-
-
Notifications
You must be signed in to change notification settings - Fork 16.4k
New issue
Have a question about this project? Sign up for a free GitHub account to open an issue and contact its maintainers and the community.
By clicking “Sign up for GitHub”, you agree to our terms of service and privacy statement. We’ll occasionally send you account related emails.
Already on GitHub? Sign in to your account
Interpreting training results and showing loss graph, YOLOv5s6 #8185
Comments
👋 Hello @Carolinejone, thank you for your interest in YOLOv5 🚀! Please visit our ⭐️ Tutorials to get started, where you can find quickstart guides for simple tasks like Custom Data Training all the way to advanced concepts like Hyperparameter Evolution. If this is a 🐛 Bug Report, please provide screenshots and minimum viable code to reproduce your issue, otherwise we can not help you. If this is a custom training ❓ Question, please provide as much information as possible, including dataset images, training logs, screenshots, and a public link to online W&B logging if available. For business inquiries or professional support requests please visit https://ultralytics.com or email support@ultralytics.com. RequirementsPython>=3.7.0 with all requirements.txt installed including PyTorch>=1.7. To get started: git clone https://github.com/ultralytics/yolov5 # clone
cd yolov5
pip install -r requirements.txt # install EnvironmentsYOLOv5 may be run in any of the following up-to-date verified environments (with all dependencies including CUDA/CUDNN, Python and PyTorch preinstalled):
StatusIf this badge is green, all YOLOv5 GitHub Actions Continuous Integration (CI) tests are currently passing. CI tests verify correct operation of YOLOv5 training (train.py), validation (val.py), inference (detect.py) and export (export.py) on macOS, Windows, and Ubuntu every 24 hours and on every commit. |
@Carolinejone 👋 Hello! Thanks for asking about improving YOLOv5 🚀 training results. I would combine both your datasets into a single dataset for best results. Also you must train longer until you see overfitting, otherwise you have not trained long enough. Most of the time good results can be obtained with no changes to the models or training settings, provided your dataset is sufficiently large and well labelled. If at first you don't get good results, there are steps you might be able to take to improve, but we always recommend users first train with all default settings before considering any changes. This helps establish a performance baseline and spot areas for improvement. If you have questions about your training results we recommend you provide the maximum amount of information possible if you expect a helpful response, including results plots (train losses, val losses, P, R, mAP), PR curve, confusion matrix, training mosaics, test results and dataset statistics images such as labels.png. All of these are located in your We've put together a full guide for users looking to get the best results on their YOLOv5 trainings below. Dataset
Model SelectionLarger models like YOLOv5x and YOLOv5x6 will produce better results in nearly all cases, but have more parameters, require more CUDA memory to train, and are slower to run. For mobile deployments we recommend YOLOv5s/m, for cloud deployments we recommend YOLOv5l/x. See our README table for a full comparison of all models.
python train.py --data custom.yaml --weights yolov5s.pt
yolov5m.pt
yolov5l.pt
yolov5x.pt
custom_pretrained.pt
python train.py --data custom.yaml --weights '' --cfg yolov5s.yaml
yolov5m.yaml
yolov5l.yaml
yolov5x.yaml Training SettingsBefore modifying anything, first train with default settings to establish a performance baseline. A full list of train.py settings can be found in the train.py argparser.
Further ReadingIf you'd like to know more a good place to start is Karpathy's 'Recipe for Training Neural Networks', which has great ideas for training that apply broadly across all ML domains: http://karpathy.github.io/2019/04/25/recipe/ Good luck 🍀 and let us know if you have any other questions! |
@glenn-jocher Thank you so much for your explanation. I intentionally separate the dataset into two as I would like to compare the impact on the model's accuracy by the two different test datasets having different drone distances from the target object. For that reason, I couldn't combine the two datasets to make it larger. !python train.py --img 640 --batch 64 --epochs 300 --data {dataset.location}/data.yaml --weights yolov5s6.pt --cache Could you please explain how to prevent overfitting and if possible explain with some coding examples? |
@Carolinejone follow recommendations in our complete guide in #8185 (comment). To reduce overfitting you can try using higher augmentation yamls in data/hyps or customize with more Albumentations. YOLOv5 🚀 applies online imagespace and colorspace augmentations in the trainloader (but not the val_loader) to present a new and unique augmented Mosaic (original image + 3 random images) each time an image is loaded for training. Images are never presented twice in the same way. Augmentation HyperparametersThe hyperparameters used to define these augmentations are in your hyperparameter file (default
yolov5/data/hyps/hyp.scratch-low.yaml Lines 6 to 34 in b94b59e
Augmentation PreviewsYou can view the effect of your augmentation policy in your train_batch*.jpg images once training starts. These images will be in your train logging directory, typically
YOLOv5 Albumentations IntegrationYOLOv5 🚀 is now fully integrated with Albumentations, a popular open-source image augmentation package. Now you can train the world's best Vision AI models even better with custom Albumentations 😃! PR #3882 implements this integration, which will automatically apply Albumentations transforms during YOLOv5 training if Example Good luck 🍀 and let us know if you have any other questions! |
@glenn-jocher I can't thank you enough. You're my lifesaver. Your explanation is very effective and useful. I changed the model hyperparameters from low to high. Is it called fine-tuning? ( I admit that I had little knowledge about fine-tuning) |
Seems like it’s now overfitting less, and you can now train longer |
@glenn-jocher Thank you so much. Now I've trained the Dataset-1 for 600 epochs and it starts overfitting after 500 epochs. (the model also stopped showing improvements after 484 epochs which I got the best results). So should I stop at epoch 500? |
@Carolinejone results look good! |
@glenn-jocher Thank you so much. I'll update the other results after training all datasets with different epochs. Again, your kind guidance is deeply appreciated. |
👋 Hello, this issue has been automatically marked as stale because it has not had recent activity. Please note it will be closed if no further activity occurs. Access additional YOLOv5 🚀 resources:
Access additional Ultralytics ⚡ resources:
Feel free to inform us of any other issues you discover or feature requests that come to mind in the future. Pull Requests (PRs) are also always welcomed! Thank you for your contributions to YOLOv5 🚀 and Vision AI ⭐! |
@Carolinejone you're welcome! Feel free to reach out if you have any more questions. Good luck with your training, and I'm looking forward to seeing your results for the other datasets. Keep up the great work! |
@glenn-jocher |
@pratikshac15 in YOLOv5, the background class refers to the class that represents the absence of any object in the image. When calculating FN (False Negative), FP (False Positive), and TN (True Negative) from the confusion matrix, these metrics are typically considered with respect to the classes that are being detected, and the background class is not factored into these calculations. Thank you for your question! |
Hello, I'm new to AI. I would like to ask for some guidance on my research. I'm doing my master thesis with YOLOv5. I'm trying to detect the anomaly of stay cables of cable-type bridges.
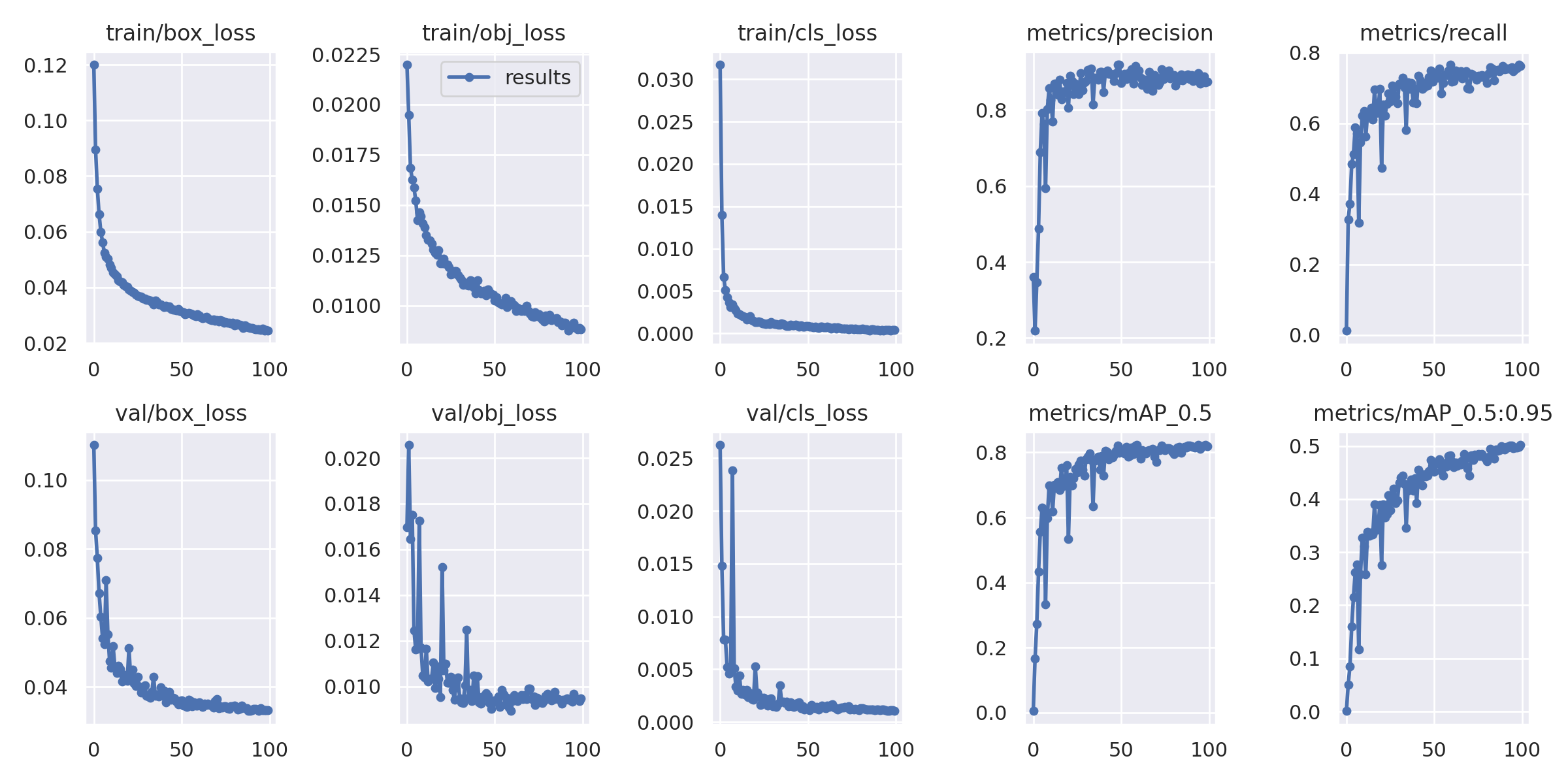
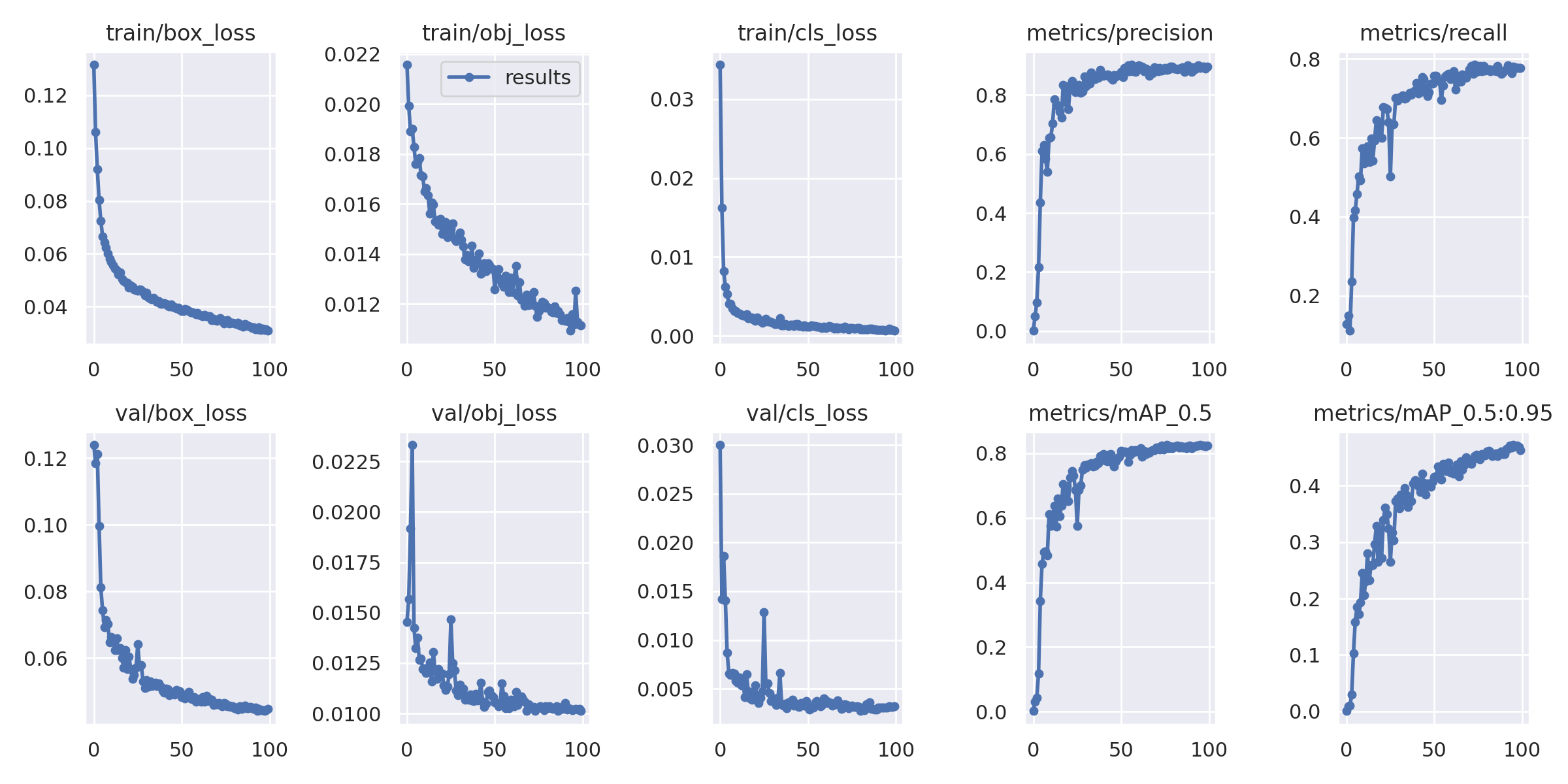
I used two datasets; Dataset- 1 has 2049 source images and 4159 images in total after combining with some augmented images to the training dataset, and Dataset- 2 has 1823 source images and 3673 images in total after combining with some augmented images to the training dataset. Train, validation and test dataset split is as follow,
Dataset -1, 3.2k: 614: 380
Dataset -2, 2.9k: 552: 299
I followed custom YOLOv5 training from Roboflow and also used the Roboflow annotator with rectangular bounding boxes. I used batch size 64 and epoch 100 with pre-trained weight YOLOv5s6 and trained on COLAB pro plus for both datasets and the rest is the same as the custom YOLOv5 training tutorial.
This is the result for Dataset-1
This is the result for Dataset-2
My question is,
Thank you so much for your help.
Edit: adding more questions:
Dataset-1 label graph
Dataset-2 label graph
Dataset-1 Class Balance
Dataset-2 Class Balance
The text was updated successfully, but these errors were encountered: