-
Notifications
You must be signed in to change notification settings - Fork 26
New issue
Have a question about this project? Sign up for a free GitHub account to open an issue and contact its maintainers and the community.
By clicking “Sign up for GitHub”, you agree to our terms of service and privacy statement. We’ll occasionally send you account related emails.
Already on GitHub? Sign in to your account
Error: "array_row" not found during SME #219
Comments
Can you write the anndata object and send me?
Anyways, it's holiday now but I will check it ASAP. |
Hi,
Commands used to get A1_count_matrix.csv and A1_spatialCoords.csv
`count_matrix = t(counts(data))
spCoords = as.data.frame(spatialCoords(data))
array_coords = colData(data)[2:3]
spatial = cbind(spCoords, array_coords)
names(spatial)[1:2] = c("imagecol", "imagerow")`
These are the commands run to import the data.
`count_matrix = pd.read_csv("A1_count_matrix.csv")
xy = pd.read_csv("A1_spatialCoords.csv")
adata = st.create_stlearn(count=count_matrix,spatial=xy,library_id="A48_A1",
image_path="tif/WSA_LngSP10193345.tif",scale=1,background_color="white")`
The `adata.write_h5ad("adata_object.h5ad", compression="gzip")` result is: [https://res-geo.cdn.office.net/assets/mail/file-icon/png/generic_16x16.png] adata_object.h5ad<https://uwprod-my.sharepoint.com/:u:/g/personal/blett_wisc_edu/Eajcx9wAG4FHgjbDuy4sflwBfDAeUdV7p4R6Lgq1XsLALw>
By adding the following after building the stlearn object, it seems to fix the issue.
`adata.obs['array_row']=xy.iloc[:,2]
adata.obs['array_col']=xy.iloc[:,3]`
Though if there is a better way to import the data from R SpatialExperiment object that would be advantageous to learn about.
Thanks and happy holidays!
*******************************************************************
Beth M. Lett, Ph.D.
Postdoctoral Trainee fellow - ERP | Ong Lab
School of Medicine and Public health (SMPH), ADMIN,
& Endocrinology and Reproductive Physiology (ERP)
Pronouns: She, Her, Hers
Office Address:
2778 WIMR West
1111 Highland Ave
Madison, WI 53705
…________________________________
From: Duy Pham ***@***.***>
Sent: Thursday, December 29, 2022 7:56 PM
To: BiomedicalMachineLearning/stLearn ***@***.***>
Cc: BETH LETT ***@***.***>; Author ***@***.***>
Subject: Re: [BiomedicalMachineLearning/stLearn] Error: "array_row" not found during SME (Issue #219)
Can you write the anndata object and send me?
adata.write_h5ad("adata_object.h5ad")
Anyways, it's holiday now but I will check it ASAP.
—
Reply to this email directly, view it on GitHub<#219 (comment)>, or unsubscribe<https://github.com/notifications/unsubscribe-auth/AH4C24W2XNZ72HK3CPJ5RDTWPY6ODANCNFSM6AAAAAATLPDXRE>.
You are receiving this because you authored the thread.Message ID: ***@***.***>
|
Good to know this issue is solved. We will try to make a function to convert from R objects like SpatialExperiment or SeuratObject in the near future. Thanks for suggestion |
There seems to be additional issues with using the create.stlearn for downstream functions. When trying to run the st.spatial.trajectory.pseudotime. When I run it on the above data it hits an error: However when I use Read10x this error does not happen. I compared the two anndata objects and noticed it stores different values in the .uns['spatial'] section. I am not sure of a good way to fix this as there are qc steps in R I wish to run before preforming clustering. Thanks for developing this tool. I am hoping something comes from this thread soon: theislab/zellkonverter#61 to create a nice way to convert between similar to singlecell. |
In this step, there is a parameter to define the distance between every adjacent nodes:
|
Hi - Thanks for the reply. I tried various values in the eps (2,18,50,100) and all values returned the same error for the data load using create.stlearn. I even tried two different 10x genomic datasets. When I switched to the Read10x method used in the trajectory example that worked. I looked at the one 10x genomics dataset annData when load using create.stlearn and Read10x. This is the first few lines of the spatial adata.uns file between the two loading methods. create.stlearn Read10x The other key difference is in the scalefactors: create.stlearn Read10x It makes sense to me that the Read10x method would have more information since the create.stlearn is only provided a base amount information. Does the fact that the create.stlearn version being whole numbers imply that the eps value needs to be higher? |
Basically, the difference is about the spatial information scale. The Visium data provided all the informatio like scalefactors. In the In downstream analysis, we use those spatial information to construct the neighborhood array for each spot/cell and also the input for the local clustering (using spatial data only) by using DBSCAN. The |
Thank you for that explanation!! By adding the scale information during creation, I was able to get the data to run through Again, thank you so much for answering my questions!! |
It will be used in the CCI prediction part if you want to calculate the distance for neighborhood spots automatically
Otherwise, it's not so important. Also, it only be useful when you use the Visium or any platform that have constant distance between spots. Hope it helps |
Good morning. I get the below error when attempting to run the st.spatial.SME.SME_normalize command.
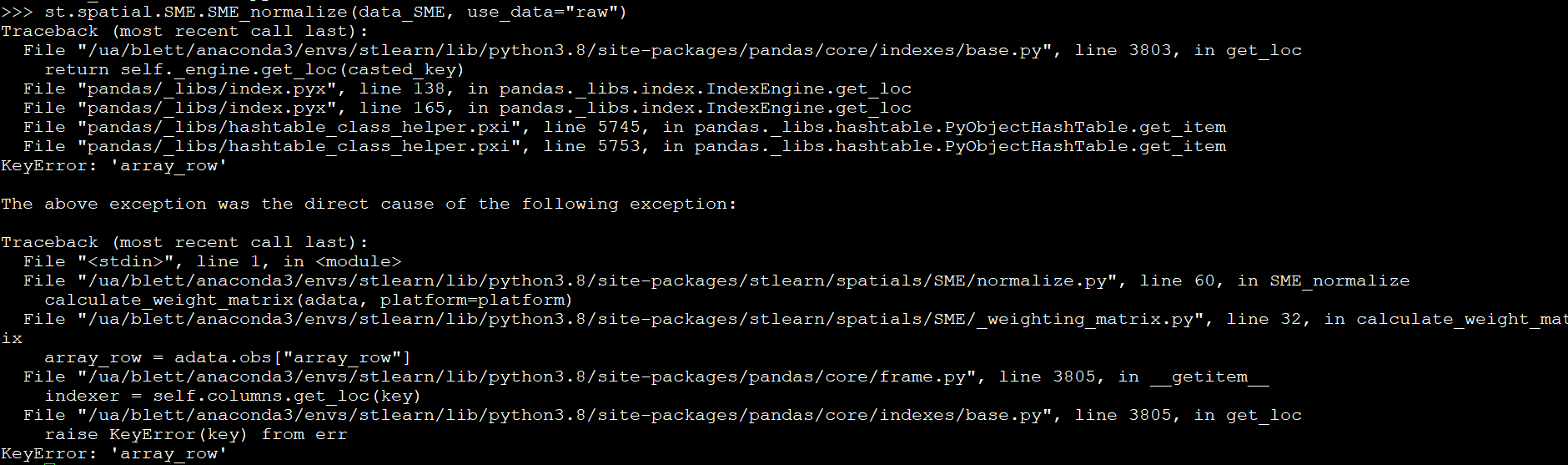
I used create_stlearn to import data where the counts_matrix is from a spatial experiment object counts(spe) command and the spatial data is from as.data.frame(spatialCoords(spe)). I even attempted to add the array_col and array_row data from my SpatialExperiment obje to the spatialcoordinates file assuming this was the issue and still obtain the above error.
Thanks
The text was updated successfully, but these errors were encountered: