-
Notifications
You must be signed in to change notification settings - Fork 9
New issue
Have a question about this project? Sign up for a free GitHub account to open an issue and contact its maintainers and the community.
By clicking “Sign up for GitHub”, you agree to our terms of service and privacy statement. We’ll occasionally send you account related emails.
Already on GitHub? Sign in to your account
ph5toms memory usage #100
Comments
Still running some tests. When I run a really big request, say for 10GB of data it more clear that memory usage is going up by the amount of data yielded each time it yields an obspy stream. I have already added some stuff to clear out ph5 tables after they are done being used. That helped a little but they really aren't that big. I'm investigating why the yielded object or data related to it isn't being garbage collected automatically after it is done being yielded. At least that is my theory so far. Using mprof and guppy right now. |
I think you may be on to something. The memory might not be freed until stop iteration is reached. See the following stack overflow posting: |
Yeah so it looks like while the iteration is going through no garbage collection can happen anywhere in the script. I tried clearing out and deleting all the tables as soon as they are un needed but mprof shows that it actually doesnt do that until the end of the script even if i explicitly tell it to with gc.collect() |
One solution I will look into is multiprocessing to see if that allows proper garbage collection |
I also read about how you can potentially force garbage collection by running memory intensive processes in a separate thread. Once the thread is finished running, the memory is freed. Do we know what is using so much memory? It seems like the first step is to figure out exactly what is causing the spike in memory usage, before figuring out a fix. |
I believe it is the yielded obspy stream not being collected. When I run it on large data sets it looks like it is jumping by the size of the obspy stream every time. A little bit of it also looks like the das_t. I added a line in in create_trace to free that memory every time it is done with a das_t but it currently doesn't do anything since garbage collection is halted. I also added code to clear all other tables when they are done but those only amount to < 10MB total memory. |
I am going to test this by modifying the script on my local machine to immediately write out the trace object instead of yielding it to see how that changes things, and to get a better idea of what is going on. |
I wonder if we have too many open HDF5 dataspaces that are causing a large memory leak: The HDF5 docs state the following:
@rsdeazevedo Do you think this could be the case? |
I don't think thats the case. I now explicitly close and delete each table after it is read. Well I try to at least, but it wont garbage collect and free the memory until the counter on the iterator is 0. Removing yielding completely fixes the problem. I'm trying to get it to work while still yielding the final stream object. I think that will work once I rewrite th1e code that yields the stations to cut to no longer yield. I think the issue is happening because we have two iterators going at the same time. I think we changed it to yield those to speed up the time to yield the first stream object. This code change will make it take a little longer until it yields the first stream object but I'm going to try to minimize that. That does remind me while fixing ph5tostationxml I will also update ph5tostationxml to free the table memory as soon as it can |
Thanks Derick the nested yielding very well could be the cause of the issue. Anther improvement I want to make is to have the For example, currently the ObsPy Fed Catalog client makes POST requests, formatted like the example below, that can be hundreds of lines.
Long requests currently time-out largely because we process each request independently (performing the same work of extracting all stations/channels more than one time). Adding support for lists of requests to the ph5tostationxml.py API will fix this problem since large amounts of data will only have to be read from each requested network one time. |
OKay got ph5toms fixed. Going to add some more memory cleanup to see if I can get it even lower and make sure its cleaning up everywhere possible. I'll create a PR in a bit. The issue was the nested yields. Getting rid of the yield in the cut station allowed it to garbage collect after every stream is yielded |
adressed in PR #108 |
ph5toms uses a lot of memory (>1Gb per request). This affects our ability to scale the web services. We need to work on making this this more efficient.
The memory usage graph looks like this for the following request on my local machine:
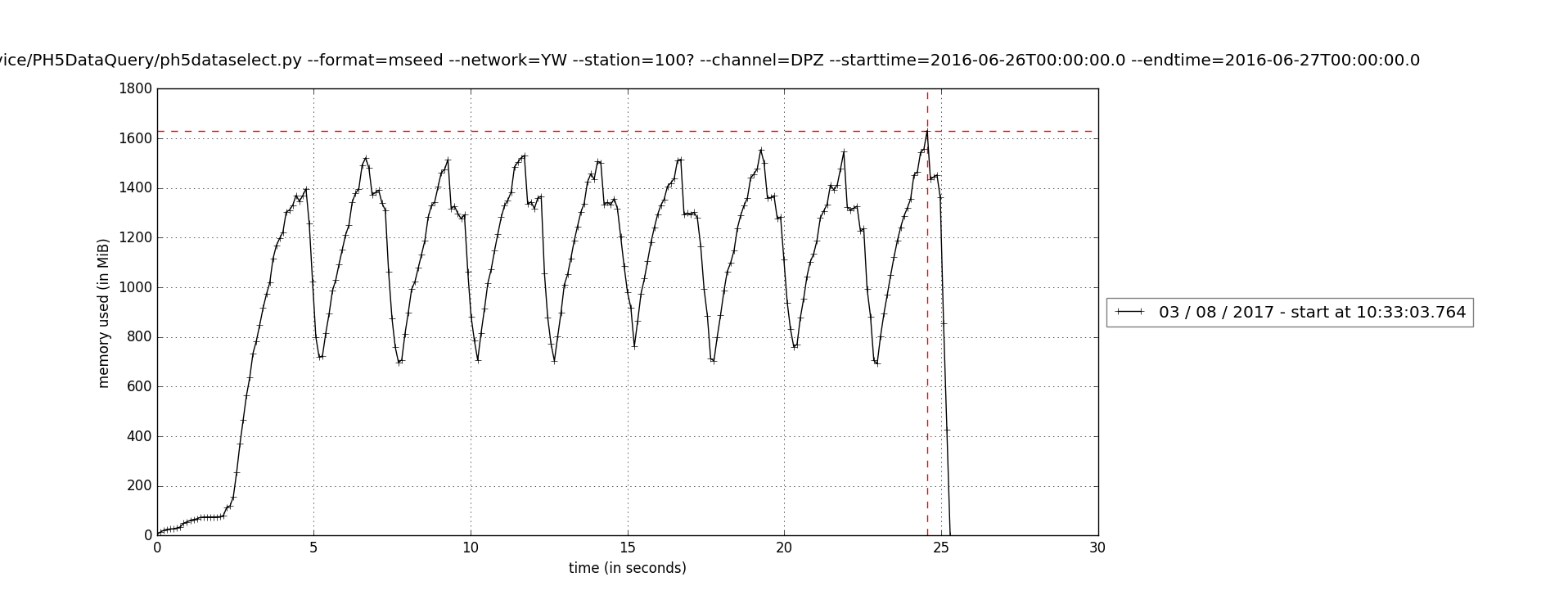
mprof run --include-children ph5toms --nickname master --ph5path /hdf5-data2/PH5_Experiments/pn4/16-015/ -o . --station 100? --channel DPZ --starttime 2016-06-26T00:00:00.0 --stoptime 2016-06-27T00:00:00.0
The text was updated successfully, but these errors were encountered: