You signed in with another tab or window. Reload to refresh your session.You signed out in another tab or window. Reload to refresh your session.You switched accounts on another tab or window. Reload to refresh your session.Dismiss alert
going through the RL Intro I stumbled something that is not yet clear to me. On https://spinningup.openai.com/en/latest/spinningup/rl_intro.html#the-rl-problem
for the expected return it says
.. math:: J(\pi) = \int_{\tau} P(\tau|\pi) R(\tau) = \underE{\tau\sim \pi}{R(\tau)}
where I would have expected
.. math:: J(\pi) = \int_{\tau} P(\tau|\pi) R(\tau) = \underE{\tau\sim P}{R(\tau)}
Hi, @aflgit. I'll try to explain how I understand this:
P does determine the next state of the environment (based on previous state and the action taken), therefore you have P(s_t+1 | s_t, a_t).
However, the expression you highlight reflects the expected outcome of a trajectory by an agent that follows a given policy pi, i.e. sampling from it. The fact that the trajectory is indeed affected by other factors like P is simply implicit in the expression.
Hi,
going through the RL Intro I stumbled something that is not yet clear to me. On https://spinningup.openai.com/en/latest/spinningup/rl_intro.html#the-rl-problem
for the expected return it says
.. math:: J(\pi) = \int_{\tau} P(\tau|\pi) R(\tau) = \underE{\tau\sim \pi}{R(\tau)}
where I would have expected
.. math:: J(\pi) = \int_{\tau} P(\tau|\pi) R(\tau) = \underE{\tau\sim P}{R(\tau)}
My understanding is that \tau is a RV distributed with respect to P, and only the actions are taken from \pi, as later clearly differentiated on https://spinningup.openai.com/en/latest/spinningup/rl_intro.html#bellman-equations
Please, can someone explain me why it says \tau\sim \pi?
Thank you very much in advance!
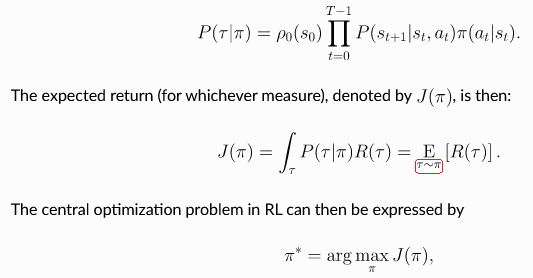
The text was updated successfully, but these errors were encountered: