-
Notifications
You must be signed in to change notification settings - Fork 37
New issue
Have a question about this project? Sign up for a free GitHub account to open an issue and contact its maintainers and the community.
By clicking “Sign up for GitHub”, you agree to our terms of service and privacy statement. We’ll occasionally send you account related emails.
Already on GitHub? Sign in to your account
path/referrer snapshot time series: bad total time range after merge #36
Comments
Initial investigation showed that in the above's special case the linkedin.com time series determined the overall range shown. That is, as part of merging individual time series the data ranges not within the linkedin.com time series range were lost. |
Found the problem: Line 519 in 47d5047
Done 'right' million times before, here I somehow fell for pandas and expected it to do magic. Which it did not. Created this snippet to show the problem, and a solution: dfa = pd.DataFrame(
list(range(10)),
columns=["A"],
index=pd.date_range("2021-01-03", periods=10, freq="D"),
)
dfb = pd.DataFrame(
list(range(10)),
columns=["B"],
index=pd.date_range("2021-01-10", periods=10, freq="D"),
)
print(dfa)
# A
# 2021-01-03 0
# 2021-01-04 1
# 2021-01-05 2
# 2021-01-06 3
# 2021-01-07 4
# 2021-01-08 5
# 2021-01-09 6
# 2021-01-10 7
# 2021-01-11 8
# 2021-01-12 9
print(dfb)
# B
# 2021-01-10 0
# 2021-01-11 1
# 2021-01-12 2
# 2021-01-13 3
# 2021-01-14 4
# 2021-01-15 5
# 2021-01-16 6
# 2021-01-17 7
# 2021-01-18 8
# 2021-01-19 9
# naive merge: just add a column from one dataframe to the other dataframe,
# and expect magic to happen
dfa['B'] = dfb['B']
# But what happens is that the index of the dataframe added to stays the same:
print(dfa)
# A B
# 2021-01-03 0 NaN
# 2021-01-04 1 NaN
# 2021-01-05 2 NaN
# 2021-01-06 3 NaN
# 2021-01-07 4 NaN
# 2021-01-08 5 NaN
# 2021-01-09 6 NaN
# 2021-01-10 7 0.0
# 2021-01-11 8 1.0
# 2021-01-12 9 2.0 A solution is to concat along a specific axis: merged = pd.concat([dfa['A'], dfb['B']], axis=1)
print(merged)
# A B
# 2021-01-03 0.0 NaN
# 2021-01-04 1.0 NaN
# 2021-01-05 2.0 NaN
# 2021-01-06 3.0 NaN
# 2021-01-07 4.0 NaN
# 2021-01-08 5.0 NaN
# 2021-01-09 6.0 NaN
# 2021-01-10 7.0 0.0
# 2021-01-11 8.0 1.0
# 2021-01-12 9.0 2.0
# 2021-01-13 NaN 3.0
# 2021-01-14 NaN 4.0
# 2021-01-15 NaN 5.0
# 2021-01-16 NaN 6.0
# 2021-01-17 NaN 7.0
# 2021-01-18 NaN 8.0
# 2021-01-19 NaN 9.0 |
Fix #36 by explicitly merging time series using pd.concat() along the 'right' axis.
Fix #36, improve style, align time ranges, and misc
This shows a range between Sep and Oct:
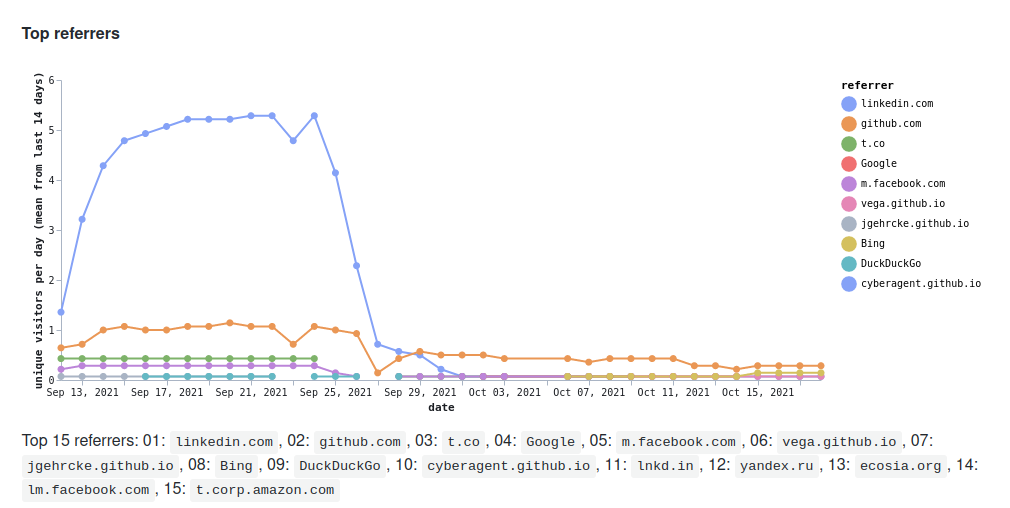
Whereas the expected range would be between Jan and Nov.
The text was updated successfully, but these errors were encountered: