You signed in with another tab or window. Reload to refresh your session.You signed out in another tab or window. Reload to refresh your session.You switched accounts on another tab or window. Reload to refresh your session.Dismiss alert
As part of my thesis project I have replicated your work in question retrieval using Tensorflow.
In your code you provide the loss for the training, as well as the performance for the dev set, but no loss for the dev set.
I have tried the following function:
def devloss0(labels, scores):
per_query_loss = []
for query_labels, query_scores in zip(labels, scores):
pos_scores = [score for label, score in zip(query_labels, query_scores) if label == 1]
neg_scores = [score for label, score in zip(query_labels, query_scores) if label == 0]
if len(pos_scores) == 0 or len(neg_scores) == 0:
continue
pos_scores = np.array(pos_scores)
neg_scores = np.repeat(np.array(neg_scores).reshape([1, -1]), pos_scores.shape[0], 0)
neg_scores = np.max(neg_scores, 1)
diff = neg_scores - pos_scores + 1.
diff = (diff > 0)*diff
per_query_loss.append(np.mean(diff))
return np.mean(np.array(per_query_loss))
but it seems to get increased after few batches, while the dev performance keeps increasing.
Only using the soft loss function that accounts for each pair loss i.e.
def devloss2(labels, scores):
query_losses = []
for query_labels, query_scores in zip(labels, scores):
pos_scores = [score for label, score in zip(query_labels, query_scores) if label == 1]
neg_scores = [score for label, score in zip(query_labels, query_scores) if label == 0]
if len(pos_scores) == 0 or len(neg_scores) == 0:
continue
pos_scores = np.array(pos_scores).reshape([-1, 1])
neg_scores = np.repeat(np.array(neg_scores).reshape([1, -1]), pos_scores.shape[0], 0)
diff = neg_scores - pos_scores + 1.
diff = (diff > 0).astype(np.float32)*diff
query_losses.append(np.mean(diff))
return np.mean(np.array(query_losses))
# example input to the functions:
# labels = [np.array([1,1,0,0,1,0,0,0]), np.array([1,1,0,0,1,0,0,0])]
# scores = [np.array([0.9,0.8,0.7,0.6,0.5,0.4,0.3,0.2]), np.array([0.9,0.8,0.7,0.6,0.5,0.4,0.3,0.2])]
does decrease the dev loss.
Do you know what's going on here? I suppose the model does not overfit, but is something that has to do with the data?
Results training a GRU model (devloss0 and devloss2 are called inside this function )
(showing MAP, MRR, P@1, P@5)
(showing devloss0 in green, devloss2 in light green and train loss in orange)
Hi @christinazavou
Sorry for such a late response. Not sure why I missed the notification of this post..
The observation is interesting. I didn't take a look at dev loss before.
I guess this is due to the difference of how training and evaluation datasets were constructed.
The training set is a combination of positive pairs + randomly sampled negative pairs;
The evaluation set is constructed in a re-ranking setup. That is, the 20 candidates of each query question were generated / retrieved by the Lucene search engine.
It is easy to separate random negative pairs from positive ones. So the training loss keeps decreasing. However, the dev set contains candidates that are much more similar to the query (because they are the outputs of a search engine). The devloss0 keeps at around the margin value (1.0), which suggests that there exists a challenging negative candidate not separated from the positive. devloss1 (pairwise loss) does decrease which suggests overall most negatives are gradually separated from the positive.
This is not an data issue i think. The training loss encourages the model to separate positives from average negative samples, not the most challenging negative samples.
Dear @taolei87 ,
As part of my thesis project I have replicated your work in question retrieval using Tensorflow.
In your code you provide the loss for the training, as well as the performance for the dev set, but no loss for the dev set.
I have tried the following function:
but it seems to get increased after few batches, while the dev performance keeps increasing.
Only using the soft loss function that accounts for each pair loss i.e.
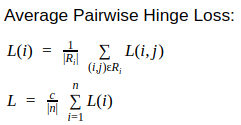
does decrease the dev loss.
Do you know what's going on here? I suppose the model does not overfit, but is something that has to do with the data?
Results training a GRU model (devloss0 and devloss2 are called inside this function )
(showing MAP, MRR, P@1, P@5)
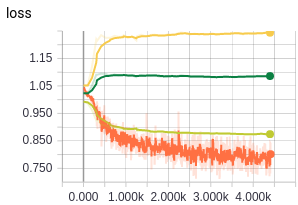
(showing devloss0 in green, devloss2 in light green and train loss in orange)
+-------+------+---------+---------+---------+---------+---------+---------+---------+---------+
| Epoch | Step | dev MAP | dev MRR | dev P@1 | dev P@5 | tst MAP | tst MRR | tst P@1 | tst P@5 |
+-------+------+---------+---------+---------+---------+---------+---------+---------+---------+
| 0 | 1 | 48.869 | 62.072 | 48.677 | 39.259 | 47.989 | 59.916 | 43.011 | 36.882 |
| 0 | 101 | 52.073 | 66.782 | 54.497 | 40.635 | 50.759 | 63.231 | 47.312 | 39.032 |
| 1 | 616 | 57.113 | 67.541 | 52.91 | 46.349 | 57.33 | 70.901 | 54.839 | 43.226 |
| 2 | 731 | 56.988 | 69.193 | 56.614 | 46.667 | 57.975 | 72.129 | 57.527 | 43.871 |
| 2 | 931 | 58.394 | 70.026 | 56.614 | 47.407 | 58.874 | 72.642 | 59.14 | 44.194 |
| 3 | 1046 | 58.385 | 70.601 | 58.201 | 46.878 | 59.244 | 74.14 | 61.29 | 44.194 |
| 4 | 1261 | 59.195 | 71.692 | 58.201 | 47.513 | 58.857 | 73.435 | 59.677 | 44.086 |
| 4 | 1361 | 58.408 | 71.76 | 58.201 | 47.831 | 58.423 | 71.093 | 55.914 | 44.086 |
| 5 | 1576 | 59.333 | 72.075 | 58.73 | 47.513 | 58.63 | 72.592 | 59.14 | 44.731 |
+-------+------+---------+---------+---------+---------+---------+---------+---------+---------+
The text was updated successfully, but these errors were encountered: