-
Notifications
You must be signed in to change notification settings - Fork 9
New issue
Have a question about this project? Sign up for a free GitHub account to open an issue and contact its maintainers and the community.
By clicking “Sign up for GitHub”, you agree to our terms of service and privacy statement. We’ll occasionally send you account related emails.
Already on GitHub? Sign in to your account
CUDA out of memory issue when training the model with single GPU #12
Comments
Same issue here |
We used GeForce RTX 2080 with 8 GB RAM. In this case, I recommend that you use smaller batch size/sample_per_gpu size, which can be defined rugd_group6.py or ganav_group6_rugd.py. However, RELLIS-3D is using sample_per_gpu size=1, so I'm not sure whether it can be run on a Nvidia RTX 2060. Another way is to reduce the crop size, which means you might not able to use the released trained model. |
Thank you sir for your quick reply regarding this issue. I reduced the samples_per_gpu parameter in GANav-offroad/configs/ours/ganav_group6_rugd.py as per your suggestion. The above issue was still persisting for 2 or 3 samples_per_GPU, but fortunately, training successfully commenced when samples_per_gpu=1.
The training phase progressed till the 10% checkpoint at which point it produced another error as below: I googled and found a solution to the error which was to set priority of DistEvalHook to LOW in \mmseg\apis\train.py. But I observed that the evaluation metrics at each checkpoint were not improving throughout the training and stayed the same as that of the 1st checkpoint. The evaluation metrics for all checkpoints were as given below: Evaluation results from the Testing phase using the trained model produced similar results as well. I am not sure what is causing this issue, and I have not altered any other parameters in the repository. Hence could you suggest what may be causing this issue? |
Have you checked the number of classes? Since its prediction is all 0 (background), I image there is something wrong with the setup.
|
To sum up:
The current version of the code corresponds to this version of the paper(https://arxiv.org/abs/2103.04233v2), which is not the latest version that was described in the accepted RAL paper. I will update the code in the next couple of days and see if there are any obvious bugs that might cause this issue. |
Hey just a quick note, I just uploaded the new code for the latest paper. Please start another issue if you still have training problems. |
Hello Sir. Firstly, thanks for your contribution through this work.
I would to enquire about the GPU Requirements when training the GANav model, especially when using a single GPU. I am attempting to train the model using a single GPU (Nvida RTX 2060) but I am facing the error : Runtime Error: CUDA Out of Memory.
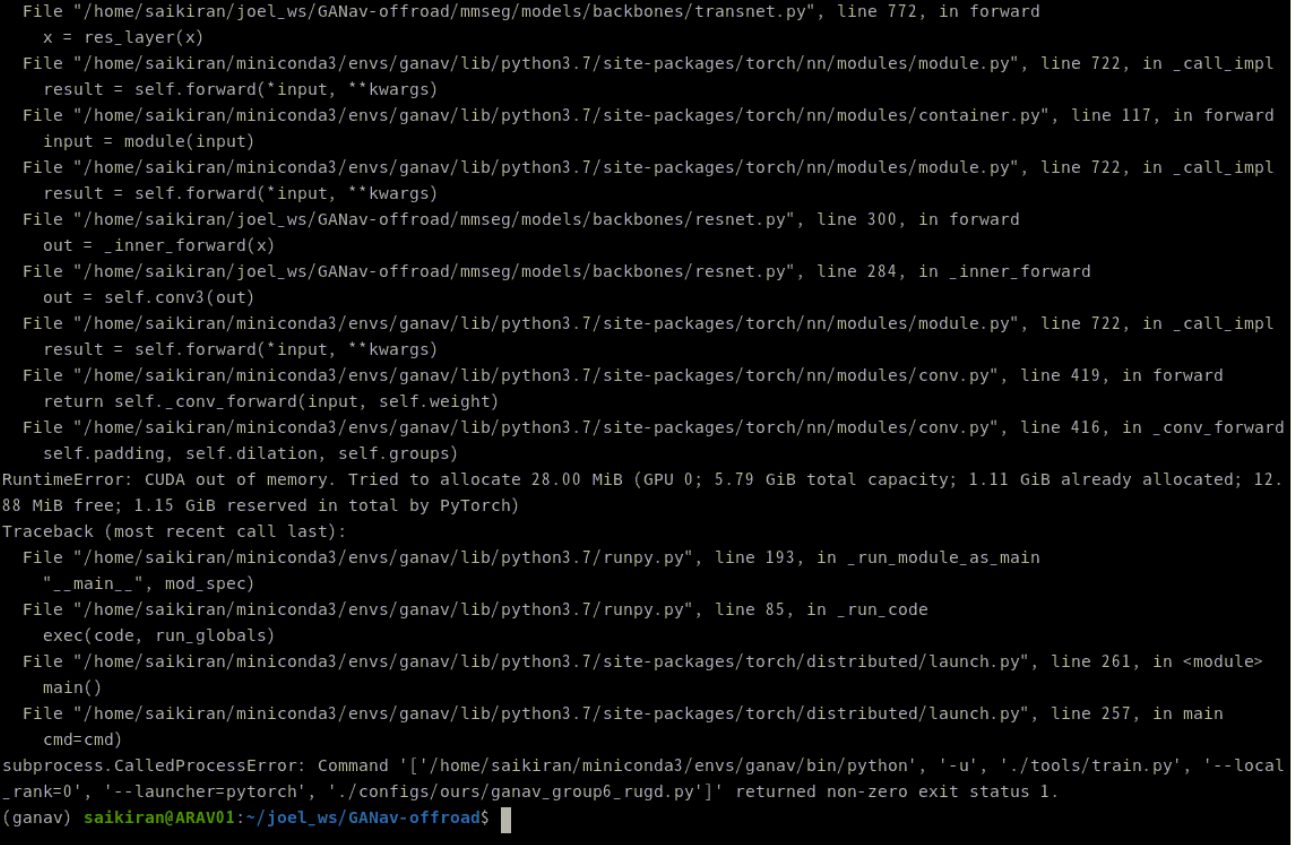
To be more specific, I am running the following code after setting up the GANav environment and processing the dataset as per readme instructions:
python -m torch.distributed.launch ./tools/train.py --launcher='pytorch' ./configs/ours/ganav_group6_rugd.py
and I am facing the error below:
PC specs and package versions & configuration used in environment for GANav:
GPU: Nvidia RTX 2060
CPU: AMD Ryzen 7 3700X
Python Version: 3.7.13
Pytorch version: 1.6.0
cudatoolkit version:10.1
mmcv-full version: 1.6.0
Dataset used: RUGD Dataset
No. of Annotation Groups: 6
Also, can you suggest some workarounds for memory management when training the model using a single GPU.
Thanks.
The text was updated successfully, but these errors were encountered: