-
Notifications
You must be signed in to change notification settings - Fork 112
New issue
Have a question about this project? Sign up for a free GitHub account to open an issue and contact its maintainers and the community.
By clicking “Sign up for GitHub”, you agree to our terms of service and privacy statement. We’ll occasionally send you account related emails.
Already on GitHub? Sign in to your account
question about <mnist_minimal_example> #2
Comments
Hi, thank you for bringing this to our attention! Concerning the L2 regularization: this is included in the pytorch optimizer, so we do not have to implement it by hand. About the quality of results: You are right, they do not look good. I will investigate, and get back to you in a few days! |
I could reproduce the issue on my end, and I also found the problem: Thank you again for bringing our attention to the issue! If you want even better results, you can also increase the number of coupling blocks to 24, and train for 480 epochs (settings used in the paper, but takes several hours). I will close the issue, but feel free to reopen if there are further problems. |
@ardizzone Hi, I also test for style transfer experiments like Fig.(7), so I modify the eval.py into style_transfer.py and result like this: I think in this configuration the cINN could not decompose into 10 essentially separate subnetworks.. because in this cause, some label is not generate image |
Hi, very interesting! For most of your samples it works fine, but as you said, some don't work at all. I just pushed the actual research code for the mnist task, used to produce the paper (some documentation and comments were still missing): 8ea0165 I also included a model checkpoint (see the readme in the experiments/mnist_cINN folder). The model from the paper is slightly larger and trained for longer, you can test the style transfer by running eval.py in the experiments/mnist_cINN folder. I am confident it can be made much smaller and more efficient using convolutional layers, instead of all fully connected, I may update in the future (or you can experiment yourself). |
@ardizzone Ok,thank you very much . when I test mnist experiments, I will check convolutional layers experiments like : colorization_minimal_experiment and colorization_cINN. Thanks for your sharing again. and I will learn more for this. |
Hi, , thank you for your sharing , I am learning your method INN. and I can not understand the demo(mnist_minimal_example) loss ? what is the meaning?? I have ever seen before.
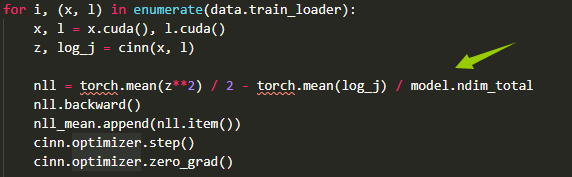
The text was updated successfully, but these errors were encountered: