-
Notifications
You must be signed in to change notification settings - Fork 19
New issue
Have a question about this project? Sign up for a free GitHub account to open an issue and contact its maintainers and the community.
By clicking “Sign up for GitHub”, you agree to our terms of service and privacy statement. We’ll occasionally send you account related emails.
Already on GitHub? Sign in to your account
[[hint]] silent=FALSE #193
Comments
Looks like an issues with classes. Can you please provide a reproducible example (always do that if you can)? |
The reproduce able example works fine so far.
|
holdout seems to play a role...
smooth::es() (via modeltime wrapper) ... is preventing the plotting in smooth (via modeltime)... code add...
Update: msg-issue: Solved, must be an internal from modeltime/tidymodels, because plotting of extracted model works as expected. Even with NA-msg. Plotting an extracted model via smooth:
Doing the same (plotting extracted model) with ADAM brings: Fehler in if (any(noVariability) && any(all.vars(formulaToUse) %in% names(noVariability))) { :
|
Can't reproduce it native. |
I can reproduce the chart-design (native) by setting h=1
I' m not sure if this is right- comparing to the book-chapter.... https://openforecast.org/adam/SES.html Hinting with ggplot+plotly
Noticed 6: It also has a problem with: 'h=1'. Since modeltime had the same for a while, it seems to be a common problem in fc-plots. Anyway, this hint was fun :-). Finally: fixing the hint... finding the lost one step forward fc (h=1, ~conditional mean)... :-).
|
I noticed yesterday, that my local versions are way to old. I am working on multiple instances, sorry. |
Windows 7, greybox 1.0.5.41001, smooth 3.1.6.41004, R version 4.0.5
|
The same code can be simplified to (for reproducibility purposes, so that I do not need to load tidyverse packages):
So, I still cannot reproduce this. |
I assume that this can be closed now. |
Should be environment related. |
Hello,
I get different model-graphs with the same data and model-definitions in adam, auto_adam and smooth::es() when setting silent=FALSE. Is this normal, and what do the x-axes of the plots represent?
I also get this graph fitting ADAM, while cross validation for loss-function. What does the x on the left side mean?
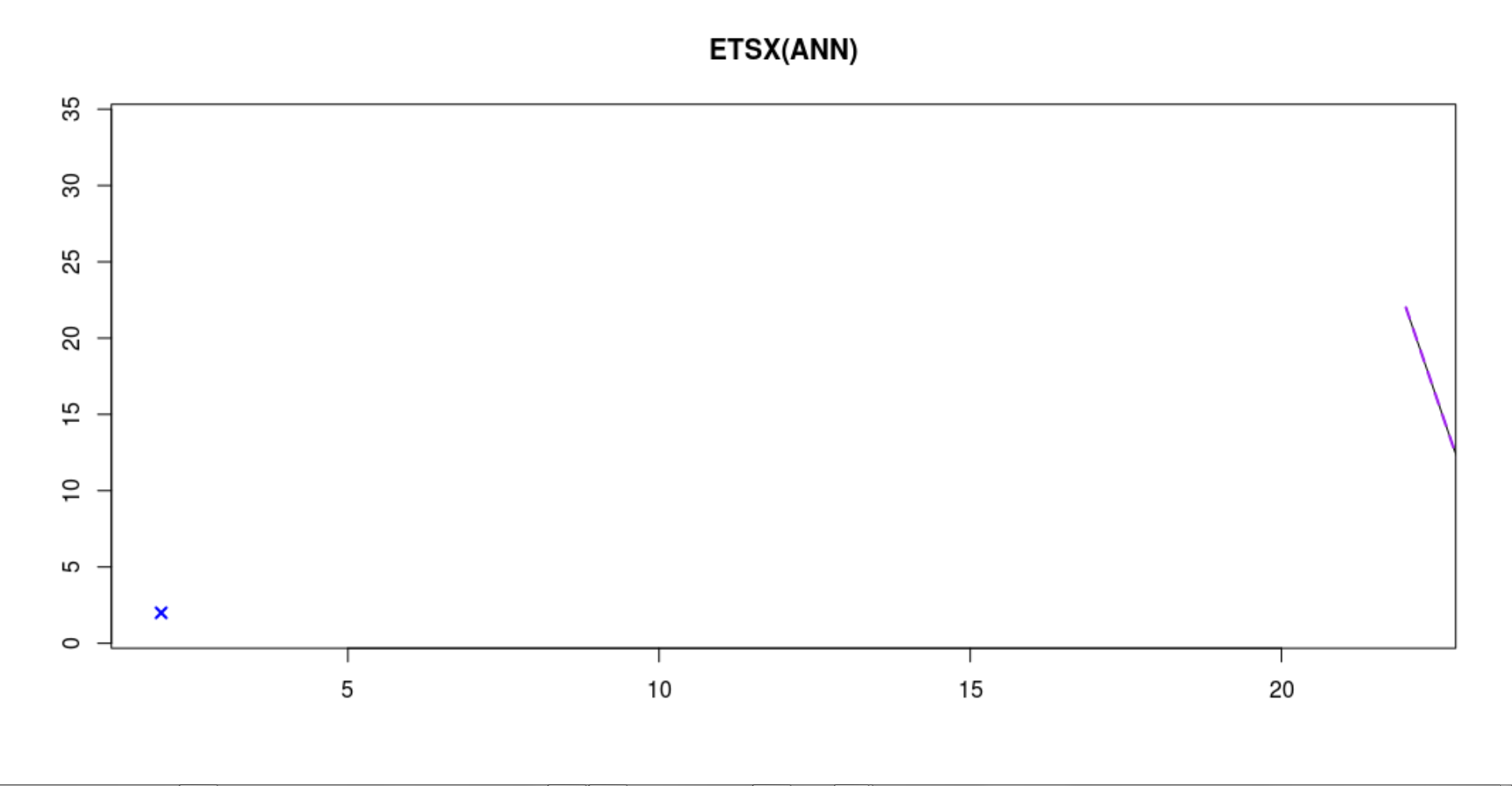
The text was updated successfully, but these errors were encountered: