-
Notifications
You must be signed in to change notification settings - Fork 50
New issue
Have a question about this project? Sign up for a free GitHub account to open an issue and contact its maintainers and the community.
By clicking “Sign up for GitHub”, you agree to our terms of service and privacy statement. We’ll occasionally send you account related emails.
Already on GitHub? Sign in to your account
Strange results on 10X hgmm10k_v3 dataset #51
Comments
I believe I have a parsing error in the newer v3 format HDF5 files from CellRanger that involve multiple genomes! I will track down this bug asap. Thanks for reporting. I think what you're seeing there is essentially a garbled output due to an input parsing error. |
For an urgent workaround, I believe you can input your data using the CellRanger mtx directory format, and then even the v3 multiple-genome data should be parsed correctly. But this is a hunch, and I still need to try it myself. Either way, I will be working on fixing that bug soon. |
Thank you for the reply! If what I saw was simply garbled output, I would expect to see some cells with high mouse gene counts. The fact that 1) 90% of mouse gene counts are removed from all cells, 2) tens of thousands human gene counts are added to cells that originally had only hundreds, and 3) the inferred priors and cutoffs look correct, makes me suspect it might be due to something else. |
Tried supplying mtx and got exactly the same result. The code for loading/parsing input seems alright. Though it doesn't read "/matrix/features/genome", the inference shouldn't care about an extra gene label, should it? CellBender/cellbender/remove_background/data/dataset.py Lines 845 to 871 in d68bf9d
What I notice is that in hgmm5k_v3 and hgmm10k_v3, nUMI per cell is distinctively lower in mouse cells than human cells, whereas in hgmm12k_v2 the difference is smaller. See plots below (blue: human, green: mouse, green: empty droplets) Could it be that this distribution somehow confused the method to (partially) model empty droplets out of mouse cells? |
I will look into that, but the fact that you gave it the |
Just wanted to add that the HgMm mix datasets are sometimes problematic in assessing ambient RNAs, as I've found 0%-3% of UMIs (depending on cell type) will end up in the wrong species cells simply due to mismapping thanks to genome, and annotation and sequencing error. |
Found it. It was coming from the use of the datatype I will push a fix for this soon. |
Thank you for the quick fix! |
Hi,
While CellBender works as expected on 10X hgmm12k (v2), on 10X hgmm10k (v3), it strangely removes large mouse gene counts and adds large human gene counts to mouse cells. 10X hgmm5k (v3) gives similar unexpected results as hgmm10k (v3). Please see logs and plots (hgmm12k and hgmm10k only) below:
hgmm12k, v2
Elbow plot, vertical line marks --expected-cells and --total-droplets-included:
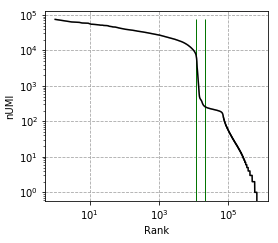
Before correction (called cells):
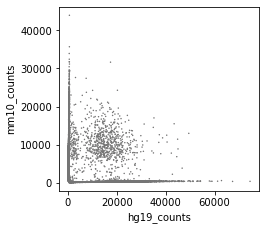
After correction (called cells):
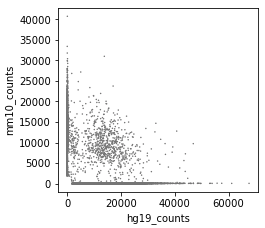
Convergence:
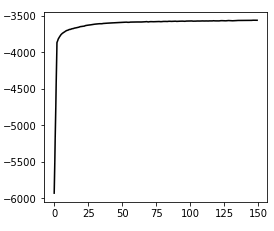
hgmm10k, v3
Elbow plot, vertical line marks --expected-cells and --total-droplets-included:
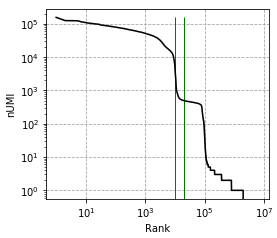
Before correction (called cells):
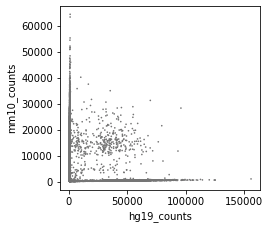
After correction (called cells):
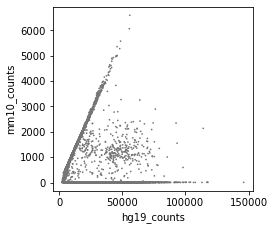
Convergence:
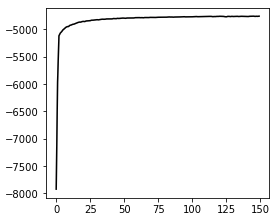
The text was updated successfully, but these errors were encountered: