-
Notifications
You must be signed in to change notification settings - Fork 192
New issue
Have a question about this project? Sign up for a free GitHub account to open an issue and contact its maintainers and the community.
By clicking “Sign up for GitHub”, you agree to our terms of service and privacy statement. We’ll occasionally send you account related emails.
Already on GitHub? Sign in to your account
How can I get INT8 weight in my model? #58
Comments
Hi, Conv layers have properties int_weight and quant_weight_scale to extract the integer weights and their scale factor, activations have method quant_act_scale() to extract the scale factor. Mapping to any hardware is up to the user and it really depends on how the quantization was set up. Alessandro |
Hi, @volcacius Different scaling of output tensor cannot directly do add operation..? class Net(nn.Module):
def __init__(self):
super(Net, self).__init__()
self.c1 = Conv2d(in, out, 3, 1, 1)
self.c2 = Conv2d(out, out, 3, 1, 1)
def forward(self,x):
r = self.c1(x)
x = self.c2(r)
return r+x |
Hello, Yes this a typical problem to address. You have two main strategies. class QuantNet(nn.Module):
def __init__(self, in_ch, out_ch):
super(Net, self).__init__()
self.shared_quant = QuantHardTanh(
bit_width=8,
quant_type=QuantType.INT,
min_val=-1.0,# arbitrary
max_val=1.0,
return_quant_tensor=True)
self.c1 = QuantConv2d(
in_ch, out_ch, 3, 1, 1,
weight_bit_width=8,
weight_quant_type=QuantType.INT)
self.c2 = QuantConv2d(
out_ch, out_ch, 3, 1, 1,
weight_bit_width=8,
weight_quant_type=QuantType.INT)
def forward(self,x):
r = self.shared_quant(self.c1(x))
x = self.shared_quant(self.c2(r))
return r+x # you can check that r1 and r2 have the same scale factors The second strategy is to share a weight quantizer between multiple conv/fc layers. This applies to any scenario where you need to have weights with the same scale factor (along the whole layer or along corresponding output channels, depending on whatever you set weight_scaling_per_output_channel=True). class NetSharedWeightQuant(nn.Module):
def __init__(self, in_ch, out_ch):
super(Net, self).__init__()
self.inp_quant = QuantHardTanh(
bit_width=8,
quant_type=QuantType.INT,
min_val=-1.0, #arbitrary
max_val=1.0,
return_quant_tensor=True)
self.c1 = QuantConv2d(
in, out, 3, 1, 1,
weight_bit_width=8,
weight_quant_type=QuantType.INT,
compute_output_scale=True,
compute_output_bit_width=True,
return_quant_tensor=True)
self.c2 = QuantConv2d(
in, out, 3, 1, 1,
weight_quant_override=self.c1.weight_quant,
weight_quant_type=QuantType.INT, # this is not necessary when you are setting weight_quant_ovveride, but otherwise [this check](https://github.com/Xilinx/brevitas/blob/d9b1e355299abd5fe0e4a5527c1f69b8371fb121/brevitas/nn/quant_conv.py#L131) would fail
compute_output_scale=True,
compute_output_bit_width=True,
return_quant_tensor=True)
def forward(self,inp):
x = self.inp_quant(inp)
r1 = self.c1(x)
r2 = self.c2(x)
return r1+r2 # you can check that r1 and r2 have the same scale factors Depending on the topology you can combine the two strategies. The good thing is that in both cases, learned scale factors adapt to the fact that they are used in multiple places. |
Hi @volcacius, thanks for the clear example. I still have a problem with a small difference between the first strategy and proxylessnas implementation. The first strategy applies shared_quant to r and x respectively and add them. But in the proxylessnas implementation, you add r and x first and then apply the shared_quant. I think when you add r and x first, their scale factors are different so I'm confused. Can you explain it to me? Thanks! |
Hello, The proxylessnas implementation is doing what the first strategy is doing, it's just the way the code it's organized that makes it harder to see. Alessandro |
Hi @volcacius, thanks for your explanation and I am now understood. I have another question about the data flow inside brevitas. I found that in the conv layer, what brevitas do is convert conv weight to quanted value and multiply it with the input. The scale of input seems not evolved in the computation. Besides QuantTensor has a Tensor and it is a float point unquantized value. So I was thinking if I just want to see the effect of quantization on the accuracy and not care about the speedup, can I only feed pytorch tensor to each operation and leave tensor scale away? And the scale problem in this issue is no longer a problem. |
Hi, Yes, you don't have to use quant tensors if you don't want to. For quantized weights and activations, they are not required. In QuantConv2d and QuantLinear, you can set Alessandro |
I am still having some issues while implementing MobilenetV2 (passing shared quantizers ), Can you share your model code. Pritesh |
Hi there,
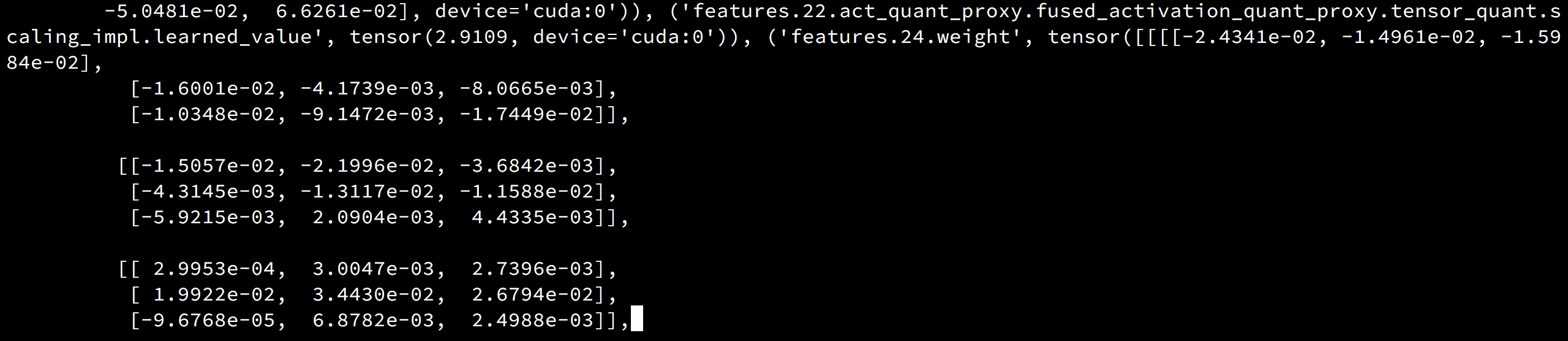
I'm trying to train a vgg16 model (use the vgg16 provided from
brevitas/examples/imagenet_classification/models/vgg.py
, and setting is following thecommon.py
) on our own datasets, and the model has trained well.I look the code in
brevitas/examples/imagenet_classification/models/common.py
and find
line7 QUANT_TYPE = QuantType.INT
Can I regard the weight in qnn.QuantConv2d will be INT type?
But when I load the model.pt(my saving model weight, using torch.load), I get the weight just like this:
How can I get the INT8 weights in my models, and how to use the weight I got to do inference on FPGA? Just directly port my weight to my VGG design on FPGA or I need to add some scaling step or something...?
The text was updated successfully, but these errors were encountered: