Commit
This commit does not belong to any branch on this repository, and may belong to a fork outside of the repository.
Rnd 102 Release CF module v1 for open source (#1)
Restructured, README, LICENSE, and format check
- Loading branch information
1 parent
eb9b5b7
commit 61e733c
Showing
15 changed files
with
1,130 additions
and
0 deletions.
There are no files selected for viewing
This file contains bidirectional Unicode text that may be interpreted or compiled differently than what appears below. To review, open the file in an editor that reveals hidden Unicode characters.
Learn more about bidirectional Unicode characters
Original file line number | Diff line number | Diff line change |
---|---|---|
@@ -0,0 +1,11 @@ | ||
Acai | ||
|
||
Copyright (c) BerryAI | ||
|
||
The MIT License | ||
|
||
Permission is hereby granted, free of charge, to any person obtaining a copy of this software and associated documentation files (the "Software"), to deal in the Software without restriction, including without limitation the rights to use, copy, modify, merge, publish, distribute, sublicense, and/or sell copies of the Software, and to permit persons to whom the Software is furnished to do so, subject to the following conditions: | ||
|
||
The above copyright notice and this permission notice shall be included in all copies or substantial portions of the Software. | ||
|
||
THE SOFTWARE IS PROVIDED "AS IS", WITHOUT WARRANTY OF ANY KIND, EXPRESS OR IMPLIED, INCLUDING BUT NOT LIMITED TO THE WARRANTIES OF MERCHANTABILITY, FITNESS FOR A PARTICULAR PURPOSE AND NONINFRINGEMENT. IN NO EVENT SHALL THE AUTHORS OR COPYRIGHT HOLDERS BE LIABLE FOR ANY CLAIM, DAMAGES OR OTHER LIABILITY, WHETHER IN AN ACTION OF CONTRACT, TORT OR OTHERWISE, ARISING FROM, OUT OF OR IN CONNECTION WITH THE SOFTWARE OR THE USE OR OTHER DEALINGS IN THE SOFTWARE. |
This file contains bidirectional Unicode text that may be interpreted or compiled differently than what appears below. To review, open the file in an editor that reveals hidden Unicode characters.
Learn more about bidirectional Unicode characters
Original file line number | Diff line number | Diff line change |
---|---|---|
@@ -0,0 +1,117 @@ | ||
# Music Recommendation by BerryAI | ||
This project is a music recommendation system developed by BerryAI. | ||
## Getting Started | ||
These instructions will get you a copy of the project up and running on your | ||
local machine for development and testing purposes. See deployment for notes | ||
on how to deploy the project on a live system. | ||
### Prerequisites Software | ||
What things you need to install the software and how to install them | ||
``` | ||
Python | ||
Python-NumPy | ||
``` | ||
#### Linux | ||
All major distributions of Linux provide packages for both Python and NumPy. | ||
#### Mac OS X | ||
``` | ||
pip install numpy | ||
``` | ||
#### Windows | ||
Personally, I will recommend Anaconda as default Python compiler. To install | ||
them, go to page | ||
``` | ||
https://www.continuum.io/downloads | ||
``` | ||
and find the proper install packages | ||
|
||
### Prerequisites Dataset | ||
In this project, we use some public open database, and they are | ||
|
||
* Last.fm 1k user data [download](http://mtg.upf.edu/static/datasets/last.fm/lastfm-dataset-1K.tar.gz) | ||
* Million Song Database[download](http://labrosa.ee.columbia.edu/millionsong/sites/default/files/AdditionalFiles/unique_tracks.txt) | ||
* Million Song Database subset [download](https://drive.google.com/file/d/0B7s9m90eW6dtMnk5Q2M1aFBfeDA/view?usp=sharing) | ||
* Echo Nest user data [download](http://labrosa.ee.columbia.edu/millionsong/sites/default/files/challenge/train_triplets.txt.zip) | ||
|
||
For convenience purpose, I have calculate the intersection between 1k user data | ||
and MSD Database. [HERE](https://drive.google.com/open?id=0B7s9m90eW6dtX084eTNXQ2NLblU) | ||
is the download link. | ||
|
||
### Installation | ||
|
||
|
||
## Usage | ||
Test functions are under ./test folder. After downloading all the data files, | ||
please put the extracted files into ./data folder. | ||
|
||
Then run | ||
``` | ||
python test_cf_hf_gd.py | ||
``` | ||
in command line under the directory of the project installed. | ||
|
||
## Algorithms included: | ||
|
||
### Collaborative Filtering Methods | ||
|
||
#### * Memory based recommendation | ||
|
||
The recommendation equation is: <br /> | ||
 | ||
|
||
Where U is the full set of all users,  is | ||
user u's rating score of item | ||
i, and  is the average rating score for user | ||
u. For similarity function , we have two | ||
approaching ways: | ||
|
||
1. K nearest neighbours: <br /> | ||
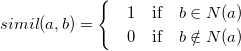 | ||
Where  is the set of neighbors of user a. | ||
2. Pearson Correlation: <br /> | ||
 | ||
|
||
#### * Matrix Factorization and Hidden Features | ||
We could use much smaller dimension matrix P, Q to represent and approximate the | ||
full rating score matrix R. That is: <br /> | ||
 | ||
|
||
Normally, we have two different approaches: | ||
|
||
1. Singular Value Decomposition | ||
|
||
R is a m*n matrix | ||
 | ||
|
||
Where: | ||
* M is m*m unitary matrix | ||
* Σ is m*n diagonal matrix with singular values | ||
* N is n*n unitary matrix | ||
|
||
With first k singular values, we could approximate R as: <br /> | ||
 | ||
|
||
Then: <br /> | ||
 | ||
|
||
2. Gradient Descent | ||
We try to minimize the norm of residue matrix: <br /> | ||
 | ||
|
||
we have two different approaches: | ||
* Classic Gradient Descent: <br /> | ||
 | ||
|
||
* Stochastic Gradient Descent with momentum: <br /> | ||
 | ||
|
||
Both methods will converge, but please be careful choosing coefficients. | ||
|
||
## Contributing | ||
1. Fork it! | ||
2. Create your feature branch: `git checkout -b my-new-feature` | ||
3. Commit your changes: `git commit -am 'Add some feature'` | ||
4. Push to the branch: `git push origin my-new-feature` | ||
5. Submit a pull request :D | ||
|
||
## License | ||
The OpenMRS source code and binaries are released under the [MIT license](../LICENSE.md) |
Empty file.
Oops, something went wrong.