The goal of this repository is to supply a simple mechanism for deploying and setting up a Darknet environment (Darknet repository). The button below deploys to an Ubuntu-based Data Science VM (NC-series) to Azure and installs the relevant dependencies.
NOTE: An Azure subscription is required - see below.
Azure subscription required.
Hardware compute fees apply. Free Trial available for new customers.
IMPORTANT NOTE: Before you proceed to use the Deploy to Azure button you must perform a one-time task to accept the terms of the data science virtual machine on your Azure subscription. You can do this by visiting Configure Programmatic Deployment
Just click this button (see below for details).
IMPORTANT NOTE: Due to JupyterHub requirements, keep your admin name for this VM all lowercase.
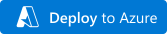
The default option (NC6
) is the cheapest GPU option, but it's also the oldest and slowest. Other GPU-enabled vmSizes are available from the template, but please see the notes in the vm sizes documentation.
Specifically note that the vCPU
core quota for _v2
and _v3
series VMs is initially set to 0 in each region for every subscription. You must request a vCPU quota increase for these families in an available region. If you choose one of the NCXX_v2
or NCXX_v3
VM sizes in the template without increasing your quota, the deployment WILL FAIL.
You define the name of a resource group, and the following services get deployed to that resource group:
- An Ubuntu-based Data Science VM with your defined user name and password.
- Storage: A storage account and 2 disks for storing data
- A network interface, virtual network, and public IP address
When you click on the Deploy to Azure
button above, a custom template will launch in the Azure portal that asks you for some information:
- Subscription you are creating the resources in
- Resource group you want to create all the resources in
- Location (i.e. Data Center where the resource group and resources physically live)
- DNS label prefix
- Admin Username
- Admin Password
- VmSize (
NC6
as default; other NC options avail, see note above) - Vm Name
All of this information is controlled with the azuredeploy.json file in the root directory of this repository.
The resources are created, and then a custom script extension is used to download the dsvm-setup.sh script from this github repository and then run it. This script is run as root and takes a single argument: the name of the admin you specify in the portal.
It does the following:
- Builds Darknet on the Ubuntu DSVM
See the dsvm-setup.sh file for details.
You should test darknet. SSH into the VM. On Windows, you can do so using a tool such as PuTTY.
Note: If you have difficulty logging in (timeout etc.), you may need to navigate to the VM in the Azure Portal and go to Networking under Settings. There, delete the Cleanuptool-Deny-103 Inbound port rule. This will allow you to temporarily access the VM with SSH. You may need to repeat this in the future.
ssh <username>@<public IP or DNS name>
cd darknet
wget https://github.com/AlexeyAB/darknet/releases/download/darknet_yolo_v4_pre/yolov4-tiny.weights
./darknet detector test ./cfg/coco.data ./cfg/yolov4-tiny.cfg ./yolov4-tiny.weights
There are test images in the data
folder under the darknet
repository to try. The image is saved each time as predictions.jpg
and may be downloaded with the Unix scp
command or Windows SCP client.
scp <username>@<public IP or DNS name>:~/darknet/predictions.jpg .
- Based on: https://github.com/jreynolds01/fastaivm
- Original image source: https://github.com/AlexeyAB/darknet/tree/master/data