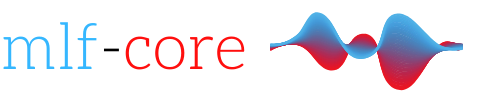
mlf-core: a framework for deterministic machine learning
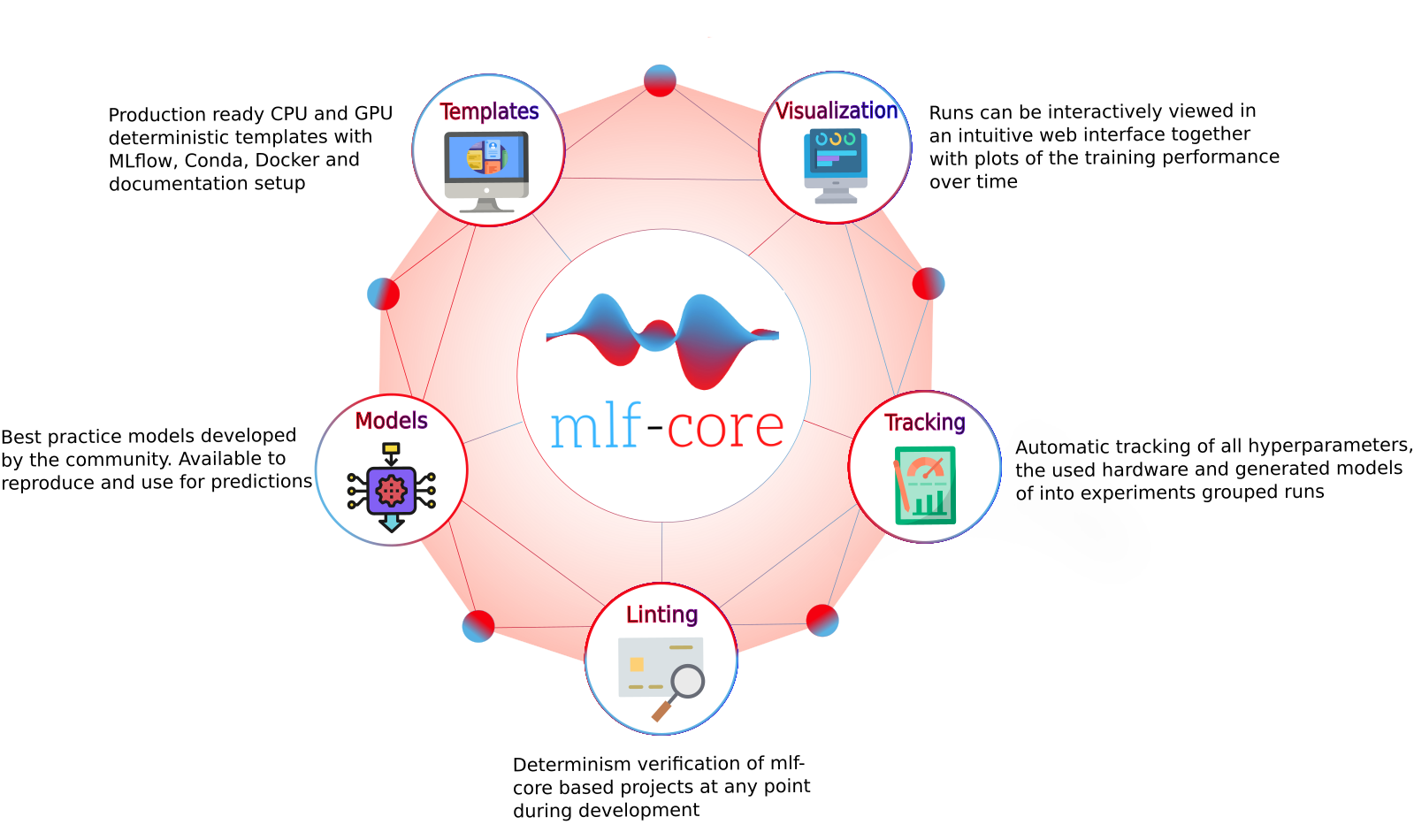
mlf-core provides CPU and GPU deterministic machine learning templates based on MLflow, Conda, Docker and a strong Github integration. Templates are available for PyTorch, TensorFlow and XGBoost. A custom linter ensures that projects stay deterministic in all phases of development and deployment.
Start your journey with mlf-core by installing it via $ pip install mlf-core
.
See Installation.
See a mlf-core project in action.
Configure mlf-core to get started.
List all available mlf-core templates.
Get detailed information on a mlf-core template.
See Get detailed template information.
Kickstart your deterministic machine laerning project with one of mlf-core's templates in no time.
See Create a project.
Use advanced linting to ensure your project always adheres to mlf-core's standards and stays deterministic.
Bump your project version across several files.
See Bumping the version of an existing project.
Sync your project with the latest mlf-core release to get the latest template features.
See Syncing a project.
Check whether you are using the latest mlf-core version and update automatically to benefit from the latest features.
See https://mlf_core.readthedocs.io/en/latest/upgrade.html.
Primary idea and main development by Lukas Heumos. mlf-core is inspired by nf-core. This package was created with cookietemple based on a modified audreyr/cookiecutter-pypackage project template using cookiecutter.