New issue
Have a question about this project? Sign up for a free GitHub account to open an issue and contact its maintainers and the community.
By clicking “Sign up for GitHub”, you agree to our terms of service and privacy statement. We鈥檒l occasionally send you account related emails.
Already on GitHub? Sign in to your account
DistributedDataParallel: GRU module gets additional processes on GPU 0 (1st GPU) and takes more memory #70404
Comments
Hi, I am also experiencing similar problem when using DDP. I find that large layer would significantly slow down the DDP training. And I can not figure out why. |
duplicate of #66203 |
Ok, got it . I think my problem is due to hardware. Because my computer case is too small to hold 2 RTX3090, so I use extension line, and this causes much IO block. Thanks so much for giving kind advice. |
@Hannibal046 sorry, I was replying to @chgwan. Your problem doesn't seem to be related to this issue, and the workaround also doesn't apply. |
It is ok. I also learn an elegant and efficient way to put the model and data in the right place. |
@ngimel Thank you very much for your elegant solution. It works well. def demo_basic(rank, world_size):
print(f"Running basic DDP example on rank {rank}.")
dist.init_process_group("nccl", rank=rank, world_size=world_size)
torch.cuda.set_device(rank)
ToyModel = nn.GRU(10, 10, 1)
model = ToyModel.cuda()
ddp_model = DDP(model, device_ids=[rank])
pbar_len = int(1e10 / 2)
for _ in range(pbar_len):
input_seq = torch.randn(4, 20,10)
input_seq = input_seq.float().cuda()
ddp_model(input_seq)
dist.destroy_process_group() |
馃悰 Describe the bug
Hi, Thank you for your concern. When I am testing a simple example with DistributedDataParallel, using a single node with 4 gpus, I found that when I used the GRU or LSTM module was taking additional processes and more memory on GPU 0. while using the Linear module was not gotten these problems. The test code snippets are as follows:
I called the script like python XX.py. And I got GRU and Linear modules results are as follows:
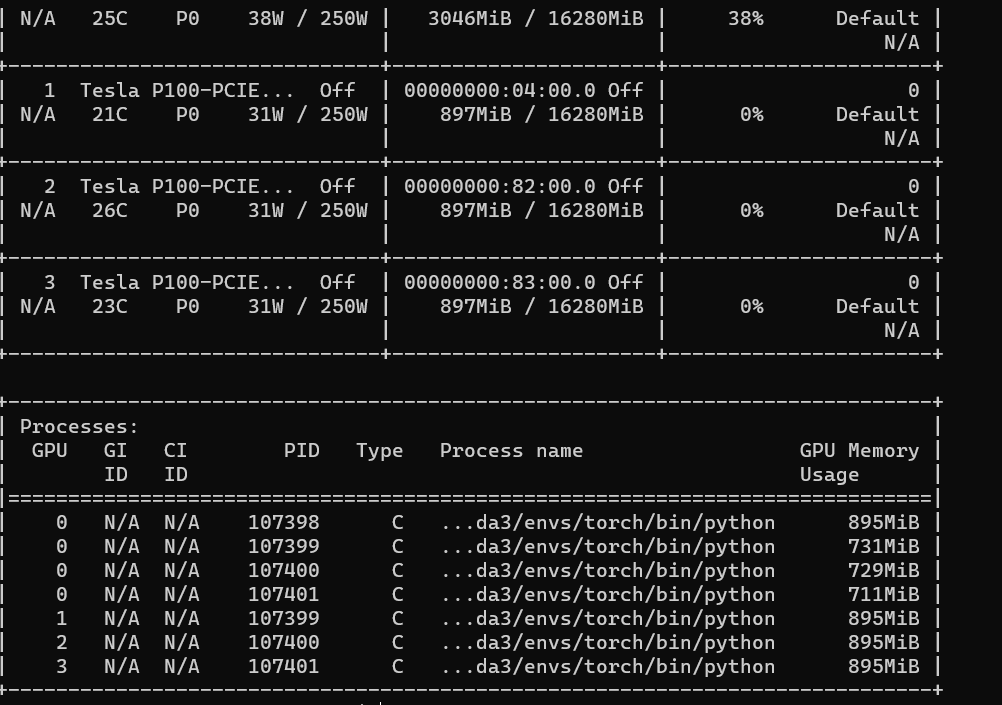
GRU module result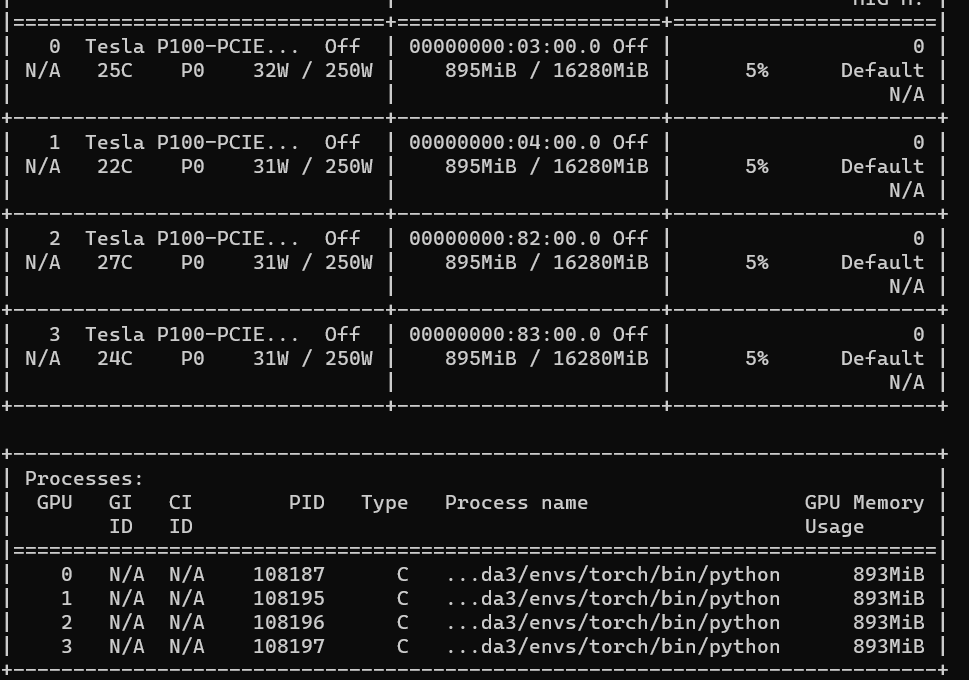
Linear module resultVersions
Collecting environment information...
PyTorch version: 1.10.0
Is debug build: False
CUDA used to build PyTorch: 10.2
ROCM used to build PyTorch: N/A
OS: CentOS Linux release 7.7.1908 (Core) (x86_64)
GCC version: (GCC) 4.8.5 20150623 (Red Hat 4.8.5-44)
Clang version: 3.4.2 (tags/RELEASE_34/dot2-final)
CMake version: Could not collect
Libc version: glibc-2.17
Python version: 3.9.7 (default, Sep 16 2021, 13:09:58) [GCC 7.5.0] (64-bit runtime)
Python platform: Linux-3.10.0-514.el7.x86_64-x86_64-with-glibc2.17
Is CUDA available: True
CUDA runtime version: Could not collect
GPU models and configuration:
GPU 0: Tesla P100-PCIE-16GB
GPU 1: Tesla P100-PCIE-16GB
GPU 2: Tesla P100-PCIE-16GB
GPU 3: Tesla P100-PCIE-16GB
Nvidia driver version: 460.27.04
cuDNN version: Could not collect
HIP runtime version: N/A
MIOpen runtime version: N/A
Versions of relevant libraries:
[pip3] mypy-extensions==0.4.3
[pip3] numpy==1.21.2
[pip3] torch==1.10.0
[pip3] torch-tb-profiler==0.1.0
[pip3] torchaudio==0.10.0
[pip3] torchinfo==1.5.4
[pip3] torchvision==0.11.1
[conda] blas 1.0 mkl defaults
[conda] cudatoolkit 10.2.89 hfd86e86_1 defaults
[conda] ffmpeg 4.3 hf484d3e_0 pytorch
[conda] mkl 2021.4.0 h06a4308_640 defaults
[conda] mkl-service 2.4.0 py39h7f8727e_0 defaults
[conda] mkl_fft 1.3.1 py39hd3c417c_0 defaults
[conda] mkl_random 1.2.2 py39h51133e4_0 defaults
[conda] mypy_extensions 0.4.3 py39h06a4308_0 defaults
[conda] numpy 1.21.2 py39h20f2e39_0 defaults
[conda] numpy-base 1.21.2 py39h79a1101_0 defaults
[conda] pytorch 1.10.0 py3.9_cuda10.2_cudnn7.6.5_0 pytorch
[conda] pytorch-mutex 1.0 cuda pytorch
[conda] torch-tb-profiler 0.1.0 pypi_0 pypi
[conda] torchaudio 0.10.0 py39_cu102 pytorch
[conda] torchinfo 1.5.4 pyhd8ed1ab_0 conda-forge
[conda] torchvision 0.11.1 py39_cu102 pytorch
cc @zou3519 @ngimel @pietern @mrshenli @pritamdamania87 @zhaojuanmao @satgera @rohan-varma @gqchen @aazzolini @osalpekar @jiayisuse @SciPioneer @H-Huang
The text was updated successfully, but these errors were encountered: