoids communication cost in emulator level. In our latest version, it supports sequential training with multiple nodes via efficient scheduler. Therefore, such a comment does not match the fact.
(3) https://arxiv.org/pdf/2105.11367v5.pdf - "Third, their datasets are primarily small-scale, because their experimental environments are unable to emulate large-scale FL deployments."
My comments: this is also misleading to paper readers. In our old version, we already support many large-scale datasets for reseachers in ML community: https://doc.fedml.ai/simulation/user_guide/datasets-and-models.html. They are widely used by many ICML/NeurIPS/ICLR papers. Recently, our latest version even support many realistic and large-scale datasetes in CV, NLP, healthcare, graph neural networks, and IoT. See some links at:
https://github.com/FedML-AI/FedML/tree/master/python/app
https://github.com/FedML-AI/FedML/tree/master/iot
Each one is supported by top-tier conference papers. For example, the NLP one (https://arxiv.org/abs/2104.08815) is connected to Huggingface and accepted to NACCL 2022.
We released FedNLP, FedGraphNN, FedCV, FedIoT and other application frameworks as early as 1.5 years ago (https://open.fedml.ai/platform/appStore), all based on the FedML core framework, after so many applications Verification has long proven its convenience. Regardless of these differences, the best way to prove "convenience" is the user data, which you can look at our GitHub stars, paper citations, platform user number, etc.
We also put together a brief introduction to APIs to see who is more convenient: https://medium.com/@FedML/fedml-releases-simple-and-flexible-apis-boosting-innovation-in-algorithm-and-system-optimization-b21c2f4b88c8
We simulate real-world heterogeneous client system performance and data in both training and testing evaluations using an open-source FL benchmark [48]: (1) Heterogeneous device runtimes (speed) of different models, network throughput/connectivity (connectivity), device model, and availability are emulated using data from AI Benchmark [1] and Network Measurements on mobiles [6].
Issues 3: issues in FedScale and Oort: unrealistic overlap between system speed, data distribution, and client device availability
Quote from https://arxiv.org/pdf/2105.11367v5.pdf: "Second, existing benchmarks often overlook system speed, connectivity, and availability of the clients (e.g., FedML (He et al., 2020) and Flower (Beutel et al., 2021)). This discourages FL efforts from considering system efficiency and leads to overly optimistic statistical performance (§2)."
My comments: this is misleading. My question is "how can you match a realistic overlapping of system speed, data distribution statistics, and client device availability?" You get them from three independent databases, which does not match the practice. Then you build Oort based on this unrealistic assumption. FedScale team never clearly answers this question. This benchmark definitely brings issues in numerical optimization theory. We ML and System researchers do not hope this misleading benchmark to misguide the research in ML area.
Moreover, such a comment ("existing benchmarks often overlook system speed, connectivity, and availability of the clients") is extremely disrespectful to the work of an industrialized team who has expertise more than this. Distributed system is the hardcore area that FedML engineering team focuses on. Maybe your team only reads part of the materials (white paper? or part of our source code?). Please refer to a comprehensive material list here:
FedML Homepage: https://fedml.ai/
FedML Open Source: https://github.com/FedML-AI
FedML Platform: https://open.fedml.ai
FedML Use Cases: https://open.fedml.ai/platform/appStore
FedML Documentation: https://doc.fedml.ai
FedML Research: https://fedml.ai/research-papers/ (50+ papers covering many aspects including security and privacy)
Issues 4: FedScale only supports running on the same number of iteration locally, however, many ICML/NeurIPS/ICLR papers (almost all) are working on the same number of epochs. This differs from the entire ML community significantly.
FedScale/fedscale/core/execution/client.py
Line 50 in 51cc4a1
Issue 5: We suspect that FedScale ICML paper violates the dual submission policy in ML community
The FedScale ICML version (ICML proceeding https://proceedings.mlr.press/v162/lai22a/lai22a.pdf) overlaps substantially with a workshop paper with proceeding (https://dl.acm.org/doi/10.1145/3477114.3488760). The workshop date is October 2021, at least 3 months earlier than the ICML 2022 submission deadline. Normally, ICML/NeurIPS/ICLR do not allow submissions that are already published somewhere else with proceeding using the same title/author/core contribution.
(1) These two papers have the same title "FedScale: Benchmarking Model and System Performance of Federated Learning at Scale".
(2) These two papers have 5 authors overlapping
Workshop authors: Fan Lai, Yinwei Dai, Xiangfeng Zhu, Harsha V. Madhyastha, Mosharaf Chowdhury
ICML authors: Fan Lai, Yinwei Dai, Sanjay S. Singapuram, Jiachen Liu, Xiangfeng Zhu, Harsha V. Madhyastha, Mosharaf Chowdhury
(two authors are added in the ICML version)
(3) substantial contribution and core argument overlapping. See the two key paragraphs in these two papers.
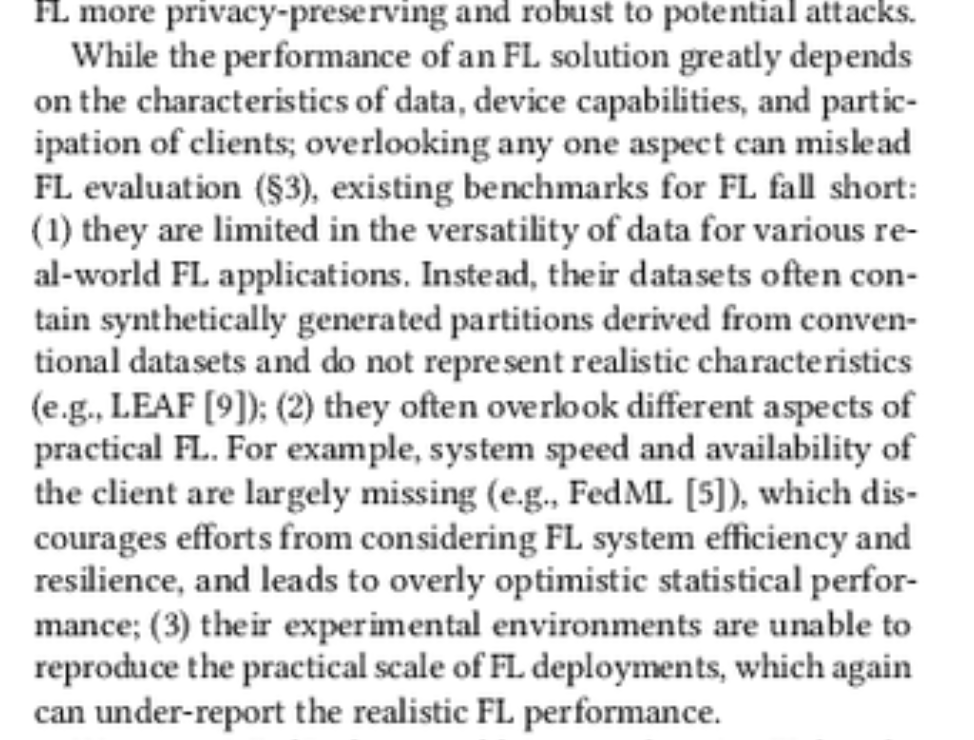
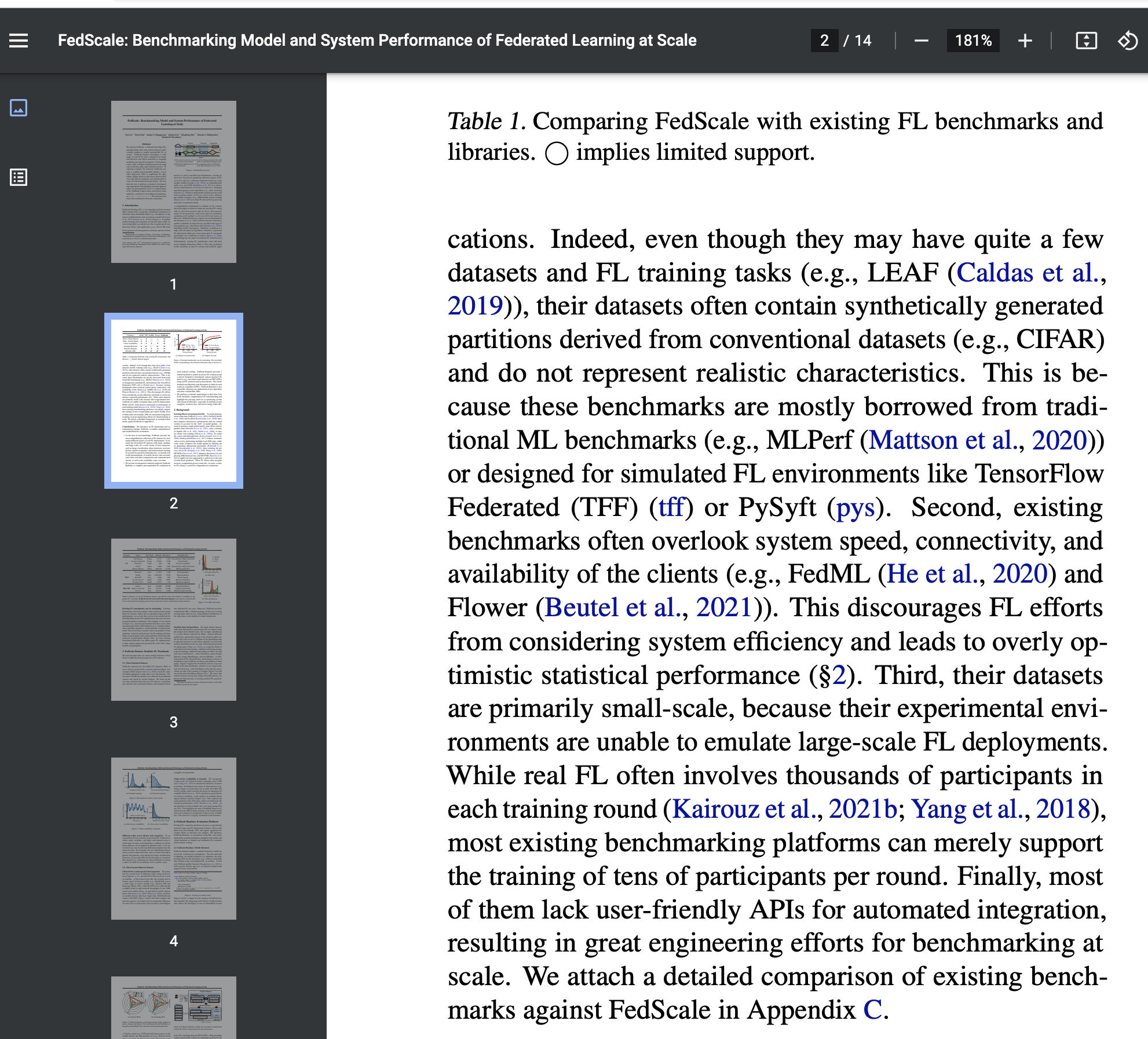
Note: these two papers are talking about the same arguments with the same wording.ICML policy: https://icml.cc/Conferences/2022/StyleAuthorInstructions
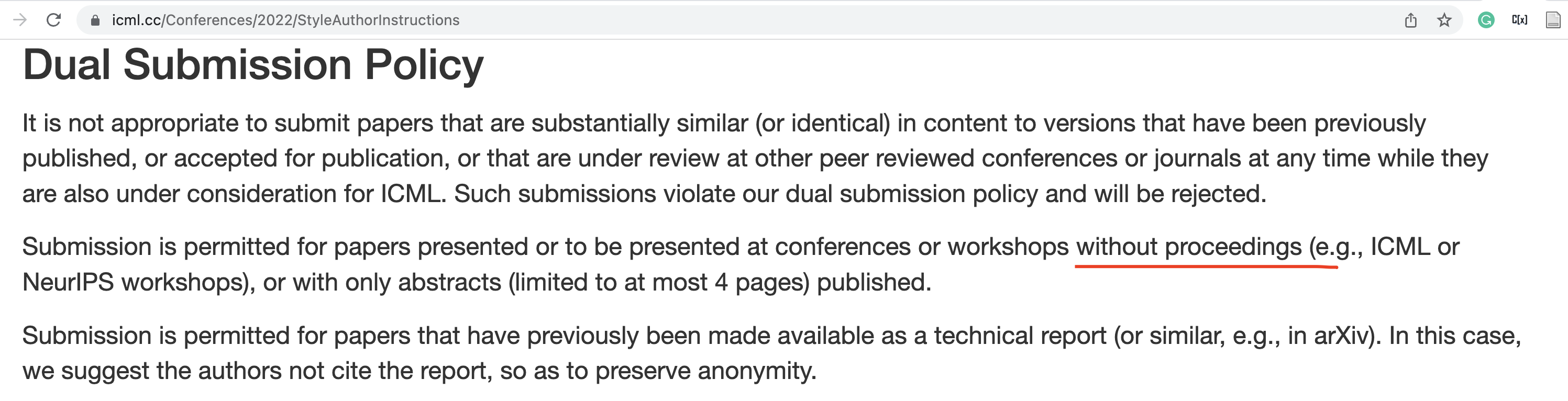
As mentioned in issue 2, FedScale ICML 2022 paper also overlaps a key contribution with another published paper at OSDI 2021:
The 2nd key argument has been mentioned in another paper Oort (highly overlapping, please compare the two papers; Oort is here: https://arxiv.org/abs/2010.06081), which does not belong to the spirit of ICML that requires independent contribution and novelty of a published paper.
Specifically, system heterogeneity (system speed, connectivity and availability) has been described in Section 2.2 of Oort's original paper, and also clearly mentioned in Section 7.1 of the experimental section. System speed, connectivity and availability are the same things as Section 3.2 in the original FedScale article. Oort says:
We simulate real-world heterogeneous client system performance and data in both training and testing evaluations using an open-source FL benchmark [48]: (1) Heterogeneous device runtimes (speed) of different models, network throughput/connectivity (connectivity), device model, and availability are emulated using data from AI Benchmark [1] and Network Measurements on mobiles [6].
Versions / Dependencies
Code: https://github.com/SymbioticLab/FedScale (51cc4a1)
Paper: https://arxiv.org/pdf/2105.11367v5.pdf (v5)
The text was updated successfully, but these errors were encountered: