This repository has been archived by the owner on Jul 2, 2021. It is now read-only.
Add this suggestion to a batch that can be applied as a single commit.
This suggestion is invalid because no changes were made to the code.
Suggestions cannot be applied while the pull request is closed.
Suggestions cannot be applied while viewing a subset of changes.
Only one suggestion per line can be applied in a batch.
Add this suggestion to a batch that can be applied as a single commit.
Applying suggestions on deleted lines is not supported.
You must change the existing code in this line in order to create a valid suggestion.
Outdated suggestions cannot be applied.
This suggestion has been applied or marked resolved.
Suggestions cannot be applied from pending reviews.
Suggestions cannot be applied on multi-line comments.
Suggestions cannot be applied while the pull request is queued to merge.
Suggestion cannot be applied right now. Please check back later.
What this is
This code exploits sparsity of
mask
used for instance segmentation to speed upscale
operation.When scaling factor is an integer, there is no difference between
resize
.When scaling factor is not an integer, there is one pixel error in horizontal or vertical direction.
Benchmark
The script below benchmarks performance in comparison to the normal
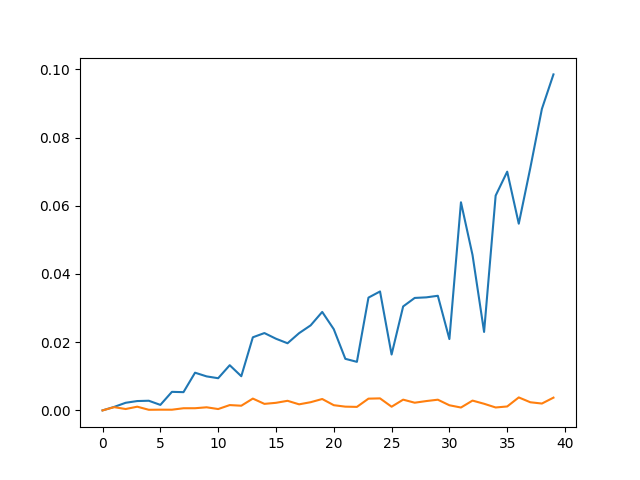
resize
.The comparison is made by varying the number of instances in an image.
The figure shows the execution time in seconds.
Blue is the time taken by
resize
and orange is the execution time ofscale_mask
.