-
Notifications
You must be signed in to change notification settings - Fork 41
New issue
Have a question about this project? Sign up for a free GitHub account to open an issue and contact its maintainers and the community.
By clicking “Sign up for GitHub”, you agree to our terms of service and privacy statement. We’ll occasionally send you account related emails.
Already on GitHub? Sign in to your account
[20211030] Weekly AI ArXiv 만담 #28
Comments
|
행사 소개
|
논문들
[1] An Open Review of OpenReview: A Critical Analysis of the Machine Learning Conference Review Process - rejected from ICLR2021 yet got a positive review: "The paper should be brought to the attention of the various committees and made available somehow at the conference and acknowledged as a useful publication."
Extra
[1] Bayesian Deep Learning and a Probabilistic Perspective of Generalization (NeurIPS 2020) 쓸데없는 논문
|
상-당한 뒷북 The Nobel Prize in Physics 2021 was awarded "for groundbreaking contributions to our understanding of complex systems" with one half jointly to Syukuro Manabe and Klaus Hasselmann "for the physical modelling of Earth's climate, quantifying variability and reliably predicting global warming" and the other half to Giorgio Parisi "for the discovery of the interplay of disorder and fluctuations in physical systems from atomic to planetary scales."
그냥 뭔가 이런 연구가 있었다는게 최신 deep learning과 large scale LM의 홍수에 잊혀지면 약간 슬플거 같애서 이렇게 소개해드려요,, (물론 저 홍수가 나쁘다는건 절대 아님다 ㅎㅎ) |
ISO/IEC JTC 1/SC 42(AI), 10월 총회 개최 완료 (Virtual Moscow, 10/18 ~ 10/30)https://www.iso.org/committee/6794475.html
FDA's Good Machine Learning Practice for Medical Device Development: Guiding Principles
Kicking off NIST AI Risk Management Frameworkhttps://www.nist.gov/news-events/events/2021/10/kicking-nist-ai-risk-management-framework 인공지능 전지역·전산업 확산 추진방향 수립 (10/29, 과기부, 4차산업혁명위원회 발표)http://www.aitimes.com/news/articleView.html?idxno=141256
The Coming Convergence of NFTs and Artificial Intelligence
Expert warns that artificial intelligence could soon be able to 'hack' human beingshttps://thehill.com/changing-america/respect/579196-expert-warns-that-artificial-intelligence-could-soon-be-able-to-hack
Bruno Maisonnier, founder and CEO of AI firm AnotherBrain
|
Facebook has gone~ Meta has come!!!!
State of AI Report 2021
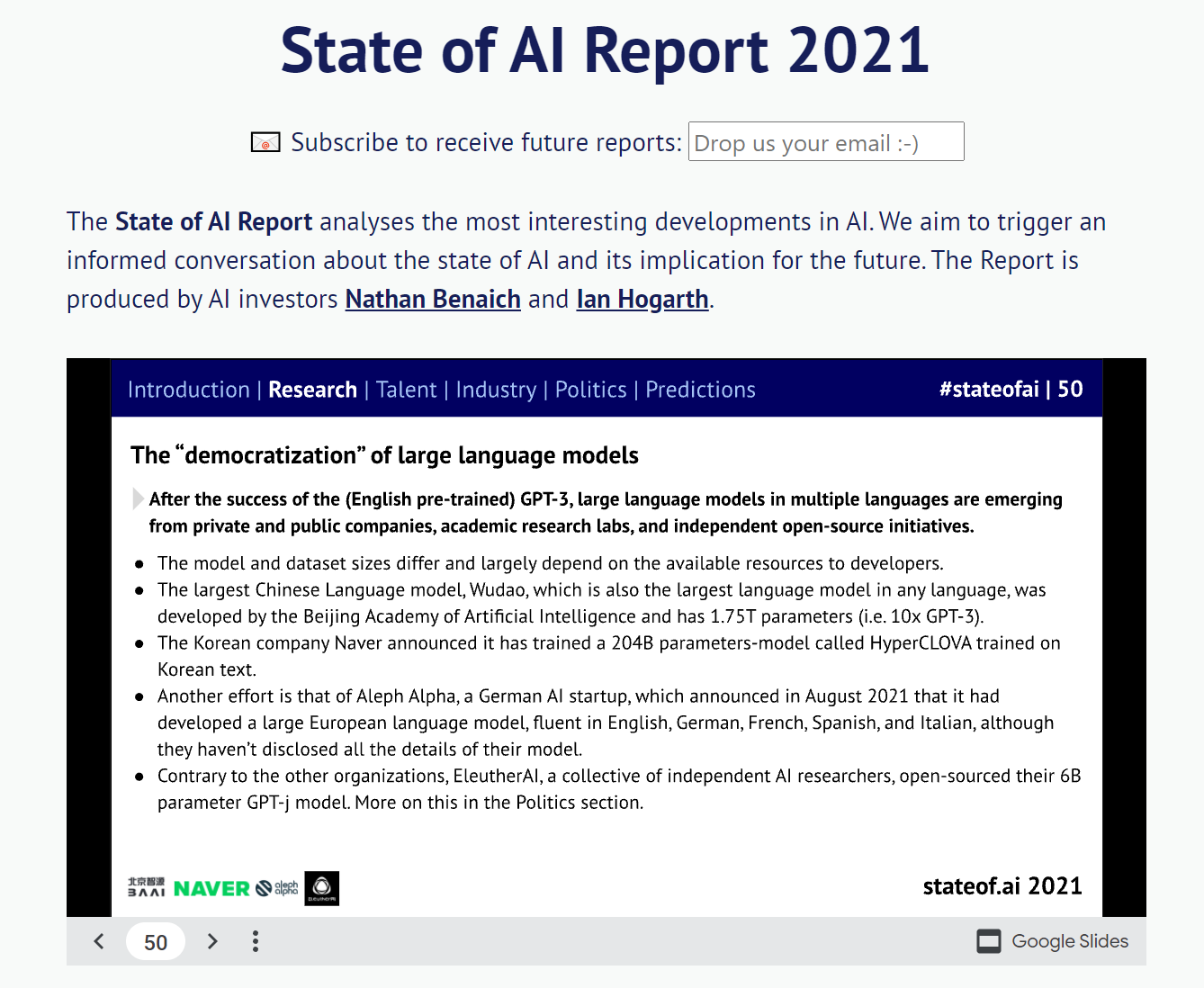
ICLR 2022 리뷰 데드라인 (사실상 화욜밤까지)
P-Tuning v2: Prompt Tuning Can Be Comparable to Fine-tuning Universally Across Scales and Tasks
Wav2CLIP: Learning Robust Audio Representations From CLIP
NormFormer: Improved Transformer Pretraining with Extra Normalization
Online Continual Learning on Class Incremental Blurry Task Configuration with Anytime Inference
Solving Math Word Problems
The text was updated successfully, but these errors were encountered: