-
Notifications
You must be signed in to change notification settings - Fork 64
New issue
Have a question about this project? Sign up for a free GitHub account to open an issue and contact its maintainers and the community.
By clicking “Sign up for GitHub”, you agree to our terms of service and privacy statement. We’ll occasionally send you account related emails.
Already on GitHub? Sign in to your account
Time encoder implementation #22
Comments
The temporal encoding is managed by the AgentFormer/model/agentformer.py Lines 33 to 99 in e4fe8dd
Hope this helps! |
Sign up for free
to join this conversation on GitHub.
Already have an account?
Sign in to comment
Hi, I really like your work in dealing with multi-agent trajectories prediction. I went through the paper and codes and popped up a quick question about the time encoder. As you mentioned in the paper, the time encoder that integrated the timestamp features differs from the original positional encoder. But I cannot find the time encoder codes in this repo. Please let me know if I missed anything. Much appreciated!
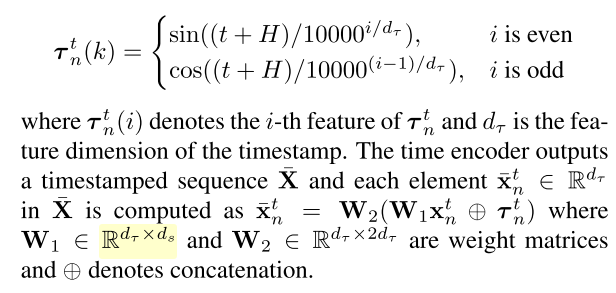
The text was updated successfully, but these errors were encountered: