-
Notifications
You must be signed in to change notification settings - Fork 246
New issue
Have a question about this project? Sign up for a free GitHub account to open an issue and contact its maintainers and the community.
By clicking “Sign up for GitHub”, you agree to our terms of service and privacy statement. We’ll occasionally send you account related emails.
Already on GitHub? Sign in to your account
If I don't want use bias on Conv2D, What can i do? #80
Comments
Hi passiduck, |
Thanks for the quick reply. |
The |
Hi @majianjia For support of legacy models, would it be possible to add support for having a no bias in the Conv Layer, or alternatively if their is a hacky way to implement the same, could you please give pointers. Thanks. |
nnom doesn't support bias because the backends must have one to work properly currently. You must add it somewhere. There are 2 ways to do it.
Hope it helps |
Thanks for the pointers.
Then just for cleaning the flow , I also introduced a bias_initializer as "random_normal", so that the max_val, min_val don't reduce to 0 for the bias layers. However, then I got the following error :
Trying to figure out the reason for the above. |
It appears that nnom uses the name of the layers instead of the instance type. In the case that the layers have a different nomenclature, then the bias_shift variable doesn't get assigned. |
Currently fails to generate a model File "../nnom-models/auto_test/venv/lib/python3.11/site-packages/nnom/nnom.py", line 731, in quantize_weights f.write('#define ' + layer.name.upper() + '_BIAS_LSHIFT '+to_cstyle(bias_shift) +'\n\n') ^^^^^^^^^^ UnboundLocalError: cannot access local variable 'bias_shift' where it is not associated with a value MobileNet does not use bias for Conv layers. Currently that is not supported in NNoM, and might be the reason of this error. Ref majianjia/nnom#80
I have tried a lot of time for using bias on Conv2d.(take weights at ckpt trained with tensorflow_1.15)

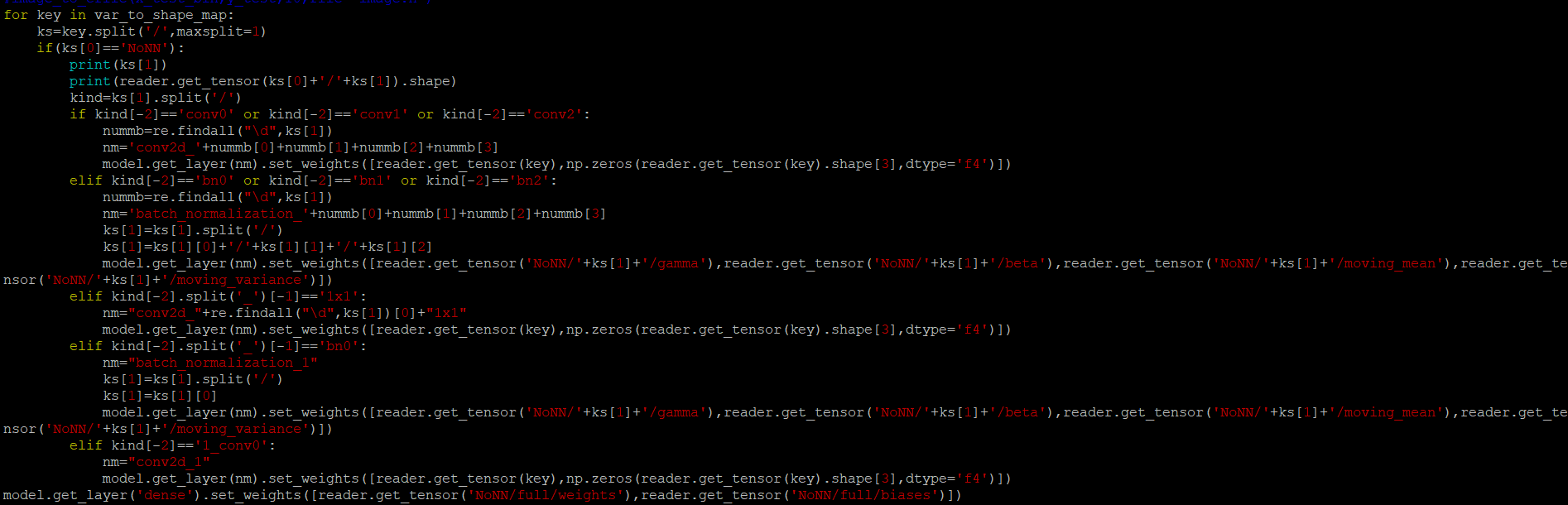
(very dirty code....sorry)
First I changed nnom_utils.py. Erased all c_b line, and Changed layer.set_weights([c_w]).
And Changed related Length of convolution layer.
Twice I added zeros bias, But np.log2 functions make error.
So I added if statement like 'if min_value'==0: min_value=1e-36'
Both ways make this problem.
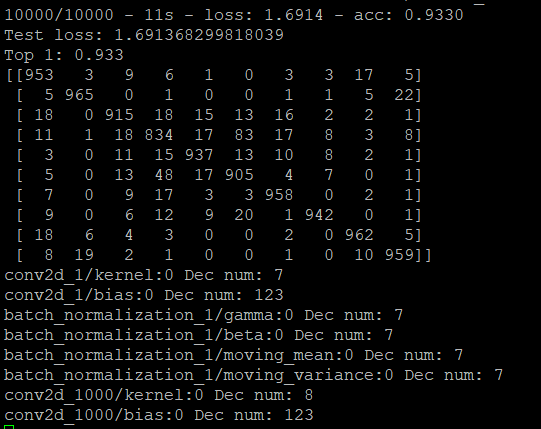
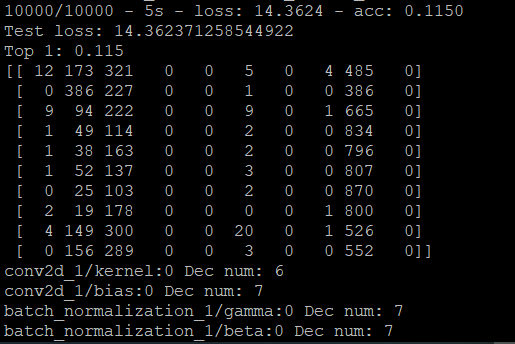
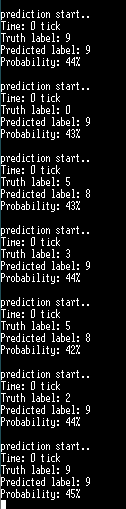
The First evaluate_model function works fine
But if I do the generate_model function and then do the evaluate function again, the evaluate_model function doesn't work fine.
And I'll show you a picture of the results on the board.
Thank you for reading the long article, and I apologize for terrible English and coding skills.
Safe Safety.
The text was updated successfully, but these errors were encountered: