Go to https://snowflake.net/ and register.
-- Use an admin role
USE ROLE ACCOUNTADMIN;
-- Create the `transform` role
CREATE ROLE IF NOT EXISTS transform;
GRANT ROLE TRANSFORM TO ROLE ACCOUNTADMIN;
-- Create the `dbt` user and assign to role
CREATE USER IF NOT EXISTS dbt
PASSWORD='dbtPassword123'
LOGIN_NAME='dbt'
MUST_CHANGE_PASSWORD=FALSE
DEFAULT_WAREHOUSE='COMPUTE_WH'
DEFAULT_ROLE='transform'
DEFAULT_NAMESPACE='AIRBNB.RAW'
COMMENT='DBT user used for data transformation';
GRANT ROLE transform to USER dbt;
-- Create our database and schemas
CREATE DATABASE IF NOT EXISTS AIRBNB;
CREATE SCHEMA IF NOT EXISTS AIRBNB.RAW;
-- Set up permissions to role `transform`
GRANT ALL ON WAREHOUSE COMPUTE_WH TO ROLE transform;
GRANT ALL ON DATABASE AIRBNB to ROLE transform;
GRANT ALL ON ALL SCHEMAS IN DATABASE AIRBNB to ROLE transform;
GRANT ALL ON FUTURE SCHEMAS IN DATABASE AIRBNB to ROLE transform;
GRANT ALL ON ALL TABLES IN SCHEMA AIRBNB.RAW to ROLE transform;
GRANT ALL ON FUTURE TABLES IN SCHEMA AIRBNB.RAW to ROLE transform
-- Set up the defaults
USE WAREHOUSE COMPUTE_WH;
USE DATABASE airbnb;
USE SCHEMA RAW;
-- Create our three tables and import the data from S3
CREATE OR REPLACE TABLE raw_listings
(id integer,
listing_url string,
name string,
room_type string,
minimum_nights integer,
host_id integer,
price string,
created_at datetime,
updated_at datetime);
COPY INTO raw_listings (id,
listing_url,
name,
room_type,
minimum_nights,
host_id,
price,
created_at,
updated_at)
from 's3://dbtlearn/listings.csv'
FILE_FORMAT = (type = 'CSV' skip_header = 1
FIELD_OPTIONALLY_ENCLOSED_BY = '"');
CREATE OR REPLACE TABLE raw_reviews
(listing_id integer,
date datetime,
reviewer_name string,
comments string,
sentiment string);
COPY INTO raw_reviews (listing_id, date, reviewer_name, comments, sentiment)
from 's3://dbtlearn/reviews.csv'
FILE_FORMAT = (type = 'CSV' skip_header = 1
FIELD_OPTIONALLY_ENCLOSED_BY = '"');
CREATE OR REPLACE TABLE raw_hosts
(id integer,
name string,
is_superhost string,
created_at datetime,
updated_at datetime);
COPY INTO raw_hosts (id, name, is_superhost, created_at, updated_at)
from 's3://dbtlearn/hosts.csv'
FILE_FORMAT = (type = 'CSV' skip_header = 1
FIELD_OPTIONALLY_ENCLOSED_BY = '"');
I'll use WSL to install DBT, alias created "winhome".
curl -o get-pip.py https://bootstrap.pypa.io/get-pip.py
python get-pip.py
pip install virtualenv
virtualenv venv
source venv/bin/activate
pip list --format=columns
pip install dbt-snowflake
dbt init airbnb
dbt config --provider snowflake --connect "account=dbt;warehouse=COMPUTE_WH;database=AIRBNB;schema=AIRBNB.RAW"
cat ~/.dbt/profiles.yml
dbt debug
WITH raw_listings AS (
SELECT * FROM AIRBNB.RAW.RAW_LISTINGS
)
SELECT
id AS listing_id,
name AS listing_name,
listing_url,
room_type,
minimum_nights,
host_id,
price AS price_str,
created_at,
updated_at
FROM
raw_listings
dbt run
WITH raw_reviews AS (
SELECT * FROM AIRBNB.RAW.RAW_REVIEWS
)
SELECT
listing_id,
date AS review_date,
reviewer_name,
comments as review_text,
sentiment as review_sentiment
FROM
raw_reviews
dbt run
WITH raw_hosts AS (
SELECT * FROM AIRBNB.RAW.RAW_HOSTS
)
SELECT
ID AS HOST_ID,
NAME AS HOST_NAME,
IS_SUPERHOST,
CREATED_AT,
UPDATED_AT
FROM RAW_HOSTS
dbt run
WITH src_listings AS (
SELECT * FROM {{ref('src_listings')}}
)
SELECT
listing_id,
listing_name,
room_type,
CASE
WHEN minimum_nights = 0 THEN 1
ELSE minimum_nights
END AS minimum_nights,
host_id,
REPLACE(
price_str,
'$'
) :: NUMBER(
10,
2
) AS price,
created_at,
updated_at
FROM src_listings
WITH src_hosts AS (
SELECT * FROM {{ref('src_hosts')}}
)
SELECT
HOST_ID,
CASE
WHEN HOST_NAME IS NOT NULL THEN HOST_NAME
ELSE NVL(HOST_NAME,'Anonymous')
END AS HOST_NAME,
IS_SUPERHOST,
CREATED_AT,
UPDATED_AT
FROM src_hosts
models:
dbtlearn:
+materialized: view
dim:
+materialized: table
SELECT * FROM "AIRBNB"."DEV"."FCT_REVIEWS" WHERE listing_id=3176;
INSERT INTO "AIRBNB"."RAW"."RAW_REVIEWS"
VALUES (3176, CURRENT_TIMESTAMP(), 'Zoltan', 'excellent stay!', 'positive');
dbt run --full-refresh
WITH
l AS (
SELECT
*
FROM
{{ ref('dim_listings_cleansed') }}
),
h AS (
SELECT *
FROM {{ ref('dim_hosts_cleansed') }}
)
SELECT
l.listing_id,
l.listing_name,
l.room_type,
l.minimum_nights,
l.price,
l.host_id,
h.host_name,
h.is_superhost as host_is_superhost,
l.created_at,
GREATEST(l.updated_at, h.updated_at) as updated_at
FROM l
LEFT JOIN h ON (h.host_id = l.host_id)
models:
dbtlearn:
+materialized: view
dim:
+materialized: table
src:
+materialized: ephemeral
DROP VIEW AIRBNB.DEV.SRC_HOSTS;
DROP VIEW AIRBNB.DEV.SRC_LISTINGS;
DROP VIEW AIRBNB.DEV.SRC_REVIEWS;
{{
config(
materialized = 'view'
)
}}
dbt seed
dbt compile
loaded_at_field: date
freshness:
warn_after: {count: 1, period: hour}
error_after: {count: 24, period: hour}
dbt source freshness
Snowflake's zero-copy cloning feature provides a convenient way to quickly take a “snapshot” of any table, schema, or database and create a derived copy of that object which initially shares the underlying storage.
dbt snapshot
UPDATE AIRBNB.RAW.RAW_LISTINGS SET MINIMUM_NIGHTS=30,
updated_at=CURRENT_TIMESTAMP() WHERE ID=3176;
SELECT * FROM AIRBNB.DEV.SCD_RAW_LISTINGS WHERE ID=3176;
Generic tests: Create source.yml (you can create whatever you want) where you can specify the tests that you want to run:
version: 2
models:
- name: dim_listings_cleansed
columns:
- name: listing_id
tests:
- unique
- not_null
dbt test
code/cat target/compiled/dbtlearn/models/{path_to_test.yml}/{test_name}
with all_values as (
select
room_type as value_field,
count(*) as n_records
from airbnb.dev.dim_listings_cleansed
group by room_type
)
select *
from all_values
where value_field not in (
'Entire home/apt - break this','Private room','Shared room','Hotel room'
)
dbt test --select dim_listings_cleansed
% macro no_nulls_in_columns(model) %}
SELECT * FROM {{ model }} WHERE
{% for col in adapter.get_columns_in_relation(model) -%}
{{ col.column }} IS NULL OR
{% endfor %}
FALSE
{% endmacro %}
no_nulls_in_columns('dim_listings_cleansed')}}
{% test positive_value(model, column_name) %}
SELECT
*
FROM
{{ model }}
WHERE
{{ column_name}} < 1
{% endtest %}
- name: minimum_nights
tests:
- positive_values
dbt test
Visit Hub GetDBT
Include the following in your packages.yml file:
packages:
- package: dbt-labs/dbt_utils
version: 0.8.6
Run dbt deps
to install the package.
{{ dbt_utils.surrogate_key(['listing_id', 'review_date', 'reviewer_name', 'review_text']) }}
dbt run --full-refresh --select fct_reviews
Documentations can be defined two ways:
- In yaml files(like schema.yml)
- In standole markdown files
Dbt ships with a lightweight documentation web server
For customizing the landing page, a special file, overview.md is used
You can add your own assets (like images) to a special project folder
dbt docs generate
Open your browser and go to http://localhost:8000/docs/
dbt docs serve
{% docs dim_listing_cleansed__minimum_nights %}
Minimum number of nights required to rent this property.
Keep in mind that old listings might have `minimum_nights` set
to 0 in the source tables. Our cleansing algorithm updates this to `1`.
{% enddocs %}
{% docs __overview__ %}
# Airbnb pipeline
Hey, welcome to our Airbnb pipeline documentation!
Here is the schema of our input data:
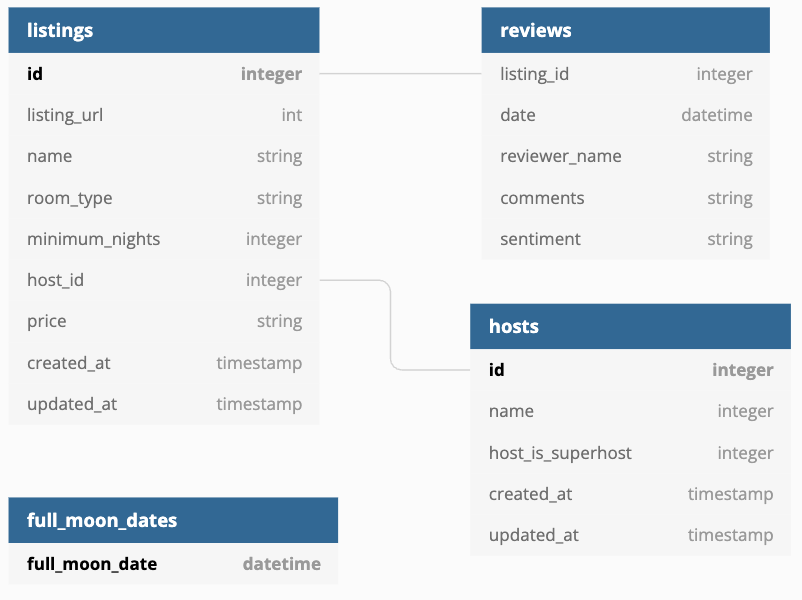
{% enddocs %}
Dbt's notion of models makes it easy for data teams to version control and collaborate on data transformations. Sometimes though, a certain sql statement doesn't quite fit into the mold of a dbt model. These more "analytical" sql files can be versioned inside of your dbt project using the analysis functionality of dbt.
WITH mart_fullmoon_reviews AS (
SELECT * FROM ref('mart_fullmoon_reviews')
)
SELECT
is_full_moon,
review_sentiment,
COUNT(*) as reviews
FROM
mart_fullmoon_reviews
GROUP BY
is_full_moon,
review_sentiment
ORDER BY
is_full_moon,
review_sentiment
dbt compile
Hooks are snippets of SQL that are executed at different times:
-
pre-hook: executed before a model, seed or snapshot is built.
-
post-hook: executed after a model, seed or snapshot is built.
-
on-run-start: executed at the start of dbt run, dbt seed or dbt snapshot
-
on-run-end: executed at the end of dbt run, dbt seed or dbt snapshot
Hooks are defined in your dbt_project.yml file. Pre- and post-hooks can also be defined in a config block
SNOWFLAKE
USE ROLE ACCOUNTADMIN;
CREATE ROLE IF NOT EXISTS REPORTER;
CREATE USER IF NOT EXISTS PRESET
PASSWORD='presetPassword123'
LOGIN_NAME='preset'
MUST_CHANGE_PASSWORD=FALSE
DEFAULT_WAREHOUSE='COMPUTE_WH'
DEFAULT_ROLE='REPORTER'
DEFAULT_NAMESPACE='AIRBNB.DEV'
COMMENT='Preset user for creating reports';
GRANT ROLE REPORTER TO USER PRESET;
GRANT ROLE REPORTER TO ROLE ACCOUNTADMIN;
GRANT ALL ON WAREHOUSE COMPUTE_WH TO ROLE REPORTER;
GRANT USAGE ON DATABASE AIRBNB TO ROLE REPORTER;
GRANT USAGE ON SCHEMA AIRBNB.DEV TO ROLE REPORTER;
GRANT SELECT ON ALL TABLES IN SCHEMA AIRBNB.DEV TO ROLE REPORTER;
GRANT SELECT ON ALL VIEWS IN SCHEMA AIRBNB.DEV TO ROLE REPORTER;
GRANT SELECT ON FUTURE TABLES IN SCHEMA AIRBNB.DEV TO ROLE REPORTER;
GRANT SELECT ON FUTURE VIEWS IN SCHEMA AIRBNB.DEV TO ROLE REPORTER;
+post-hook:
- "GRANT SELECT ON {{ this }} TO ROLE REPORTER"
cat ~/.dbt/profiles.yml
Exposures make it possible to define and describe a downstream use of your dbt project, such as in a dashboard, application, or data science pipeline. By defining exposures, you can then:
- run, test, and list resources that feed into your exposure
- populate a dedicated page in the auto-generated documentation site with context relevant to data consumers
Declaring an exposure
Exposures are defined in .yml files nested under an exposures: key.
dbt docs generate
and dbt docs serve