-
Notifications
You must be signed in to change notification settings - Fork 1.4k
New issue
Have a question about this project? Sign up for a free GitHub account to open an issue and contact its maintainers and the community.
By clicking “Sign up for GitHub”, you agree to our terms of service and privacy statement. We’ll occasionally send you account related emails.
Already on GitHub? Sign in to your account
reduction window is vital for the model to pick up alignment. #280
Comments
Read up a bit on your implementation and it seems very promising. Going to give it a go with a fork I've been working on that is struggling to learn attention fully. I was looking into applying something similar (but not nearly as elegant) myself. Can you provide a link to the paper you reference in your fork's README? |
Hi, the paper is available at https://arxiv.org/abs/1909.01145 |
@bfs18 Hi, tried your fork but somehow I am getting NaN's on gradient.norm and mi loss, any ideas? I trained master successfully with the same data. |
Hi @onyedikilo
|
@bfs18 Sorry I couldn't understand what you meant with
Can you explain it in different words? |
Hi @onyedikilo
When setting |
@bfs18 |
Hi @hadaev8 , this line has no influence on the numerical values of gradients. When calculating taco_loss, the variables of CTC recognizer is not used, so the gradients of taco_loss with respect to these variables are None. The gradients become zero tensors after adding this line. |
I can confirm that the alignment picks up significantly faster with my data set. |
Hi @onyedikilo , thanks a lot for your confirmation. |
@bfs18 |
Hi @hadaev8 , This is caused by that the CTC loss is over weighted. When CTC loss is over weighted, the model would depend more on the text input to reduce the total loss. It leads to a diagonal alignment combined with the Local Sensitive Attention. Setting |
Well, I read again paper
Should you point where should be this lstm layer? |
Hi, the paper uses a internal Tensorflow implementation. It is a bit different from the open-sourced fork. In the open-sourced fork a ff_layer with relu activation is used to mix the information. It is this line https://github.com/bfs18/tacotron2/blob/8f8605ee0f67f6f571e74725030f16b13e4c7d2d/model.py#L388 |
Finally got around to trying out your fork on my modified spectrums and I can confirm it picked up attention much faster! Thanks! |
@bfs18 |
Hi @hadaev8
yes.
I just use the same dimension as the decoder_rnn_dim, of which the value is 1024. |
Hi @xDuck , I am glad to hear that. |
@bfs18 |
Hi @hadaev8 , |
@bfs18 My gradients indeed suffer. |
Hi @hadaev8 , The paper is bit complicated. I haven't go through it.
|
Hey @bfs18 Just wanted to let you know your fork is working great with my GST adaption as well based on Google's GST paper. Alignment learning super quickly and my models produce recognizable speech in about 3 hours on a 2070 Graphics card - way faster than before. |
@rafaelvalle I should mention I am using bark-scale spectrograms with 18 channels and 2 pitch features for my spectrograms along with a lpcnet-forked vocoder (Targetting faster than realtime CPU inference. Currently 1/3 realtime speed on a 2017 macbook pro for synthesis). I have noticed in general that speeds up training a lot too (less features to predict). Samples attached of her after not much training with different GST reference clips. Single-Speaker LJSpeech used - These are from my very first test. |
Hi @xDuck , thanks for your information. |
Hi @rafaelvalle , setting the teacher forcing input to the global mean to a certain percentage is a stable trick, which boosts up alignment learning a lot. The extra CTC loss would speed up alignment learning and reduce bad case. However, it is a bit tricky to tune. |
Hi @hadaev8
How did you solve your problem?
I don't quite get what are you trying to say. I guess you are saying the tail of the alignment is different form the above figures. It's a bit wired. I am also wondering. However, the padding frames are not important. |
I turn off ctc loss then it became too low. |
@chazo1994 I had not heard of this paper before, though reading it, very interesting indeed! In regards to your problem, I don't fully understand (I still consider myself new to Deep Learning so not too useful), but I will try to help with parts I do understand. I've also heard guided attention from espnet a few times, though looking further, I believe they just Diagonal-Guided Attention. Maybe explore FastSpeech/ForwardTacotron |
I would try smaller dropout but over-smoothed mel spectrogram and horizontal line noise is big problems. |
@bfs18 |
I report my results with MMI and DFR: Drop Frame Rate = 0 Drop Frame Rate = 0.1 Drop Frame Rate = 0.2 34k_Step gaf is nan after 30k step (I modified code to train model with mixed_precision). |
@bfs18 |
Hi @chazo1994 It seems that numerical errors occurred in your running.
I found gradient adaptive factor works better. I use that trick instead. |
@bfs18 |
@chazo1994 thank you for sharing this, can you share spectrogram reconstruction training and validation loss? |
This is my validation loss with MMI and DFR=0.2. |
@bfs18 Just trying out your fork for the first time and followed instructions in this thread with ljspeech pretrained model. Running into the following error. Any idea why?
Appreciate any feedback. |
you cannot use the pretrained Tacotron2 model of this branch. The model structure has been modified. |
@bfs18 I am using your fork with my dataset and it just started to align, but I am facing some problems when I try to use inference.ipynb with the tacotron model trained with your fork and the waveglow model; but, when I use this very same waveglow model that I have mentioned with the tacotron model trained with the NVidia repository, I have no problem. The problem is this one: AttributeError: 'WN' object has no attribute 'cond_layer' If I am not wrong, the convert_model.py (from WaveGlow) should be used in this case, right? I have used it, but this error persists. I need to use WaveGlow. |
@titocaco |
@CookiePPP it works! Thank you! =) |
FP16 Run: False When i run your code https://github.com/bfs18/tacotron2 , i meet this bug,Can you give me some suggestions? |
Can lpcnet help me with this issue: #463? |
Yes, Tacotron 2 + LPCNet should get you to be able to perform inference on
CPU but the best speeds I was able to achieve were about 2x real-time on a
current gen intel CPU with AVX2 support.
…On Wed, Mar 24, 2021 at 6:06 AM Erfolgreich charismatisch < ***@***.***> wrote:
I should mention I am using bark-scale spectrograms with 18 channels and 2
pitch features for my spectrograms along with a lpcnet-forked vocoder
Can lpcnet help me with this issue: #463
<#463>?
—
You are receiving this because you were mentioned.
Reply to this email directly, view it on GitHub
<#280 (comment)>,
or unsubscribe
<https://github.com/notifications/unsubscribe-auth/ABICRIOQ52NG47IWO5X6C7DTFG2RTANCNFSM4JIESUGQ>
.
|
Interesting. How do you use your nvidia Tacotron2 model with LPCNet?
|
You will have to adjust the number of mels (and maybe other Params) used
and feed it bark spectrograms for training from scratch. I made a lot of
modifications that I don’t really remember, but it is not a simple task.
On Wed, Mar 24, 2021 at 7:08 AM Erfolgreich charismatisch <
***@***.***> wrote:
… Interesting. How do you use your nvidia Tacotron2 model with LPCNet?
Yes, Tacotron 2 + LPCNet should get you to be able to perform inference on
CPU but the best speeds I was able to achieve were about 2x real-time on a
current gen intel CPU with AVX2 support.
… <#m_7142798940015613202_>
On Wed, Mar 24, 2021 at 6:06 AM Erfolgreich charismatisch < *@*.***>
wrote: I should mention I am using bark-scale spectrograms with 18 channels
and 2 pitch features for my spectrograms along with a lpcnet-forked vocoder
Can lpcnet help me with this issue: #463
<#463> <#463
<#463>>? — You are receiving
this because you were mentioned. Reply to this email directly, view it on
GitHub <#280 (comment)
<#280 (comment)>>,
or unsubscribe
https://github.com/notifications/unsubscribe-auth/ABICRIOQ52NG47IWO5X6C7DTFG2RTANCNFSM4JIESUGQ
.
—
You are receiving this because you were mentioned.
Reply to this email directly, view it on GitHub
<#280 (comment)>,
or unsubscribe
<https://github.com/notifications/unsubscribe-auth/ABICRIJN3URWMU7W455TFCDTFHBZPANCNFSM4JIESUGQ>
.
|
If you had to do it all over again, how would you start? PS: Can you share a diff between your files and the vanilla files?
|
I’ve already mostly abandoned the project after considering my research
“completed”. I do not have the diff accessible anymore, sorry. As for doing
it over again, now there are better alternatives like SqueezeWave, HiFi
GAN, etc. Keep in mind you will trade quality for speed in the vocoders, it
is hard to compare to the quality of WaveGlow.
This project was not designed to run on the CPU (rightfully so, NVIDA makes
GPUs not CPUs), so it might not be what you are looking for - but it does a
damn good job on GPUs.
…On Wed, Mar 24, 2021 at 7:22 AM Erfolgreich charismatisch < ***@***.***> wrote:
Well, that does not exactly sound encouraging ;)
If you had to do it all over again, how would you start?
PS: Can you share a diff between your files and the vanilla files?
You will have to adjust the number of mels (and maybe other Params) used
and feed it bark spectrograms for training from scratch. I made a lot of
modifications that I don’t really remember, but it is not a simple task.
—
You are receiving this because you were mentioned.
Reply to this email directly, view it on GitHub
<#280 (comment)>,
or unsubscribe
<https://github.com/notifications/unsubscribe-auth/ABICRIMYDWUMCMRV2SXQ7R3TFHDQ3ANCNFSM4JIESUGQ>
.
|
Yes. Which setup would you recommend for my goal? EDIT: I just tried SqeezeWave, but Nvidia is in it yet again, this time in apex. Therefore I get
|
I cannot give you a good answer without knowing everything about what you
want to do. I suggest you do some research and evaluate your options in
your setup.
…On Wed, Mar 24, 2021 at 8:18 AM Erfolgreich charismatisch < ***@***.***> wrote:
Yes. Which setup would you recommend for my goal?
I’ve already mostly abandoned the project after considering my research
“completed”. I do not have the diff accessible anymore, sorry. As for doing
it over again, now there are better alternatives like SqueezeWave, HiFi
GAN, etc. Keep in mind you will trade quality for speed in the vocoders, it
is hard to compare to the quality of WaveGlow. This project was not
designed to run on the CPU (rightfully so, NVIDA makes GPUs not CPUs), so
it might not be what you are looking for - but it does a damn good job on
GPUs.
—
You are receiving this because you were mentioned.
Reply to this email directly, view it on GitHub
<#280 (comment)>,
or unsubscribe
<https://github.com/notifications/unsubscribe-auth/ABICRIPOE47XMZXCJKNJ243TFHKALANCNFSM4JIESUGQ>
.
|
Tutorial: Training on GPU with Colab, Inference with CPU on Server here. |
hey guys, I just published the comprehensive tacotron2 which includes reduction factor (reduction window) and other techniques to boost up model robustness and efficiency. also you can play around with the pre-trained models. check the following link: |
Hi, can someone pls explain me what are the x and y axis of Mel spectrogram. And how is it different from alignment graph of x and y coordinates. Thanks! |
There's a implementation with FP16? |
The hparams.py says
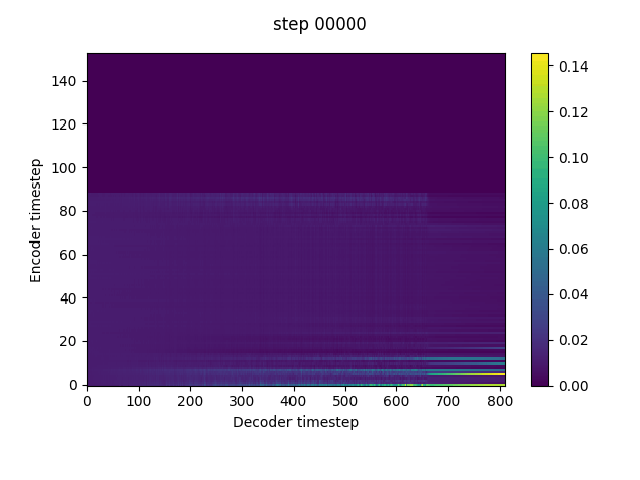
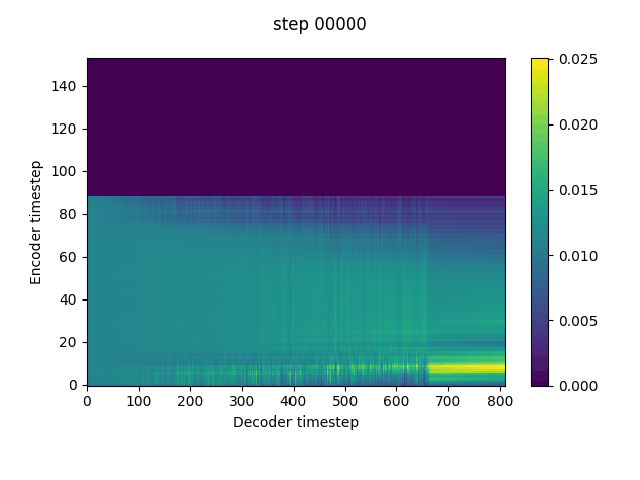
n_frames_per_step=1, # currently only 1 is supported
, but reduction window is very important for them model to pick up alignment. Using a reduction window can be considered as dropping teacher forcing frames at equal intervals, and thus increases the information gap between the teacher forcing input and the target. Tacotron2 tends to predict the target from the autoregressive input (teacher forcing input at training) without exploiting the conditional text if the information gap is not large enough.The reduction window can be replaced by a frame dropout trick if it is not continent to implement in the current code. Just set the teacher forcing input frames to the global mean according to a certain percentage.
In implement this in my fork. It can pick up alignment at much earlier steps without warmstart.
my fork
NVIDIA-tacotorn2
The text was updated successfully, but these errors were encountered: