CausalTree split criterions fix and fit optimization #557
Add this suggestion to a batch that can be applied as a single commit.
This suggestion is invalid because no changes were made to the code.
Suggestions cannot be applied while the pull request is closed.
Suggestions cannot be applied while viewing a subset of changes.
Only one suggestion per line can be applied in a batch.
Add this suggestion to a batch that can be applied as a single commit.
Applying suggestions on deleted lines is not supported.
You must change the existing code in this line in order to create a valid suggestion.
Outdated suggestions cannot be applied.
This suggestion has been applied or marked resolved.
Suggestions cannot be applied from pending reviews.
Suggestions cannot be applied on multi-line comments.
Suggestions cannot be applied while the pull request is queued to merge.
Suggestion cannot be applied right now. Please check back later.
Proposed changes
Hi, this PR contains several fixes for causal trees issues I have recently found:
It turned out that
sum_total
andsq_sum_total
attributes inRegressionCriterion
were calculated using weights as multipliers. However, in CausalTree these weights have two values: eps for control, 1 for treatment. So, total outcome values for a tree split were incorrect. Link: _criterion.pyxThere was incorrect calculation of
weighted_n_node_samples
attribute inRegressionCriterion
. It is important to get the right impurity values and feature importance. Links: _criterion.pyx, _tree.pyxThere is a more efficient way of calculating node and children impurity for each split.
CausalTree
fit()
measurements clearly show this:Additional code for time measurements: test.zip
Related issue: #541.
So, I added
NodeInfo
andSplitState
structs incriterion.pyx
for clarity and updatedCausalRegressionCriterion
. These steps allowed me to get rid of the loops inCausalMSE
and even make this class more concise.Curiously, the qini score with synthetic data has slightly changed since this update.
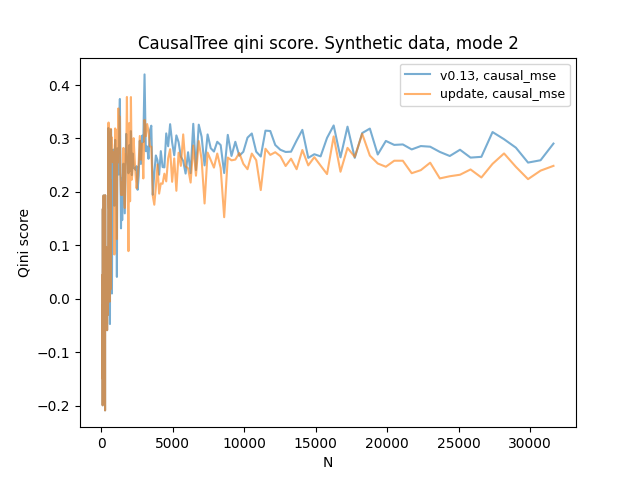
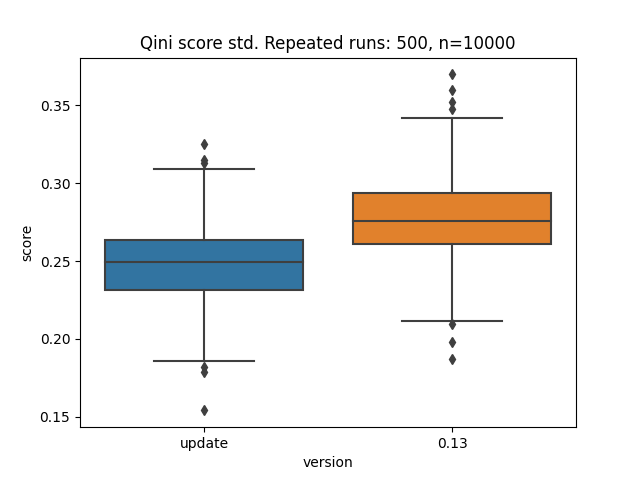
Additional updates:
conftest.py
to get the desired type of regression synthetic data in any test file.Types of changes
What types of changes does your code introduce to CausalML?
Put an
x
in the boxes that applyChecklist
Put an
x
in the boxes that apply. You can also fill these out after creating the PR. If you're unsure about any of them, don't hesitate to ask. We're here to help! This is simply a reminder of what we are going to look for before merging your code.Further comments