You signed in with another tab or window. Reload to refresh your session.You signed out in another tab or window. Reload to refresh your session.You switched accounts on another tab or window. Reload to refresh your session.Dismiss alert
Hi, thanks for creating this repo. I have been using your ppo_continuous code for training a robot in ROS. I am training it for 2500 episodes with 400 max time steps and also updating the weights after 400 steps with K_epochs as 80. I strangely find that after each update step there is a spike in the RAM memory. Although this shouldn't be happening because after the update step the clear_memory() gets called which basically deletes all the previous states, actions, log_probs, rewards and is_terminals. And also, in the K_epoch the gradients are made zero for K iterations.
Can you please provide a solution for this?
Hey, did you make sure that the length of the of memory is 400 and the clear_memory() is getting called at each update? maybe print it before updating ? because that is the only variable that can stack memory over episodes.
Also, I see that you have modified the code to add some logging variables, maybe that is causing the problem ? check if the problem persists while running it with the same code as in the repository, by changing the environment only.
If not that, then I am not sure what might cause this.
Okay i figured it out, it was that i was appending the losses for plotting the graphs. But I was appending also all the gradients with it, which was the main cause for the memory. So I did .append(loss.item()) which stores only the values and not the gradients.
Hi, thanks for creating this repo. I have been using your ppo_continuous code for training a robot in ROS. I am training it for 2500 episodes with 400 max time steps and also updating the weights after 400 steps with K_epochs as 80. I strangely find that after each update step there is a spike in the RAM memory. Although this shouldn't be happening because after the update step the clear_memory() gets called which basically deletes all the previous
states, actions, log_probs, rewards and is_terminals
. And also, in the K_epoch the gradients are made zero for K iterations.Can you please provide a solution for this?
Have a look at this figure
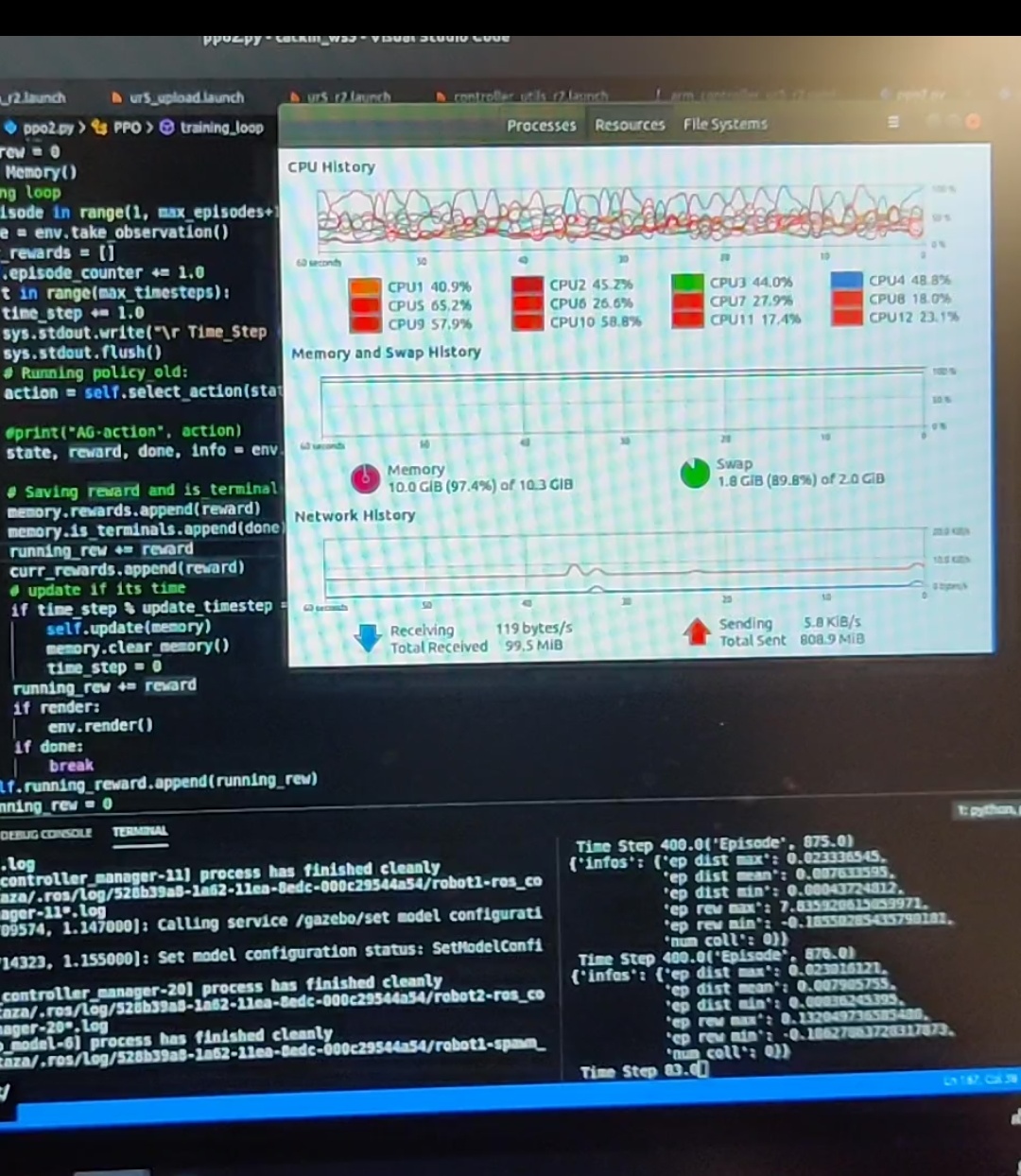
There is a spike in memory after every update
I have briefly described this issue on stack overflow as well
https://stackoverflow.com/q/59281995/10879393
The text was updated successfully, but these errors were encountered: